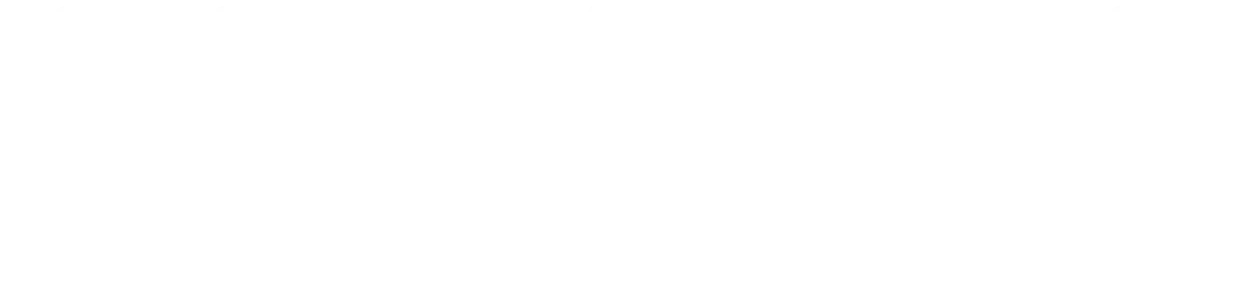
- Help Center
GET STARTED
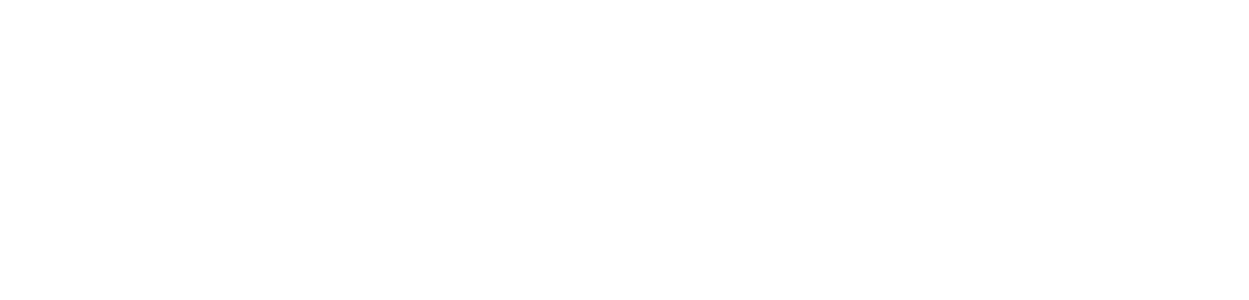
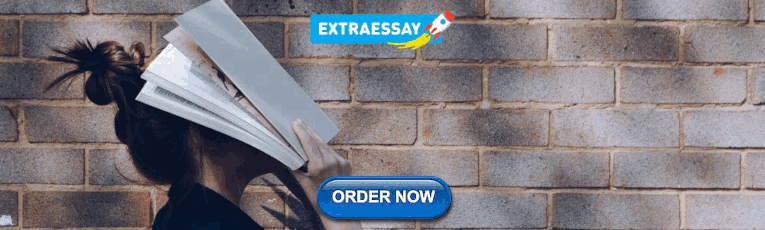
COLLABORATE ON YOUR REVIEWS WITH ANYONE, ANYWHERE, ANYTIME
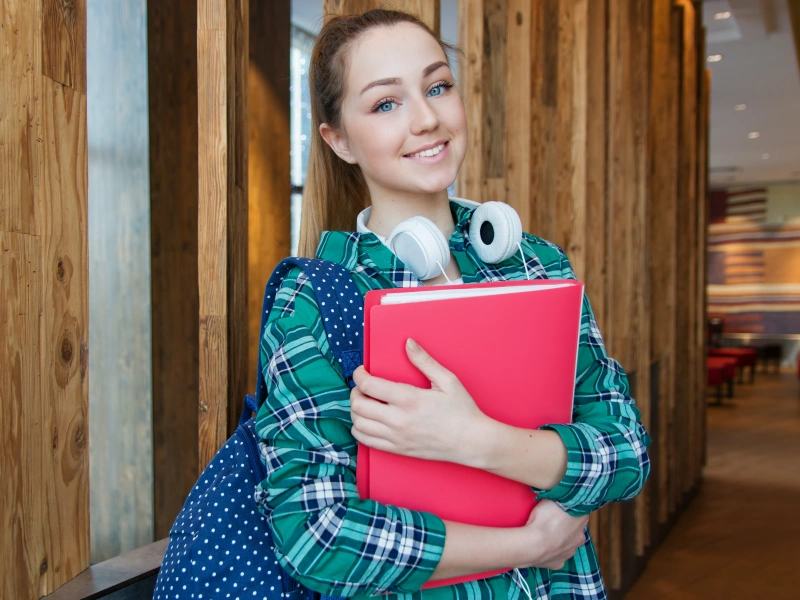
Save precious time and maximize your productivity with a Rayyan membership. Receive training, priority support, and access features to complete your systematic reviews efficiently.
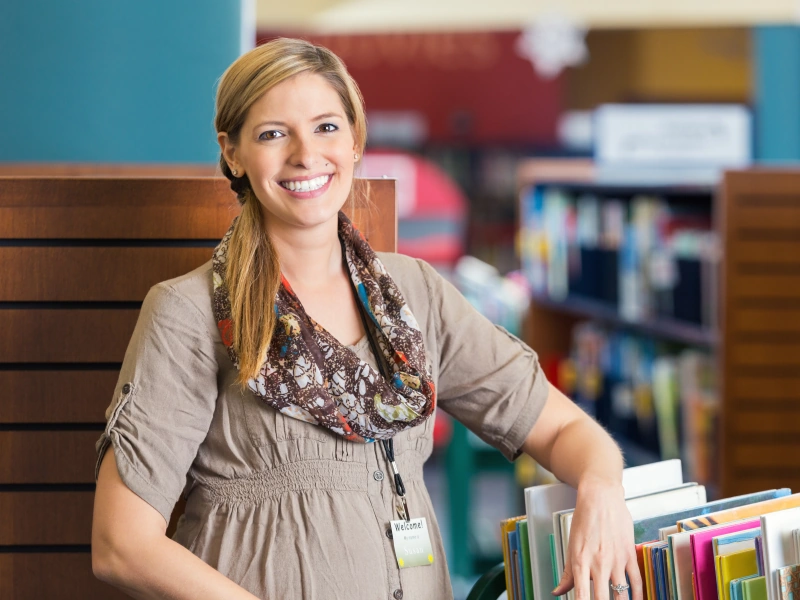
Rayyan Teams+ makes your job easier. It includes VIP Support, AI-powered in-app help, and powerful tools to create, share and organize systematic reviews, review teams, searches, and full-texts.
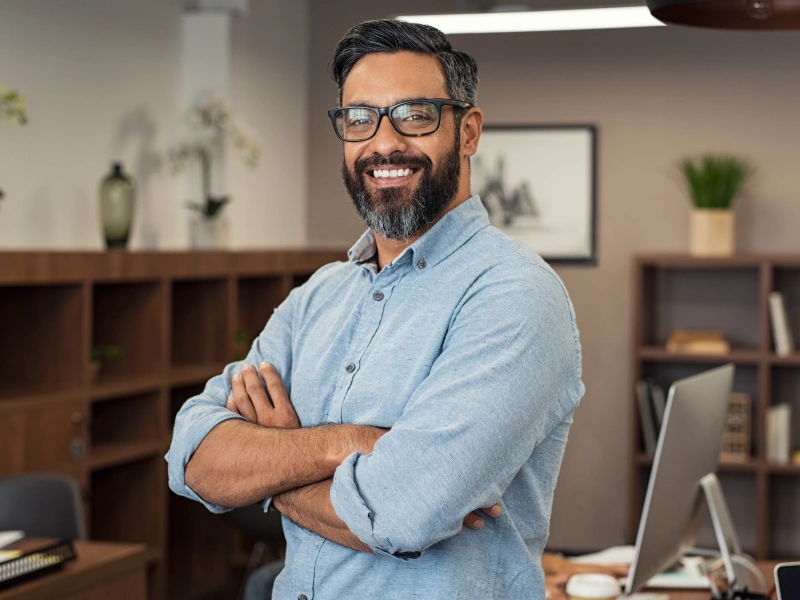
RESEARCHERS
Rayyan makes collaborative systematic reviews faster, easier, and more convenient. Training, VIP support, and access to new features maximize your productivity. Get started now!
Over 1 billion reference articles reviewed by research teams, and counting...
Intelligent, scalable and intuitive.
Rayyan understands language, learns from your decisions and helps you work quickly through even your largest systematic literature reviews.
WATCH A TUTORIAL NOW
Solutions for Organizations and Businesses
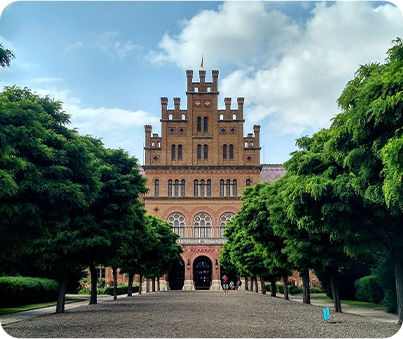
Rayyan Enterprise and Rayyan Teams+ make it faster, easier and more convenient for you to manage your research process across your organization.
- Accelerate your research across your team or organization and save valuable researcher time.
- Build and preserve institutional assets, including literature searches, systematic reviews, and full-text articles.
- Onboard team members quickly with access to group trainings for beginners and experts.
- Receive priority support to stay productive when questions arise.
- SCHEDULE A DEMO
- LEARN MORE ABOUT RAYYAN TEAMS+
RAYYAN SYSTEMATIC LITERATURE REVIEW OVERVIEW
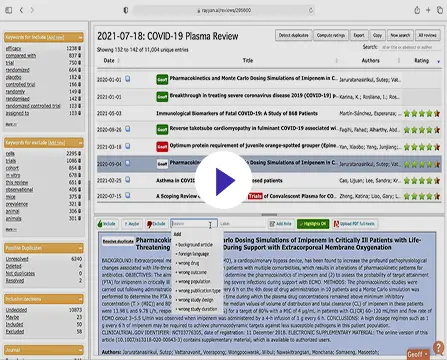
LEARN ABOUT RAYYAN’S PICO HIGHLIGHTS AND FILTERS
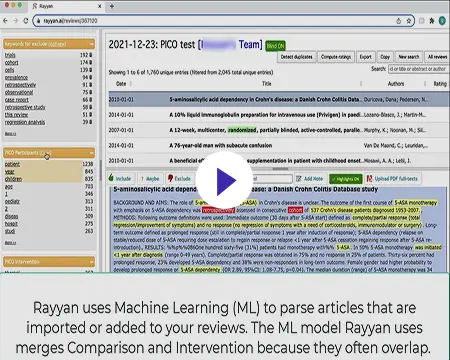
Join now to learn why Rayyan is trusted by already more than 500,000 researchers
Individual plans, teams plans.
For early career researchers just getting started with research.
Free forever
- 3 Active Reviews
- Invite Unlimited Reviewers
- Import Directly from Mendeley
- Industry Leading De-Duplication
- 5-Star Relevance Ranking
- Advanced Filtration Facets
- Mobile App Access
- 100 Decisions on Mobile App
- Standard Support
- Revoke Reviewer
- Online Training
- PICO Highlights & Filters
- PRISMA (Beta)
- Auto-Resolver
- Multiple Teams & Management Roles
- Monitor & Manage Users, Searches, Reviews, Full Texts
- Onboarding and Regular Training
Professional
For researchers who want more tools for research acceleration.
Per month billed annually
- Unlimited Active Reviews
- Unlimited Decisions on Mobile App
- Priority Support
- Auto-Resolver
For currently enrolled students with valid student ID.
Per month billed annually
Billed monthly
For a team that wants professional licenses for all members.
Per-user, per month, billed annually
- Single Team
- High Priority Support
For teams that want support and advanced tools for members.
- Multiple Teams
- Management Roles
For organizations who want access to all of their members.
Annual Subscription
Contact Sales
- Organizational Ownership
- For an organization or a company
- Access to all the premium features such as PICO Filters, Auto-Resolver, PRISMA and Mobile App
- Store and Reuse Searches and Full Texts
- A management console to view, organize and manage users, teams, review projects, searches and full texts
- Highest tier of support – Support via email, chat and AI-powered in-app help
- GDPR Compliant
- Single Sign-On
- API Integration
- Training for Experts
- Training Sessions Students Each Semester
- More options for secure access control
ANNUAL ONLY
Per-user, billed monthly
Rayyan Subscription
membership starts with 2 users. You can select the number of additional members that you’d like to add to your membership.
Total amount:
Click Proceed to get started.
Great usability and functionality. Rayyan has saved me countless hours. I even received timely feedback from staff when I did not understand the capabilities of the system, and was pleasantly surprised with the time they dedicated to my problem. Thanks again!
This is a great piece of software. It has made the independent viewing process so much quicker. The whole thing is very intuitive.
Rayyan makes ordering articles and extracting data very easy. A great tool for undertaking literature and systematic reviews!
Excellent interface to do title and abstract screening. Also helps to keep a track on the the reasons for exclusion from the review. That too in a blinded manner.
Rayyan is a fantastic tool to save time and improve systematic reviews!!! It has changed my life as a researcher!!! thanks
Easy to use, friendly, has everything you need for cooperative work on the systematic review.
Rayyan makes life easy in every way when conducting a systematic review and it is easy to use.
Log in using your username and password
- Search More Search for this keyword Advanced search
- Latest content
- For authors
- Browse by collection
- BMJ Journals More You are viewing from: Google Indexer
You are here
- Volume 13, Issue 7
- Artificial intelligence in systematic reviews: promising when appropriately used
- Article Text
- Article info
- Citation Tools
- Rapid Responses
- Article metrics

- http://orcid.org/0000-0003-1727-0608 Sanne H B van Dijk 1 , 2 ,
- Marjolein G J Brusse-Keizer 1 , 3 ,
- Charlotte C Bucsán 2 , 4 ,
- http://orcid.org/0000-0003-1071-6769 Job van der Palen 3 , 4 ,
- Carine J M Doggen 1 , 5 ,
- http://orcid.org/0000-0002-2276-5691 Anke Lenferink 1 , 2 , 5
- 1 Health Technology & Services Research, Technical Medical Centre , University of Twente , Enschede , The Netherlands
- 2 Pulmonary Medicine , Medisch Spectrum Twente , Enschede , The Netherlands
- 3 Medical School Twente , Medisch Spectrum Twente , Enschede , The Netherlands
- 4 Cognition, Data & Education, Faculty of Behavioural, Management & Social Sciences , University of Twente , Enschede , The Netherlands
- 5 Clinical Research Centre , Rijnstate Hospital , Arnhem , The Netherlands
- Correspondence to Dr Anke Lenferink; a.lenferink{at}utwente.nl
Background Systematic reviews provide a structured overview of the available evidence in medical-scientific research. However, due to the increasing medical-scientific research output, it is a time-consuming task to conduct systematic reviews. To accelerate this process, artificial intelligence (AI) can be used in the review process. In this communication paper, we suggest how to conduct a transparent and reliable systematic review using the AI tool ‘ASReview’ in the title and abstract screening.
Methods Use of the AI tool consisted of several steps. First, the tool required training of its algorithm with several prelabelled articles prior to screening. Next, using a researcher-in-the-loop algorithm, the AI tool proposed the article with the highest probability of being relevant. The reviewer then decided on relevancy of each article proposed. This process was continued until the stopping criterion was reached. All articles labelled relevant by the reviewer were screened on full text.
Results Considerations to ensure methodological quality when using AI in systematic reviews included: the choice of whether to use AI, the need of both deduplication and checking for inter-reviewer agreement, how to choose a stopping criterion and the quality of reporting. Using the tool in our review resulted in much time saved: only 23% of the articles were assessed by the reviewer.
Conclusion The AI tool is a promising innovation for the current systematic reviewing practice, as long as it is appropriately used and methodological quality can be assured.
PROSPERO registration number CRD42022283952.
- systematic review
- statistics & research methods
- information technology
This is an open access article distributed in accordance with the Creative Commons Attribution 4.0 Unported (CC BY 4.0) license, which permits others to copy, redistribute, remix, transform and build upon this work for any purpose, provided the original work is properly cited, a link to the licence is given, and indication of whether changes were made. See: https://creativecommons.org/licenses/by/4.0/ .
https://doi.org/10.1136/bmjopen-2023-072254
Statistics from Altmetric.com
Request permissions.
If you wish to reuse any or all of this article please use the link below which will take you to the Copyright Clearance Center’s RightsLink service. You will be able to get a quick price and instant permission to reuse the content in many different ways.
Strengths and limitations of this study
Potential pitfalls regarding the use of artificial intelligence in systematic reviewing were identified.
Remedies for each pitfall were provided to ensure methodological quality. A time-efficient approach is suggested on how to conduct a transparent and reliable systematic review using an artificial intelligence tool.
The artificial intelligence tool described in the paper was not evaluated for its accuracy.
Medical-scientific research output has grown exponentially since the very first medical papers were published. 1–3 The output in the field of clinical medicine increased and keeps doing so. 4 To illustrate, a quick PubMed search for ‘cardiology’ shows a fivefold increase in annual publications from 10 420 (2007) to 52 537 (2021). Although the medical-scientific output growth rate is not higher when compared with other scientific fields, 1–3 this field creates the largest output. 3 Staying updated by reading all published articles is therefore not feasible. However, systematic reviews facilitate up-to-date and accessible summaries of evidence, as they synthesise previously published results in a transparent and reproducible manner. 5 6 Hence, conclusions can be drawn that provide the highest considered level of evidence in medical research. 5 7 Therefore, systematic reviews are not only crucial in science, but they have a large impact on clinical practice and policy-making as well. 6 They are, however, highly labour-intensive to conduct due to the necessity of screening a large amount of articles, which results in a high consumption of research resources. Thus, efficient and innovative reviewing methods are desired. 8
An open-source artificial intelligence (AI) tool ‘ASReview’ 9 was published in 2021 to facilitate the title and abstract screening process in systematic reviews. Applying this tool facilitates researchers to conduct more efficient systematic reviews: simulations already showed its time-saving potential. 9–11 We used the tool in the study selection of our own systematic review and came across scenarios that needed consideration to prevent loss of methodological quality. In this communication paper, we provide a reliable and transparent AI-supported systematic reviewing approach.
We first describe how the AI tool was used in a systematic review conducted by our research group. For more detailed information regarding searches and eligibility criteria of the review, we refer to the protocol (PROSPERO registry: CRD42022283952). Subsequently, when deciding on the AI screening-related methodology, we applied appropriate remedies against foreseen scenarios and their pitfalls to maintain a reliable and transparent approach. These potential scenarios, pitfalls and remedies will be discussed in the Results section.
In our systematic review, the AI tool ‘ASReview’ (V.0.17.1) 9 was used for the screening of titles and abstracts by the first reviewer (SHBvD). The tool uses an active researcher-in-the-loop machine learning algorithm to rank the articles from high to low probability of eligibility for inclusion by text mining. The AI tool offers several classifier models by which the relevancy of the included articles can be determined. 9 In a simulation study using six large systematic review datasets on various topics, a Naïve Bayes (NB) and a term frequency-inverse document frequency (TF-IDF) outperformed other model settings. 10 The NB classifier estimates the probability of an article being relevant, based on TF-IDF measurements. TF-IDF measures the originality of a certain word within the article relative to the total number of articles the word appears in. 12 This combination of NB and TF-IDF was chosen for our systematic review.
Before the AI tool can be used for the screening of relevant articles, its algorithm needs training with at least one relevant and one irrelevant article (ie, prior knowledge). It is assumed that the more prior knowledge, the better the algorithm is trained at the start of the screening process, and the faster it will identify relevant articles. 9 In our review, the prior knowledge consisted of three relevant articles 13–15 selected from a systematic review on the topic 16 and three randomly picked irrelevant articles.
After training with the prior knowledge, the AI tool made a first ranking of all unlabelled articles (ie, articles not yet decided on eligibility) from highest to lowest probability of being relevant. The first reviewer read the title and abstract of the number one ranked article and made a decision (‘relevant’ or ‘irrelevant’) following the eligibility criteria. Next, the AI tool took into account this additional knowledge and made a new ranking. Again, the next top ranked article was proposed to the reviewer, who made a decision regarding eligibility. This process of AI making rankings and the reviewer making decisions, which is also called ‘researcher-in-the-loop’, was repeated until the predefined data-driven stopping criterion of – in our case – 100 subsequent irrelevant articles was reached. After the reviewer rejected what the AI tool puts forward as ‘most probably relevant’ a hundred times, it was assumed that there were no relevant articles left in the unseen part of the dataset.
The articles that were labelled relevant during the title and abstract screening were each screened on full text independently by two reviewers (SHBvD and MGJB-K, AL, JvdP, CJMD, CCB) to minimise the influence of subjectivity on inclusion. Disagreements regarding inclusion were solved by a third independent reviewer.
How to maintain reliability and transparency when using AI in title and abstract screening
A summary of the potential scenarios, and their pitfalls and remedies, when using the AI tool in a systematic review is given in table 1 . These potential scenarios should not be ignored, but acted on to maintain reliability and transparency. Figure 1 shows when and where to act on during the screening process reflected by the Preferred Reporting Items for Systematic Reviews and Meta-Analyses (PRISMA) flowchart, 17 from literature search results to publishing the review.
- Download figure
- Open in new tab
- Download powerpoint
Flowchart showing when and where to act on when using ASReview in systematic reviewing. Adapted the PRISMA flowchart from Haddaway et al . 17
- View inline
Per-scenario overview of potential pitfalls and how to prevent these when using ASReview in a systematic review
In our systematic review, by means of broad literature searches in several scientific databases, a first set of potentially relevant articles was identified, yielding 8456 articles, enough to expect the AI tool to be efficient in the title and abstract screening (scenario ① was avoided, see table 1 ). Subsequently, this complete set of articles was uploaded in reference manager EndNote X9 18 and review manager Covidence, 19 where 3761 duplicate articles were removed. Given that EndNote has quite low sensitivity in identifying duplicates, additional deduplication in Covidence was considered beneficial. 20 Deduplication is usually applied in systematic reviewing, 20 but is increasingly important prior to the use of AI. Since multiple decisions regarding a duplicate article weigh more than one, this will disproportionately influence classification and possibly the results ( table 1 , scenario ② ). In our review, a deduplicated set of articles was uploaded in the AI tool. Prior to the actual AI-supported title and abstract screening, the reviewers (SHBvD and AL, MGJB-K) trained themselves with a small selection of 74 articles. The first reviewer became familiar with the ASReview software, and all three reviewers learnt how to apply the eligibility criteria, to minimise personal influence on the article selection ( table 1 , scenario ③ ).
Defining the stopping criterion used in the screening process is left to the reviewer. 9 An optimal stopping criterion in active learning is considered a perfectly balanced trade-off between a certain cost (in terms of time spent) of screening one more article versus the predictive performance (in terms of identifying a new relevant article) that could be increased by adding one more decision. 21 The optimal stopping criterion in systematic reviewing would be the moment that screening additional articles will not result in more relevant articles being identified. 22 Therefore, in our review, we predetermined a data-driven stopping criterion for the title and abstract screening as ‘100 consecutive irrelevant articles’ in order to prevent the screening from being stopped before or a long time after all relevant articles were identified ( table 1 , scenario ④ ).
