- Privacy Policy

Home » Intervening Variable – Definition, Types and Example
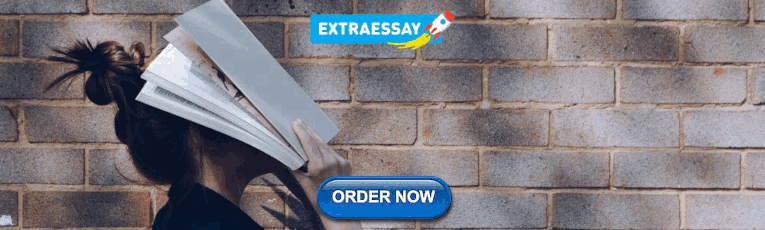
Intervening Variable – Definition, Types and Example
Table of Contents

Intervening Variable
Definition:
An intervening variable, also known as a mediating variable, is a hypothetical construct that explains the relationship between two other variables. It is a variable that comes between the independent variable (cause) and the dependent variable (effect) in a causal relationship, and helps to explain how the independent variable influences the dependent variable.
In other words, an intervening variable is a variable that exists between the independent variable and the dependent variable, and it helps to explain how the independent variable affects the dependent variable.
Types of Intervening Variable
There are two main types of intervening variables: mediating variables and moderating variables.
Mediating Variables
A mediating variable is an intervening variable that explains the relationship between an independent variable and a dependent variable. It occurs when the independent variable affects the mediating variable, which in turn affects the dependent variable. For example, in the relationship between smoking and lung cancer, the mediating variable could be the damage to the lungs caused by smoking. Smoking damages the lungs, which increases the likelihood of developing lung cancer.
Moderating Variables
A moderating variable is an intervening variable that affects the strength or direction of the relationship between an independent variable and a dependent variable. It occurs when the relationship between the independent variable and the dependent variable depends on the level of the moderating variable. For example, in the relationship between stress and performance, the moderating variable could be the level of social support. High levels of social support can moderate the negative effects of stress on performance, whereas low levels of social support can exacerbate those negative effects.
Examples of Intervening Variable
Here are some examples of intervening variables:
- Stress : Suppose you are investigating the relationship between workload and productivity in the workplace. However, you find that stress plays a mediating role in this relationship, meaning that as workload increases, so does stress, and as stress increases, productivity decreases.
- Education : Let’s say you are researching the effect of parental income on a child’s academic performance. Education could be an intervening variable because it plays a role in how income affects academic performance. Children from families with higher income levels may have access to better schools and resources, which can lead to better academic performance.
- Self-esteem : Suppose you are studying the relationship between bullying and mental health. Self-esteem could be an intervening variable because bullying can lower one’s self-esteem, which in turn can lead to depression and anxiety.
- Motivation : Let’s say you are investigating the effect of rewards on performance in the workplace. Motivation could be an intervening variable because rewards can increase motivation, which in turn can lead to higher performance levels.
- Social support : Suppose you are studying the effect of a new treatment for a chronic illness. Social support could be an intervening variable because individuals who receive social support may be more likely to adhere to the treatment regimen, which in turn can lead to better health outcomes.
Applications of Intervening Variable
Here are some applications of intervening variables in various fields:
- Psychology : In psychology, intervening variables are used to understand how a particular behavior is influenced by internal and external factors. For example, if researchers want to understand how stress affects academic performance, they may measure the level of stress (intervening variable) and its impact on academic performance.
- Education : In education, intervening variables can be used to understand how different teaching methods affect student learning. For example, if researchers want to understand how a particular teaching method improves student performance, they may measure the intervening variables, such as motivation or engagement, and their effect on academic achievement.
- Health : In health research, intervening variables can be used to understand how a particular intervention affects health outcomes. For example, if researchers want to understand how a new medication affects blood pressure, they may measure intervening variables such as heart rate or cholesterol levels to help explain how the medication works.
- Marketing : In marketing, intervening variables are used to understand how advertising and other marketing efforts affect consumer behavior. For example, if a company wants to understand how a new advertising campaign affects consumer purchasing decisions, they may measure intervening variables such as brand awareness or consumer attitudes towards the product.
- Economics : In economics, intervening variables can be used to understand how changes in one economic factor affect another. For example, if economists want to understand how changes in interest rates affect consumer spending, they may measure intervening variables such as income or savings rates to help explain the relationship between the two.
- Testing theories: Researchers may use intervening variables to test theories about how certain factors influence outcomes. For example, a researcher may hypothesize that social support mediates the relationship between stress and depression. By measuring social support as an intervening variable, the researcher can test whether the data supports the theory.
Purpose of Intervening Variable
The purpose of intervening variables is to provide a theoretical explanation for the relationship between the independent variable and the dependent variable. They help to explain how and why the independent variable affects the dependent variable by identifying the underlying mechanisms or processes that link the two variables.
By identifying an intervening variable, researchers can better understand the causal relationships between variables and can develop more accurate models and theories. Intervening variables also allow researchers to test the mechanisms through which the independent variable affects the dependent variable, providing insights into how interventions can be developed to bring about desired changes.
When to use Intervening Variable
Here are some situations where intervening variables may be useful:
- When the relationship between two variables is complex and difficult to understand: In such cases, an intervening variable can help to clarify the relationship by identifying the underlying mechanisms that link the two variables.
- When there are multiple potential causes for the dependent variable: In such cases, an intervening variable can help to identify the specific factor or factors that are responsible for the relationship between the independent variable and the dependent variable.
- When the independent variable has an indirect effect on the dependent variable : In such cases, an intervening variable can help to identify the specific intermediate step or steps that link the independent variable to the dependent variable.
Advantages of Intervening Variable
Here are some of the main advantages of using intervening variables:
- Provides a deeper understanding of the relationship between variables: Intervening variables help to explain the underlying mechanisms or processes that link the independent variable to the dependent variable, providing a more in-depth understanding of the relationship.
- Tests causal relationships: Intervening variables allow researchers to test the causal relationship between the independent variable and the dependent variable by identifying the intermediate steps or processes that link the two variables.
- I mproves the accuracy of predictions: By identifying and including intervening variables in the analysis, researchers can create more accurate models and predictions about the relationship between variables.
- Helps to develop interventions: By identifying the mechanisms that link the independent variable to the dependent variable, intervening variables can inform the development of interventions or programs to bring about desired changes.
- Reduces the risk of spurious relationships: Intervening variables help to clarify the relationship between variables by accounting for other factors that may influence the relationship, reducing the risk of spurious or false relationships.
Limitations of Intervening Variable
Here are some of the main limitations of intervening variables:
- Difficulty in identifying and measuring intervening variables: Intervening variables can be difficult to identify and measure accurately, which can lead to measurement error and bias in the analysis.
- Over-reliance on statistical techniques : The identification of intervening variables often relies on statistical techniques, such as regression analysis, which can be limited by the assumptions and limitations of the statistical model.
- Complexity of relationships: Intervening variables are most useful in situations where the relationship between the independent variable and the dependent variable is complex and not well understood. However, in some cases, the relationship may be simple, and the use of intervening variables may add unnecessary complexity to the analysis.
- Lack of generalizability: The relationship between intervening variables and the dependent variable may be specific to the particular context or population being studied, limiting the generalizability of the findings to other contexts or populations.
- Difficulty in establishing causality: While intervening variables can help to test causal relationships between variables, establishing causality can be challenging, and it may be difficult to rule out alternative explanations for the observed relationships.
About the author
Muhammad Hassan
Researcher, Academic Writer, Web developer
You may also like

Control Variable – Definition, Types and Examples

Moderating Variable – Definition, Analysis...

Qualitative Variable – Types and Examples

Variables in Research – Definition, Types and...

Categorical Variable – Definition, Types and...