Due to the fact that the stopping criterion was reached after 1063 of the 4695 articles, only a part of the total number of articles was seen. Therefore, this approach might be sensitive to possible mistakes when articles are screened by only one reviewer, influencing the algorithm, possibly resulting in an incomplete selection of articles ( table 1 , scenario ③ ). 23 As a remedy, second reviewers (AL, MGJB-K) checked 20% of the titles and abstracts seen by the first reviewer. This 20% had a comparable ratio regarding relevant versus irrelevant articles over all articles seen. The percentual agreement and Cohen’s Kappa (κ), a measure for the inter-reviewer agreement above chance, were calculated to express the reliability of the decisions taken. 24 The decisions were agreed in 96% and κ was 0.83. A κ equal of at least 0.6 is generally considered high, 24 and thus it was assumed that the algorithm was reliably trained by the first reviewer.
The reporting of the use of the AI tool should be transparent. If the choices made regarding the use of the AI tool are not entirely reported ( table 1 , scenario ⑤ ), the reader will not be able to properly assess the methodology of the review, and review results may even be graded as low-quality due to the lack of transparent reporting. The ASReview tool offers the possibility to extract a data file providing insight into all decisions made during the screening process, in contrast to various other ‘black box’ AI-reviewing tools. 9 This file will be published alongside our systematic review to provide full transparency of our AI-supported screening. This way, the screening with AI is reproducible (remedy to scenario ⑥ , table 1 ).
Results of AI-supported study selection in a systematic review
We experienced an efficient process of title and abstract screening in our systematic review. Whereas the screening was performed with a database of 4695 articles, the stopping criterion was reached after 1063 articles, so 23% were seen. Figure 2A shows the proportion of articles identified as being relevant at any point during the AI-supported screening process. It can be observed that the articles are indeed prioritised by the active learning algorithm: in the beginning, relatively many relevant articles were found, but this decreased as the stopping criterion (vertical red line) was approached. Figure 2B compares the screening progress when using the AI tool versus manual screening. The moment the stopping criterion was reached, approximately 32 records would have been found when the titles and abstract would have been screened manually, compared with 142 articles labelled relevant using the AI tool. After the inter-reviewer agreement check, 142 articles proceeded to the full text reviewing phase, of which 65 were excluded because these were no articles with an original research format, and three because the full text could not be retrieved. After full text reviewing of the remaining 74 articles, 18 articles from 13 individual studies were included in our review. After snowballing, one additional article from a study already included was added.
Relevant articles identified after a certain number of titles and abstracts were screened using the AI tool compared with manual screening.
In our systematic review, the AI tool considerably reduced the number of articles in the screening process. Since the AI tool is offered open source, many researchers may benefit from its time-saving potential in selecting articles. Choices in several scenarios regarding the use of AI, however, are still left open to the researcher, and need consideration to prevent pitfalls. These include the choice whether or not to use AI by weighing the costs versus the benefits, the importance of deduplication, double screening to check inter-reviewer agreement, a data-driven stopping criterion to optimally use the algorithm’s predictive performance and quality of reporting of the AI-related methodology chosen. This communication paper is, to our knowledge, the first elaborately explaining and discussing these choices regarding the application of this AI tool in an example systematic review.
The main advantage of using the AI tool is the amount of time saved. Indeed, in our study, only 23% of the total number of articles were screened before the predefined stopping criterion was met. Assuming that all relevant articles were found, the AI tool saved 77% of the time for title and abstract screening. However, time should be invested to become acquainted with the tool. Whether the expected screening time saved outweighs this time investment is context-dependent (eg, researcher’s digital skills, systematic reviewing skills, topic knowledge). An additional advantage is that research questions previously unanswerable due to the insurmountable number of articles to screen in a ‘classic’ (ie, manual) review, now actually are possible to answer. An example of the latter is a review screening over 60 000 articles, 25 which would probably never have been performed without AI supporting the article selection.
Since the introduction of the ASReview tool in 2021, it was applied in seven published reviews. 25–31 An important note to make is that only one 25 clearly reported AI-related choices in the methods and a complete and transparent flowchart reflecting the study selection process in the Results section. Two reviews reported a relatively small number (<400) of articles to screen, 26 27 of which more than 75% of the articles were screened before the stopping criterion was met, so the amount of time saved was limited. Also, three reviews reported many initial articles (>6000) 25 28 29 and one reported 892 articles, 31 of which only 5%–10% needed to be screened. So in these reviews, the AI tool saved an impressive amount of screening time. In our systematic review, 3% of the articles were labelled relevant during the title and abstract screening and eventually, <1% of all initial articles were included. These percentages are low, and are in line with the three above-mentioned reviews (1%–2% and 0%–1%, respectively). 25 28 29 Still, relevancy and inclusion rates are much lower when compared with ‘classic’ systematic reviews. A study evaluating the screening process in 25 ‘classic’ systematic reviews showed that approximately 18% was labelled relevant and 5% was actually included in the reviews. 32 This difference is probably due to more narrow literature searches in ‘classic’ reviews for feasibility purposes compared with AI-supported reviews, resulting in a higher proportion of included articles.
In this paper, we show how we applied the AI tool, but we did not evaluate it in terms of accuracy. This means that we have to deal with a certain degree of uncertainty. Despite the data-driven stopping criterion there is a chance that relevant articles were missed, as 77% was automatically excluded. Considering this might have been the case, first, this could be due to wrong decisions of the reviewer that would have undesirably influenced the training of the algorithm by which the articles were labelled as (ir)relevant and the order in which they were presented to the reviewer. Relevant articles could have therefore remained unseen if the stopping criterion was reached before they were presented to the reviewer. As a remedy, in our own systematic review, of the 20% of the articles screened by the first reviewer, relevancy was also assessed by another reviewer to assess inter-reviewer reliability, which was high. It should be noted, though, that ‘classic’ title and abstract screening is not necessarily better than using AI, as medical-scientific researchers tend to assess one out of nine abstracts wrongly. 32 Second, the AI tool may not have properly ranked highly relevant to irrelevant articles. However, given that simulations proved this AI tool’s accuracy before 9–11 this was not considered plausible. Since our study applied, but did not evaluate, the AI tool, we encourage future studies evaluating the performance of the tool across different scientific disciplines and contexts, since research suggests that the tool’s performance depends on the context, for example, the complexity of the research question. 33 This could not only enrich the knowledge about the AI tool, but also increases certainty about using it. Also, future studies should investigate the effects of choices made regarding the amount of prior knowledge that is provided to the tool, the number of articles defining the stopping criterion, and how duplicate screening is best performed, to guide future users of the tool.
Although various researcher-in-the-loop AI tools for title and abstract screening have been developed over the years, 9 23 34 they often do not develop into usable mature software, 34 which impedes AI to be permanently implemented in research practice. For medical-scientific research practice, it would therefore be helpful if large systematic review institutions, like Cochrane and PRISMA, would consider to ‘officially’ make AI part of systematic reviewing practice. When guidelines on the use of AI in systematic reviews are made available and widely recognised, AI-supported systematic reviews can be uniformly conducted and transparently reported. Only then we can really benefit from AI’s time-saving potential and reduce our research time waste.
Our experience with the AI tool during the title and abstract screening was positive as it has highly accelerated the literature selection process. However, users should consider applying appropriate remedies to scenarios that may form a threat to the methodological quality of the review. We provided an overview of these scenarios, their pitfalls and remedies. These encourage reliable use and transparent reporting of AI in systematic reviewing. To ensure the continuation of conducting systematic reviews in the future, and given their importance for medical guidelines and practice, we consider this tool as an important addition to the review process.
Ethics approval
Not applicable.
- Bornmann L ,
- Haunschild R ,
- Michels C ,
- Haghani M ,
- Zwack CC , et al
- McKenzie JE ,
- Bossuyt PM , et al
- Gurevitch J ,
- Koricheva J ,
- Nakagawa S , et al
- Rohrich RJ ,
- Bastian H ,
- Glasziou P ,
- van de Schoot R ,
- de Bruin J ,
- Schram R , et al
- Ferdinands G ,
- de Bruin J , et al
- Ferdinands G
- Havrlant L ,
- Kreinovich V
- Li Y , et al
- Jalloul F ,
- Ayed S , et al
- Andrijevic I ,
- Milutinov S ,
- Lozanov Crvenkovic Z , et al
- Hawkins NM ,
- Virani SA , et al
- Haddaway NR ,
- Pritchard CC , et al
- Clarivate Analytics
- Veritas Health Innovation
- McKeown S ,
- Ishibashi H ,
- Blaizot A ,
- Veettil SK ,
- Saidoung P , et al
- Bernardes RC ,
- Botina LL ,
- Araújo R dos S , et al
- Silva GFS ,
- Fagundes TP ,
- Teixeira BC , et al
- Miranda L ,
- Pütz B , et al
- Schouw HM ,
- Huisman LA ,
- Janssen YF , et al
- Schuengel C ,
- Sterkenburg PS , et al
- Procházková M ,
- Lu J , et al
- Lam L , et al
- Tetzlaff J , et al
- Marshall IJ ,
Contributors SHBvD proposed the methodology and conducted the study selection. MGJB-K, CJMD and AL critically reflected on the methodology. MGJB-K and AL contributed substantially to the study selection. CCB, JvdP and CJMD contributed to the study selection. The manuscript was primarily prepared by SHBvD and critically revised by all authors. All authors read and approved the final manuscript.
Funding The systematic review is conducted as part of the RE-SAMPLE project. RE-SAMPLE has received funding from the European Union’s Horizon 2020 research and innovation programme (grant agreement no. 965315).
Competing interests None declared.
Provenance and peer review Not commissioned; externally peer reviewed.
Read the full text or download the PDF:
Using artificial intelligence methods for systematic review in health sciences: A systematic review
Affiliations.
- 1 Department of Pharmacotherapy, College of Pharmacy, University of Utah, Utah, USA.
- 2 Faculty of Pharmacy, Chiang Mai University, Chiang Mai, Thailand.
- 3 School of Computing, Robert Gordon University, Aberdeen, Scotland, UK.
- 4 The Rowett Institute, University of Aberdeen, Aberdeen, Scotland, UK.
- 5 School of Medicine, Faculty of Health and Medical Sciences, Taylors University, Selangor, Malaysia.
- 6 School of Pharmacy, Monash University Malaysia, Selangor, Malaysia.
- 7 IDEAS Center, Veterans Affairs Salt Lake City Healthcare System, Salt Lake City, Utah, USA.
- PMID: 35174972
- DOI: 10.1002/jrsm.1553
The exponential increase in published articles makes a thorough and expedient review of literature increasingly challenging. This review delineated automated tools and platforms that employ artificial intelligence (AI) approaches and evaluated the reported benefits and challenges in using such methods. A search was conducted in 4 databases (Medline, Embase, CDSR, and Epistemonikos) up to April 2021 for systematic reviews and other related reviews implementing AI methods. To be included, the review must use any form of AI method, including machine learning, deep learning, neural network, or any other applications used to enable the full or semi-autonomous performance of one or more stages in the development of evidence synthesis. Twelve reviews were included, using nine different tools to implement 15 different AI methods. Eleven methods were used in the screening stages of the review (73%). The rest were divided: two in data extraction (13%) and two in risk of bias assessment (13%). The ambiguous benefits of the data extractions, combined with the reported advantages from 10 reviews, indicating that AI platforms have taken hold with varying success in evidence synthesis. However, the results are qualified by the reliance on the self-reporting of the review authors. Extensive human validation still appears required at this stage in implementing AI methods, though further evaluation is required to define the overall contribution of such platforms in enhancing efficiency and quality in evidence synthesis.
Keywords: artificial intelligence; evidence synthesis; machine learning; systematic reviews.
© 2022 John Wiley & Sons Ltd.
Publication types
- Systematic Review
- Artificial Intelligence*
- Machine Learning
- Systematic Reviews as Topic*
Thank you for visiting nature.com. You are using a browser version with limited support for CSS. To obtain the best experience, we recommend you use a more up to date browser (or turn off compatibility mode in Internet Explorer). In the meantime, to ensure continued support, we are displaying the site without styles and JavaScript.
- View all journals
- Explore content
- About the journal
- Publish with us
- Sign up for alerts
- Correspondence
- Published: 16 January 2023
PRISMA AI reporting guidelines for systematic reviews and meta-analyses on AI in healthcare
- Giovanni E. Cacciamani ORCID: orcid.org/0000-0002-8892-5539 1 , 2 , 3 , 4 , 5 ,
- Timothy N. Chu 1 , 2 , 3 ,
- Daniel I. Sanford 1 , 2 , 3 ,
- Andre Abreu 1 , 2 , 3 , 4 , 5 ,
- Vinay Duddalwar ORCID: orcid.org/0000-0002-4808-5715 3 , 6 ,
- Assad Oberai 7 , 8 ,
- C.-C. Jay Kuo 9 ,
- Xiaoxuan Liu 10 , 11 , 12 ,
- Alastair K. Denniston ORCID: orcid.org/0000-0001-7849-0087 10 , 11 , 12 , 13 , 14 ,
- Baptiste Vasey 15 , 16 ,
- Peter McCulloch ORCID: orcid.org/0000-0002-3210-8273 15 ,
- Robert F. Wolff 17 ,
- Sue Mallett ORCID: orcid.org/0000-0002-0596-8200 18 ,
- John Mongan 19 , 20 ,
- Charles E. Kahn Jr ORCID: orcid.org/0000-0002-6654-7434 21 ,
- Viknesh Sounderajah 22 ,
- Ara Darzi ORCID: orcid.org/0000-0001-7815-7989 22 ,
- Philipp Dahm 23 ,
- Karel G. M. Moons 24 ,
- Eric Topol ORCID: orcid.org/0000-0002-1478-4729 25 ,
- Gary S. Collins ORCID: orcid.org/0000-0002-2772-2316 26 ,
- David Moher ORCID: orcid.org/0000-0003-2434-4206 27 ,
- Inderbir S. Gill 1 , 2 , 3 , 4 , 5 &
- Andrew J. Hung 1 , 2 , 5
Nature Medicine volume 29 , pages 14–15 ( 2023 ) Cite this article
3945 Accesses
23 Citations
84 Altmetric
Metrics details
- Research data
- Translational research
Systematic reviews and meta-analyses play an essential part in guiding clinical practice at the point of care, as well as in the formulation of clinical practice guidelines and health policy 1 , 2 . There are three essential components to an impactful systematic review. First, the design of a study should be based upon a robust research question and search strategy. Second, minimization of bias should be enhanced by using quality-assessment tools and study-design-specific eligibility criteria. Third, reporting of results should be conducted transparently through adherence to expert-derived reporting items. Thousands of systematic reviews, including meta-analyses, are produced annually, with an increasing proportion reporting on artificial intelligence (AI) interventions in health care. With this rapid expansion, there is a need for reporting guidelines tailored to AI 3 , 4 , 5 , 6 , 7 that will support high-quality, reproducible, and clinically relevant systematic reviews.
AI is being integrated rapidly into society and in medicine. A literature search of studies referencing AI in health care over the past 20 years returned more than 70,000 published articles. Given that interest in AI is reaching an all-time high, there arise new concerns regarding the quality of these studies, including a lack of: clear explainability of how AI algorithms function; strong evidence of effectiveness in clinical settings; and standardized reporting within primary studies. Efforts have been made to improve understanding of this technology to allow for critical appraisal of AI interventions and to reduce inconsistencies in how studies are structured, as well as reporting of data, methods and results 3 , 5 , 7 . As systematic reviews on AI interventions increase, so does the importance of the transparency and reproducibility of reported data.
This is a preview of subscription content, access via your institution
Relevant articles
Open Access articles citing this article.
Predicting non-muscle invasive bladder cancer outcomes using artificial intelligence: a systematic review using APPRAISE-AI
- Jethro C. C. Kwong
- , Jeremy Wu
- … Girish S. Kulkarni
npj Digital Medicine Open Access 18 April 2024
Reporting guidelines in medical artificial intelligence: a systematic review and meta-analysis
- Fiona R. Kolbinger
- , Gregory P. Veldhuizen
- … Jakob Nikolas Kather
Communications Medicine Open Access 11 April 2024
Human-AI interaction in skin cancer diagnosis: a systematic review and meta-analysis
- Isabelle Krakowski
- , Jiyeong Kim
- … Eleni Linos
npj Digital Medicine Open Access 09 April 2024
Access options
Access Nature and 54 other Nature Portfolio journals
Get Nature+, our best-value online-access subscription
24,99 € / 30 days
cancel any time
Subscribe to this journal
Receive 12 print issues and online access
195,33 € per year
only 16,28 € per issue
Buy this article
- Purchase on Springer Link
- Instant access to full article PDF
Prices may be subject to local taxes which are calculated during checkout
Page, M. J. et al. Syst. Rev. 10 , 89 (2021).
Article Google Scholar
Moher, D. et al. Epidemiology 22 , 128 (2011).
Liu, X. et al. Nat. Med. 26 , 1364–1374 (2020).
Article CAS Google Scholar
Mongan, J. et al. Radiol. Artif. Int. 2 , e200029 (2020).
Sounderajah, V. et al. Nat. Med. 26 , 807–808 (2020).
Vasey, B. et al. Nat. Med. 28 , 924–933 (2022).
Cruz Rivera, S. et al. Nat. Med. 26 , 1351–1363 (2020).
The CONSORT-AI and SPIRIT-AI Steering Group. Nat. Med. 25 , 1467–1468 (2019).
Sounderajah, V. et al. Nat. Med. 27 , 1663–1665 (2021).
Moher, D. et al. PLoS Med. 7 , e1000217 (2010).
Download references
Author information
Authors and affiliations.
USC Institute of Urology and Catherine and Joseph Aresty Department of Urology, Keck School of Medicine, University of Southern California, Los Angeles, CA, USA
Giovanni E. Cacciamani, Timothy N. Chu, Daniel I. Sanford, Andre Abreu, Inderbir S. Gill & Andrew J. Hung
AI Center at USC Urology, USC Institute of Urology, University of Southern California, Los Angeles, CA, USA
Department of Radiology, University of Southern California, Los Angeles, CA, USA
Giovanni E. Cacciamani, Timothy N. Chu, Daniel I. Sanford, Andre Abreu, Vinay Duddalwar & Inderbir S. Gill
Center for Image-Guided and Focal Therapy for Prostate Cancer, Institute of Urology and Catherine and Joseph Aresty Department of Urology, Keck School of Medicine, University of Southern California, Los Angeles, CA, USA
Giovanni E. Cacciamani, Andre Abreu & Inderbir S. Gill
Norris Comprehensive Cancer Center, Institute of Urology, Keck School of Medicine of the University of Southern California, Los Angeles, CA, USA
Giovanni E. Cacciamani, Andre Abreu, Inderbir S. Gill & Andrew J. Hung
USC Radiomics Laboratory, Keck School of Medicine, Department of Radiology, University of Southern California, Los Angeles, CA, USA
Vinay Duddalwar
Department of Aerospace and Mechanical Engineering, Viterbi School of Engineering, University of Southern California, Los Angeles, CA, USA
Assad Oberai
Department of Biomedical Engineering, Viterbi School of Engineering, University of Southern California, Los Angeles, CA, USA
Ming Hsieh Department of Electrical and Computer Engineering, University of Southern California, Los Angeles, CA, USA
C.-C. Jay Kuo
University Hospitals Birmingham NHS Foundation Trust, Birmingham, UK
Xiaoxuan Liu & Alastair K. Denniston
Institute of Inflammation and Ageing, College of Medical and Dental Sciences, University of Birmingham, Birmingham, UK
Birmingham Health Partners Centre for Regulatory Science and Innovation, University of Birmingham, Birmingham, UK
NIHR Birmingham Biomedical Research Centre, University of Birmingham, Birmingham, UK
Alastair K. Denniston
Health Data Research, London, UK
Nuffield Department of Surgical Sciences, University of Oxford, Oxford, UK
Baptiste Vasey & Peter McCulloch
Department of Surgery, Geneva University Hospital, Geneva, Switzerland
Baptiste Vasey
Kleijnen Systematic Reviews Ltd, Escrick, York, UK
Robert F. Wolff
Centre for Medical Imaging, University College London, London, UK
Sue Mallett
Department of Radiology and Biomedical Imaging, University of California San Francisco, San Francisco, CA, USA
John Mongan
Center for Intelligent Imaging, University of California San Francisco, San Francisco, CA, USA
Department of Radiology and Institute for Biomedical Informatics, University of Pennsylvania, Philadelphia, PA, USA
Charles E. Kahn Jr
Institute of Global Health Innovation, Imperial College London, London, UK
Viknesh Sounderajah & Ara Darzi
Minneapolis VAMC, Urology Section and University of Minnesota, Department of Urology, Minneapolis, MN, USA
Philipp Dahm
Julius Center for Health Sciences and Primary Care, UMC Utrecht, Utrecht University, Utrecht, The Netherlands
Karel G. M. Moons
Scripps Research Translational Institute, Scripps Research, La Jolla, CA, USA
Centre for Statistics in Medicine, Nuffield Department of Orthopaedics, Rheumatology & Musculoskeletal Sciences, University of Oxford, Oxford, UK
Gary S. Collins
Centre for Journalology, Clinical Epidemiology Program, Ottawa Hospital Research Institute, Ottawa, Canada
David Moher
You can also search for this author in PubMed Google Scholar
Corresponding author
Correspondence to Giovanni E. Cacciamani .
Ethics declarations
Competing interests.
P.D. serves as coordinating editor of Cochrane Urology . G.S.C. is the director of the UK EQUATOR Centre. D.M. is the director of the Canadian EQUATOR Centre. X.L. is an industry fellow (observer) with Hardian Health. A.J.H. is a consultant for Intuitive. I.S.G. is a consultant for STEBA. J.M. is a consultant for Siemens. C.E.K. receives salary support as editor of Radiology: Artificial Intelligence . V.D. is a consultant for Radmetrix Inc. and Westat Inc., and is an advisory board member to Deeptek Inc. A.K.D. is chair of the Health Security initiative at Flagship Pioneering UK Ltd. The other authors declare no competing interests.
Rights and permissions
Reprints and permissions
About this article
Cite this article.
Cacciamani, G.E., Chu, T.N., Sanford, D.I. et al. PRISMA AI reporting guidelines for systematic reviews and meta-analyses on AI in healthcare. Nat Med 29 , 14–15 (2023). https://doi.org/10.1038/s41591-022-02139-w
Download citation
Published : 16 January 2023
Issue Date : January 2023
DOI : https://doi.org/10.1038/s41591-022-02139-w
Share this article
Anyone you share the following link with will be able to read this content:
Sorry, a shareable link is not currently available for this article.
Provided by the Springer Nature SharedIt content-sharing initiative
This article is cited by
A whirl of radiomics-based biomarkers in cancer immunotherapy, why is large scale validation still lacking.
- Marta Ligero
- Bente Gielen
- Raquel Perez-Lopez
npj Precision Oncology (2024)
Artificial intelligence in surgery
- Chris Varghese
- Ewen M. Harrison
- Eric J. Topol
Nature Medicine (2024)
- Gregory P. Veldhuizen
- Jakob Nikolas Kather
Communications Medicine (2024)
- Jiyeong Kim
- Eleni Linos
npj Digital Medicine (2024)
Artificial intelligence and urology: ethical considerations for urologists and patients
- Giovanni E. Cacciamani
- Andrew Chen
- Andrew J. Hung
Nature Reviews Urology (2024)
Quick links
- Explore articles by subject
- Guide to authors
- Editorial policies
Sign up for the Nature Briefing: Translational Research newsletter — top stories in biotechnology, drug discovery and pharma.