Independent Variable – Definition, Types and...
How Intervening Variables Work in Sociology
- Key Concepts
- Major Sociologists
- News & Issues
- Research, Samples, and Statistics
- Recommended Reading
- Archaeology
An intervening variable is something that impacts the relationship between an independent and a dependent variable . Usually, the intervening variable is caused by the independent variable , and is itself a cause of the dependent variable.
For example, there is an observed positive correlation between level of education and level of income, such that people with higher levels of education tend to earn higher levels of income. This observable trend, however, is not directly causal in nature. Occupation serves as the intervening variable between the two, since education level (the independent variable) influences what kind of occupation one will have (the dependent variable), and therefore how much money one will earn. In other words, more schooling tends to mean a higher status job, which in turn tends to bring a higher income.
How an Intervening Variable Works
When researchers conduct experiments or studies they are usually interested in understanding the relationship between two variables: an independent and a dependent variable. The independent variable is usually hypothesized to be the cause of the dependent variable, and the research is designed to prove whether or not this is true.
In many cases, like the link between education and income described above, a statistically significant relationship is observable, but it is not proven that the indirect variable is directly causing the dependent variable to behave as it does. When this occurs researchers then hypothesize what other variables could be influencing the relationship, or how a variable might "intervene" between the two. With the example given above, occupation intervenes to mediate the connection between level of education and level of income. (Statisticians consider an intervening variable to be a kind of mediating variable.)
Thinking causally, the intervening variable follows the independent variable but precedes the dependent variable. From a research standpoint, it clarifies the nature of the relationship between the independent and dependent variables.
Other Examples of Intervening Variables in Sociology Research
Another example of an intervening variable that sociologists monitor is the effect of systemic racism on college completion rates. There is a documented relationship between race and college completion rates.
Research shows that among 25 to 29-year-old adults in the U.S., Asian Americans are most likely to have completed college, followed by Whites, while Blacks and Hispanics have much lower rates of college completion. This represents a statistically significant relationship between race (independent variable) and level of education (dependent variable). However, it is not accurate to say that race itself influences level of education. Rather, the experience of racism is an intervening variable between the two.
Many studies have shown that racism has a strong effect on the quality of K-12 education that one receives in the U.S. The nation's long history of segregation and housing patterns today mean that the nation's least-funded schools primarily serve students of color while the nation's best-funded schools primarily serve White students. In this way, racism intervenes to affect the quality of education.
Additionally, studies have shown that implicit racial biases among educators lead to Black and Latino students receiving less encouragement and more discouragement in the classroom than White and Asian students, and also, that they are more regularly and harshly punished for acting out. This means that racism, as it manifests in the thoughts and actions of educators, once again intervenes to impact college completion rates on the basis of race. There are numerous other ways in which racism acts as an intervening variable between race and level of education.
- Difference Between Independent and Dependent Variables
- Null Hypothesis Examples
- Examples of Independent and Dependent Variables
- What It Means When a Variable Is Spurious
- Understanding Path Analysis
- Independent Variable Definition and Examples
- Definition of a Hypothesis
- Visualizing Social Stratification in the U.S.
- Structural Equation Modeling
- What Are the Elements of a Good Hypothesis?
- Control Variable
- Correlation Analysis in Research
- Scientific Method Vocabulary Terms
- Dependent Variable Definition and Examples
- The Role of a Controlled Variable in an Experiment
- Lambda and Gamma as Defined in Sociology
Intervening Variables
- Living reference work entry
- First Online: 24 April 2018
- Cite this living reference work entry
- Heidi L. Shaw 3
320 Accesses
1 Citations
An intervening variable is an abstract scientific concept that scientists invoke to label or summarize relationships between independent and dependent variables across a variety of circumstances. Commonly cited examples of intervening variables include gravity, thirst, learning, and intelligence.
Introduction
During his presidential address to the American Psychological Association in 1937, Edward C. Tolman used the term intervening variable in his comments regarding theories and facts:
But what is a theory? According to Professor Hull, a theory is a set of definitions and postulates proposed by the theorist (on the basis presumably of some already found facts) from which other empirically testable facts, or as he calls them, theorems, can be logically deduced. These deduced theorems will be new empirical relationships which the theorist—or more often, his research assistants—can, then and there, be set to look for. For my own nefarious purposes, however, I wish to phrase...
This is a preview of subscription content, log in via an institution to check access.
Access this chapter
Institutional subscriptions
Bugelski, B. R. (1956). The psychology of learning . New York: Henry Holt and Company.
Google Scholar
Chaplin, J. P., & Krawiec, T. S. (1960). Systems and theories of psychology . New York: Holt, Rinehart, & Winston.
Gardner, R. A., & Gardner, B. T. (1998). The structure of learning: From sign stimulus to sign language . Mahwah: Lawrence Erlbaum Associates.
Goodwin, C. J. (2015). A history of modern psychology . Hoboken: Wiley.
Halliday, T. H. (1983). Chapter 4: Motivation. In T. H. Halliday & P. J. B. Slater (Eds.), Animal behaviour: Causes and effects (Vol. 1, pp. 100–133). Oxford, UK: Blackwell Scientific Publications.
Hilgard, E. R. (1956). Theories of learning (2nd ed.). New York: Appleton-Century-Crofts.
Hilgard, E. R., & Bower, G. H. (1966). Theory of learning (3rd ed.). New York: Meredith Publishing Company.
Hilgard, E. R., & Bower, G. H. (1975). Theories of learning (4th ed.). Englewood Cliffs: Prentice-Hall.
Kimble, K. (1961). Hilgard and Marquis’ conditioning and learning (2nd ed.). New York: Appleton-Century-Crofts.
MacCorquodale, K., & Meehl, P. E. (1948). On a distinction between hypothetical constructs and intervening variables. Psychological Review, 55 (2), 95–107.
Article Google Scholar
Mendel, G. (1865). Experiments in plant hybridization . http://www.esp.org/foundations/genetics/classical/gm-65.pdf
Tolman, E. C. (1938). The determiners of behavior at a choice point. Psychological Review, 45 (1), 1–41.
Download references
Author information
Authors and affiliations.
Yakima Valley College, Yakima, WA, USA
Heidi L. Shaw
You can also search for this author in PubMed Google Scholar
Corresponding author
Correspondence to Heidi L. Shaw .
Editor information
Editors and affiliations.
Oakland University , Rochester, Michigan, USA
Jennifer Vonk
Department of Psychology, Oakland University Department of Psychology, Rochester, Michigan, USA
Todd Shackelford
Section Editor information
Department of Psychology, Southern Oregon University, Ashland, OR, USA
Mark A. Krause
Rights and permissions
Reprints and permissions
Copyright information
© 2018 Springer International Publishing AG, part of Springer Nature
About this entry
Cite this entry.
Shaw, H.L. (2018). Intervening Variables. In: Vonk, J., Shackelford, T. (eds) Encyclopedia of Animal Cognition and Behavior. Springer, Cham. https://doi.org/10.1007/978-3-319-47829-6_1047-1
Download citation
DOI : https://doi.org/10.1007/978-3-319-47829-6_1047-1
Received : 10 April 2018
Accepted : 10 April 2018
Published : 24 April 2018
Publisher Name : Springer, Cham
Print ISBN : 978-3-319-47829-6
Online ISBN : 978-3-319-47829-6
eBook Packages : Springer Reference Behavioral Science and Psychology Reference Module Humanities and Social Sciences Reference Module Business, Economics and Social Sciences
- Publish with us
Policies and ethics
- Find a journal
- Track your research
- Bipolar Disorder
- Therapy Center
- When To See a Therapist
- Types of Therapy
- Best Online Therapy
- Best Couples Therapy
- Best Family Therapy
- Managing Stress
- Sleep and Dreaming
- Understanding Emotions
- Self-Improvement
- Healthy Relationships
- Student Resources
- Personality Types
- Guided Meditations
- Verywell Mind Insights
- 2024 Verywell Mind 25
- Mental Health in the Classroom
- Editorial Process
- Meet Our Review Board
- Crisis Support
Types of Variables in Psychology Research
Examples of Independent and Dependent Variables
Kendra Cherry, MS, is a psychosocial rehabilitation specialist, psychology educator, and author of the "Everything Psychology Book."
:max_bytes(150000):strip_icc():format(webp)/IMG_9791-89504ab694d54b66bbd72cb84ffb860e.jpg)
James Lacy, MLS, is a fact-checker and researcher.
:max_bytes(150000):strip_icc():format(webp)/James-Lacy-1000-73de2239670146618c03f8b77f02f84e.jpg)
Dependent and Independent Variables
- Intervening Variables
- Extraneous Variables
- Controlled Variables
- Confounding Variables
- Operationalizing Variables
Frequently Asked Questions
Variables in psychology are things that can be changed or altered, such as a characteristic or value. Variables are generally used in psychology experiments to determine if changes to one thing result in changes to another.
Variables in psychology play a critical role in the research process. By systematically changing some variables in an experiment and measuring what happens as a result, researchers are able to learn more about cause-and-effect relationships.
The two main types of variables in psychology are the independent variable and the dependent variable. Both variables are important in the process of collecting data about psychological phenomena.
This article discusses different types of variables that are used in psychology research. It also covers how to operationalize these variables when conducting experiments.
Students often report problems with identifying the independent and dependent variables in an experiment. While this task can become more difficult as the complexity of an experiment increases, in a psychology experiment:
- The independent variable is the variable that is manipulated by the experimenter. An example of an independent variable in psychology: In an experiment on the impact of sleep deprivation on test performance, sleep deprivation would be the independent variable. The experimenters would have some of the study participants be sleep-deprived while others would be fully rested.
- The dependent variable is the variable that is measured by the experimenter. In the previous example, the scores on the test performance measure would be the dependent variable.
So how do you differentiate between the independent and dependent variables? Start by asking yourself what the experimenter is manipulating. The things that change, either naturally or through direct manipulation from the experimenter, are generally the independent variables. What is being measured? The dependent variable is the one that the experimenter is measuring.
Intervening Variables in Psychology
Intervening variables, also sometimes called intermediate or mediator variables, are factors that play a role in the relationship between two other variables. In the previous example, sleep problems in university students are often influenced by factors such as stress. As a result, stress might be an intervening variable that plays a role in how much sleep people get, which may then influence how well they perform on exams.
Extraneous Variables in Psychology
Independent and dependent variables are not the only variables present in many experiments. In some cases, extraneous variables may also play a role. This type of variable is one that may have an impact on the relationship between the independent and dependent variables.
For example, in our previous example of an experiment on the effects of sleep deprivation on test performance, other factors such as age, gender, and academic background may have an impact on the results. In such cases, the experimenter will note the values of these extraneous variables so any impact can be controlled for.
There are two basic types of extraneous variables:
- Participant variables : These extraneous variables are related to the individual characteristics of each study participant that may impact how they respond. These factors can include background differences, mood, anxiety, intelligence, awareness, and other characteristics that are unique to each person.
- Situational variables : These extraneous variables are related to things in the environment that may impact how each participant responds. For example, if a participant is taking a test in a chilly room, the temperature would be considered an extraneous variable. Some participants may not be affected by the cold, but others might be distracted or annoyed by the temperature of the room.
Other extraneous variables include the following:
- Demand characteristics : Clues in the environment that suggest how a participant should behave
- Experimenter effects : When a researcher unintentionally suggests clues for how a participant should behave
Controlled Variables in Psychology
In many cases, extraneous variables are controlled for by the experimenter. A controlled variable is one that is held constant throughout an experiment.
In the case of participant variables, the experiment might select participants that are the same in background and temperament to ensure that these factors don't interfere with the results. Holding these variables constant is important for an experiment because it allows researchers to be sure that all other variables remain the same across all conditions.
Using controlled variables means that when changes occur, the researchers can be sure that these changes are due to the manipulation of the independent variable and not caused by changes in other variables.
It is important to also note that a controlled variable is not the same thing as a control group . The control group in a study is the group of participants who do not receive the treatment or change in the independent variable.
All other variables between the control group and experimental group are held constant (i.e., they are controlled). The dependent variable being measured is then compared between the control group and experimental group to see what changes occurred because of the treatment.
Confounding Variables in Psychology
If a variable cannot be controlled for, it becomes what is known as a confounding variabl e. This type of variable can have an impact on the dependent variable, which can make it difficult to determine if the results are due to the influence of the independent variable, the confounding variable, or an interaction of the two.
Operationalizing Variables in Psychology
An operational definition describes how the variables are measured and defined in the study. Before conducting a psychology experiment , it is essential to create firm operational definitions for both the independent variable and dependent variables.
For example, in our imaginary experiment on the effects of sleep deprivation on test performance, we would need to create very specific operational definitions for our two variables. If our hypothesis is "Students who are sleep deprived will score significantly lower on a test," then we would have a few different concepts to define:
- Students : First, what do we mean by "students?" In our example, let’s define students as participants enrolled in an introductory university-level psychology course.
- Sleep deprivation : Next, we need to operationally define the "sleep deprivation" variable. In our example, let’s say that sleep deprivation refers to those participants who have had less than five hours of sleep the night before the test.
- Test variable : Finally, we need to create an operational definition for the test variable. For this example, the test variable will be defined as a student’s score on a chapter exam in the introductory psychology course.
Once all the variables are operationalized, we're ready to conduct the experiment.
Variables play an important part in psychology research. Manipulating an independent variable and measuring the dependent variable allows researchers to determine if there is a cause-and-effect relationship between them.
A Word From Verywell
Understanding the different types of variables used in psychology research is important if you want to conduct your own psychology experiments. It is also helpful for people who want to better understand what the results of psychology research really mean and become more informed consumers of psychology information .
Independent and dependent variables are used in experimental research. Unlike some other types of research (such as correlational studies ), experiments allow researchers to evaluate cause-and-effect relationships between two variables.
Researchers can use statistical analyses to determine the strength of a relationship between two variables in an experiment. Two of the most common ways to do this are to calculate a p-value or a correlation. The p-value indicates if the results are statistically significant while the correlation can indicate the strength of the relationship.
In an experiment on how sugar affects short-term memory, sugar intake would be the independent variable and scores on a short-term memory task would be the independent variable.
In an experiment looking at how caffeine intake affects test anxiety, the amount of caffeine consumed before a test would be the independent variable and scores on a test anxiety assessment would be the dependent variable.
Just as with other types of research, the independent variable in a cognitive psychology study would be the variable that the researchers manipulate. The specific independent variable would vary depending on the specific study, but it might be focused on some aspect of thinking, memory, attention, language, or decision-making.
American Psychological Association. Operational definition . APA Dictionary of Psychology.
American Psychological Association. Mediator . APA Dictionary of Psychology.
Altun I, Cınar N, Dede C. The contributing factors to poor sleep experiences in according to the university students: A cross-sectional study . J Res Med Sci . 2012;17(6):557-561. PMID:23626634
Skelly AC, Dettori JR, Brodt ED. Assessing bias: The importance of considering confounding . Evid Based Spine Care J . 2012;3(1):9-12. doi:10.1055/s-0031-1298595
- Evans, AN & Rooney, BJ. Methods in Psychological Research. Thousand Oaks, CA: SAGE Publications; 2014.
- Kantowitz, BH, Roediger, HL, & Elmes, DG. Experimental Psychology. Stamfort, CT: Cengage Learning; 2015.
By Kendra Cherry, MSEd Kendra Cherry, MS, is a psychosocial rehabilitation specialist, psychology educator, and author of the "Everything Psychology Book."
What is an Intervening Variable?
An intervening variable is a variable that affects the relationship between an independent variable and a dependent variable .
Often this type of variable can appear when researchers are studying the relationship between two variables and don’t realize that another variable is actually intervening in the relationship.
Intervening variables pop up in many different research situations. Here are a few examples.
Example 1: Education & Spending
Researchers may be interested in the relationship between education (the independent variable) and yearly spending (the dependent variable).
After collecting data on education level and yearly spending for 1,000 individuals, they find that there is a strong positive correlation between the two variables. In particular, they find that individuals who have more education tend to spend more.
However, without realizing it the researchers have failed to take note of the intervening variable income . It turns out that individuals who have higher levels of education tend to hold higher-paying jobs, which means they naturally have more money to spend.

Example 2: Poverty and Life Expectancy
Researchers may be interested in the relationship between poverty (the independent variable) and life expectancy (the dependent variable).
After collecting data on poverty and life expectancy for 10,000 individuals, they find that there is a strong correlation between the two variables. In particular, they find that more impoverished individuals tend to have lower life expectancies.
However, without realizing it the researchers have failed to take note of the intervening variable healthcare . It turns out that individuals who are more impoverished have less reliable access to healthcare, which naturally means that they have lower life expectancies.

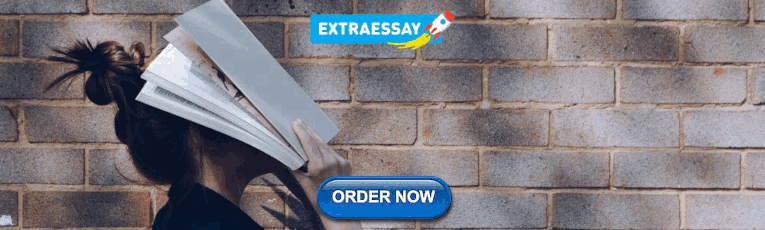
Example 3: Hours Spent Practicing & Points per Game
A sports researcher may be interested in the relationship between hours spent practicing by players (the independent variable) and their average points per game (the dependent variable).
After collecting data on hours spent practicing and points per game for 100 players, they find that there is a strong correlation between the two variables. In particular, they find that players who practice more tend to average more points per game.
However, without realizing it the researcher has failed to take note of the intervening variable minutes played . It turns out that individuals who practice for more hours are around the coach more who then gets to know the player better and tends to put them in the game more, which gives then more opportunities to score more points.

The Importance of Identifying Intervening Variables
Understanding intervening variables can often help researchers clarify the relationship between an independent and dependent variable because the intervening variables are often the true variable that explains variations in the dependent variable.
In many cases, the independent variable causes changes in some intervening variable, which then causes changes in the dependent variable under study.
By identifying the intervening variable, it becomes easier to understand the actual relationship between the independent and dependent variable.
Technical Note: Intervening variables are sometimes also referred to as mediating variables or intermediary variables.
Additional Resources
What are Extraneous Variables? What are Concomitant Variables? What is Reverse Causation? What is a Confounding Variable?
How to Normalize Columns in a Pandas DataFrame
How to use dunnett’s test for multiple comparisons, related posts, how to normalize data between -1 and 1, vba: how to check if string contains another..., how to interpret f-values in a two-way anova, how to create a vector of ones in..., how to determine if a probability distribution is..., what is a symmetric histogram (definition & examples), how to find the mode of a histogram..., how to find quartiles in even and odd..., how to calculate sxy in statistics (with example), how to calculate expected value of x^3.