- Oracle Mode
- Oracle Mode – Advanced
- Exploration Mode
- Simulation Mode
- Simulation Infrastructure
Join the movement towards fast, open, and transparent systematic reviews
ASReview LAB v1.5 is out!
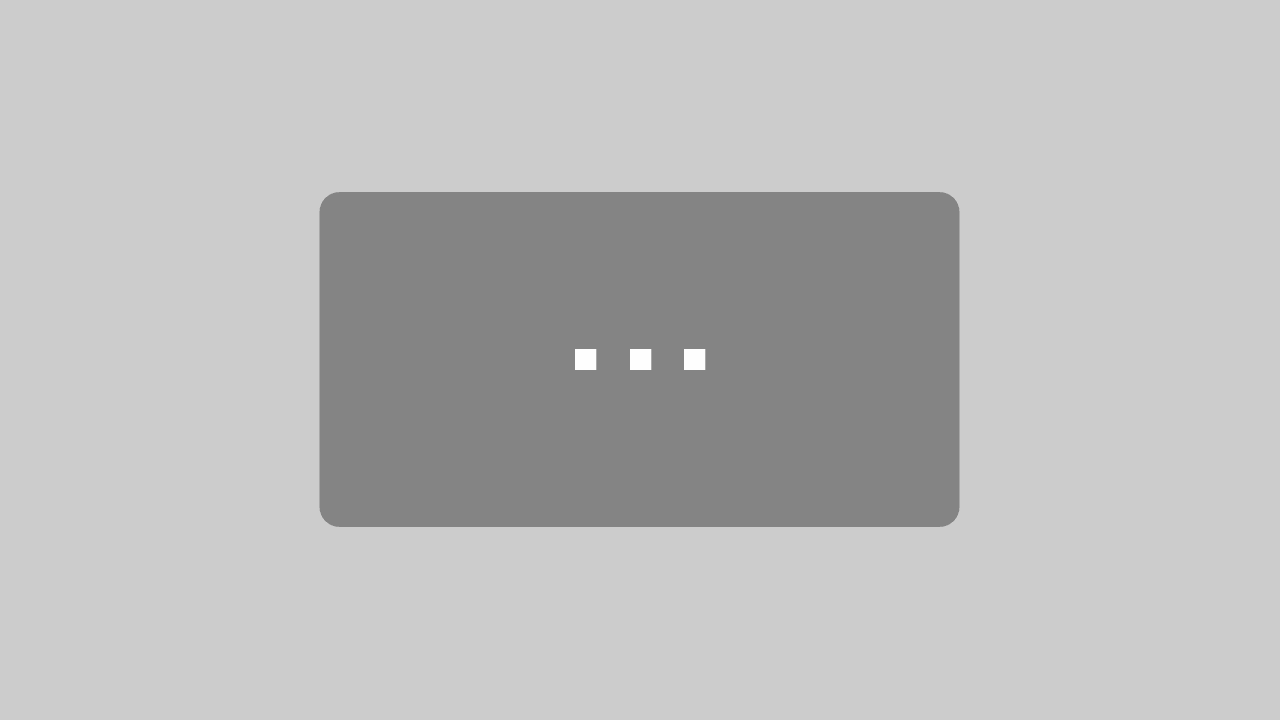
By loading the video, you agree to YouTube's privacy policy. Learn more
Always unblock YouTube
ASReview uses state-of-the-art active learning techniques to solve one of the most interesting challenges in systematically screening large amounts of text : there’s not enough time to read everything!
The project has grown into a vivid worldwide community of researchers, users, and developers. ASReview is coordinated at Utrecht University and is part of the official AI-labs at the university.
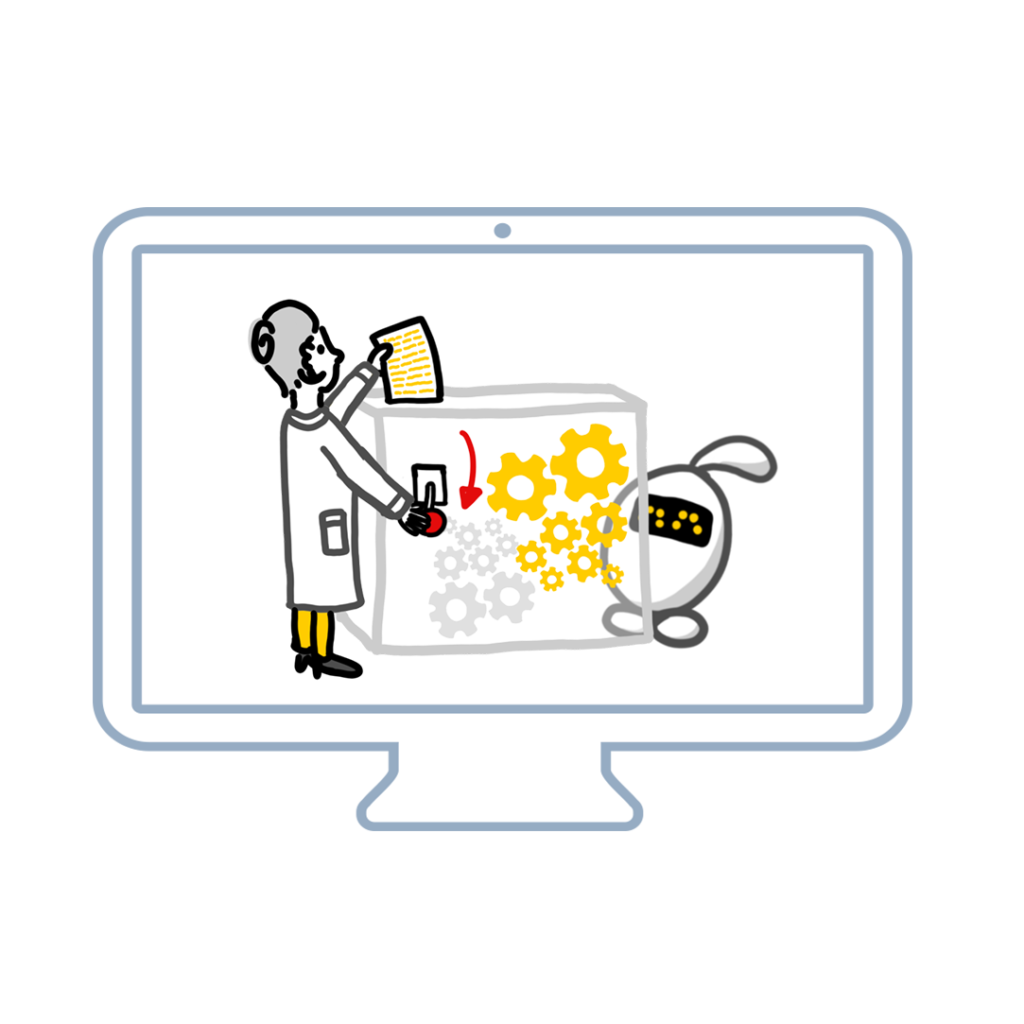
Free, Open and Transparent
The software is installed on your device locally. This ensures that nobody else has access to your data, except when you share it with others. Nice, isn’t it?
- Free and open source
- Local or server installation
- Full control over your data
- Follows the Reproducibility and Data Storage Checklist for AI-Aided Systematic Reviews
In 2 minutes up and running
With the smart project setup features, you can start a new project in minutes. Ready, set, start screening!
- Create as many projects as you want
- Choose your own or an existing dataset
- Select prior knowledge
- Select your favorite active learning algorithm
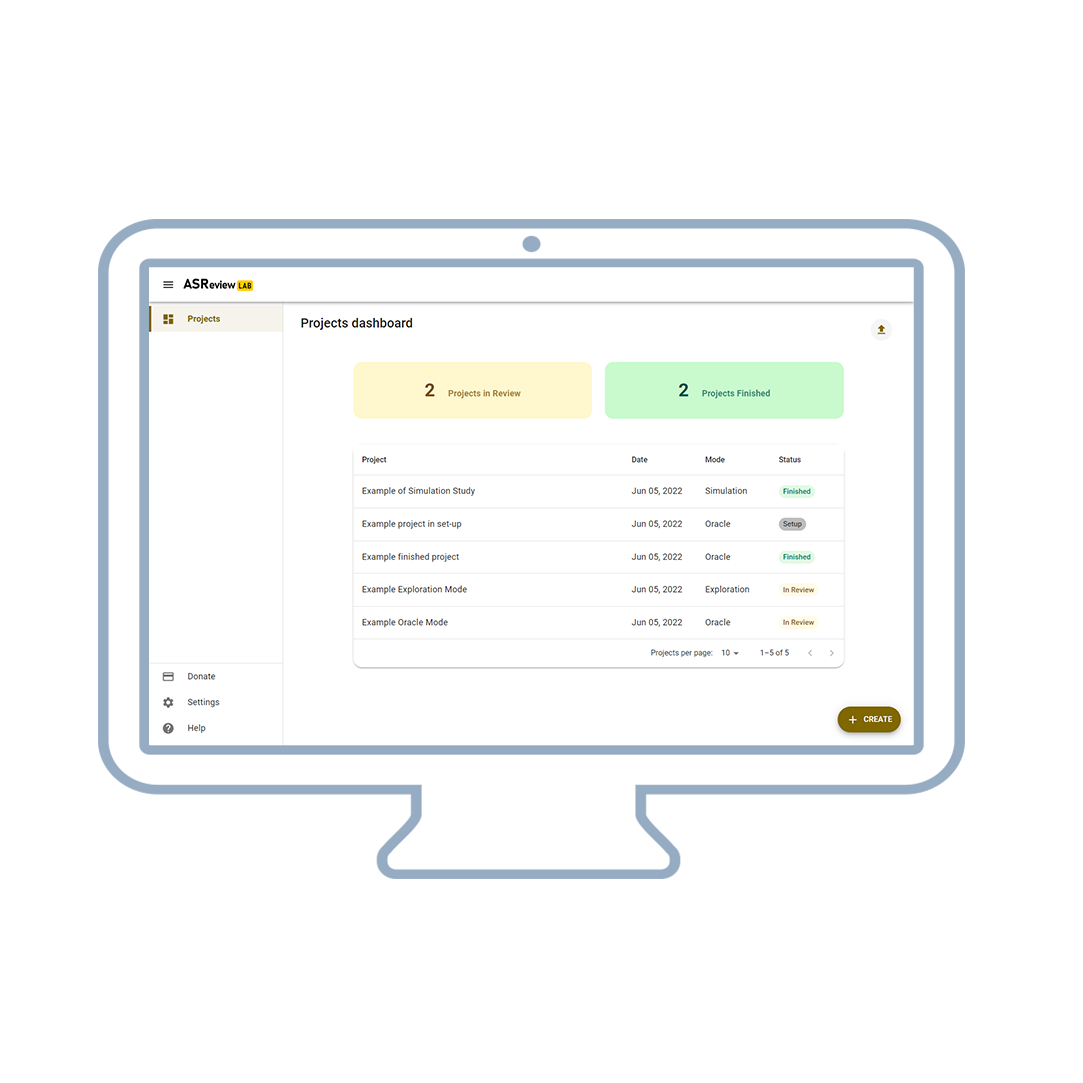
Three modi to choose from
ASReview LAB can be used for:
- Screening with the Oracle Mode , including advanced options
- Teaching using the Exploration Mode
- Validating algorithms using the Simulation Mode
We also offer an open-source research infrastructure to run large-scale simulation studies for validating newly developed AI algorithms.
Follow the development
Open-source means:
- All annotated source code is available
- You can see the developers at work in open Pull Requests
- Open Pull Request show in what direction the project is developing
- Anyone can contribute!
Give a GitHub repo a star if you like our work.
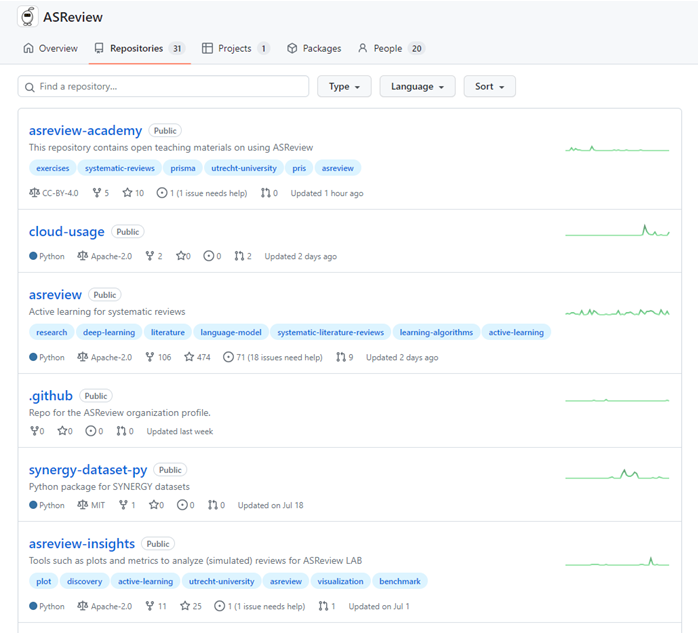
Join the community
A community-driven project means:
- The project is a joined endeavor
- Your contribution matters!
Join the movement towards transparent AI-aided reviewing
Beginner -> User -> Developer -> Maintainer
Organizations
Github stars
Join the ASReview Development Fund
Many users donate their time to continue the development of the different software tools that are part of the ASReview universe. Also, donations and research grants make innovations possible!
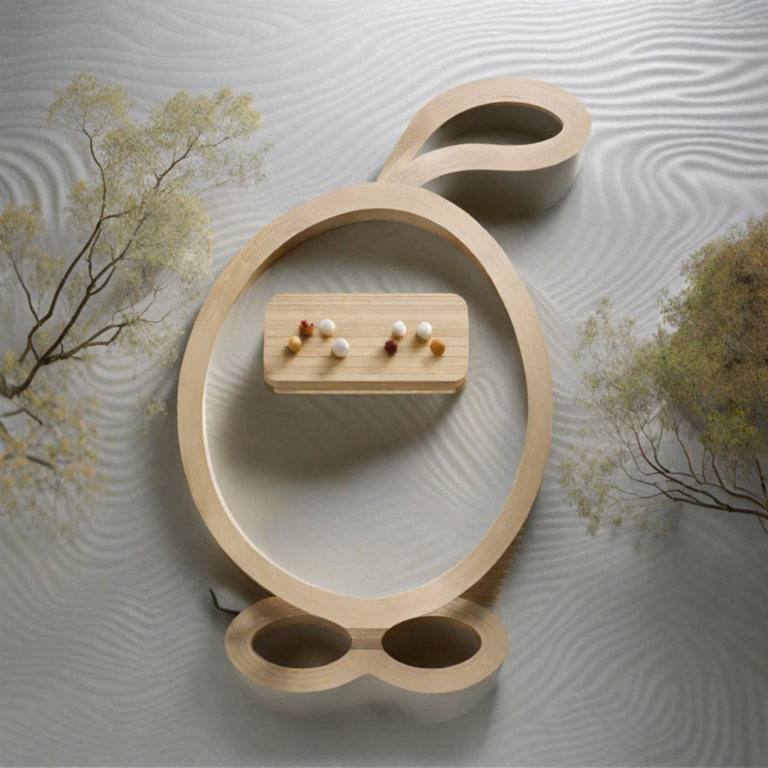
Navigating the Maze of Models in ASReview
Starting a systematic review can feel like navigating through a maze, with countless articles and endless…
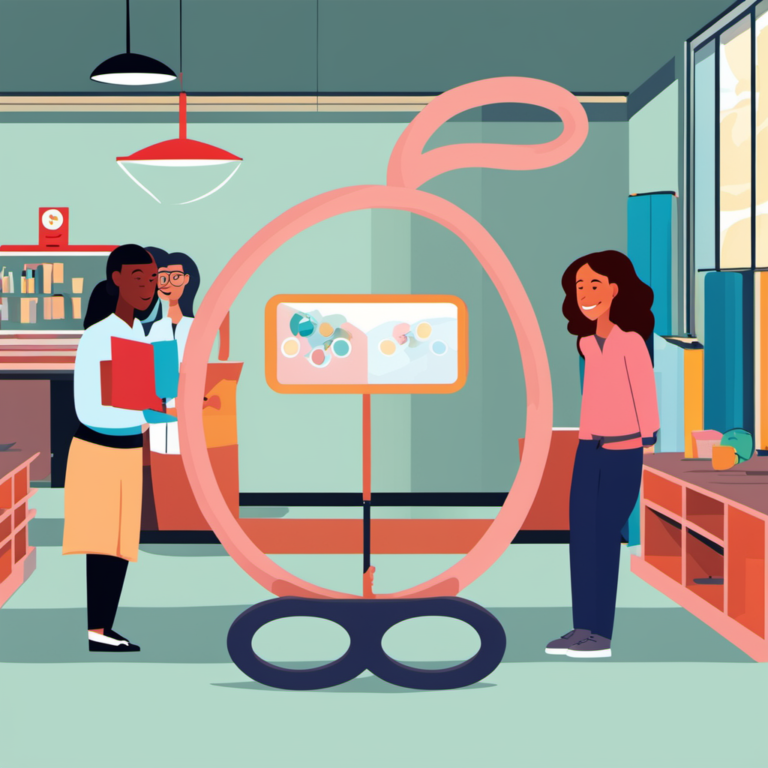
ASReview LAB Class 101
ASReview LAB Class 101 Welcome to ASReview LAB class 101, an introduction to the most important…
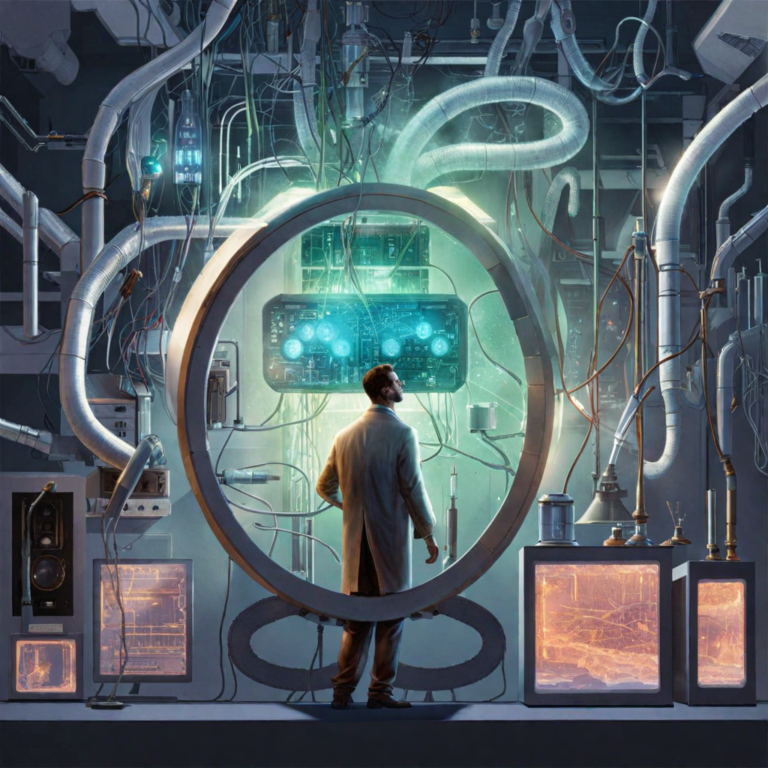
Introducing the Noisy Label Filter (NLF) procedure in systematic reviews
The ASReview team developed a procedure to overcome replication issues in creating a dataset for simulation…
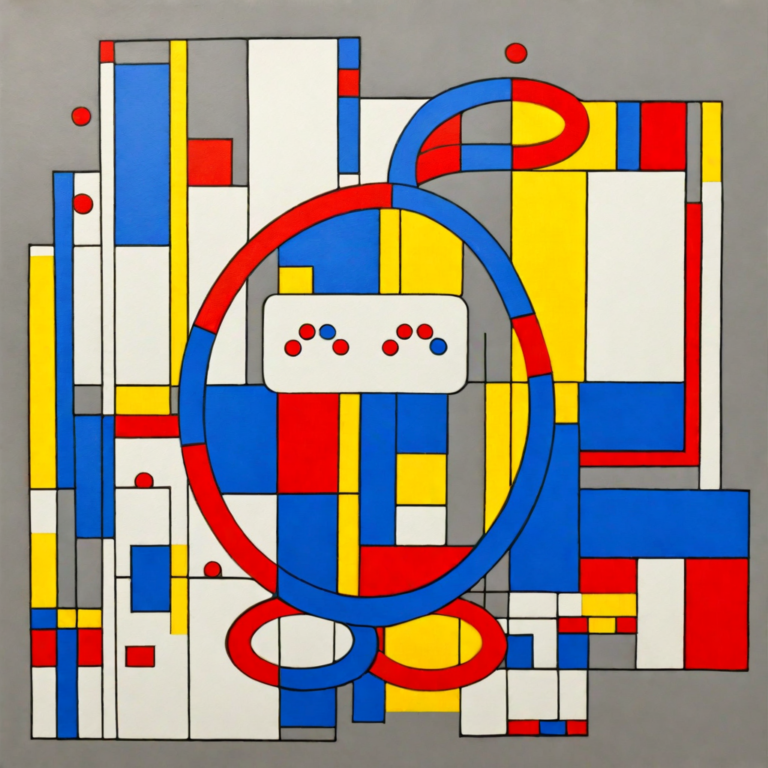
Seven ways to integrate ASReview in your systematic review workflow
Seven ways to integrate ASReview in your systematic review workflow Systematic reviewing using software implementing Active…
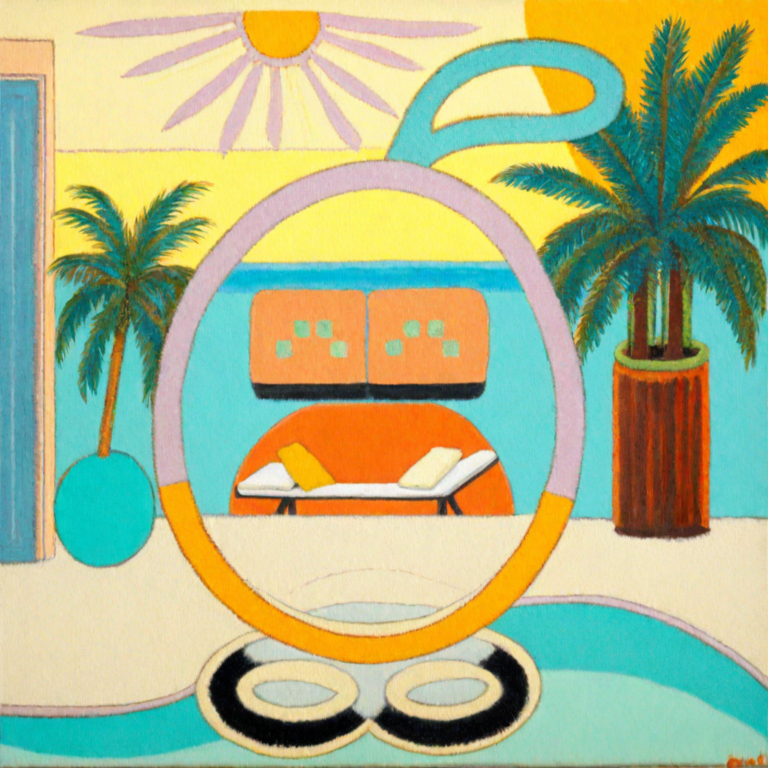
Active Learning Explained
Active Learning Explained The rapidly evolving field of artificial intelligence (AI) has allowed the development of…
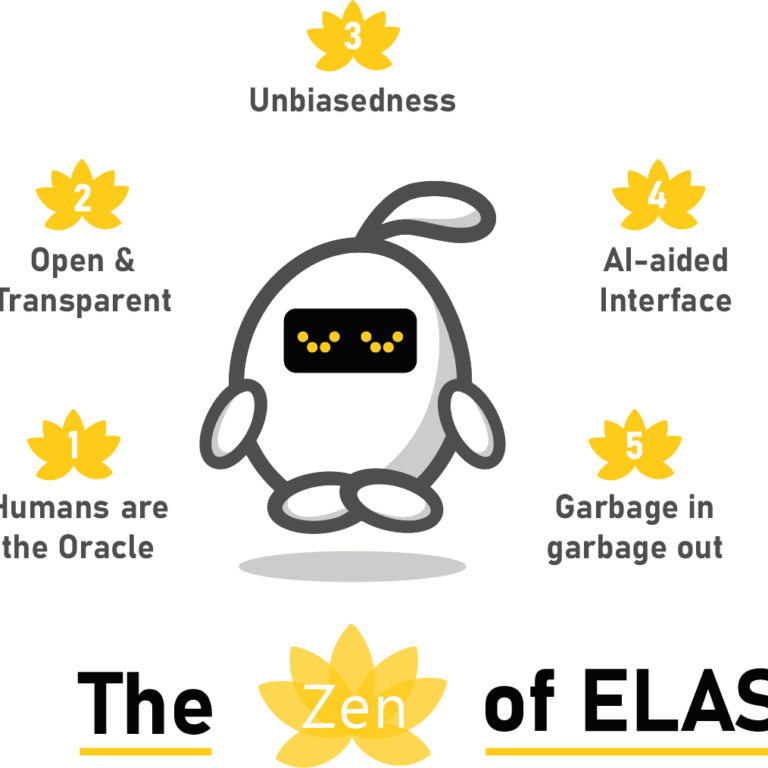
the Zen of Elas
The Zen of Elas Elas is the Mascotte of ASReview and your Electronic Learning Assistant who…
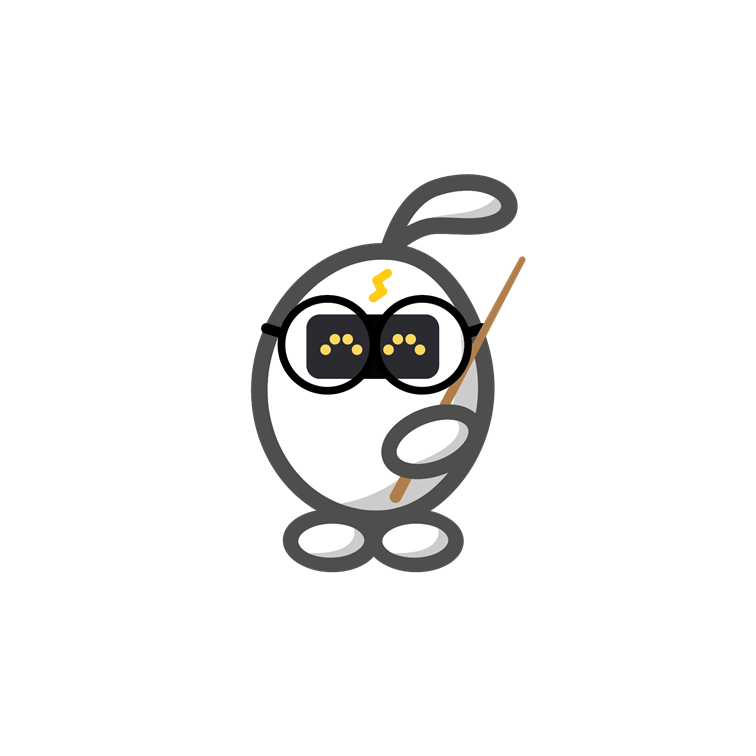
Five ways to get involved in ASReview
Five ways to get involved in ASReview ASReview LAB is open and free (Libre) software, maintained…
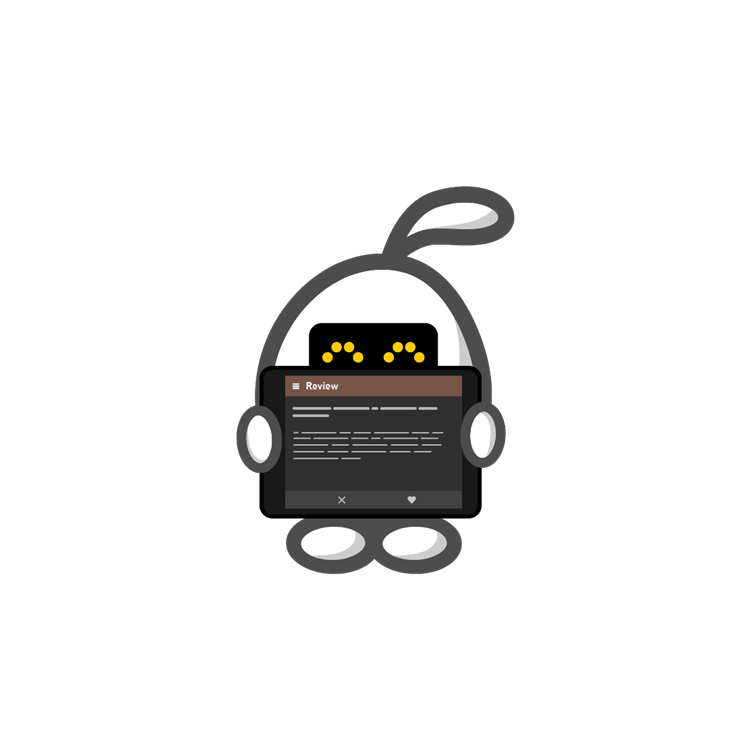
Connecting RIS import to export functionalities
What’s new in v0.19? Connecting RIS import to export functionalities Download ASReview LAB 0.19Update to ASReview…
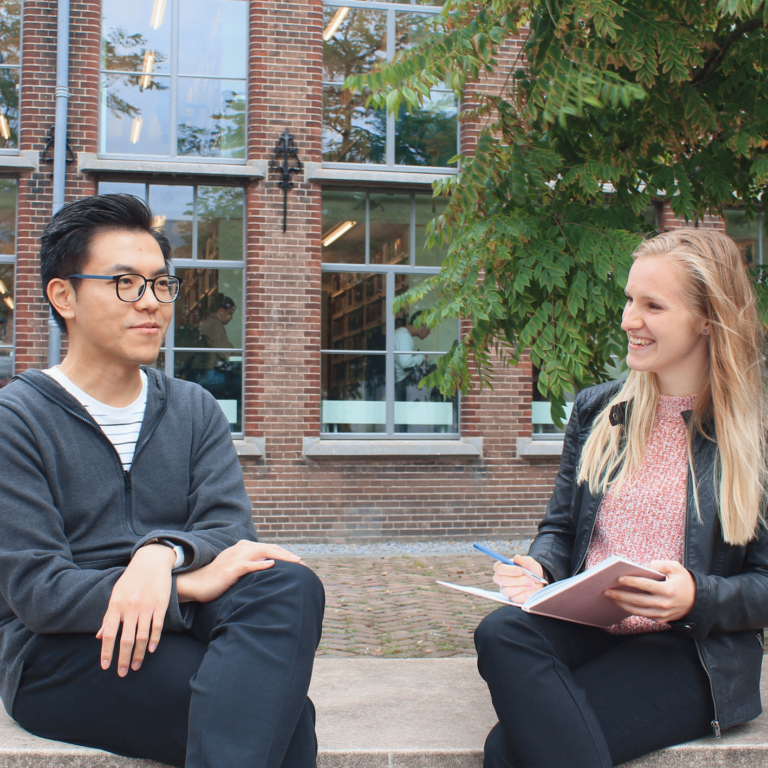
Meet the new ASReview Maintainer: Yongchao Ma
Meet Front-End Developer and ASReview Maintainer Yongchao Ma As a user of ASReview, you are probably…
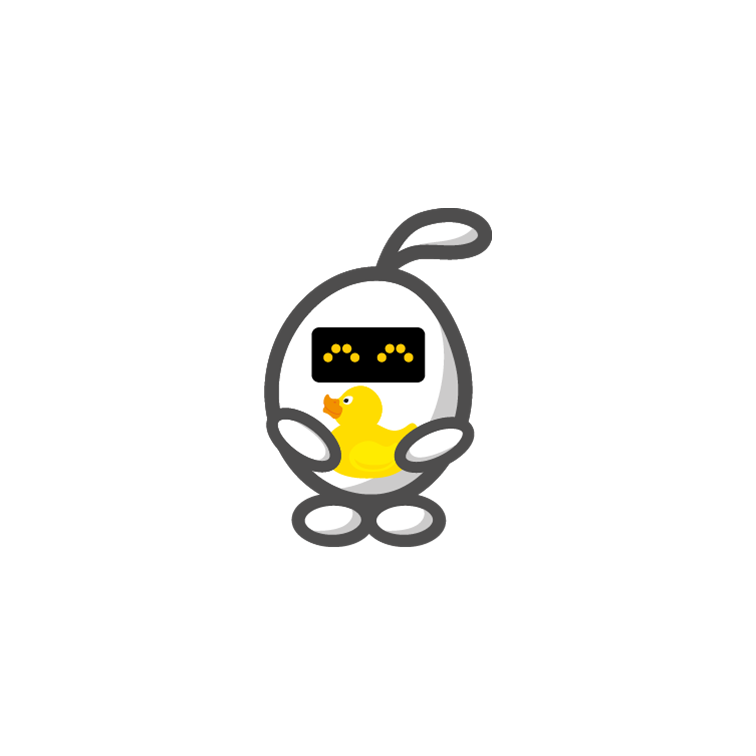
UPDATED: ASReview Hackathon for Follow the Money
This event has passed The winners of the hackathon were: Data pre-processing: Teamwork by: Raymon van…
What’s new in release 0.18?
More models more options, available now! Version 0.18 slowly opens ways to the soon to be…
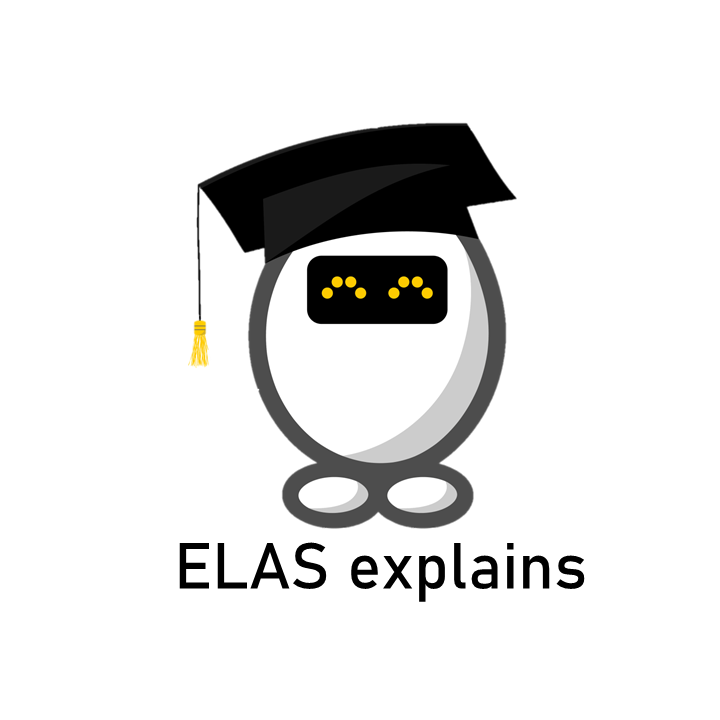
Simulation Mode Class 101
Simulation Mode Class 101 Have you ever done a systematic review manually, but wondered how much…
- Open access
- Published: 15 January 2022
Automation of literature screening using machine learning in medical evidence synthesis: a diagnostic test accuracy systematic review protocol
- Yuelun Zhang 1 na1 ,
- Siyu Liang 2 na1 ,
- Yunying Feng 3 na1 ,
- Qing Wang 4 ,
- Feng Sun 5 ,
- Shi Chen 2 ,
- Yiying Yang 3 ,
- Huijuan Zhu 2 &
- Hui Pan 2
Systematic Reviews volume 11 , Article number: 11 ( 2022 ) Cite this article
8868 Accesses
15 Citations
13 Altmetric
Metrics details
Systematic review is an indispensable tool for optimal evidence collection and evaluation in evidence-based medicine. However, the explosive increase of the original literatures makes it difficult to accomplish critical appraisal and regular update. Artificial intelligence (AI) algorithms have been applied to automate the literature screening procedure in medical systematic reviews. In these studies, different algorithms were used and results with great variance were reported. It is therefore imperative to systematically review and analyse the developed automatic methods for literature screening and their effectiveness reported in current studies.
An electronic search will be conducted using PubMed, Embase, ACM Digital Library, and IEEE Xplore Digital Library databases, as well as literatures found through supplementary search in Google scholar, on automatic methods for literature screening in systematic reviews. Two reviewers will independently conduct the primary screening of the articles and data extraction, in which nonconformities will be solved by discussion with a methodologist. Data will be extracted from eligible studies, including the basic characteristics of study, the information of training set and validation set, and the function and performance of AI algorithms, and summarised in a table. The risk of bias and applicability of the eligible studies will be assessed by the two reviewers independently based on Quality Assessment of Diagnostic Accuracy Studies (QUADAS-2). Quantitative analyses, if appropriate, will also be performed.
Automating systematic review process is of great help in reducing workload in evidence-based practice. Results from this systematic review will provide essential summary of the current development of AI algorithms for automatic literature screening in medical evidence synthesis and help to inspire further studies in this field.
Systematic review registration
PROSPERO CRD42020170815 (28 April 2020).
Peer Review reports
Systematic reviews synthesise the results of multiple original publications to provide clinicians with comprehensive knowledge and current optimal evidence in answering certain research questions. The major steps of a systematic review are defining a structured review question, developing inclusion criteria, searching in the databases, screening for relevant studies, collecting data from relevant studies, assessing the risk of bias critically, undertaking meta-analyses where appropriate, and assessing reporting biases [ 1 , 2 , 3 ]. A systematic review aims to provide a complete, exhaustive summary of current literature relevant to a research question with an objective and transparent approach. In the light of these characteristics, systematic reviews, in particular those combining high quality evidence, which used to be at the very top of the medical evidence pyramid [ 4 ] and now become regarded as an indispensable tool for evidence viewing [ 5 ], are widely used by reviewers in the practice of evidence-based medicine.
However, conducting systematic reviews for clinical decision making is time-consuming and labour-intensive, as the reviewers are supposed to perform a thorough search to identify any literatures that may be relevant, read through all abstracts of retrieved literatures, and identify the potential candidates for further full-text screening [ 6 ]. For original researches, the median time from the publication to their first inclusion in a systematic review ranged from 2.5 to 6.5 years [ 7 ]. It usually takes over a year to publish a systematic review from the time of literature search [ 8 ]. However, with advances in clinical research, this evidence and systematic review conclusions it generates may be out of date within several years. With the explosive increase of original research articles, reviewers have found difficulty identifying most relevant evidence in time, let alone updating systematic reviews periodically [ 9 ]. Therefore, researchers are exploring automatic methods to improve the efficacy of evidence synthesis while reducing the workload of systematic reviews.