An official website of the United States government
The .gov means it’s official. Federal government websites often end in .gov or .mil. Before sharing sensitive information, make sure you’re on a federal government site.
The site is secure. The https:// ensures that you are connecting to the official website and that any information you provide is encrypted and transmitted securely.
- Publications
- Account settings
Preview improvements coming to the PMC website in October 2024. Learn More or Try it out now .
- Advanced Search
- Journal List
- Perspect Clin Res
- v.10(3); Jul-Sep 2019
Study designs: Part 4 – Interventional studies
Rakesh aggarwal.
Director, Jawaharlal Institute of Postgraduate Medical Education and Research, Puducherry, India
Priya Ranganathan
1 Department of Anaesthesiology, Tata Memorial Centre, Mumbai, Maharashtra, India
In the fourth piece of this series on research study designs, we look at interventional studies (clinical trials). These studies differ from observational studies in that the investigator decides whether or not a participant will receive the exposure (or intervention). In this article, we describe the key features and types of interventional studies.
INTRODUCTION
In previous articles in this series, we introduced the concept of study designs[ 1 ] and have described in detail the observational study designs – descriptive[ 2 ] as well as analytical.[ 3 ] In this and another future piece, we will discuss the interventional study designs.
In observational studies, a researcher merely documents the presence of exposure(s) and outcome(s) as they occur, without trying to alter the course of natural events. By contrast, in interventional studies, the researcher actively interferes with nature – by performing an intervention in some or all study participants – to determine the effect of exposure to the intervention on the natural course of events. An example would be a study in which the investigator randomly assigns the participants to receive either aspirin or a placebo for a specific duration to determine whether the drug has an effect on the future risk of developing cerebrovascular events. In this example, aspirin (the “intervention”) is the “exposure,” and the risk of cerebrovascular events is the “outcome.” Interventional studies in humans are also commonly referred to as “trials.”
Interventional studies, by their very design, are prospective. This sometimes leads to confusion between interventional and prospective cohort study designs. For instance, the study design in the above example appears analogous to that of a prospective cohort study in which people attending a wellness clinic are asked whether they take aspirin regularly and then followed for a few years for occurrence of cerebrovascular events. The basic difference is that in the interventional study, it is the investigators who assign each person to take or not to take aspirin, whereas in the cohort study, this is determined by an extraneous factor.
Interventional studies can be divided broadly into two main types: (i) “controlled clinical trials” (or simply “clinical trials” or “trials”), in which individuals are assigned to one of two or more competing interventions, and (ii) “community trials” (or field trials), in which entire groups, e.g., villages, neighbourhoods, schools or districts, are assigned to different interventions.
The interventions can be quite varied; examples include administration of a drug or vaccine or dietary supplement, performance of a diagnostic or therapeutic procedure, and introduction of an educational tool. Depending on whether the intervention is aimed at preventing the occurrence of a disease (e.g., administration of a vaccine, boiling of water, distribution of condoms or of an educational pamphlet) or at providing relief to or curing patients with a disease (e.g., antiretroviral drugs in HIV-infected persons), a trial may also be referred to as “preventive trial” or “therapeutic trial”.
VARIOUS TYPES OF INTERVENTIONAL STUDY DESIGNS
Several variations of interventional study designs with varying complexity are possible, and each of these is described below. Of these, the most commonly used and possibly the strongest design is a randomized controlled trial (RCT).
Randomized controlled trials
In an RCT, a group of participants fulfilling certain inclusion and exclusion criteria is “randomly” assigned to two separate groups, each receiving a different intervention. Random assignment implies that each participant has an equal chance of being allocated to the two groups.
The use of randomization is a major distinguishing feature and strength of this study design. A well-implemented randomization procedure is expected to result in two groups that are comparable overall, when both measured and unmeasured factors are taken into account. Thus, theoretically, the two groups differ only in the intervention received, and any difference in outcomes between them is thus related to the effect of intervention.
The term “controlled” refers to the presence of a concurrent control or comparator group. These studies have two or more groups – treatment and control. The control group receives no intervention or another intervention that resembles the test intervention in some ways but lacks its activity (e.g., placebo or sham procedure, referred to also as “placebo-controlled” or “sham-controlled” trials) or another active treatment (e.g., the current standard of care). The outcomes are then compared between the intervention and the comparator groups.
If an effort is made to ensure that other factors are similar across groups, then the availability of data from the comparator group allows a stronger inference about the effect of the intervention being tested than is possible in studies that lack a control group.
Some additional methodological features are often added to this study design to further improve the validity of a trial. These include allocation concealment, blinding, intention-to-treat analysis, measurement of compliance, minimizing the dropouts, and ensuring appropriate sample size. These will be discussed in the next piece.
Nonrandomized controlled clinical trials
In this design, participants are assigned to different intervention arms without following a “random” procedure. For instance, this may be based on the investigator's convenience or whether the participant can afford a particular drug or not. Although such a design can suggest a possible relationship between the intervention and the outcome, it is susceptible to bias – with patients in the two groups being potentially dissimilar – and hence validity of the results obtained is low.
Interventional studies without concurrent controls
When a new intervention, e.g., a new drug, becomes available, it is possible to a researcher to assign a group of persons to receive it and compare the outcome in them to that in a similar group of persons followed up in the past without this treatment (”historical controls”). This is liable to a high risk of bias, e.g., through differences in the severity of disease or other factors in the two groups or through improvement over time in the available supportive care.
Before–after (pre–post) studies
In this design, a variable of interest is measured before and after an intervention in the same participants. Examples include measurement of glycated hemoglobin of a group of persons before and after administration of a new drug (in a particular dose schedule and at a particular time in relation to it) or number of traffic accident deaths in a city before and after implementation of a policy of mandatory helmet use for two-wheeler drivers.
Such studies have a single arm and lack a comparator arm. The only basis of deriving a conclusion from these studies is the temporal relationship of the measurements to the intervention. However, the outcome can instead be related to other changes that occurred around the same time as the intervention, e.g., change in diet or implementation of alcohol use restrictions, respectively, in the above examples. The change can also represent a natural variation (e.g., diurnal or seasonal) in the variable of interest or a change in the instrument used to measure it. Thus, the outcomes observed in such studies cannot be reliably attributed to the specific intervention, making this a weaker design than RCT.
Some believe that the before-after design is comparable to observational design and that only studies with a “comparator” group, as discussed above, are truly interventional studies.
Factorial study design
If two (or more) interventions are available for a particular disease condition, the relevant question is not only whether each drug is efficacious but also whether a combination of the two is more efficacious than either of them alone.
The simplest factorial design is a 2 × 2 factorial design. Let us think of two interventions: A and B. The participants are randomly allocated to one of four combinations of these interventions – A alone, B alone, both A and B, and neither A nor B (control). This design allows (i) comparison of each intervention with the control group, (ii) comparison of the two interventions with each other, and (iii) investigation of possible interactions between the two treatments (whether the effect of the combination differs from the sum of effects of A and B when given separately). As an example, in a recent study, infants in South India being administered a rotavirus vaccine were randomly assigned to receive a zinc supplement and a probiotic, only probiotic (with zinc placebo), only zinc supplement (with probiotic placebo), or neither (probiotic placebo and zinc placebo).[ 4 ] Neither zinc nor probiotic led to any change in the immunogenicity of the vaccine, but the group receiving the zinc-probiotic combination had a modest improvement.
This design allows the study of two interventions in the same trial without unduly increasing the required number of participants, as also the study of interaction between the two treatments.
Crossover study design
This is a special type of interventional study design, in which study participants intentionally “crossover” to the other intervention arm. Each participant first receives one intervention (usually by random allocation, as described above). At the end of this “ first” intervention, each participant is switched over to the other intervention. Most often, the two interventions are separated by a washout period to get rid of the effect of the first intervention and to allow each participant to return to the baseline state. For example, in a recent study, obese participants underwent two 5-day inpatient stays – with a 1-month washout period between them, during which they consumed a smoothie containing 48-g walnuts or a macronutrient-matched placebo smoothie without nuts and underwent measurement of several blood analytes, hemodynamics, and gut microbiota.[ 5 ]
This design has the advantages of (i) each participant serving as his/her own control, thereby reducing the effect of interindividual variability, and (ii) needing fewer participants than a parallel-arm RCT. However, this design can be used only for disease conditions which are stable and cannot be cured, and where interventions provide only transient relief. For instance, this design would be highly useful for comparing the effect of two anti-inflammatory drugs on symptoms in patients with long-standing rheumatoid arthritis.
Cluster randomized trials
Sometimes, an intervention cannot be easily administered to individuals but can be applied to groups. In such cases, a trial can be done by assigning “clusters” – some logical groups of participants – to receive or not receive the intervention.
As an example, a study in Greece looked at the effect of providing meals in schools on household food security.[ 6 ] The 51 schools in this study were randomly allocated to provide or not provide a healthy meal every day to students; schools in both the groups provided an educational intervention.
However, such studies need a somewhat larger sample size than individual-randomized studies and the use of special statistical tools for data analysis.
Financial support and sponsorship
Conflicts of interest.
There are no conflicts of interest.