Recent progresses in computer science show a promising future that more intelligent works can be accomplished with the aid of automatic technologies, such as pattern recognition and machine learning (ML). Being seen as a subset of artificial intelligence (AI), ML utilises algorithms to build mathematical models based on training data in order to make predictions or decisions without being explicitly programmed [ 10 ]. Various ML studies have been introduced in the medical field, such as diagnosis, prognosis, genetic analysis, and drug screening, to support clinical decision making [ 11 , 12 , 13 , 14 ]. When it comes to automatic methods for systematic reviews, models for automatic literature screening have been explored to reduce repetitive work and save time for reviewers [ 15 , 16 ].
To date, limited research has been focused on automatic methods used for biomedical literature screening in systematic review process. Automated literature classification systems [ 17 ] or hybrid relevance rating models [ 18 ] were tested in specific datasets, yet further extension of review datasets and performance improvement are required. To address this gap in knowledge, this article describes the protocol for a systematic review aiming at summarising existing automatic methods to screen relevant biomedical literature in the systematic review process, and evaluating the accuracy of the AI tools.
The primary objective of this review is to assess the diagnostic accuracy of AI algorithms (index test) compared with gold-standard human investigators (reference standard) for screening relevant literatures from original literatures identified by electronic search in systematic review. The secondary objective of this review is to describe the time and work saved by AI algorithms in literature screening. Additionally, we plan to conduct subgroup analyses to explore the potential factors that associate with the accuracy of AI algorithms.
Study registration
We prepared this protocol following the Preferred Reporting Items for Systematic Review and Meta-Analysis Protocols (PRISMA-P) [ 19 ]. This systematic review has been registered on PROSPERO (Registration number: CRD42020170815, 28 April 2020).
Review question
Our review question was refined using PRISMA-DTA framework, as detailed in Table 1 . In this systematic review, “literatures” refer to the subjects of the diagnostic test (the “participants” in Table 1 ), and “studies” refer to the studies included in our review.
Inclusion and exclusion criteria
We will include studies in medical research that reported a structured study question, described the source of the training or validation sets, developed or employed AI models for automatic literature screening, and used the screening results from human investigators as the reference standard.
We will exclude traditional clinical studies in human participants, editorials, commentaries, or other non-original reports. Pure methodological studies in AI algorithms without application in evidence synthesis will be excluded as well.
Information source and search strategy
An experienced methodologist will conduct searches in major public electronic medical and computer science databases, including PubMed, Embase, ACM Digital Library, and IEEE Xplore Digital Library, for publications ranged from January 2000 to present. We set this time range because to the best of our knowledge, AI algorithms prior to 2000 are unlikely to be applicable in evidence synthesis [ 20 ]. In addition to the literature search, we will also find more relevant studies through checking the reference lists of included studies identified by electronic search. Related abstracts and preprints will be searched in Google scholar. There are no language restrictions in searches. We will use free text words, MeSH/EMTREE terms, IEEE Terms, INSPEC Terms, and ACM Computing Classification System to develop strategies related to three major concepts: systematic review, literature screening, and AI. Multiple synonyms for each concept will be incorporated into the search. The Systematic Review Toolbox ( http://systematicreviewtools.com/ ) will also be utilised to detect potential automation methods in medical research evidence synthesis. Detailed search strategy used in PubMed is shown in Supplementary Material 1.
Study selection
Literatures with titles and abstracts from online electronic databases will be downloaded and imported into EndNote X9.3.2 software (Thomson Reuters, Toronto, Ontario, Canada) for further process after removing duplications.
All studies will be screened independently by 2 authors based on the titles and abstracts. Those which do not meet the inclusion criteria will be excluded with specific reasons. Disagreements will be solved by discussion with a methodologist if necessary. After the initial screening, the full texts of the potentially relevant studies will be independently reviewed by the two authors to make decisions on final inclusions. Conflicts will be resolved in the same way as they were initially screened. Excluded studies will be listed and noted according to PRISMA-DTA flowchart.
Data collection
A data collection form will be used for information extraction. Data from the eligible studies will be independently extracted and verified by two investigators. Disagreements will be resolved through discussion and consultation with the original publication. We will also try to contact the authors to collect the missing data. If one study did not report detailed accuracy data or did not provide enough data that are essential to calculate the accuracy data, this study will be omitted from the quantitative data synthesis.
The following data will be extracted from the original studies: characteristics of study, information of training set and validation set, and the function and performance of AI algorithms. The definitions of variables in data extraction are shown in Table 2 .
Risk of bias assessment, applicability, and levels of evidence
Two authors will independently assess risk of bias and applicability with a checklist based on Quality Assessment of Diagnostic Accuracy Studies (QUADAS-2) [ 21 ]. The QUADAS-2 contains 4 domains, respectively regarding patient selection, index test, reference standard, and flow and timing risk of bias. The risk of bias is classified as “low”, “high”, or “unclear”. Studies with high risk of bias will be excluded in the sensitivity analysis.
In this systematic review, the “participants” are literatures rather than human subjects. The index test is AI model used for automatic literature screening. Therefore, we will slightly revise the QUADAS-2 to fit our research context (Table 3 ). We deleted one signal question in the QUADAS-2 “was there an appropriate interval between index test and reference standard”. The purpose of this signal question in the original version of the QUADAS-2 is to judge the bias caused by the change of disease status between the index test and the reference test. The “disease status”, or the final inclusion status of one literature in our research context, will not change; thus, there are no such concerns.
The levels of the evidence body will be evaluated by the Grading of Recommendations, Assessment, Development and Evaluations (GRADE) framework [ 22 ].
Diagnostic accuracy measures
We will extract the data of per study in a two-by-two contingency table from the formal publication text, appendices, or by contacting the main authors to collect sensitivity, specificity, precision, negative predictive value (NPV), positive predictive value (PPV), negative likelihood ratio (NLR), positive likelihood ratio (PLR), diagnostic odds ratios (DOR), F-measure, and accuracy with 95% CI. If the outcomes cannot be formulated in a two-by-two contingency table, we will extract the reported performance data. If possible, we will also assess the area under the curve (AUC), as the two-by-two contingency table may not be available in some scenarios.
Qualitative and quantitative synthesis of results
We will qualitatively describe the application of AI in literature screening and evaluate and compare the accuracy of the AI tools. If there were adequate details and homogeneous data for the quantitative meta-analysis, we will combine the accuracy of AI algorithms in literature screening using the random-effects Rutter-Gatsonis hierarchical summarised receiver operating characteristic curve (HSROC) model which was recommended by the Cochrane Collaboration for combining the evidence for diagnostic accuracy [ 23 ]. The effect of threshold will be incorporated in the model in which heterogeneous thresholds among different studies will be allowed. The combined point estimates of accuracy will be retrieved from the summarised receiver operating characteristic curve (ROC).
Subgroup analyses and meta-regression will be used to explore the between-study heterogeneity. We will explore the following predefined sources of heterogeneity: (1) AI algorithm type, (2) study area of validation set (targeted specific diseases, interventions, or a general area), (3) searched electronic databases (PubMed, EMBASE, or others), and (4) proportion of eligible to original studies (the number of eligible literature identified in the screening step divided by the number of original literature identified during the electronic search). Furthermore, we will analyse the possible sources of heterogeneity from both dataset and methodological perspectives in HSROC as covariates following the recommendations from the Cochrane Handbook for Diagnostic Tests Review [ 23 ]. We regarded the factor as a source of heterogeneity if the coefficient of the covariate in the HSROC model was statistically significant. We will not evaluate the reporting bias (e.g. publication bias) since the hypothesis underlying the commonly used methods, such as funnel plot or Egger’s test, may not be satisfied in our research context. Data were analysed using R software, version 4.0.2 (R Foundation for Statistical Computing, Vienna, Austria) with two-tailed probability of type I error of 0.05 ( α =0.05).