Research Variables 101
Independent variables, dependent variables, control variables and more
By: Derek Jansen (MBA) | Expert Reviewed By: Kerryn Warren (PhD) | January 2023
If you’re new to the world of research, especially scientific research, you’re bound to run into the concept of variables , sooner or later. If you’re feeling a little confused, don’t worry – you’re not the only one! Independent variables, dependent variables, confounding variables – it’s a lot of jargon. In this post, we’ll unpack the terminology surrounding research variables using straightforward language and loads of examples .
Overview: Variables In Research
What (exactly) is a variable.
The simplest way to understand a variable is as any characteristic or attribute that can experience change or vary over time or context – hence the name “variable”. For example, the dosage of a particular medicine could be classified as a variable, as the amount can vary (i.e., a higher dose or a lower dose). Similarly, gender, age or ethnicity could be considered demographic variables, because each person varies in these respects.
Within research, especially scientific research, variables form the foundation of studies, as researchers are often interested in how one variable impacts another, and the relationships between different variables. For example:
- How someone’s age impacts their sleep quality
- How different teaching methods impact learning outcomes
- How diet impacts weight (gain or loss)
As you can see, variables are often used to explain relationships between different elements and phenomena. In scientific studies, especially experimental studies, the objective is often to understand the causal relationships between variables. In other words, the role of cause and effect between variables. This is achieved by manipulating certain variables while controlling others – and then observing the outcome. But, we’ll get into that a little later…
The “Big 3” Variables
Variables can be a little intimidating for new researchers because there are a wide variety of variables, and oftentimes, there are multiple labels for the same thing. To lay a firm foundation, we’ll first look at the three main types of variables, namely:
- Independent variables (IV)
- Dependant variables (DV)
- Control variables
What is an independent variable?
Simply put, the independent variable is the “ cause ” in the relationship between two (or more) variables. In other words, when the independent variable changes, it has an impact on another variable.
For example:
- Increasing the dosage of a medication (Variable A) could result in better (or worse) health outcomes for a patient (Variable B)
- Changing a teaching method (Variable A) could impact the test scores that students earn in a standardised test (Variable B)
- Varying one’s diet (Variable A) could result in weight loss or gain (Variable B).
It’s useful to know that independent variables can go by a few different names, including, explanatory variables (because they explain an event or outcome) and predictor variables (because they predict the value of another variable). Terminology aside though, the most important takeaway is that independent variables are assumed to be the “cause” in any cause-effect relationship. As you can imagine, these types of variables are of major interest to researchers, as many studies seek to understand the causal factors behind a phenomenon.
Need a helping hand?
What is a dependent variable?
While the independent variable is the “ cause ”, the dependent variable is the “ effect ” – or rather, the affected variable . In other words, the dependent variable is the variable that is assumed to change as a result of a change in the independent variable.
Keeping with the previous example, let’s look at some dependent variables in action:
- Health outcomes (DV) could be impacted by dosage changes of a medication (IV)
- Students’ scores (DV) could be impacted by teaching methods (IV)
- Weight gain or loss (DV) could be impacted by diet (IV)
In scientific studies, researchers will typically pay very close attention to the dependent variable (or variables), carefully measuring any changes in response to hypothesised independent variables. This can be tricky in practice, as it’s not always easy to reliably measure specific phenomena or outcomes – or to be certain that the actual cause of the change is in fact the independent variable.
As the adage goes, correlation is not causation . In other words, just because two variables have a relationship doesn’t mean that it’s a causal relationship – they may just happen to vary together. For example, you could find a correlation between the number of people who own a certain brand of car and the number of people who have a certain type of job. Just because the number of people who own that brand of car and the number of people who have that type of job is correlated, it doesn’t mean that owning that brand of car causes someone to have that type of job or vice versa. The correlation could, for example, be caused by another factor such as income level or age group, which would affect both car ownership and job type.
To confidently establish a causal relationship between an independent variable and a dependent variable (i.e., X causes Y), you’ll typically need an experimental design , where you have complete control over the environmen t and the variables of interest. But even so, this doesn’t always translate into the “real world”. Simply put, what happens in the lab sometimes stays in the lab!
As an alternative to pure experimental research, correlational or “ quasi-experimental ” research (where the researcher cannot manipulate or change variables) can be done on a much larger scale more easily, allowing one to understand specific relationships in the real world. These types of studies also assume some causality between independent and dependent variables, but it’s not always clear. So, if you go this route, you need to be cautious in terms of how you describe the impact and causality between variables and be sure to acknowledge any limitations in your own research.
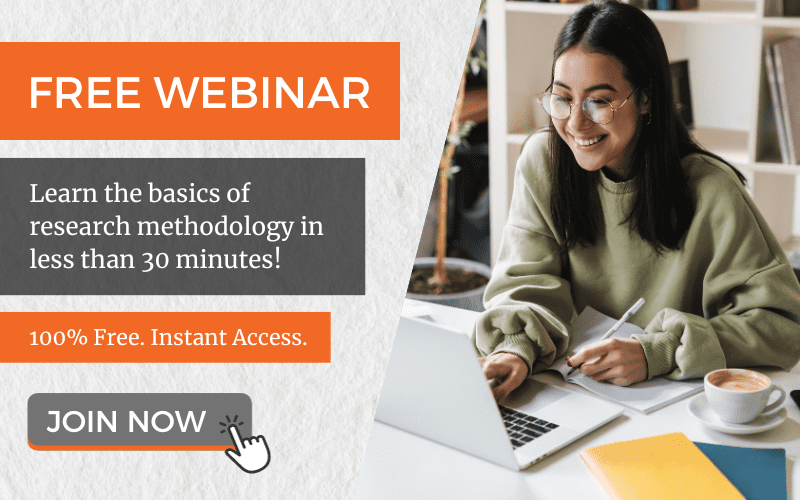
What is a control variable?
In an experimental design, a control variable (or controlled variable) is a variable that is intentionally held constant to ensure it doesn’t have an influence on any other variables. As a result, this variable remains unchanged throughout the course of the study. In other words, it’s a variable that’s not allowed to vary – tough life 🙂
As we mentioned earlier, one of the major challenges in identifying and measuring causal relationships is that it’s difficult to isolate the impact of variables other than the independent variable. Simply put, there’s always a risk that there are factors beyond the ones you’re specifically looking at that might be impacting the results of your study. So, to minimise the risk of this, researchers will attempt (as best possible) to hold other variables constant . These factors are then considered control variables.
Some examples of variables that you may need to control include:
- Temperature
- Time of day
- Noise or distractions
Which specific variables need to be controlled for will vary tremendously depending on the research project at hand, so there’s no generic list of control variables to consult. As a researcher, you’ll need to think carefully about all the factors that could vary within your research context and then consider how you’ll go about controlling them. A good starting point is to look at previous studies similar to yours and pay close attention to which variables they controlled for.