Systematic review has developed rapidly within the last decades and plays a key role in enabling the spread of evidence-based practice. Systematic review, though costing less than primary research in money expenditure, is still time-consuming and labour-intensive. Conducting systematic review begins with electronic database searching for a specific research question, then at least two reviewers read each abstract of retrieved records to identify potential candidate literatures for full-text screening. Only 2.9% retrieved records are relevant and included in the final synthesis on average [ 24 ]; typically, reviewers have to find the proverbial needle in the haystack of irrelevant titles and abstracts. Computational scientists have developed various algorithms for automatic literature screening. Developing an automatic literature screening instrument will be source-saving and improve the quality of systematic review by liberating reviewers from repetitive work. In this systematic review, we aim to describe and evaluate the development process and algorithms used in various AI literature screening systems, in order to build a pipeline for the update of existing tools and creation of new models.
The accuracy of automatic literature screening instruments varied widely in different algorithms and review topics [ 17 ]. The automatic literature screening systems can reach a sensitivity as high as 95%, despite at the expense of specificity, since reviewers try to include every publication relative to the topic of review. As the automatic systems may have a low specificity, it is also important to evaluate how much reviewing work the reviewers can save in the step of screening. We will not only assess the diagnostic accuracy of AI screening algorithms compared with human investigators, but also collect the information of work saved by AI algorithms in literature screening. Additionally, we plan to conduct subgroup analyses to identify potential factors that associate with the accuracy and efficacy of AI algorithms.
As far as we know, this will be the first systematic review to evaluate AI algorithms for automatic literature screening in evidence synthesis. Few systematic reviews have focused on the application of AI algorithms in medical practice. The literature search strategies in previous published systematic reviews rarely use specific algorithms as search terms. Most of them generally use words such as “artificial intelligence” and “machine learning” in strategies, which may lose the studies that only reported one specific algorithm. In order to include AI-related studies as much as possible, our search strategy contained all of the AI algorithms commonly used in the past 50 years, and it was reviewed by an expert in ML. The process of literature screening can be assessed under the framework of the diagnostic test. Findings from this proposed systematic review will provide a comprehensive and essential summary of the application of AI algorithms for automatic literature screening in evidence synthesis. The proposed systematic review may also help to improve and promote the automatic methods in evidence synthesis in the future by locating and identifying the potential weakness in the current AI models and methods.
Availability of data and materials
The datasets used and analysed during the current study are available from the corresponding author on reasonable request.
Abbreviations
- Artificial intelligence
Area under the curve
Diagnostic odds ratio
Grading of Recommendations, Assessment, Development and Evaluations
Hierarchical summarised receiver operating characteristic curve
Negative likelihood ratio
Negative predictive value
Positive likelihood ratio
Positive predictive value
Preferred Reporting Items for Systematic Review and Meta-Analysis Protocols
Quality Assessment of Diagnostic Accuracy Studies
Receiver operating characteristic curve
Support vector machine
Higgins J, Thomas J, Chandler J, et al. Cochrane handbook for systematic reviews of interventions version 6.0 (updated July 2019)Cochrane, 2019. Reference Source; 2020.
Google Scholar
Mulrow CD, Cook D. Systematic reviews: synthesis of best evidence for health care decisions: ACP Press; 1998.
Armstrong R, Hall BJ, Doyle J, Waters E. ‘Scoping the scope’ of a cochrane review. J Public Health. 2011;33(1):147–50.
Article Google Scholar
Paul M, Leibovici L. Systematic review or meta-analysis? Their place in the evidence hierarchy. Clin Microbiol Infect. 2014;20(2):97–100. https://doi.org/10.1111/1469-069112489 2014(1469-0691 (Electronic)):97-100.
Article CAS PubMed Google Scholar
Murad MH, Asi N, Alsawas M, Alahdab F. New evidence pyramid. Evid Based Med. 2016;21(4):125.
Bigby M. Evidence-based medicine in a nutshell: a guide to finding and using the best evidence in caring for patients. Arch Dermatol. 1998;134(12):1609–18.
CAS PubMed Google Scholar
Bragge P, Clavisi O, Turner T, Tavender E, Collie A, Gruen RL. The global evidence mapping initiative: scoping research in broad topic areas. BMC Med Res Methodol. 2011;11(1):92.
Sampson M, Shojania KG, Garritty C, Horsley T, Ocampo M, Moher D. Systematic reviews can be produced and published faster. J Clin Epidemiol. 2008;61(6):531–6.
Shojania K, Sampson M, Ansari M, Ji J, Doucette S, Moher D. How quickly do systematic reviews go out of date? A survival analysis. Ann Intern Med. 2007;147(4):224–33 2007(1539-3704 (Electronic)):224-233.
Bishop CM. Pattern recognition and machine learning: Springer; 2006.
Wang L-Y, Chakraborty A, Comaniciu D. Molecular diagnosis and biomarker identification on SELDI proteomics data by ADTBoost method. Paper presented at: 2005 IEEE Engineering in Medicine and Biology 27th Annual Conference. 2006.
Cetin MS, Houck JM, Vergara VM, Miller RL, Calhoun V. Multimodal based classification of schizophrenia patients. Paper presented at: 2015 37th Annual International Conference of the IEEE Engineering in Medicine and Biology Society (EMBC). 2015.
Sun Y, Loparo K. Information extraction from free text in clinical trials with knowledge-based distant supervision. Paper presented at: 2019 IEEE 43rd Annual Computer Software and Applications Conference (COMPSAC). 2019.
Li M, Lu Y, Niu Z, Wu F-X. United complex centrality for identification of essential proteins from PPI networks. IEEE/ACM Transact Comput Biol Bioinform. 2015;14(2):370–80.
Whittington C, Feinman T, Lewis SZ, Lieberman G, Del Aguila M. Clinical practice guidelines: machine learning and natural language processing for automating the rapid identification and annotation of new evidence. J Clin Oncol. 2019;37.
Turner MD, Chakrabarti C, Jones TB, et al. Automated annotation of functional imaging experiments via multi-label classification. Front Neurosci. 2013;7:240.
Cohen AM, Hersh WR, Peterson K, Yen P-Y. Reducing workload in systematic review preparation using automated citation classification. J Am Med Inform Assoc. 2006;13(2):206–19.
Article CAS Google Scholar
Rúbio TR, Gulo CA. Enhancing academic literature review through relevance recommendation: using bibliometric and text-based features for classification. Paper presented at: 2016 11th Iberian Conference on Information Systems and Technologies (CISTI). 2016.
Shamseer L, Moher D, Clarke M, et al. Preferred reporting items for systematic review and meta-analysis protocols (PRISMA-P) 2015: elaboration and explanation. BMJ. 2015;350:g7647.
Jonnalagadda SR, Goyal P, Huffman MD. Automating data extraction in systematic reviews: a systematic review. Syst Rev. 2015;4:78.
Whiting PF, Rutjes AW, Westwood ME, et al. QUADAS-2: a revised tool for the quality assessment of diagnostic accuracy studies. Ann Intern Med. 2011;155(8):529–36.
Guyatt GH, Oxman AD, Vist GE, et al. GRADE: an emerging consensus on rating quality of evidence and strength of recommendations. BMJ. 2008;336(7650):924–6.
Macaskill P, Gatsonis C, Deeks J, Harbord R, Takwoingi Y. Cochrane handbook for systematic reviews of diagnostic test accuracy. Version 09 0. London: The Cochrane Collaboration; 2010.
Sampson M, Tetzlaff J, Urquhart C. Precision of healthcare systematic review searches in a cross-sectional sample. Res Synth Methods. 2011;2(2):119–25.
Download references
Acknowledgements
We thank Professor Siyan Zhan (Department of Epidemiology and Biostatistics, School of Public Health, Peking University Health Science Center, [email protected] ) for her critical comments in designing this study. We also thank Dr. Bin Zhang (Institute of Medical Information/Medical Library, Chinese Academy of Medical Sciences & Peking Union Medical College, [email protected] ) for her critical suggestions in developing search strategies.
This study will be supported by the Undergraduate Innovation and Entrepreneurship Training Program (Number 202010023001). The sponsors have no role in study design, data collection, data analysis, interpretations of findings, and decisions for dissemination.
Author information
Yuelun Zhang, Siyu Liang, and Yunying Feng contributed equally to this work and should be regarded as co-first authors.
Authors and Affiliations
Medical Research Center, Peking Union Medical College Hospital, Chinese Academy of Medical Sciences and Peking Union Medical College, Beijing, China
Yuelun Zhang
Department of Endocrinology, Peking Union Medical College Hospital, Chinese Academy of Medical Sciences and Peking Union Medical College, 1 Shuaifuyuan, Dongcheng District, Beijing, China
Siyu Liang, Shi Chen, Huijuan Zhu & Hui Pan
Eight-year Program of Clinical Medicine, Peking Union Medical College Hospital, Chinese Academy of Medical Sciences and Peking Union Medical College, Beijing, China
Yunying Feng, Yiying Yang & Xin He
Research Institute of Information and Technology, Tsinghua University, Beijing, China
Department of Epidemiology and Biostatistics, School of Public Health, Peking University Health Science Center, Beijing, China
You can also search for this author in PubMed Google Scholar
Contributions
H Pan conceived this research. This protocol was designed by YL Zhang, SY Liang, and YY Feng. YY Yang, X He, Q Wang, F Sun, S Chen, and HJ Zhu provided critical suggestions and comments on the manuscript. YL Zhang, SY Liang, and YY Feng wrote the manuscript. All authors read and approved the final manuscript. H Pan is the guarantor for this manuscript.
Corresponding author
Correspondence to Hui Pan .
Ethics declarations
Ethics approval and consent to participate.
This research is exempt from ethics approval because the work is carried out on published documents.
Consent for publication
Not applicable.
Competing interests
The authors declare that they have no competing interests.
Additional information
Publisher’s note.
Springer Nature remains neutral with regard to jurisdictional claims in published maps and institutional affiliations.
Supplementary Information
Additional file 1: supplementary table 1.
. Search strategy for PubMed.
Rights and permissions
Open Access This article is licensed under a Creative Commons Attribution 4.0 International License, which permits use, sharing, adaptation, distribution and reproduction in any medium or format, as long as you give appropriate credit to the original author(s) and the source, provide a link to the Creative Commons licence, and indicate if changes were made. The images or other third party material in this article are included in the article's Creative Commons licence, unless indicated otherwise in a credit line to the material. If material is not included in the article's Creative Commons licence and your intended use is not permitted by statutory regulation or exceeds the permitted use, you will need to obtain permission directly from the copyright holder. To view a copy of this licence, visit http://creativecommons.org/licenses/by/4.0/ . The Creative Commons Public Domain Dedication waiver ( http://creativecommons.org/publicdomain/zero/1.0/ ) applies to the data made available in this article, unless otherwise stated in a credit line to the data.
Reprints and permissions
About this article
Cite this article.
Zhang, Y., Liang, S., Feng, Y. et al. Automation of literature screening using machine learning in medical evidence synthesis: a diagnostic test accuracy systematic review protocol. Syst Rev 11 , 11 (2022). https://doi.org/10.1186/s13643-021-01881-5
Download citation
Received : 20 August 2020
Accepted : 27 December 2021
Published : 15 January 2022
DOI : https://doi.org/10.1186/s13643-021-01881-5
Share this article
Anyone you share the following link with will be able to read this content:
Sorry, a shareable link is not currently available for this article.
Provided by the Springer Nature SharedIt content-sharing initiative
- Evidence-based practice
- Natural language process
- Systematic review
- Diagnostic test accuracy
Systematic Reviews
ISSN: 2046-4053
- Submission enquiries: Access here and click Contact Us
- General enquiries: [email protected]

An official website of the United States government
The .gov means it’s official. Federal government websites often end in .gov or .mil. Before sharing sensitive information, make sure you’re on a federal government site.
The site is secure. The https:// ensures that you are connecting to the official website and that any information you provide is encrypted and transmitted securely.
- Publications
- Account settings
Preview improvements coming to the PMC website in October 2024. Learn More or Try it out now .
- Advanced Search
- Journal List
- JMIR Med Inform
- v.8(7); 2020 Jul
Role of Artificial Intelligence in Patient Safety Outcomes: Systematic Literature Review
Avishek choudhury.
1 School of Systems and Enterprises, Stevens Institute of Technology, Hoboken, NJ, United States
Associated Data
Preferred Reporting Items for Systematic Reviews and Meta-Analysis (PRISMA) checklist.
Artificial intelligence (AI) provides opportunities to identify the health risks of patients and thus influence patient safety outcomes.
The purpose of this systematic literature review was to identify and analyze quantitative studies utilizing or integrating AI to address and report clinical-level patient safety outcomes.
We restricted our search to the PubMed, PubMed Central, and Web of Science databases to retrieve research articles published in English between January 2009 and August 2019. We focused on quantitative studies that reported positive, negative, or intermediate changes in patient safety outcomes using AI apps, specifically those based on machine-learning algorithms and natural language processing. Quantitative studies reporting only AI performance but not its influence on patient safety outcomes were excluded from further review.
We identified 53 eligible studies, which were summarized concerning their patient safety subcategories, the most frequently used AI, and reported performance metrics. Recognized safety subcategories were clinical alarms (n=9; mainly based on decision tree models), clinical reports (n=21; based on support vector machine models), and drug safety (n=23; mainly based on decision tree models). Analysis of these 53 studies also identified two essential findings: (1) the lack of a standardized benchmark and (2) heterogeneity in AI reporting.
Conclusions
This systematic review indicates that AI-enabled decision support systems, when implemented correctly, can aid in enhancing patient safety by improving error detection, patient stratification, and drug management. Future work is still needed for robust validation of these systems in prospective and real-world clinical environments to understand how well AI can predict safety outcomes in health care settings.
Introduction
Patient safety is defined as the absence of preventable harm to a patient and minimization of the risk of harm associated with the health care process [ 1 , 2 ]. Every part of the care-giving process involves a certain degree of inherent risk. Since resolution WHA55.18 on “Quality of Care: Patient Safety” at the 55th World Health Assembly was proposed in 2002, there has been increasing attention paid to patient safety concerns and adverse events in health care settings [ 3 ]. Despite the safety initiatives and investments made by federal and local governments, private agencies, and concerned institutions, studies continue to report unfavorable patient safety outcomes [ 4 , 5 ].
The integration of artificial intelligence (AI) into the health care system is not only changing dynamics such as the role of health care providers but is also creating new potential to improve patient safety outcomes [ 6 ] and the quality of care [ 7 ]. The term AI can be broadly defined as a computer program that is capable of making intelligent decisions [ 8 ]. The operational definition of AI we adopt in this review is the ability of a computer or health care device to analyze extensive health care data, reveal hidden knowledge, identify risks, and enhance communication [ 9 ]. In this regard, AI encompasses machine learning and natural language processing. Machine learning enables computers to utilize labeled (supervised learning) or unlabeled (unsupervised learning) data to identify latent information or make predictions about the data without explicit programming [ 9 ]. Among different types of AI, machine learning and natural language processing specifically have societal impacts in the health care domain [ 10 ] and are also frequently used in the health care field [ 9 - 12 ].
The third category within machine learning is known as reinforcement learning, in which an algorithm attempts to accomplish a task while learning from its successes and failures [ 9 ]. Machine learning also encompasses artificial neural networks or deep learning [ 13 ]. Natural language processing focuses on building a computer’s ability to understand human language and consecutively transform text to machine-readable structured data, which can then be analyzed by machine-learning techniques [ 14 ]. In the literature, the boundary defining natural language processing and machine learning is not clearly defined. However, as illustrated in Figure 1 , studies in the field of health care have been using natural language processing in conjunction with machine-learning algorithms [ 15 ].

Schematic illustration of how natural language processing converts unstructured text to machine-readable structured data, which can then be analyzed by machine-learning algorithms.
AI has potential to assist clinicians in making better diagnoses [ 16 - 18 ], and has contributed to the fields of drug development [ 19 - 21 ], personalized medicine, and patient care monitoring [ 14 , 22 - 24 ]. AI has also been embedded in electronic health record (EHR) systems to identify, assess, and mitigate threats to patient safety [ 25 ]. However, with the deployment of AI in health care, several risks and challenges can emerge at an individual level (eg, awareness, education, trust), macrolevel (eg, regulation and policies, risk of injuries due to AI errors), and technical level (eg, usability, performance, data privacy and security).
The measure of AI accuracy does not necessarily indicate clinical efficiency [ 26 ]. Another common measure, the area under the receiver operating characteristic curve (AUROC), is also not necessarily the best metric for clinical applicability [ 27 ]. Such AI metrics might not be easily understood by clinicians or might not be clinically meaningful [ 28 ]. Moreover, AI models have been evaluated using a variety of parameters and report different measure(s) such as the F1 score, accuracy, and false-positive rate, which are indicative of different aspects of AI’s analytical performance. Understanding the functioning of complex AI requires technical knowledge that is not common among clinicians. Moreover, clinicians do not necessarily have the training to identify underlying glitches of the AI, such as data bias, overfitting, or other software errors that might result in misleading outcomes. Such flaws in AI can result in incorrect medication dosage and poor treatment [ 29 - 33 ].
Furthermore, a system error in a widely used AI might lead to mass patient injuries compared to a limited number of patient injuries due to a provider’s error [ 34 ]. Additionally, there have been instances where traditional analytical methods outperformed machine-learning techniques [ 9 ]. Owing to the wide range of effectiveness of AI, it is crucial to understand both the promising and deterring impacts of AI on patient safety outcomes [ 35 ].
AI in the health care system can assist at both the “clinical” and “diagnostic” levels [ 36 ]. AI provides a powerful tool that can be implemented within the health care domain to reveal subtle patterns in data, and these patterns can then be interpreted by clinicians to identify new clinical and health-related issues [ 9 ]. Recent studies and reviews have primarily focused on the performance of AI at the diagnostic level, such as for disease identification [ 37 - 42 ], and the application of AI robotics in surgery and disease management [ 43 - 46 ]. Other studies have also implemented AI technologies to assist at the clinical level, including assessing fall risks [ 47 ] and medication errors [ 48 , 49 ]. However, many of these studies are centered around AI development and performance and there is a notable lack of studies reviewing the role and impact of AI used at the clinical level on patient safety outcomes.
Many studies have reported high accuracy of AI in health care. However, its actual influence (negative or positive) can only be realized when it is integrated into clinical settings or interpreted and used by care providers [ 50 ]. Therefore, in our view, patient safety and AI performance might not necessarily complement each other. AI in health care depends on data sources such as EHR systems, sensor data, and patient-reported data. EHR systems may contain more severe cases for specific patient populations. Certain patient populations may have more ailments or may be seen at multiple institutions. Certain subgroups of patients with rare diseases may not exist in sufficient numbers for a predictive analytic algorithm. Thus, clinical data retrieved from EHRs might be prone to biases [ 9 , 50 ]. Owing to these potential biases, AI accuracy might be misleading [ 51 ] when trained on a small subgroup or small sample size of patients with rare ailments.
Furthermore, patients with limited access to health care may receive fewer diagnostic tests and medications and may have insufficient health information in the EHR to trigger an early intervention [ 52 ]. In addition, institutions record patient information differently; as a result, if AI models trained at one institution are implemented to analyze data at another institution, this may result in errors [ 52 ]. For instance, machine-learning algorithms developed at a university hospital to predict patient-reported outcome measures, which tend to be documented by patients who have high education as well as high income, may not be applicable when implemented at a community hospital that primarily serves underrepresented patient groups with low income.
A review [ 53 ] conducted in 2017 reported that only about 54% of studies that developed prediction models based on EHRs accounted for missing data. Recent studies and reviews have been primarily focusing on the performance and influence of AI systems at a diagnostic level, such as for disease identification [ 37 - 42 ], and the influence of AI robotics in surgery and disease management [ 43 - 46 ]; however, there is a lack of studies reviewing and reporting the impact of AI used at the clinical level on patient safety outcomes, as well as characteristics of the AI algorithms used. Thus, it is essential to study how AI has been shown to influence patient safety outcomes at the clinical level, along with reported AI performance in the literature. In this systematic review, we address this gap by exploring the studies that utilized AI algorithms as defined in this review to address and report changes in patient safety outcomes at the clinical level ( Figure 2 ).

Artificial intelligence (AI) route to patient safety via “Clinical” and “Diagnostic” level interventions. DSS: decision support system.
Protocol Registration
This systematic review is reported according to the Preferred Reporting Items for Systematic Reviews and Meta-Analysis (PRISMA) guidelines [ 54 ]. We followed the PRISMA Checklist (see Multimedia Appendix 1 ). Our protocol [ 55 ] was registered with the Open Science Framework on September 15, 2019.
Information Sources
We searched for peer-reviewed publications in the PubMed, PubMed Central, and Web of Science databases from January 2009 to August 2019 to identify articles within the scope and eligibility criteria of this systematic literature review.
Search Strategy
We followed a systematic approach of creating all search terms to capture all related and eligible papers in the searched databases. Keywords used in the search were initially determined by a preliminary review of the literature and then modified based on feedback from content experts as well as our institution’s librarian.
We then refined the search strategy in collaboration with the librarian to ensure that all clinical-level patient safety-related papers (as shown in Figure 2 ) were covered in our review and determined the Medical Subject Headings (MeSH) terms. We grouped the query keywords, which were derived from MeSH terms and combined through Boolean (AND/OR) operators to identify all relevant studies that matched with our scope and inclusion criteria.
The keywords consisted of MeSH terms such as “safety [MeSH]” and “artificial intelligence [MeSH],” in combination with narrower MeSH terms (subheadings/related words/phrases) and free text for “artificial intelligence” and “safety.” We also included broader key terms to encompass all latent risk factors affecting patient safety. The final search keywords ( Figure 3 ) described below were used to explore all databases.

Medical Subject Heading (MeSH) terms and free text used in the systematic literature review.
MeSH terms are organized in a tree-like hierarchy, with more specific (narrower) terms arranged beneath broader terms. By default, PubMed includes all of the narrow items in the search in a strategy known as “exploding” the MeSH term [ 56 ]. Moreover, the inclusion of MeSH terms optimizes the search strategy [ 56 ]. Therefore, the final search query for PubMed was as follows: (“patient safety” OR “safety” [MeSH] OR “drug safety” OR “safety-based Drug withdraws” [MeSH] OR “medication error” OR “Medication Error” [MeSH] OR “medication reconciliation” OR “near miss” OR “inappropriate prescribing” OR “clinical error” OR “Clinical alarms” [MeSH]) AND (“Machine learning” [MeSH] OR “Machine learning” OR “Deep learning” [MeSH] OR “Deep learning” OR “natural language processing” [MeSH] OR “natural language processing”).
Inclusion and Exclusion Criteria
This study focused on peer-reviewed publications satisfying the following two primary conditions: (1) implementation of machine-learning or natural language processing techniques to address patient safety concerns, and (2) discussing or reporting the impact or changes in clinical-level patient safety outcomes. Any papers that failed to satisfy both conditions were excluded from this review. For instance, studies only focusing on developing or evaluating machine-learning models that did not report or discuss changes or impact on clinical-level patient safety outcomes were excluded, as well as studies that used AI beyond our scopes, such as robotics or computer vision. Secondary research such as reviews, commentaries, and conceptual articles was excluded from this study. The search was restricted to papers published in English between January 2009 and August 2019.
Study Selection and Quality Assurance
The two authors together reviewed all of the retrieved publications for eligibility. We first screened the publications by studying the titles and abstracts and removed duplications. We then read the full text for the remaining papers and finalized the selection. To minimize any selection bias, all discrepancies were resolved by discussion requiring consensus from both reviewers and the librarian. Before finalizing the list of papers, we consulted our results and searched keywords with the librarian to ensure that no relevant articles were missed.