Of course, you won’t always be able to control every possible variable, and so, in many cases, you’ll just have to acknowledge their potential impact and account for them in the conclusions you draw. Every study has its limitations , so don’t get fixated or discouraged by troublesome variables. Nevertheless, always think carefully about the factors beyond what you’re focusing on – don’t make assumptions!
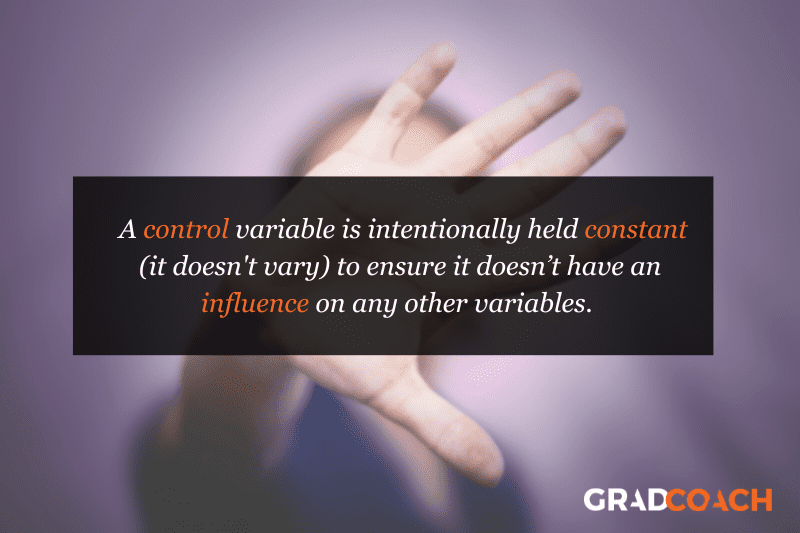
Other types of variables
As we mentioned, independent, dependent and control variables are the most common variables you’ll come across in your research, but they’re certainly not the only ones you need to be aware of. Next, we’ll look at a few “secondary” variables that you need to keep in mind as you design your research.
- Moderating variables
- Mediating variables
- Confounding variables
- Latent variables
Let’s jump into it…
What is a moderating variable?
A moderating variable is a variable that influences the strength or direction of the relationship between an independent variable and a dependent variable. In other words, moderating variables affect how much (or how little) the IV affects the DV, or whether the IV has a positive or negative relationship with the DV (i.e., moves in the same or opposite direction).
For example, in a study about the effects of sleep deprivation on academic performance, gender could be used as a moderating variable to see if there are any differences in how men and women respond to a lack of sleep. In such a case, one may find that gender has an influence on how much students’ scores suffer when they’re deprived of sleep.
It’s important to note that while moderators can have an influence on outcomes , they don’t necessarily cause them ; rather they modify or “moderate” existing relationships between other variables. This means that it’s possible for two different groups with similar characteristics, but different levels of moderation, to experience very different results from the same experiment or study design.
What is a mediating variable?
Mediating variables are often used to explain the relationship between the independent and dependent variable (s). For example, if you were researching the effects of age on job satisfaction, then education level could be considered a mediating variable, as it may explain why older people have higher job satisfaction than younger people – they may have more experience or better qualifications, which lead to greater job satisfaction.
Mediating variables also help researchers understand how different factors interact with each other to influence outcomes. For instance, if you wanted to study the effect of stress on academic performance, then coping strategies might act as a mediating factor by influencing both stress levels and academic performance simultaneously. For example, students who use effective coping strategies might be less stressed but also perform better academically due to their improved mental state.
In addition, mediating variables can provide insight into causal relationships between two variables by helping researchers determine whether changes in one factor directly cause changes in another – or whether there is an indirect relationship between them mediated by some third factor(s). For instance, if you wanted to investigate the impact of parental involvement on student achievement, you would need to consider family dynamics as a potential mediator, since it could influence both parental involvement and student achievement simultaneously.
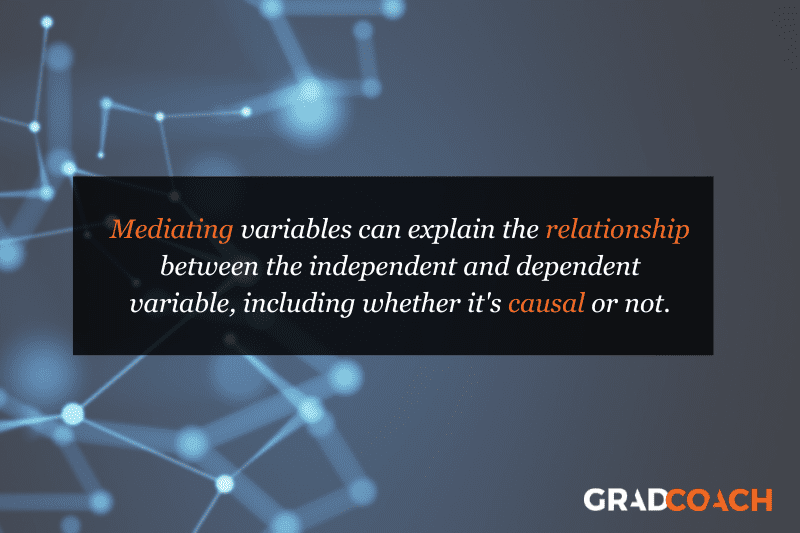
What is a confounding variable?
A confounding variable (also known as a third variable or lurking variable ) is an extraneous factor that can influence the relationship between two variables being studied. Specifically, for a variable to be considered a confounding variable, it needs to meet two criteria:
- It must be correlated with the independent variable (this can be causal or not)
- It must have a causal impact on the dependent variable (i.e., influence the DV)
Some common examples of confounding variables include demographic factors such as gender, ethnicity, socioeconomic status, age, education level, and health status. In addition to these, there are also environmental factors to consider. For example, air pollution could confound the impact of the variables of interest in a study investigating health outcomes.
Naturally, it’s important to identify as many confounding variables as possible when conducting your research, as they can heavily distort the results and lead you to draw incorrect conclusions . So, always think carefully about what factors may have a confounding effect on your variables of interest and try to manage these as best you can.
What is a latent variable?
Latent variables are unobservable factors that can influence the behaviour of individuals and explain certain outcomes within a study. They’re also known as hidden or underlying variables , and what makes them rather tricky is that they can’t be directly observed or measured . Instead, latent variables must be inferred from other observable data points such as responses to surveys or experiments.
For example, in a study of mental health, the variable “resilience” could be considered a latent variable. It can’t be directly measured , but it can be inferred from measures of mental health symptoms, stress, and coping mechanisms. The same applies to a lot of concepts we encounter every day – for example:
- Emotional intelligence
- Quality of life
- Business confidence
- Ease of use
One way in which we overcome the challenge of measuring the immeasurable is latent variable models (LVMs). An LVM is a type of statistical model that describes a relationship between observed variables and one or more unobserved (latent) variables. These models allow researchers to uncover patterns in their data which may not have been visible before, thanks to their complexity and interrelatedness with other variables. Those patterns can then inform hypotheses about cause-and-effect relationships among those same variables which were previously unknown prior to running the LVM. Powerful stuff, we say!
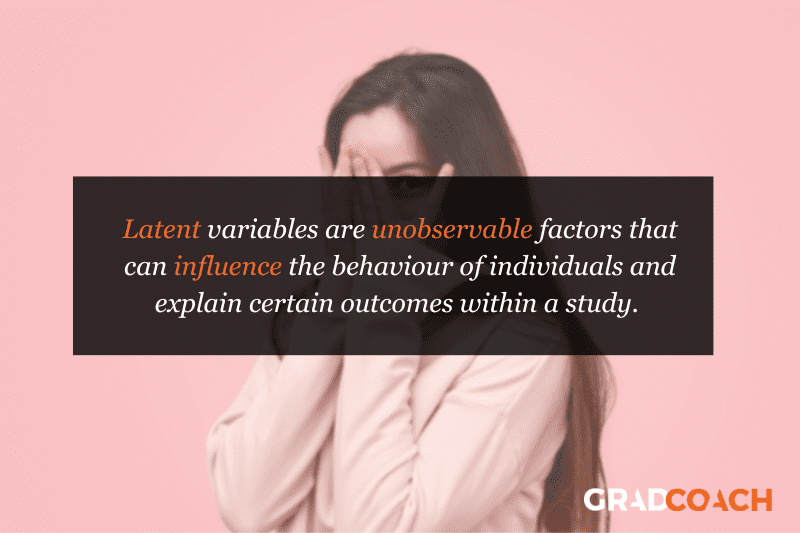
Let’s recap
In the world of scientific research, there’s no shortage of variable types, some of which have multiple names and some of which overlap with each other. In this post, we’ve covered some of the popular ones, but remember that this is not an exhaustive list .
To recap, we’ve explored:
- Independent variables (the “cause”)
- Dependent variables (the “effect”)
- Control variables (the variable that’s not allowed to vary)
If you’re still feeling a bit lost and need a helping hand with your research project, check out our 1-on-1 coaching service , where we guide you through each step of the research journey. Also, be sure to check out our free dissertation writing course and our collection of free, fully-editable chapter templates .
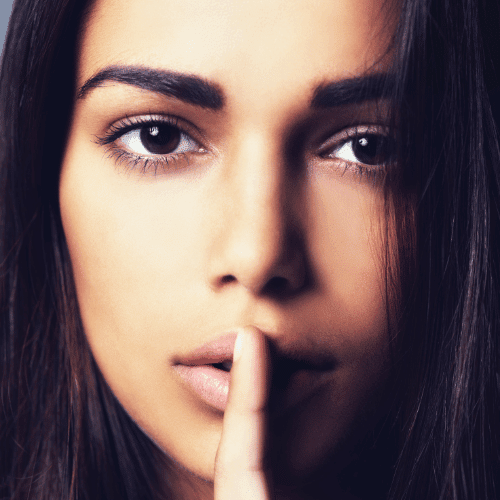
Psst... there’s more!
This post was based on one of our popular Research Bootcamps . If you're working on a research project, you'll definitely want to check this out ...
You Might Also Like:
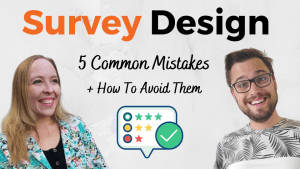
Very informative, concise and helpful. Thank you
Helping information.Thanks
practical and well-demonstrated
Very helpful and insightful
Submit a Comment Cancel reply
Your email address will not be published. Required fields are marked *
Save my name, email, and website in this browser for the next time I comment.
- Print Friendly