A data abstraction form was used to record standardized information from each paper as follows: authors, aims, objectives of the study, methods, and findings. Using this form, we categorized each article based on the type of AI algorithm as well as clinical-level patient safety outcomes reported.
Study Selection
Figure 4 illustrates the flowchart of the selection process of the articles included in this systematic literature review. The initial search using a set of queries returned 272 publications in PubMed, 1976 publications in PubMed Central, and 248 publications in Web of Science for a total of 2496 articles. We used EndNote X9.3.2 to manage the filtering and duplication removal process. As a first step, we removed duplicates (n=101), all review/opinion/perspective papers (n=120), and posters or short abstracts (n=127). The two authors then applied a second filtering step by reading abstracts and titles (n=2148). The screening process followed the inclusion and exclusion criteria explained above, resulting in 80 papers eligible for a full-text review. The authors then removed 27 more articles based on the full-text review. Hence, the final number of studies included in the systematic review was 53, with consensus from both authors.

Preferred Reporting Items for Systematic Reviews and Meta-Analysis (PRISMA) flow chart illustrating the process of selecting eligible publications for inclusion in the systematic review. WoS: Web of Science.
Table 1 outlines all characteristics of the final selected studies (n=53), including the objective of the study and AI methods used, as well as classification of all articles by latent risk factors of patient safety according to (a) Clinical Alarms/Alerts, (b) Clinical Reports, and (c) Adverse Drug Event/Drug Safety. Table 1 also reports the findings obtained regarding changes in patient safety outcomes.
Evidentiary table of 53 selected publications.
a AI: artificial intelligence.
b KNN: K-nearest neighbor.
c NB: naive Bayes.
d LR: logistic regression.
e SVM: support vector machine.
f RF: random forest.
g AUC: area under the curve.
h ICU: intensive care unit.
i MMD: multimodal section.
j DT: decision tree.
k BCT: binary classification tree.
l RDAC: regularized discriminant analysis classifier.
m NN: neural network.
n DEWS: deep learning–based early warning system.
o MEWS: modified early warning system.
p AUPRC: area under the precision-recall curve.
q J48: decision tree algorithm.
r CC: closure classifier.
s MLP: multilayer perceptron.
t NLP: natural language processing.
u CNN: convolutional neural network.
v ET: extra tree.
w RNN: recurrent neural network.
x NCPS: National Center for Patient Safety.
y XGB: extreme gradient boosting.
z LASSO: least absolute shrinkage and selection operator.
aa HIT: health information technology.
bb PSE: patient safety event.
cc PANDIT: Patient Assisting Net-Based Diabetes Insulin Titration.
dd CHAID: Chi square automatic interaction detector.
ee CART: classification and regression tree.
ff SVR: support vector regression.
gg NN-BP: neural network-back propagation.
hh MT: model tree.
ii ABC4D: Advanced Bolus Calculator For Diabetes.
jj CSS: clinical support system.
kk BiLSTM: bi-long short-term memory neural network.
ll CRF-NN: conditional random field neural network.
mm LSTM-RNN: long short-term memory-recurrent neural network.
nn CRF: conditional random field neural network.
oo LRM: logistic regression probability model.
pp BNM: Bayesian network model.
qq BCP-NN: Bayesian confidence propagation neural network.
The studies mostly reported positive changes in patient safety outcomes, and in most cases improved or outperformed traditional methods. For instance, AI was successful in minimizing false alarms in several studies and also improved real-time safety reporting systems ( Table 1 ). AI was also able to extract useful information from clinical reports. For example, AI helped in classifying patients based on their ailments and severity, identified common incidents such as fall risks, delivery delays, hospital information technology errors, bleeding complications, and others that pose risks to patient safety. AI also helped in minimizing adverse drug effects. Further, some studies reported poor outcomes of AI, in which AI’s classification accuracy was lower than that of clinicians or existing standards.
Table 2 outlines the performance and accuracy measures of AI models used by the final selected studies, demonstrating the heterogeneity in AI performance measures adopted by different studies.
Performance of artificial intelligence.
a AUROC: area under the receiver operating characteristic curve.
b SVM: support vector machine.
c N/A: not applicable (Not reported).
d BCPNN: Bayesian confidence propagation neural network.
e NLP: natural language processing.
g CRF: conditional random field.
h RNN: recurrent neural network.
i BiLSTM: Bi-long short-term memory neural network.
j CARD: casual association rule discovery.
k LSVM: linear support vector machine.
l HAI: hospital-associated infection.
m SSI: surgical site infection.
n LRTI: lower respiratory tract infection.
o UTI: urinary tract infection.
p BSI: bloodstream infection.
q ADE: adverse drug event.
r CDS: clinical decision support.
s ABC4D: Advanced Bolus Calculator For Diabetes.
t CBR: case-based reasoning.
u AI: artificial intelligence.
v KNN: K-nearest neighbor.
w SVR: support vector regression.
x MLP: multilayer perceptron.
y CART: classification and regression tree.
z CHAID: Chi square automatic interaction detector.
aa PANDIT: Patient Assisting Net-Based Diabetes Insulin Titration.
bb SELF: semisupervised local Fisher discriminant analysis.
cc LASSO: least absolute shrinkage and selection operator.
dd AIML: artificial intelligence markup language.
ee RNN: recurrent neural network.
ff AUPRC: area under the precision-recall curve.
gg VieWS: VitalPac Early Warning Score.
hh CDSS: clinical decision support system.
ii EHR: electronic health record.
Study Themes and Findings
Clinical alarms and alerts.
Nine publications addressed clinical alarms/alerts using AI techniques. The most widely used method was random forest (n=5) followed by support vector machine (n=3) and neural network/deep learning (n=3).
Studies under this category used electrocardiogram data from the PhysioNet Challenge public database and PhysioNet MIMIC II database. Five studies focused on reducing false alarm rates arising due to cardiac ailments such as arrhythmia and cardiac arrest in an intensive care unit setting [ 58 - 61 , 65 ]. The remaining four studies focused on improving the performance of clinical alarms in classifying clinical deterioration such as fluctuation in vital signs [ 57 ], predicting adverse events [ 62 ], identifying adverse medication events [ 63 ], and deterioration of patient health with hematologic malignancies [ 64 ].
Clinical Reports
We identified 21studies concerning clinical reports. Studies in this group primarily focused on extracting information from clinical reports such as safety reports (internal to the hospital), patient feedback, EHR notes, and others typically derived from incident monitoring systems and patient safety organizations. The most widely used method was support vector machine (n=11), followed by natural language processing (n=7) and naïve Bayes (n=5). We also identified decision trees (n=4), deep learning models (n=3), J48 (n=2), and other (n=9) algorithms.
The majority of articles focused on automating the process of patient safety classifications. These studies used machine learning and natural language processing techniques to classify clinical incidents [ 66 ] from the Incident Information Management System and to identify risky incidents [ 71 , 79 , 81 , 108 ] in patient safety reports retrieved from different sources, including the university database and the Veterans Affairs National Center for Patient Safety database. Some studies also analyzed medication reports [ 49 ] from structured and unstructured data obtained from the patient safety organization, and evaluated patient feedback [ 69 ] retrieved from the Patient Advocacy Reporting System developed at Vanderbilt and associated institutions.
Several studies focused on classifying the type and severity of patient safety incident reports using data collected by different sources such as universities [ 75 ], and incident reporting systems such as Advanced Incident Management Systems (across Australia) and Riskman [ 67 , 75 , 76 ]. Others analyzed hospital clinical notes internally (manually annotated by clinicians and a quality committee) and data retrieved from patient safety organizations to identify adverse incidents such as delayed medication [ 68 ], fall risks [ 47 , 67 ], near misses, patient misidentification, spelling errors, and ambiguity in clinical notes [ 109 ]. One study analyzed clinical study descriptions from clinicaltrials.gov and implemented an AI system to detect all abbreviations and identify their meaning to minimize incorrect interpretations [ 70 ]. Another study used inpatient laboratory test reports from Sunquest Laboratory Information System and identified wrong blood in tube errors [ 80 ].
Studies used clinical reports from various sources, including patient safety organizations, EHR data from Veterans Health Administration and Berkshire Health Systems, and deidentified notes from the Medical Information Mart for Intensive Care. These studies focused on extracting relevant information [ 74 , 77 , 82 , 84 ] to predict bleeding risks among critically ill patients [ 73 ], postoperative surgical complications [ 78 ], mortality risk [ 83 ], and other factors such as lab test results and vital signs [ 77 ] influencing patient safety outcomes.
Adverse Drug Events or Drug Safety
Twenty-three publications were classified under drug safety. These studies primarily addressed adverse effects related to drug reactions. The most widely used method was random forest (n=8), followed by natural language processing (n=7) and logistic regression (n=6). Algorithms including natural language processing (n=5), logistic regression (n=4), mobile or web apps (n=3), AI devices (n=2), and others (n=5) were also used.
Studies in this category retrieved data from different repositories such as DrugBank, Side Effect Resource, the Food and Drug Administration (FDA)’s adverse event reporting system, University of Massachusetts Medical School, Observational Medical Outcomes Partnership database, and Human Protein-Protein Interaction database to identify adverse drug interactions and reactions that can potentially negatively influence patient health [ 86 - 88 , 101 , 102 , 105 - 107 , 110 ]. Some studies also used AI to predict drug interactions by analyzing EHR data [ 88 ], unstructured discharge notes [ 90 ], and clinical charts [ 99 , 104 ]. One study also used AI to identify drugs that were withdrawn from the commercial markets by the FDA [ 100 ].
Some studies used AI to predict the dosage of medicines such as insulin, digoxin, and warfarin [ 85 , 89 , 91 , 95 ]. AI in drug safety was also used to scan through the hospital’s EHR data and identify medication errors (ie, wrong medication prescriptions) [ 96 ]. One study used AI to monitor stroke patients and track their medication (anticoagulation) intake [ 93 ]. Several studies used AI to predict a medication that a patient could be consuming but was missing from their medication list or health records [ 92 , 94 , 97 ]. Another study used AI to review clinical notes and identify evidence of opioid abuse [ 98 ].
Visual Representations of Safety and Chronology of the Studies
Figure 5 illustrates the details of patient safety issues/outcomes studied and reported under each classified theme using AI algorithms at the clinical level.

Identified factors influencing patient safety outcomes. EHR: electronic health record.
Figure 6 further shows how the application of AI in studies reporting patient safety outcomes in our review evolved over time between January 2009 and August 2019.

Timeline of artificial intelligence application to address factors influencing patient safety (clinical reports, drug safety, and clinical alarms) between 2009 and August 2019. ABC4D: Advanced Bolus Calculator For Diabetes; AI: artificial intelligence; BCP-NN: Bayesian confidence propagation neural network; BCT: binary classification tree; BiLSTM: bi-long short-term memory neural network; BNM: Bayesian network model; CART: classification and regression tree; CHAID: Chi-square automatic interaction detector; CRF-NN: conditional random field neural network; DEWS: deep learning-based early warning system; DT: decision tree; KNN, K-nearest neighbor; LASSO: least absolute shrinkage and selection operator; LR: logistic regression; LSTM-RNN: long short-term memory-recurrent neural network; MEWS: modified early warning system; ML: machine learning; MLP: multilayer perception; MMD; multimodal detection; MT: model tree; NB: naive Bayes; NLP: natural language processing; NN: neural network; NN-BP: neural network back propagation; PANDIT: Patient Assisting Net-Based Diabetes Insulin Titration; RF: random forest; RNN: recurrent neural network; SVM: support vector machine; SVR, support vector regression; XGB; extreme gradient boosting.
Principal Findings
Many studies have been conducted to exhibit the analytical performance of AI in health care, particularly as a diagnostic and prognostic tool. To our knowledge, this is the first systematic review exploring and portraying studies that show the influence of AI (machine-learning and natural language processing techniques) on clinical-level patient safety outcomes. We identified 53 studies within the scope of the review. These 53 studies used 38 different types of AI systems/models to address patient safety outcomes, among which support vector machine (n=17) and natural language processing (n=12) were the most frequently used. Most of the reviewed studies reported positive changes in patient safety outcomes.
Analysis of all studies showed that there is a lack of a standardized benchmark among reported AI models. Despite varying AI performance, most studies have reported a positive impact on safety outcomes ( Table 2 ), thus indicating that safety outcomes do not necessarily correlate to AI performance measures [ 26 ]. For example, one identified study with an accuracy of 0.63 that implemented Patient Assisting Net-Based Diabetes Insulin Titration (PANDIT) reported a negative impact of AI on safety outcomes. The PANDIT-generated recommendations that did not match with the recommendations of nurses (1.4% of the recommendations) were identified as unsafe [ 85 ]. In contrast, the study implementing natural language processing to extract clinical information from patient safety reports showed a positive impact on patient safety outcomes with accuracy of 0.53 [ 81 ]. Similarly, the FDA-approved computer-aided diagnosis of the 1990s, which significantly increased the recall rate of diagnosis, did not improve safety or patient outcomes [ 111 ]. According to our review, AI algorithms are rarely scrutinized against a standard of care (clinicians or clinical gold standard). Relying on AI outcomes that have not been evaluated against a standard benchmark that meets clinical requirements can be misleading. A study conducted in 2008 [ 112 ] developed and validated an advanced version of the QRISK cardiovascular disease risk algorithm (QRISK2). The study reported improved performance of QRISK2 when compared to its earlier version. However, QRISK2 was not compared against any clinical gold standard. Eight years later, in 2016, The Medicines & Healthcare Products Regulatory Agency identified an error in the QRISK 2 calculator [ 113 ]; QRISK2 underestimated or overestimated the potential risk of cardiovascular disease. The regulatory agency reported that a third of general practitioner surgeries in England might have been affected [ 113 ] due to the error in QRISK2. Globally, there are several Standards Development Organizations developing information technology and AI standards to address varying standardization needs in the domain of cloud computing, cybersecurity, and the internet of things [ 114 ]. However, there has been minimal effort to standardize AI in the field of health care. Health care comprises multiple departments, each having unique or different requirements (clinical standards). Thus, health care requires so-called “vertical standards,” which are standards developed for specific application areas such as drug safety (pharmaceuticals), specific surgeries, outpatients and inpatients with specific health concerns, and emergency departments [ 114 ]. In contrast, standards that are not correctly tailored for a specific purpose may hamper patient safety.
Without a standardized benchmark, it becomes challenging to evaluate whether a particular AI system meets clinical requirements (gold standard) or performs significantly better (improves patient safety) or worse (harms patient) than other similar systems in a given health care context. To generate the best possible (highest) performance outcome, AI algorithms may include unreliable confounders into the computing process. For instance, in one study, an algorithm was more likely to classify a skin lesion as malignant if an image (input data) had a ruler in it because the presence of a ruler correlated with an increased likelihood of a cancerous lesion [ 115 ]. The presence of surgical skin markings has also been shown to falsely increase a deep-learning model’s melanoma probability scores and hence the false-positive rate [ 116 ]. Moreover, there has been great emphasis focused on the importance to standardization of AI by developed countries such as the European Union, United States, China, and Japan. For instance, on February 11, 2019, the President of the United States issued an Executive Order (EO 13859) [ 117 ] directing federal agencies to actively participate in AI standards development. According to the Center for Data Innovation and The National Institute of Standards and Technology, a standardized AI benchmark can serve as a mechanism to evaluate and compare AI systems [ 114 ]. FDA Commissioner Scott Gottlieb acknowledged the importance of AI standardization that can assure that ongoing algorithm changes follow prespecified performance objectives and use a validation process that ensures safety [ 118 ].
Another major finding of this review is high heterogeneity in AI reporting. AI systems have been developed to help clinicians in estimating risks and making informed decisions. However, the evidence indicates that the quality of reporting of AI model studies is heterogeneous (not standard). Table 2 demonstrates how different studies that implemented the same AI used different evaluation metrics to measure its performance. Heterogeneity in AI reporting also makes the comparison of algorithms across studies challenging and might cause difficulties in obtaining consensus while attempting to select the best AI for a given situation. Algorithms not only need to be subjected to comparison on the same data that are representative of the target population but also the same evaluation metrics; thus, standardized reporting of AI studies would be beneficial. The current Transparent Reporting of a multivariable prediction model for Individual Prognosis Or Diagnosis (TRIPOD) consists of 22-item checklists that aim to improve the reporting of studies developing or validating a prediction model [ 119 , 120 ]. Studies in our review did not use TRIPOD to report findings. The possible reason behind this can be the design of TRIPOD, which focuses on a regression-based prediction model.
However, the explanation and elaboration document provides examples of good reporting methods, which are focused on models developed using regression. Therefore, a new version of the TRIPOD statement that is specific to AI/machine-learning systems (TRIPOD-ML) is in development. It will focus on the introduction of machine-learning prediction algorithms to establish methodological and reporting standards for machine-learning studies in health care [ 121 ].
Our findings also identified the need to determine the importance of an AI evaluation metric. In particular, it is important to determine which evaluation metric(s) should be measured in a given health care context. AUROC is considered to be a superior metric for classification accuracy, particularly when unbalanced datasets are used [ 122 , 123 ] because it is unaffected by unbalanced data, which is typical in health care. However, 36 studies in our review did not report AUROC. Evaluation measures such as precision-recall can also reflect model performance accurately [ 123 ]; however, only 11 studies in our review evaluated AI based on precision-recall. Using inappropriate measures to evaluate AI performance might impose a threat to patient safety. However, no threat to patient safety due to the use of inappropriate AI evaluation metric was identified in our review. Future studies should report the importance of evaluation metrics and determine which measure (single or multiple measures) is more important and a better representation of patient safety outcomes. More studies are needed to explore the evaluation metric(s) that should be considered before recommending an AI model.
The findings of our review demonstrate that drug safety, followed by the analysis of clinical reports, has been the most common area of interest for the use of AI to address clinical-level patient safety concerns. The wrong medication or improper dosage can result in fatal patient health outcomes and medical malpractice [ 91 ]. Of all drug safety concerns, issues related to inappropriate doses of high-alert medications are of great interest to the Joint Commission on Accreditation of Healthcare Organizations [ 91 , 124 ]. Medical errors are reported as the third leading cause of death in the United States. The majority of the papers in our review implemented AI to address drug safety (n=23) concerns, which is one of the most significant contributors to overall medical errors. These publications improved patient safety by identifying adverse drug reactions and preventing incorrect medications or overdoses. Future studies should further explore how to use AI systems on a larger scale to diminish medication errors at hospitals and clinics to save more lives.
Finally, the studies reviewed in this paper have addressed safety issues as identified by the Health Insurance Portability and Accountability Act (HIPAA) and the US Department of Health & Human Services (HHS). The HIPAA regulations identify risk analysis as part of the administrative safeguard requirement to improve patient safety. The HHS advocates analysis of clinical notes to track, detect, and evaluate potential risks to patients. Many studies (n=21) in our review used AI to identify patient risk from clinical notes. These studies used AI and clinical reports to extract safety-related information such as fall risks, pyxis discrepancies, patient misidentification, patient severity, and postoperative surgical complications. Our findings exhibit how, with the help of AI techniques such as natural language processing, clinical notes and reports have been used as a data source to extract patient data regarding a broad range of safety issues, including clinical notes, discharge notes, and other issues [ 69 , 70 , 73 , 84 ]. Our review also indicates that AI has the potential to provide valuable insights to treat patients correctly by identifying future health or safety risks [ 125 ], to improve health care quality, and reduce clinical errors [ 126 ]. Despite being recognized as one of the major factors responsible for fatigue, burnout in clinicians, and patient harm [ 61 , 127 - 129 ], only 9 studies in our review used AI to improve clinical alarms. Although studies addressing clinical alarms reported positive outcomes by minimizing false alarms and identifying patient health deterioration, the limited number of studies (n= 9) addressing these issues shows that the field is still in a nascent period of investigation. Thus, more research is needed to confirm the impact of AI on patient safety outcomes.
Recommendations for Future Research
Future studies should work toward establishing a gold standard (for various health care contexts/ disease types/problem types) against which AI performance can be measured. Future research, as suggested by Kelly and others in 2019 [ 119 ], should also develop a common independent test (preferably for different problem types, drug safety/clinical alarms/clinical reports) using unenriched representative sample data that are not available to train algorithms.
Our review acknowledges that no single measure captures all of the desirable properties of a model, and multiple measures are typically required to summarize model performance. However, different measures are indicative of different types of analytical performance. Future studies should develop a standard framework that can guide clinicians in interpreting the clinical meaning of AI’s evaluation metrics before integrating it into the clinical workflow. Future studies should also report a quantifiable measure of AI demonstrating not only its analytical performance but also its impact on patient safety (long and short term), reliability, domain-specific risks, and uncertainty. Additionally, studies should also ensure data standardization.
Health databases or storage systems are often not compatible (integratable) across different hospitals, care providers, or different departments in the same hospital. Data in health care are largely unorganized and unstructured [ 9 , 50 ]. Since the performance of AI heavily depends on data, regulatory bodies should invest in data infrastructure such as standardization of EHRs and integration of different health databases. AI trained on unstructured or biased data might generate misleading results [ 51 ]. According to the National Institute of Standards and Technology (NIST), standardized data can make the training data (machine learning input) more visible and usable to authorized users. It can also ensure data quality and improve AI performance.
Most of the safety initiatives implemented in health care over the last decade have been focused on analyzing historical events to learn and evolve [ 130 , 131 ]. The same was also observed in our review. AI models were trained on past data. However, in health care, outcomes are satisfactory because providers make sensible and just-in-time adjustments according to the demands of the situation. Future work should train AI on the critical adjustments made by clinicians, so that AI can adapt to different conditions in the same manner as clinicians.
The integration of AI systems into the health system will alter the role of providers. Ideally, AI systems are expected to assist providers in making faster and more accurate decisions and to deliver personalized patient care. However, lack of appropriate knowledge of using complex AI systems and interpreting their outcome might impose a high cognitive workload on providers. Thus, the medical education system should incorporate necessary AI training for providers so that they can better understand the basic functioning of AI systems and extract clinically meaningful insight from the outcomes of AI.
Limitation of this Review
This study encompasses publications that matched our inclusion criteria and operational definition of AI and patient safety. In addition, we limited the scope of AI to only machine learning and natural language processing at a clinical level. This review also only included studies published in English in the last 10 years.
This systematic review identified critical research gaps that need attention from the scientific community. The majority of the studies in the review have not highlighted significant aspects of AI, such as (a) heterogeneity in AI reporting, (b) lack of a standardized benchmark, and (c) need to determine the importance of AI evaluation metric. The identified flaws of AI systems indicate that further research is needed, as well as the involvement of the FDA and NIST to develop a framework standardizing AI evaluation measures and set a benchmark to ensure patient safety. Thus, our review encourages the health care domain and AI developers to adopt an interdisciplinary and systems approach to study the overall impact of AI on patient safety outcomes and other contexts in health care.
Abbreviations
Multimedia appendix 1.
Conflicts of Interest: None declared.
Analyze research papers at superhuman speed
Search for research papers, get one sentence abstract summaries, select relevant papers and search for more like them, extract details from papers into an organized table.
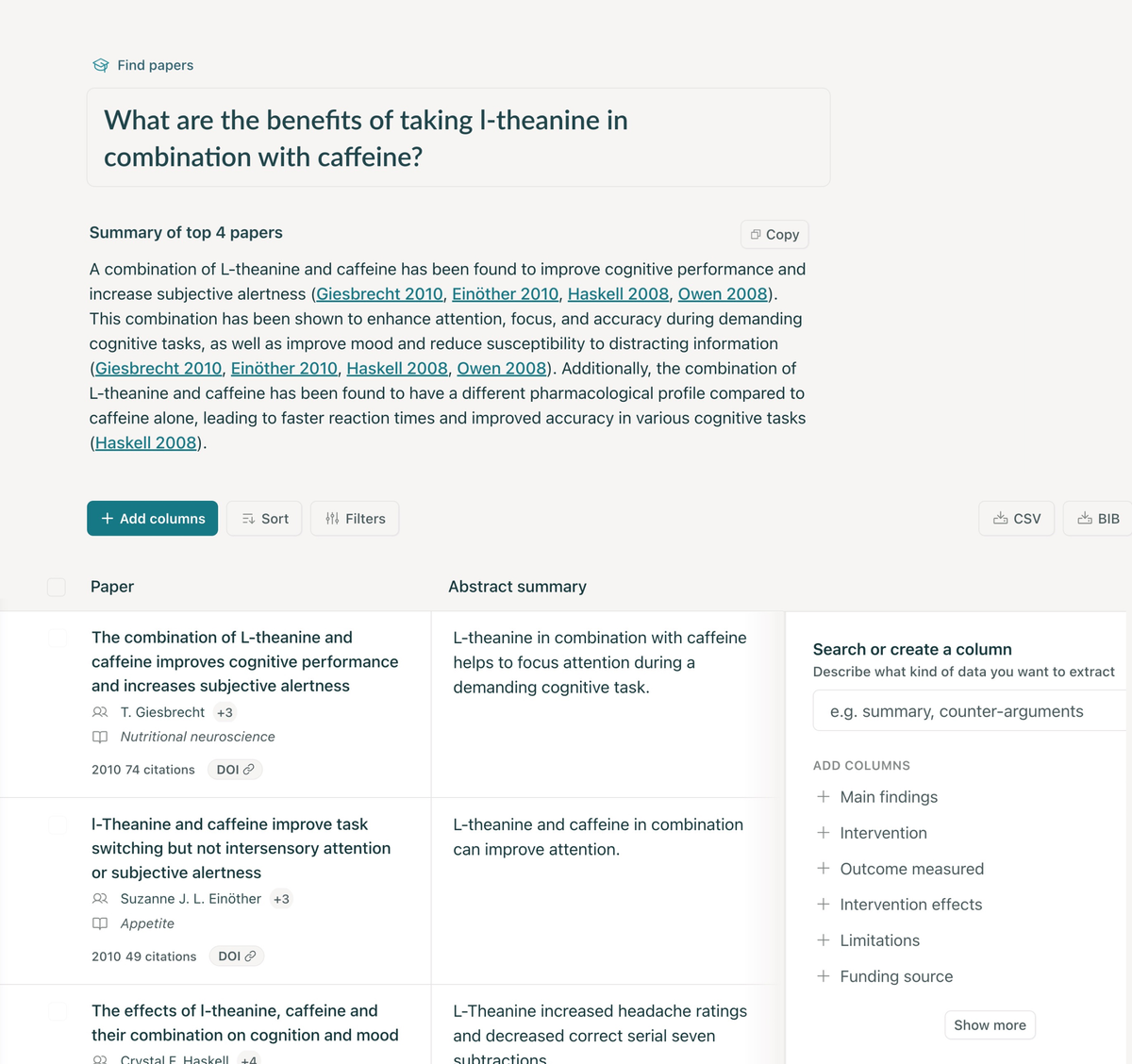
Find themes and concepts across many papers
Don't just take our word for it.
.webp)
Tons of features to speed up your research
Upload your own pdfs, orient with a quick summary, view sources for every answer, ask questions to papers, research for the machine intelligence age, pick a plan that's right for you, get in touch, enterprise and institutions, custom pricing, common questions. great answers., how do researchers use elicit.
Over 2 million researchers have used Elicit. Researchers commonly use Elicit to:
- Speed up literature review
- Find papers they couldn’t find elsewhere
- Automate systematic reviews and meta-analyses
- Learn about a new domain
Elicit tends to work best for empirical domains that involve experiments and concrete results. This type of research is common in biomedicine and machine learning.
What is Elicit not a good fit for?
Elicit does not currently answer questions or surface information that is not written about in an academic paper. It tends to work less well for identifying facts (e.g. “How many cars were sold in Malaysia last year?”) and theoretical or non-empirical domains.
What types of data can Elicit search over?
Elicit searches across 125 million academic papers from the Semantic Scholar corpus, which covers all academic disciplines. When you extract data from papers in Elicit, Elicit will use the full text if available or the abstract if not.
How accurate are the answers in Elicit?
A good rule of thumb is to assume that around 90% of the information you see in Elicit is accurate. While we do our best to increase accuracy without skyrocketing costs, it’s very important for you to check the work in Elicit closely. We try to make this easier for you by identifying all of the sources for information generated with language models.
What is Elicit Plus?
Elicit Plus is Elicit's subscription offering, which comes with a set of features, as well as monthly credits. On Elicit Plus, you may use up to 12,000 credits a month. Unused monthly credits do not carry forward into the next month. Plus subscriptions auto-renew every month.
What are credits?
Elicit uses a credit system to pay for the costs of running our app. When you run workflows and add columns to tables it will cost you credits. When you sign up you get 5,000 credits to use. Once those run out, you'll need to subscribe to Elicit Plus to get more. Credits are non-transferable.
How can you get in contact with the team?
Please email us at [email protected] or post in our Slack community if you have feedback or general comments! We log and incorporate all user comments. If you have a problem, please email [email protected] and we will try to help you as soon as possible.
What happens to papers uploaded to Elicit?
When you upload papers to analyze in Elicit, those papers will remain private to you and will not be shared with anyone else.
How accurate is Elicit?
Training our models on specific tasks, searching over academic papers, making it easy to double-check answers, save time, think more. try elicit for free..
Help | Advanced Search
Computer Science > Computers and Society
Title: ethics of ai: a systematic literature review of principles and challenges.
Abstract: Ethics in AI becomes a global topic of interest for both policymakers and academic researchers. In the last few years, various research organizations, lawyers, think tankers and regulatory bodies get involved in developing AI ethics guidelines and principles. However, there is still debate about the implications of these principles. We conducted a systematic literature review (SLR) study to investigate the agreement on the significance of AI principles and identify the challenging factors that could negatively impact the adoption of AI ethics principles. The results reveal that the global convergence set consists of 22 ethical principles and 15 challenges. Transparency, privacy, accountability and fairness are identified as the most common AI ethics principles. Similarly, lack of ethical knowledge and vague principles are reported as the significant challenges for considering ethics in AI. The findings of this study are the preliminary inputs for proposing a maturity model that assess the ethical capabilities of AI systems and provide best practices for further improvements.
Submission history
Access paper:.
- Other Formats

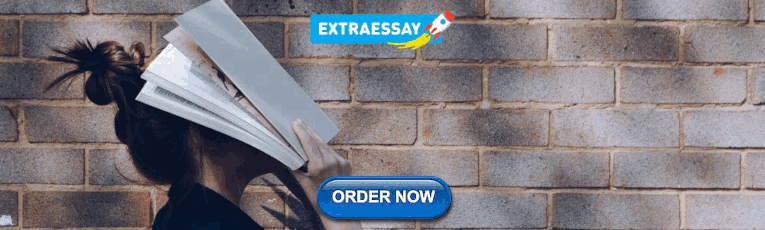
References & Citations
- Google Scholar
- Semantic Scholar
DBLP - CS Bibliography
Bibtex formatted citation.

Bibliographic and Citation Tools
Code, data and media associated with this article, recommenders and search tools.
- Institution
arXivLabs: experimental projects with community collaborators
arXivLabs is a framework that allows collaborators to develop and share new arXiv features directly on our website.
Both individuals and organizations that work with arXivLabs have embraced and accepted our values of openness, community, excellence, and user data privacy. arXiv is committed to these values and only works with partners that adhere to them.
Have an idea for a project that will add value for arXiv's community? Learn more about arXivLabs .
- Review article
- Open access
- Published: 31 October 2023
Role of AI chatbots in education: systematic literature review
- Lasha Labadze ORCID: orcid.org/0000-0002-8884-2792 1 ,
- Maya Grigolia ORCID: orcid.org/0000-0001-9043-7932 2 &
- Lela Machaidze ORCID: orcid.org/0000-0001-5958-5662 3
International Journal of Educational Technology in Higher Education volume 20 , Article number: 56 ( 2023 ) Cite this article
50k Accesses
28 Citations
153 Altmetric
Metrics details
A Correction to this article was published on 15 April 2024
This article has been updated
AI chatbots shook the world not long ago with their potential to revolutionize education systems in a myriad of ways. AI chatbots can provide immediate support by answering questions, offering explanations, and providing additional resources. Chatbots can also act as virtual teaching assistants, supporting educators through various means. In this paper, we try to understand the full benefits of AI chatbots in education, their opportunities, challenges, potential limitations, concerns, and prospects of using AI chatbots in educational settings. We conducted an extensive search across various academic databases, and after applying specific predefined criteria, we selected a final set of 67 relevant studies for review. The research findings emphasize the numerous benefits of integrating AI chatbots in education, as seen from both students' and educators' perspectives. We found that students primarily gain from AI-powered chatbots in three key areas: homework and study assistance, a personalized learning experience, and the development of various skills. For educators, the main advantages are the time-saving assistance and improved pedagogy. However, our research also emphasizes significant challenges and critical factors that educators need to handle diligently. These include concerns related to AI applications such as reliability, accuracy, and ethical considerations.
Introduction
The traditional education system faces several issues, including overcrowded classrooms, a lack of personalized attention for students, varying learning paces and styles, and the struggle to keep up with the fast-paced evolution of technology and information. As the educational landscape continues to evolve, the rise of AI-powered chatbots emerges as a promising solution to effectively address some of these issues. Some educational institutions are increasingly turning to AI-powered chatbots, recognizing their relevance, while others are more cautious and do not rush to adopt them in modern educational settings. Consequently, a substantial body of academic literature is dedicated to investigating the role of AI chatbots in education, their potential benefits, and threats.
AI-powered chatbots are designed to mimic human conversation using text or voice interaction, providing information in a conversational manner. Chatbots’ history dates back to the 1960s and over the decades chatbots have evolved significantly, driven by advancements in technology and the growing demand for automated communication systems. Created by Joseph Weizenbaum at MIT in 1966, ELIZA was one of the earliest chatbot programs (Weizenbaum, 1966 ). ELIZA could mimic human-like responses by reflecting user inputs as questions. Another early example of a chatbot was PARRY, implemented in 1972 by psychiatrist Kenneth Colby at Stanford University (Colby, 1981 ). PARRY was a chatbot designed to simulate a paranoid patient with schizophrenia. It engaged in text-based conversations and demonstrated the ability to exhibit delusional behavior, offering insights into natural language processing and AI. Developed by Richard Wallace in 1995, ALICE (Artificial Linguistic Internet Computer Entity) was an early example of a chatbot using natural language processing techniques that won the Loebner Prize Turing Test in 2000–2001 (Wallace, 1995 ), which challenged chatbots to convincingly simulate human-like conversation. Later in 2001 ActiveBuddy, Inc. developed the chatbot SmarterChild that operated on instant messaging platforms such as AOL Instant Messenger and MSN Messenger (Hoffer et al., 2001 ). SmarterChild was a chatbot that could carry on conversations with users about a variety of topics. It was also able to learn from its interactions with users, which made it more and more sophisticated over time. In 2011 Apple introduced Siri as a voice-activated personal assistant for its iPhone (Aron, 2011 ). Although not strictly a chatbot, Siri showcased the potential of conversational AI by understanding and responding to voice commands, performing tasks, and providing information. In the same year, IBM's Watson gained fame by defeating human champions in the quiz show Jeopardy (Lally & Fodor, 2011 ). It demonstrated the power of natural language processing and machine learning algorithms in understanding complex questions and providing accurate answers. More recently, in 2016, Facebook opened its Messenger platform for chatbot development, allowing businesses to create AI-powered conversational agents to interact with users. This led to an explosion of chatbots on the platform, enabling tasks like customer support, news delivery, and e-commerce (Holotescu, 2016 ). Google Duplex, introduced in May 2018, was able to make phone calls and carry out conversations on behalf of users. It showcased the potential of chatbots to handle complex, real-time interactions in a human-like manner (Dinh & Thai, 2018 ; Kietzmann et al., 2018 ).
More recently, more sophisticated and capable chatbots amazed the world with their abilities. Among them, ChatGPT and Google Bard are among the most profound AI-powered chatbots. ChatGPT is an artificial intelligence chatbot developed by OpenAI. It was first announced in November 2022 and is available to the general public. ChatGPT’s rival Google Bard chatbot, developed by Google AI, was first announced in May 2023. Both Google Bard and ChatGPT are sizable language model chatbots that undergo training on extensive datasets of text and code. They possess the ability to generate text, create diverse creative content, and provide informative answers to questions, although their accuracy may not always be perfect. The key difference is that Google Bard is trained on a dataset that includes text from the internet, while ChatGPT is trained on a dataset that includes text from books and articles. This means that Google Bard is more likely to be up-to-date on current events, while ChatGPT is more likely to be accurate in its responses to factual questions (AlZubi et al., 2022 ; Rahaman et al., 2023 ; Rudolph et al., 2023 ).
Chatbots are now used across various sectors, including education. Most of the latest intelligent AI chatbots are web-based platforms that adapt to the behaviors of both instructors and learners, enhancing the educational experience (Chassignol et al., 2018 ; Devedzic, 2004 ; Kahraman et al., 2010 ; Peredo et al., 2011 ). AI chatbots have been applied in both instruction and learning within the education sector. Chatbots specialize in personalized tutoring, homework help, concept learning, standardized test preparation, discussion and collaboration, and mental health support. Some of the most popular AI-based tools /chatbots used in education are:
Bard, introduced in 2022, is a large language model chatbot created by Google AI. Its capabilities include generating text, language translation, producing various types of creative content, and providing informative responses to questions. (Rudolph et al., 2023 ). Bard is still under development, but it has the potential to be a valuable tool for education.
ChatGPT, launched in 2022 by OpenAI, is a large language model chatbot that can generate text, produce diverse creative content, and deliver informative answers to questions (Dergaa et al., 2023 ; Khademi, 2023 ; Rudolph et al., 2023 ). However, as discussed in the results section of this paper, there are numerous concerns related to the use of ChatGPT in education, such as accuracy, reliability, ethical issues, etc.
Ada, launched in 2017, is a chatbot that is used to provide personalized tutoring to students. It can answer questions, provide feedback, and facilitate individualized learning for students (Kabiljo et al., 2020 ; Konecki et al., 2023 ). However, the Ada chatbot has limitations in understanding complex queries. It could misinterpret context and provide inaccurate responses
Replika, launched in 2017, is an AI chatbot platform that is designed to be a friend and companion for students. It can listen to students' problems, offer advice, and help them feel less alone (Pentina et al., 2023 ; Xie & Pentina, 2022 ). However, given the personal nature of conversations with Replika, there are valid concerns regarding data privacy and security.
Socratic, launched in 2013, had the goal of creating a community that made learning accessible to all students. Currently, Socratic is an AI-powered educational platform that was acquired by Google in 2018. While not a chatbot per se, it has a chatbot-like interface and functionality designed to assist students in learning new concepts (Alsanousi et al., 2023 ; Moppel, 2018 ; St-Hilaire et al., 2022 ). Like with other chatbots, a concern arises where students might excessively rely on Socratic for learning. This could lead to a diminished emphasis on critical thinking, as students may opt to use the platform to obtain answers without gaining a genuine understanding of the underlying concepts.
Habitica, launched in 2013, is used to help students develop good study habits. It gamifies the learning process, making it more fun and engaging for students. Students can use Habitica to manage their academic tasks, assignments, and study schedules. By turning their to-do list into a game-like experience, students are motivated to complete their tasks and build productive habits (Sales & Antunes, 2021 ; Zhang, 2023 ). However, the gamified nature of Habitica could inadvertently introduce distractions, especially for students who are easily drawn into the gaming aspect rather than focusing on their actual academic responsibilities.
Piazza launched in 2009, is used to facilitate discussion and collaboration in educational settings, particularly in classrooms and academic institutions. It provides a space for students and instructors to engage in discussions, ask questions, and share information related to course content and assignments (Ruthotto et al., 2020 ; Wang et al., 2020 ). Because discussions on Piazza are user-generated, the quality and accuracy of responses can vary. This variability may result in situations where students do not receive accurate and helpful information.
We will likely see even more widespread adoption of chatbots in education in the years to come as technology advances further. Chatbots have enormous potential to improve teaching and learning. A large body of literature is devoted to exploring the role, challenges, and opportunities of chatbots in education. This paper gathers and synthesizes this vast amount of literature, providing a comprehensive understanding of the current research status concerning the influence of chatbots in education. By conducting a systematic review, we seek to identify common themes, trends, and patterns in the impact of chatbots on education and provide a holistic view of the research, enabling researchers, policymakers, and educators to make evidence-based decisions. One of the main objectives of this paper is to identify existing research gaps in the literature to pinpoint areas where further investigation is needed, enabling researchers to contribute to the knowledge base and guide future research efforts. Firstly, we aim to understand the primary advantages of incorporating AI chatbots in education, focusing on the perspectives of students. Secondly, we seek to explore the key advantages of integrating AI chatbots from the standpoint of educators. Lastly, we endeavor to comprehensively analyze the major concerns expressed by scholars regarding the integration of AI chatbots in educational settings. Corresponding research questions are formulated in the section below. Addressing these research questions, we aim to contribute valuable insights that shed light on the potential benefits and challenges associated with the utilization of AI chatbots in the field of education.
The paper follows a structured outline comprising several sections. Initially, we provide a summary of existing literature reviews. Subsequently, we delve into the methodology, encompassing aspects such as research questions, the search process, inclusion and exclusion criteria, as well as the data extraction strategy. Moving on, we present a comprehensive analysis of the results in the subsequent section. Finally, we conclude by addressing the limitations encountered during the study and offering insights into potential future research directions.
Summary of existing literature reviews
Drawing from extensive systematic literature reviews, as summarized in Table 1 , AI chatbots possess the potential to profoundly influence diverse aspects of education. They contribute to advancements in both teaching and learning processes. However, it is essential to address concerns regarding the irrational use of technology and the challenges that education systems encounter while striving to harness its capacity and make the best use of it.
It is evident that chatbot technology has a significant impact on overall learning outcomes. Specifically, chatbots have demonstrated significant enhancements in learning achievement, explicit reasoning, and knowledge retention. The integration of chatbots in education offers benefits such as immediate assistance, quick access to information, enhanced learning outcomes, and improved educational experiences. However, there have been contradictory findings related to critical thinking, learning engagement, and motivation. Deng and Yu ( 2023 ) found that chatbots had a significant and positive influence on numerous learning-related aspects but they do not significantly improve motivation among students. Contrary, Okonkwo and Ade-Ibijola (Okonkwo & Ade-Ibijola, 2021 ), as well as (Wollny et al., 2021 ) find that using chatbots increases students’ motivation.
In terms of application, chatbots are primarily used in education to teach various subjects, including but not limited to mathematics, computer science, foreign languages, and engineering. While many chatbots follow predetermined conversational paths, some employ personalized learning approaches tailored to individual student needs, incorporating experiential and collaborative learning principles. Challenges in chatbot development include insufficient training datasets, a lack of emphasis on usability heuristics, ethical concerns, evaluation methods, user attitudes, programming complexities, and data integration issues.
Although existing systematic reviews have provided valuable insights into the impact of chatbot technology in education, it's essential to acknowledge that the field of chatbot development is continually emerging and requires timely, and updated analysis to ensure that the information and assessments reflect the most recent advancements, trends, or developments in chatbot technology. The latest chatbot models have showcased remarkable capabilities in natural language processing and generation. Additional research is required to investigate the role and potential of these newer chatbots in the field of education. Therefore, our paper focuses on reviewing and discussing the findings of these new-generation chatbots' use in education, including their benefits and challenges from the perspectives of both educators and students.
There are a few aspects that appear to be missing from the existing literature reviews: (a) The existing findings focus on the immediate impact of chatbot usage on learning outcomes. Further research may delve into the enduring impacts of integrating chatbots in education, aiming to assess their sustainability and the persistence of the observed advantages over the long term. (b) The studies primarily discuss the impact of chatbots on learning outcomes as a whole, without delving into the potential variations based on student characteristics. Investigating how different student groups, such as age, prior knowledge, and learning styles, interact with chatbot technology could provide valuable insights. (c) Although the studies highlight the enhancements in certain learning components, further investigation could explore the specific pedagogical strategies employed by chatbots to achieve these outcomes. Understanding the underlying mechanisms and instructional approaches utilized by chatbots can guide the development of more effective and targeted educational interventions. (d) While some studies touch upon user attitudes and acceptance, further research can delve deeper into the user experience of interacting with chatbots in educational settings. This includes exploring factors such as usability, perceived usefulness, satisfaction, and preferences of students and teachers when using chatbot technology.
Addressing these gaps in the existing literature would significantly benefit the field of education. Firstly, further research on the impacts of integrating chatbots can shed light on their long-term sustainability and how their advantages persist over time. This knowledge is crucial for educators and policymakers to make informed decisions about the continued integration of chatbots into educational systems. Secondly, understanding how different student characteristics interact with chatbot technology can help tailor educational interventions to individual needs, potentially optimizing the learning experience. Thirdly, exploring the specific pedagogical strategies employed by chatbots to enhance learning components can inform the development of more effective educational tools and methods. Lastly, a deeper exploration of the user experience with chatbots, encompassing usability, satisfaction, and preferences, can provide valuable insights into enhancing user engagement and overall satisfaction, thus guiding the future design and implementation of chatbot technology in education.
Methodology
A systematic review follows a rigorous methodology, including predefined search criteria and systematic screening processes, to ensure the inclusion of relevant studies. This comprehensive approach ensures that a wide range of research is considered, minimizing the risk of bias and providing a comprehensive overview of the impact of AI in education. Firstly, we define the research questions and corresponding search strategies and then we filter the search results based on predefined inclusion and exclusion criteria. Secondly, we study selected articles and synthesize results and lastly, we report and discuss the findings. To improve the clarity of the discussion section, we employed Large Language Model (LLM) for stylistic suggestions.
Research questions
Considering the limitations observed in previous literature reviews, we have developed three research questions for further investigation:
What are the key advantages of incorporating AI chatbots in education from the viewpoint of students?
What are the key advantages of integrating AI chatbots in education from the viewpoint of educators?
What are the main concerns raised by scholars regarding the integration of AI chatbots in education?
Exploring the literature that focuses on these research questions, with specific attention to contemporary AI-powered chatbots, can provide a deeper understanding of the impact, effectiveness, and potential limitations of chatbot technology in education while guiding its future development and implementation. This paper will help to better understand how educational chatbots can be effectively utilized to enhance education and address the specific needs and challenges of students and educators.
Search process
The search for the relevant literature was conducted in the following databases: ACM Digital Library, Scopus, IEEE Xplore, and Google Scholar. The search string was created using Boolean operators, and it was structured as follows: (“Education” or “Learning” or “Teaching”) and (“Chatbot” or “Artificial intelligence” or “AI” or “ChatGPT”). Initially, the search yielded a total of 563 papers from all four databases. Search filters were applied based on predefined inclusion and exclusion criteria, followed by a rigorous data extraction strategy as explained below.