Statistics Made Easy
What is a Moderating Variable? Definition & Example
A moderating variable is a type of variable that affects the relationship between a dependent variable and an independent variable .
When performing regression analysis , we’re often interested in understanding how changes in an independent variable affect a dependent variable. However, sometimes a moderating variable can affect this relationship.
For example, suppose we want to fit a regression model in which we use the independent variable hours spent exercising each week to predict the dependent variable resting heart rate .

We suspect that more hours spent exercising is associated with a lower resting heart rate. However, this relationship could be affected by a moderating variable such as gender .
It’s possible that each extra hour of exercise causes resting heart rate to drop more for men compared to women.

Another example of a moderating variable could be age . It’s likely that each extra hour of exercise causes resting heart rate to drop more for younger people compared to older people.

Properties of Moderating Variables
Moderating variables have the following properties:
1. Moderating variables can be qualitative or quantitative .
Qualitative variables are variables that take on names or labels. Examples include:
- Gender (Male or Female)
- Education Level (High School Degree, Bachelor’s Degree, Master’s Degree, etc.)
- Marital Status (Single, Married, Divorced)
Quantitative variables are variables that take on numerical values. Examples include:
- Square Footage
- Population Size
In the previous examples, gender was a qualitative variable that could affect the relationship between hours studied and resting heart rate while age was a quantitative variable that could potentially affect the relationship.
2. Moderating variables can affect the relationship between an independent and dependent variable in a variety of ways.
Moderating variables can have the following effects:
- Strengthen the relationship between two variables.
- Weaken the relationship between two variables.
- Negate the relationship between two variables.
Depending on the situation, a moderating variable can moderate the relationship between two variables in many different ways.
How to Test for Moderating Variables
If X is an independent variable (sometimes called a “predictor” variable) and Y is a dependent variable (sometimes called a “response” variable), then we could write a regression equation to describe the relationship between the two variables as follows:
Y = β 0 + β 1 X
If we suspect that some other variable, Z , is a moderator variable, then we could fit the following regression model:
Y = β 0 + β 1 X 1 + β 2 Z + β 3 XZ
In this equation, the term XZ is known as an interaction term .
If the p-value for the coefficient of XZ in the regression output is statistically significant, then this indicates that there is a significant interaction between X and Z and Z should be included in the regression model as a moderator variable.
We would write the final model as:
Y = β 0 + β 1 X + β 2 Z + β 3 XZ
If the p-value for the coefficient of XZ in the regression output is not statistically significant, then Z is not a moderator variable.
However it’s possible that the coefficient for Z could still be statistically significant. In this case, we would simply include Z as another independent variable in the regression model.
We would then write the final model as:
Y = β 0 + β 1 X + β 2 Z
Additional Resources
How to Read and Interpret a Regression Table How to Use Dummy Variables in Regression Analysis Introduction to Confounding Variables
Featured Posts

Hey there. My name is Zach Bobbitt. I have a Masters of Science degree in Applied Statistics and I’ve worked on machine learning algorithms for professional businesses in both healthcare and retail. I’m passionate about statistics, machine learning, and data visualization and I created Statology to be a resource for both students and teachers alike. My goal with this site is to help you learn statistics through using simple terms, plenty of real-world examples, and helpful illustrations.
One Reply to “What is a Moderating Variable? Definition & Example”
Nicely explained Moderation concept.
Leave a Reply Cancel reply
Your email address will not be published. Required fields are marked *
Join the Statology Community
Sign up to receive Statology's exclusive study resource: 100 practice problems with step-by-step solutions. Plus, get our latest insights, tutorials, and data analysis tips straight to your inbox!
By subscribing you accept Statology's Privacy Policy.

A .gov website belongs to an official government organization in the United States.
A lock ( ) or https:// means you've safely connected to the .gov website. Share sensitive information only on official, secure websites.
- Talking with Your Healthcare Provider
- Birth Defects Statistics
- Birth Defects Resources
- Birth Defects Awareness Month
- Living with Down Syndrome
- Conversation Tips
- Growth Charts for Down Syndrome
- Accessing NBDPS and BD-STEPS Data
- Birth Defects Awareness Month Social Media Resources
- About Alcohol Use During Pregnancy
Down Syndrome
- Down syndrome is a genetic condition where a person is born with an extra chromosome.
- This can affect how their brain and body develop.
- People diagnosed with Down syndrome can lead healthy lives with supportive care.
Down syndrome is a condition in which a person has an extra copy of chromosome 21. Chromosomes are small "packages" of genes in the body's cells, which determine how the body forms and functions.
When babies are growing, the extra chromosome changes how their body and brain develop. This can cause both physical and mental challenges.
People with Down syndrome often have developmental challenges, such as being slower to learn to speak than other children.
Distinct physical signs of Down syndrome are usually present at birth and become more apparent as the baby grows. They can include facial features, such as:
- A flattened face, especially the bridge of the nose
- Almond-shaped eyes that slant up
- A tongue that tends to stick out of the mouth
Other physical signs can include:
- A short neck
- Small ears, hands, and feet
- A single line across the palm of the hand (palmar crease)
- Small pinky fingers
- Poor muscle tone or loose joints
- Shorter-than-average height
Some people with Down syndrome have other medical problems as well. Common health problems include:
- Congenital heart defects
- Hearing loss
- Obstructive sleep apnea
Down syndrome is the most common chromosomal condition diagnosed in the United States. Each year, about 5,700 babies born in the US have Down syndrome. 1
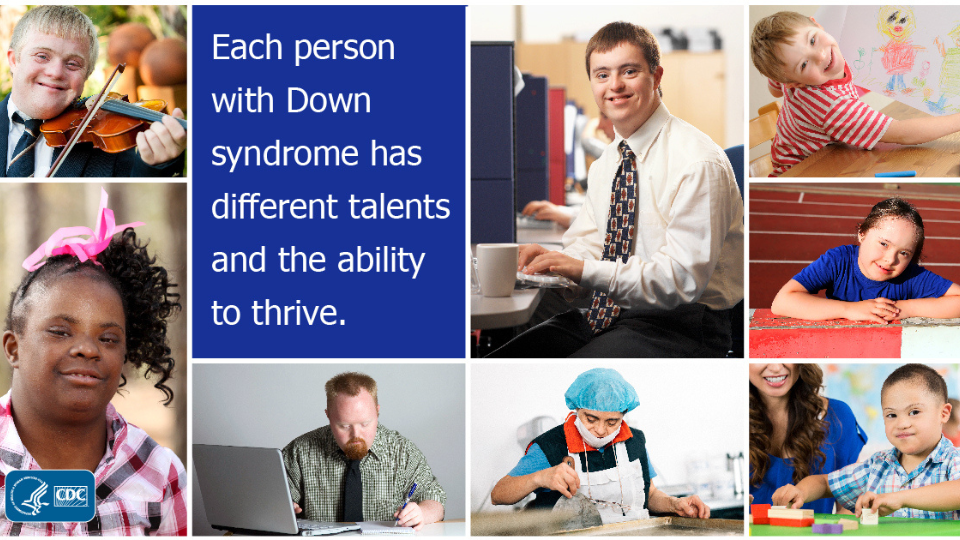
There are three types of Down syndrome. The physical features and behaviors are similar for all three types.
With Trisomy 21, each cell in the body has three separate copies of chromosome 21. About 95% of people with Down syndrome have Trisomy 21.
Translocation Down syndrome
In this type, an extra part or a whole extra chromosome 21 is present. However, the extra chromosome is attached or "trans-located" to a different chromosome rather than being a separate chromosome 21. This type accounts for about 3% of people with Down syndrome.
Mosaic Down syndrome
Mosaic means mixture or combination. In this type, some cells have three copies of chromosome 21, but other cells have the typical two copies. People with mosaic Down syndrome may have fewer features of the condition. This type accounts for about 2% of people with Down syndrome.
Risk factors
We don't know for sure why Down syndrome occurs or how many different factors play a role. We do know that some things can affect your risk of having a baby with Down syndrome.
One factor is your age when you get pregnant. The risk of having a baby with Down syndrome increases with age, especially if you are 35 years or older when you get pregnant. 2 3 4
However, the majority of babies with Down syndrome are still born to mothers less than 35 years old. This is because there are many more births among younger women. 5 6
Regardless of age, parents who have one child with Down syndrome are at an increased risk of having another child with Down syndrome. 7
Screening and diagnosis
There are two types of tests available to detect Down syndrome during pregnancy: screening tests and diagnostic tests. A screening test can tell you if your pregnancy has a higher chance of being affected Down syndrome. Screening tests don't provide an absolute diagnosis.
Diagnostic tests can typically detect if a baby will have Down syndrome, but they carry more risk. Neither screening nor diagnostic tests can predict the full impact of Down syndrome on a baby.
The views of these organizations are their own and do not reflect the official position of CDC.
Down Syndrome Resource Foundation (DSRF) : The DSRF supports people living with Down syndrome and their families with individualized and leading-edge educational programs, health services, information resources, and rich social connections so each person can flourish in their own right.
GiGi's Playhouse : GiGi's Playhouse provides free educational, therapeutic-based, and career development programs for individuals with Down syndrome, their families, and the community, through a replicable playhouse model.
Global Down Syndrome Foundation : This foundation is dedicated to significantly improving the lives of people with Down syndrome through research, medical care, education and advocacy.
National Association for Down Syndrome : The National Association for Down Syndrome supports all persons with Down syndrome in achieving their full potential. They seek to help families, educate the public, address social issues and challenges, and facilitate active participation.
National Down Syndrome Society (NDSS) : NDSS seeks to increase awareness and acceptance of those with Down syndrome.
- Stallings, E. B., Isenburg, J. L., Rutkowski, R. E., Kirby, R. S., Nembhard, W.N., Sandidge, T., Villavicencio, S., Nguyen, H. H., McMahon, D. M., Nestoridi, E., Pabst, L. J., for the National Birth Defects Prevention Network. National population-based estimates for major birth defects, 2016–2020. Birth Defects Research. 2024 Jan;116(1), e2301.
- Allen EG, Freeman SB, Druschel C, et al. Maternal age and risk for trisomy 21 assessed by the origin of chromosome nondisjunction: a report from the Atlanta and National Down Syndrome Projects. Hum Genet. 2009 Feb;125(1):41-52.
- Ghosh S, Feingold E, Dey SK. Etiology of Down syndrome: Evidence for consistent association among altered meiotic recombination, nondisjunction, and maternal age across populations. Am J Med Genet A. 2009 Jul;149A(7):1415-20.
- Sherman SL, Allen EG, Bean LH, Freeman SB. Epidemiology of Down syndrome. Ment Retard Dev Disabil Res Rev. 2007;13(3):221-7.
- Olsen CL, Cross PK, Gensburg LJ, Hughes JP. The effects of prenatal diagnosis, population ageing, and changing fertility rates on the live birth prevalence of Down syndrome in New York State, 1983-1992. Prenat Diagn. 1996 Nov;16(11):991-1002.
- Adams MM, Erickson JD, Layde PM, Oakley GP. Down's syndrome. Recent trends in the United States. JAMA. 1981 Aug 14;246(7):758-60.
- Morris JK, Mutton DE, Alberman E. Recurrences of free trisomy 21: analysis of data from the National Down Syndrome Cytogenetic Register. Prenatal Diagnosis: Published in Affiliation With the International Society for Prenatal Diagnosis. 2005 Dec 15;25(12):1120-8.
Birth Defects
About one in every 33 babies is born with a birth defect. Although not all birth defects can be prevented, people can increase their chances of having a healthy baby by managing health conditions and adopting healthy behaviors before becoming pregnant.
For Everyone
Health care providers, public health.
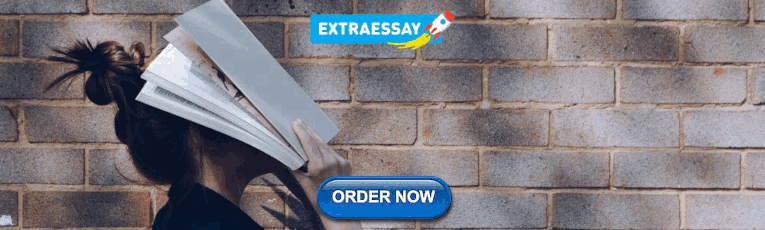
IMAGES
VIDEO
COMMENTS
Here are some examples of intervening variables: Stress: Suppose you are investigating the relationship between workload and productivity in the workplace. However, you find that stress plays a mediating role in this relationship, meaning that as workload increases, so does stress, and as stress increases, productivity decreases.
An intervening variable is a variable that affects the relationship between an independent variable and a dependent variable.. Often this type of variable can appear when researchers are studying the relationship between two variables and don't realize that another variable is actually intervening in the relationship.. Intervening variables pop up in many different research situations.
An intervening variable is a hypothetical variable used to explain causal links between other variables. Intervening variables cannot be observed in an experiment (that's why they are hypothetical). For example, there is an association between being poor and having a shorter life span. Just because someone is poor doesn't mean that will ...
An intervening variable is something that impacts the relationship between an independent and a dependent variable. Usually, the intervening variable is caused by the independent variable, and is itself a cause of the dependent variable. For example, there is an observed positive correlation between level of education and level of income, such ...
Definition. An intervening variable is an abstract scientific concept that scientists invoke to label or summarize relationships between independent and dependent variables across a variety of circumstances. Commonly cited examples of intervening variables include gravity, thirst, learning, and intelligence.
Intervening variable: An intervening variable is a variable that helps explain the relationship between two variables. Independent variable : An independent variable is the variable that the ...
Published on March 1, 2021 by Pritha Bhandari . Revised on June 22, 2023. A mediating variable (or mediator) explains the process through which two variables are related, while a moderating variable (or moderator) affects the strength and direction of that relationship. Including mediators and moderators in your research helps you go beyond ...
Intervening Variables in Psychology . Intervening variables, also sometimes called intermediate or mediator variables, are factors that play a role in the relationship between two other variables. In the previous example, sleep problems in university students are often influenced by factors such as stress.
An intervening variable is a variable that affects the relationship between an independent variable and a dependent variable. Often this type of variable can appear when researchers are studying the relationship between two variables and don't realize that another variable is actually intervening in the relationship. Intervening variables pop up in many different research situations.
An intervening variable is an abstract. scienti fic concept that scientists invoke to label. or summarize relationships between independent. and dependent variables across a variety of cir ...
The term "intervening variable," as used by behavioral theorists such as Clark Hull and Edward Tolman, describes a variable that mediates the relationship between a stimulus and response (Hilgard, 1958). Behavioral theorists were not consistent in their use of the term intervening variable and often disagreed about what even constituted an ...
This video lecture discusses very briefly the meaning and nature of intervening variables in research.Transcript of this video lecture is available at: http...
The primary focus is on mediating and moderating variables for intervention research but many issues apply to nonintervention research as well. ... Mediating and moderating variables are examples of third variables. Most research focuses on the relation between two variables—an independent variable X and an outcome variable Y. Example ...
Lastly, there is an intervening variable. An intervening, meditating variable can connect and describe the relationship between dependent and independent variables [27]. An intervening variable ...
Example (salt tolerance experiment) Independent variables (aka treatment variables) Variables you manipulate in order to affect the outcome of an experiment. The amount of salt added to each plant's water. Dependent variables (aka response variables) Variables that represent the outcome of the experiment.
A variable that changes the relationship between dependent and independent variables by strengthening or weakening the intervening variable's effect Example Access to health care: If wealth is the independent variable, and a long life span is a dependent variable, a researcher might hypothesize that access to quality health care is the ...
In the fourth piece of this series on research study designs, we look at interventional studies (clinical trials). These studies differ from observational studies in that the investigator decides whether or not a participant will receive the exposure (or intervention). In this article, we describe the key features and types of interventional ...
While the independent variable is the " cause ", the dependent variable is the " effect " - or rather, the affected variable. In other words, the dependent variable is the variable that is assumed to change as a result of a change in the independent variable. Keeping with the previous example, let's look at some dependent variables ...
Example: Confounding vs. extraneous variables Having participants who work in scientific professions (in labs) is a confounding variable in your study, because this type of work correlates with wearing a lab coat and better scientific reasoning.. People who work in labs would regularly wear lab coats and may have higher scientific knowledge in general.
Antecedent variables can be present in a variety of research scenarios. Some examples include: Example 1: Age & Income. Suppose researchers are interested in studying the relationship between age and annual income. However, an antecedent variable that could help explain (or partially explain) the relationship between the two variables that ...
A moderating variable is a type of variable that affects the relationship between a dependent variable and an independent variable.. When performing regression analysis, we're often interested in understanding how changes in an independent variable affect a dependent variable.However, sometimes a moderating variable can affect this relationship. For example, suppose we want to fit a ...
The independent variable is the cause. Its value is independent of other variables in your study. The dependent variable is the effect. Its value depends on changes in the independent variable. Example: Independent and dependent variables. You design a study to test whether changes in room temperature have an effect on math test scores.
Adaptive workplace outcomes, such as employee work engagement, job performance, and satisfaction are positively associated with physical and psychological well-being, while maladaptive workplace outcomes, including work-related disengagement, dissatisfaction, stress, boredom, fatigue, and burnout, are negatively associated with well-being. Researchers have applied self-determination theory to ...
Global Down Syndrome Foundation: This foundation is dedicated to significantly improving the lives of people with Down syndrome through research, medical care, education and advocacy. National Association for Down Syndrome: The National Association for Down Syndrome supports all persons with Down syndrome in achieving their full potential. They ...