Inclusion and exclusion criteria
In our review process, we carefully adhered to the inclusion and exclusion criteria specified in Table 2 . Criteria were determined to ensure the studies chosen are relevant to the research question (content, timeline) and maintain a certain level of quality (literature type) and consistency (language, subject area).
Data extraction strategy
All three authors collaborated to select the articles, ensuring consistency and reliability. Each article was reviewed by at least two co-authors. The article selection process involved the following stages: Initially, the authors reviewed the studies' metadata, titles, abstracts, keywords and eliminated articles that were not relevant to research questions. This reduced the number of studies to 139. Next, the authors evaluated the quality of the studies by assessing research methodology, sample size, research design, and clarity of objectives, further refining the selection to 85 articles. Finally, the authors thoroughly read the entire content of the articles. Studies offering limited empirical evidence related to our research questions were excluded. This final step reduced the number of papers to 67. Figure 1 presents the article selection process.

Flow diagram of selecting studies
In this section, we present the results of the reviewed articles, focusing on our research questions, particularly with regard to ChatGPT. ChatGPT, as one of the latest AI-powered chatbots, has gained significant attention for its potential applications in education. Within just eight months of its launch in 2022, it has already amassed over 100 million users, setting new records for user and traffic growth. ChatGPT stands out among AI-powered chatbots used in education due to its advanced natural language processing capabilities and sophisticated language generation, enabling more natural and human-like conversations. It excels at capturing and retaining contextual information throughout interactions, leading to more coherent and contextually relevant conversations. Unlike some educational chatbots that follow predetermined paths or rely on predefined scripts, ChatGPT is capable of engaging in open-ended dialogue and adapting to various user inputs. Its adaptability allows it to write articles, stories, and poems, provide summaries, accommodate different perspectives, and even write and debug computer code, making it a valuable tool in educational settings (Baidoo-Anu & Owusu Ansah, 2023 ; Tate et al., 2023 ; Williams, 2023 ).
Advantages for students
Research question 1. what are the key advantages of incorporating ai chatbots in education from the viewpoint of students.
The integration of chatbots and virtual assistants into educational settings has the potential to transform support services, improve accessibility, and contribute to more efficient and effective learning environments (Chen et al., 2023 ; Essel et al., 2022 ). AI tools have the potential to improve student success and engagement, particularly among those from disadvantaged backgrounds (Sullivan et al., 2023 ). However, the existing literature highlights an important gap in the discussion from a student’s standpoint. A few existing research studies addressing the student’s perspective of using ChatGPT in the learning process indicate that students have a positive view of ChatGPT, appreciate its capabilities, and find it helpful for their studies and work (Kasneci et al., 2023 ; Shoufan, 2023 ). Students acknowledge that ChatGPT's answers are not always accurate and emphasize the need for solid background knowledge to utilize it effectively, recognizing that it cannot replace human intelligence (Shoufan, 2023 ). Common most important benefits identified by scholars are:
Homework and Study Assistance. AI-powered chatbots can provide detailed feedback on student assignments, highlighting areas of improvement and offering suggestions for further learning (Celik et al., 2022 ). For example, ChatGPT can act as a helpful study companion, providing explanations and clarifications on various subjects. It can assist with homework questions, offering step-by-step solutions and guiding students through complex problems (Crawford et al., 2023 ; Fauzi et al., 2023 ; Lo, 2023 ; Qadir, 2023 ; Shidiq, 2023 ). According to Sedaghat ( 2023 ) experiment, ChatGPT performed similarly to third-year medical students on medical exams, and could write quite impressive essays. Students can also use ChatGPT to quiz themselves on various subjects, reinforcing their knowledge and preparing for exams (Choi et al., 2023 ; Eysenbach, 2023 ; Sevgi et al., 2023 ; Thurzo et al., 2023 ).
Flexible personalized learning. AI-powered chatbots in general are now able to provide individualized guidance and feedback to students, helping them navigate through challenging concepts and improve their understanding. These systems can adapt their teaching strategies to suit each student's unique needs (Fariani et al., 2023 ; Kikalishvili, 2023 ; Schiff, 2021 ). Students can access ChatGPT anytime, making it convenient. According to Kasneci et al. ( 2023 ), ChatGPT's interactive and conversational nature can enhance students' engagement and motivation, making learning more enjoyable and personalized. (Khan et al., 2023 ) examine the impact of ChatGPT on medical education and clinical management, highlighting its ability to offer students tailored learning opportunities.
Skills development. It can aid in the enhancement of writing skills (by offering suggestions for syntactic and grammatical corrections) (Kaharuddin, 2021 ), foster problem-solving abilities (by providing step-by-step solutions) (Benvenuti et al., 2023 ), and facilitate group discussions and debates (by furnishing discussion structures and providing real-time feedback) (Ruthotto et al., 2020 ; Wang et al., 2020 ).
It's important to note that some papers raise concerns about excessive reliance on AI-generated information, potentially leading to a negative impact on student’s critical thinking and problem-solving skills (Kasneci et al., 2023 ). For instance, if students consistently receive solutions or information effortlessly through AI assistance, they might not engage deeply in understanding the topic.
Advantages for educators
Research question 2. what are the key advantages of integrating ai chatbots in education from the viewpoint of educators.
With the current capabilities of AI and its future potential, AI-powered chatbots, like ChatGPT, can have a significant impact on existing instructional practices. Major benefits from educators’ viewpoint identified in the literature are:
Time-Saving Assistance. AI chatbot administrative support capabilities can help educators save time on routine tasks, including scheduling, grading, and providing information to students, allowing them to allocate more time for instructional planning and student engagement. For example, ChatGPT can successfully generate various types of questions and answer keys in different disciplines. However, educators should exercise critical evaluation and customization to suit their unique teaching contexts. The expertise, experience, and comprehension of the teacher are essential in making informed pedagogical choices, as AI is not yet capable of replacing the role of a science teacher (Cooper, 2023 ).
Improved pedagogy. Educators can leverage AI chatbots to augment their instruction and provide personalized support. According to Herft ( 2023 ), there are various ways in which teachers can utilize ChatGPT to enhance their pedagogical approaches and assessment methods. For instance, Educators can leverage the capabilities of ChatGPT to generate open-ended question prompts that align precisely with the targeted learning objectives and success criteria of the instructional unit. By doing so, teachers can tailor educational content to cater to the distinct needs, interests, and learning preferences of each student, offering personalized learning materials and activities (Al Ka’bi, 2023 ; Fariani et al., 2023 ).
Concerns raised by scholars
Research question 3. what are the main concerns raised by scholars regarding the integration of ai chatbots in education.
Scholars' opinions on using AI in this regard are varied and diverse. Some see AI chatbots as the future of teaching and learning, while others perceive them as a potential threat. The main arguments of skeptical scholars are threefold:
Reliability and Accuracy. AI chatbots may provide biased responses or non-accurate information (Kasneci et al., 2023 ; Sedaghat, 2023 ). If the chatbot provides incorrect information or guidance, it could mislead students and hinder their learning progress. According to Sevgi et al. ( 2023 ), although ChatGPT exhibited captivating and thought-provoking answers, it should not be regarded as a reliable information source. This point is especially important for medical education. Within the field of medical education, it is crucial to guarantee the reliability and accuracy of the information chatbots provide (Khan et al., 2023 ). If the training data used to develop an AI chatbot contains biases, the chatbot may inadvertently reproduce those biases in its responses, potentially including skewed perspectives, stereotypes, discriminatory language, or biased recommendations. This is of particular concern in an educational context.
Fair assessments. One of the challenges that educators face with the integration of Chatbots in education is the difficulty in assessing students' work, particularly when it comes to written assignments or responses. AI-generated text detection, while continually improving, is not yet foolproof and can produce false negatives or positives. This creates uncertainty and can undermine the credibility of the assessment process. Educators may struggle to discern whether the responses are genuinely student-generated or if they have been provided by an AI, affecting the accuracy of grading and feedback. This raises concerns about academic integrity and fair assessment practices (AlAfnan et al., 2023 ; Kung et al., 2023 ).
Ethical issues. The integration of AI chatbots in education raises several ethical implications, particularly concerning data privacy, security, and responsible AI use. As AI chatbots interact with students and gather data during conversations, necessitating the establishment of clear guidelines and safeguards. For example, medical education frequently encompasses the acquisition of knowledge pertaining to delicate and intimate subjects, including patient confidentiality and ethical considerations within the medical field and thus ethical and proper utilization of chatbots holds significant importance (Masters, 2023 ; Miao & Ahn, 2023 ; Sedaghat, 2023 ; Thurzo et al., 2023 ).
For these and other geopolitical reasons, ChatGPT is banned in countries with strict internet censorship policies, like North Korea, Iran, Syria, Russia, and China. Several nations prohibited the usage of the application due to privacy apprehensions. Meanwhile, North Korea, China, and Russia, in particular, contended that the U.S. might employ ChatGPT for disseminating misinformation. Conversely, OpenAI restricts access to ChatGPT in certain countries, such as Afghanistan and Iran, citing geopolitical constraints, legal considerations, data protection regulations, and internet accessibility as the basis for this decision. Italy became the first Western country to ban ChatGPT (Browne, 2023 ) after the country’s data protection authority called on OpenAI to stop processing Italian residents’ data. They claimed that ChatGPT did not comply with the European General Data Protection Regulation. However, after OpenAI clarified the data privacy issues with Italian data protection authority, ChatGPT returned to Italy. To avoid cheating on school homework and assignments, ChatGPT was also blocked in all New York school devices and networks so that students and teachers could no longer access it (Elsen-Rooney, 2023 ; Li et al., 2023 ). These examples highlight the lack of readiness to embrace recently developed AI tools. There are numerous concerns that must be addressed in order to gain broader acceptance and understanding.
To summarize, incorporating AI chatbots in education brings personalized learning for students and time efficiency for educators. Students benefit from flexible study aid and skill development. However, concerns arise regarding the accuracy of information, fair assessment practices, and ethical considerations. Striking a balance between these advantages and concerns is crucial for responsible integration in education.
The integration of artificial intelligence (AI) chatbots in education has the potential to revolutionize how students learn and interact with information. One significant advantage of AI chatbots in education is their ability to provide personalized and engaging learning experiences. By tailoring their interactions to individual students’ needs and preferences, chatbots offer customized feedback and instructional support, ultimately enhancing student engagement and information retention. However, there are potential difficulties in fully replicating the human educator experience with chatbots. While they can provide customized instruction, chatbots may not match human instructors' emotional support and mentorship. Understanding the importance of human engagement and expertise in education is crucial. A teacher's role encompasses more than just sharing knowledge. They offer students guidance, motivation, and emotional support—elements that AI cannot completely replicate.
We find that AI chatbots may benefit students as well as educators in various ways, however, there are significant concerns that need to be addressed in order to harness its capabilities effectively. Specifically, educational institutions should implement preventative measures. This includes (a) creating awareness among students, focusing on topics such as digital inequality, the reliability and accuracy of AI chatbots, and associated ethical considerations; and (b) offering regular professional development training for educators. This training should initially focus on enabling educators to integrate diverse in-class activities and assignments into the curriculum, aimed at nurturing students’ critical thinking and problem-solving skills while ensuring fair performance evaluation. Additionally, this training should cover educating educators about the capabilities and potential educational uses of AI chatbots, along with providing them with best practices for effectively integrating these tools into their teaching methods.
As technology continues to advance, AI-powered educational chatbots are expected to become more sophisticated, providing accurate information and offering even more individualized and engaging learning experiences. They are anticipated to engage with humans using voice recognition, comprehend human emotions, and navigate social interactions. Consequently, their potential impact on future education is substantial. This includes activities such as establishing educational objectives, developing teaching methods and curricula, and conducting assessments (Latif et al., 2023 ). Considering Microsoft's extensive integration efforts of ChatGPT into its products (Rudolph et al., 2023 ; Warren, 2023 ), it is likely that ChatGPT will become widespread soon. Educational institutions may need to rapidly adapt their policies and practices to guide and support students in using educational chatbots safely and constructively manner (Baidoo-Anu & Owusu Ansah, 2023 ). Educators and researchers must continue to explore the potential benefits and limitations of this technology to fully realize its potential.
The widespread adoption of chatbots and their increasing accessibility has sparked contrasting reactions across different sectors, leading to considerable confusion in the field of education. Among educators and learners, there is a notable trend—while learners are excited about chatbot integration, educators’ perceptions are particularly critical. However, this situation presents a unique opportunity, accompanied by unprecedented challenges. Consequently, it has prompted a significant surge in research, aiming to explore the impact of chatbots on education.
In this article, we present a systematic review of the latest literature with the objective of identifying the potential advantages and challenges associated with integrating chatbots in education. Through this review, we have been able to highlight critical gaps in the existing research that warrant further in-depth investigation. Addressing these gaps will be instrumental in optimizing the implementation of chatbots and harnessing their full potential in the educational landscape, thereby benefiting both educators and students alike. Further research will play a vital role in comprehending the long-term impact, variations based on student characteristics, pedagogical strategies, and the user experience associated with integrating chatbots in education.
From the viewpoint of educators, integrating AI chatbots in education brings significant advantages. AI chatbots provide time-saving assistance by handling routine administrative tasks such as scheduling, grading, and providing information to students, allowing educators to focus more on instructional planning and student engagement. Educators can improve their pedagogy by leveraging AI chatbots to augment their instruction and offer personalized support to students. By customizing educational content and generating prompts for open-ended questions aligned with specific learning objectives, teachers can cater to individual student needs and enhance the learning experience. Additionally, educators can use AI chatbots to create tailored learning materials and activities to accommodate students' unique interests and learning styles.
Incorporating AI chatbots in education offers several key advantages from students' perspectives. AI-powered chatbots provide valuable homework and study assistance by offering detailed feedback on assignments, guiding students through complex problems, and providing step-by-step solutions. They also act as study companions, offering explanations and clarifications on various subjects. They can be used for self-quizzing to reinforce knowledge and prepare for exams. Furthermore, these chatbots facilitate flexible personalized learning, tailoring their teaching strategies to suit each student's unique needs. Their interactive and conversational nature enhances student engagement and motivation, making learning more enjoyable and personalized. Also, AI chatbots contribute to skills development by suggesting syntactic and grammatical corrections to enhance writing skills, providing problem-solving guidance, and facilitating group discussions and debates with real-time feedback. Overall, students appreciate the capabilities of AI chatbots and find them helpful for their studies and skill development, recognizing that they complement human intelligence rather than replace it.
The presence of AI chatbots also brought lots of skepticism among scholars. While some see transformative potential, concerns loom over reliability, accuracy, fair assessments, and ethical dilemmas. The fear of misinformation compromised academic integrity, and data privacy issues cast an eerie shadow over the implementation of AI chatbots. Based on the findings of the reviewed papers, it is commonly concluded that addressing some of the challenges related to the use of AI chatbots in education can be accomplished by introducing preventative measures. More specifically, educational institutions must prioritize creating awareness among students about the risks associated with AI chatbots, focusing on essential aspects like digital inequality and ethical considerations. Simultaneously, investing in the continuous development of educators through targeted training is key. Empowering educators to effectively integrate AI chatbots into their teaching methods, fostering critical thinking and fair evaluation, will pave the way for a more effective and engaging educational experience.
The implications of the research findings for policymakers and researchers are extensive, shaping the future integration of chatbots in education. The findings emphasize the need to establish guidelines and regulations ensuring the ethical development and deployment of AI chatbots in education. Policies should specifically focus on data privacy, accuracy, and transparency to mitigate potential risks and build trust within the educational community. Additionally, investing in research and development to enhance AI chatbot capabilities and address identified concerns is crucial for a seamless integration into educational systems. Researchers are strongly encouraged to fill the identified research gaps through rigorous studies that delve deeper into the impact of chatbots on education. Exploring the long-term effects, optimal integration strategies, and addressing ethical considerations should take the forefront in research initiatives.
Availability of data and materials
The data and materials used in this paper are available upon request. The comprehensive list of included studies, along with relevant data extracted from these studies, is available from the corresponding author upon request.
Change history
15 april 2024.
A Correction to this paper has been published: https://doi.org/10.1186/s41239-024-00461-6
Al Ka’bi, A. (2023). Proposed artificial intelligence algorithm and deep learning techniques for development of higher education. International Journal of Intelligent Networks, 4 , 68–73.
Article Google Scholar
AlAfnan, M. A., Dishari, S., Jovic, M., & Lomidze, K. (2023). Chatgpt as an educational tool: Opportunities, challenges, and recommendations for communication, business writing, and composition courses. Journal of Artificial Intelligence and Technology, 3 (2), 60–68.
Google Scholar
Alsanousi, B., Albesher, A. S., Do, H., & Ludi, S. (2023). Investigating the user experience and evaluating usability issues in ai-enabled learning mobile apps: An analysis of user reviews. International Journal of Advanced Computer Science and Applications , 14(6).
AlZubi, S., Mughaid, A., Quiam, F., & Hendawi, S. (2022). Exploring the Capabilities and Limitations of ChatGPT and Alternative Big Language Models. Artificial Intelligence and Applications .
Aron, J. (2011). How innovative is Apple’s new voice assistant. Siri, NewScientist , 212 (2836), 24
Baidoo-Anu, D., & Owusu Ansah, L. (2023). Education in the era of generative artificial intelligence (AI): Understanding the potential benefits of ChatGPT in promoting teaching and learning. Available at SSRN 4337484 .
Benvenuti, M., Cangelosi, A., Weinberger, A., Mazzoni, E., Benassi, M., Barbaresi, M., & Orsoni, M. (2023). Artificial intelligence and human behavioral development: A perspective on new skills and competencies acquisition for the educational context. Computers in Human Behavior, 148 , 107903.
Browne, R. (2023). Italy became the first Western country to ban ChatGPT. Here’s what other countries are doing . CNBC (Apr. 4, 2023).
Celik, I., Dindar, M., Muukkonen, H., & Järvelä, S. (2022). The promises and challenges of artificial intelligence for teachers: A systematic review of research. TechTrends, 66 (4), 616–630.
Chassignol, M., Khoroshavin, A., Klimova, A., & Bilyatdinova, A. (2018). Artificial Intelligence trends in education: A narrative overview. Procedia Computer Science, 136 , 16–24.
Chen, L., Chen, P., & Lin, Z. (2020). Artificial intelligence in education: A review. IEEE Access, 8 , 75264–75278.
Chen, Y., Jensen, S., Albert, L. J., Gupta, S., & Lee, T. (2023). Artificial intelligence (AI) student assistants in the classroom: Designing chatbots to support student success. Information Systems Frontiers, 25 (1), 161–182.
Choi, J. H., Hickman, K. E., Monahan, A., & Schwarcz, D. (2023). Chatgpt goes to law school. Available at SSRN .
Colby, K. M. (1981). PARRYing. Behavioral and Brain Sciences, 4 (4), 550–560.
Cooper, G. (2023). Examining science education in chatgpt: An exploratory study of generative artificial intelligence. Journal of Science Education and Technology, 32 (3), 444–452.
Crawford, J., Cowling, M., & Allen, K.-A. (2023). Leadership is needed for ethical ChatGPT: Character, assessment, and learning using artificial intelligence (AI). Journal of University Teaching and Learning Practice, 20 (3), 02.
Crompton, H., & Burke, D. (2023). Artificial intelligence in higher education: The state of the field. International Journal of Educational Technology in Higher Education, 20 (1), 1–22.
Deng, X., & Yu, Z. (2023). A meta-analysis and systematic review of the effect of chatbot technology use in sustainable education. Sustainability, 15 (4), 2940.
Dergaa, I., Chamari, K., Zmijewski, P., & Saad, H. B. (2023). From human writing to artificial intelligence generated text: Examining the prospects and potential threats of ChatGPT in academic writing. Biology of Sport, 40 (2), 615–622.
Devedzic, V. (2004). Web intelligence and artificial intelligence in education. Journal of Educational Technology and Society, 7 (4), 29–39.
Dinh, T. N., & Thai, M. T. (2018). AI and blockchain: A disruptive integration. Computer, 51 (9), 48–53.
Elsen-Rooney, M. (2023). NYC education department blocks ChatGPT on school devices, networks. Retrieved Jan , 25 , 2023.
Essel, H. B., Vlachopoulos, D., Tachie-Menson, A., Johnson, E. E., & Baah, P. K. (2022). The impact of a virtual teaching assistant (chatbot) on students’ learning in Ghanaian higher education. International Journal of Educational Technology in Higher Education, 19 (1), 1–19.
Eysenbach, G. (2023). The role of ChatGPT, generative language models, and artificial intelligence in medical education: A conversation with ChatGPT and a call for papers. JMIR Medical Education, 9 (1), e46885.
Fariani, R. I., Junus, K., & Santoso, H. B. (2023). A systematic literature review on personalised learning in the higher education context. Technology, Knowledge and Learning, 28 (2), 449–476.
Fauzi, F., Tuhuteru, L., Sampe, F., Ausat, A. M. A., & Hatta, H. R. (2023). Analysing the role of ChatGPT in improving student productivity in higher education. Journal on Education, 5 (4), 14886–14891.
Herft, A. (2023). A Teacher’s Prompt Guide to ChatGPT aligned with’What Works Best’ .
Hoffer, R., Kay, T., Levitan, P., & Klein, S. (2001). Smarterchild . ActiveBuddy.
Holotescu, C. (2016). MOOCBuddy: A Chatbot for personalized learning with MOOCs. RoCHI , 91–94.
Kabiljo, M., Vidas-Bubanja, M., Matic, R., & Zivkovic, M. (2020). Education system in the republic of serbia under COVID-19 conditions: Chatbot-acadimic digital assistant of the belgrade business and arts academy of applied studies. Knowledge-International Journal, 43 (1), 25–30.
Kaharuddin, A. (2021). Assessing the effect of using artificial intelligence on the writing skill of Indonesian learners of English. Linguistics and Culture Review, 5 (1), 288.
Kahraman, H. T., Sagiroglu, S., & Colak, I. (2010). Development of adaptive and intelligent web-based educational systems. In 2010 4th International Conference on Application of Information and Communication Technologies , 1–5.
Kasneci, E., Seßler, K., Küchemann, S., Bannert, M., Dementieva, D., Fischer, F., Gasser, U., Groh, G., Günnemann, S., & Hüllermeier, E. (2023). ChatGPT for good? On opportunities and challenges of large language models for education. Learning and Individual Differences, 103 , 102274.
Khademi, A. (2023). Can ChatGPT and bard generate aligned assessment items? A reliability analysis against human performance. ArXiv Preprint ArXiv:2304.05372.
Khan, R. A., Jawaid, M., Khan, A. R., & Sajjad, M. (2023). ChatGPT-Reshaping medical education and clinical management. Pakistan Journal of Medical Sciences, 39 (2), 605.
Kietzmann, J., Paschen, J., & Treen, E. (2018). Artificial intelligence in advertising: How marketers can leverage artificial intelligence along the consumer journey. Journal of Advertising Research, 58 (3), 263–267.
Kikalishvili, S. (2023). Unlocking the potential of GPT-3 in education: Opportunities, limitations, and recommendations for effective integration. Interactive Learning Environments , 1–13.
Konecki, M., Konecki, M., & Biškupić, I. (2023). Using artificial intelligence in higher education. In Proceedings of the 15th International Conference on Computer Supported Education .
Krstić, L., Aleksić, V., & Krstić, M. (2022). Artificial intelligence in education: A review .
Kuhail, M. A., Alturki, N., Alramlawi, S., & Alhejori, K. (2023). Interacting with educational chatbots: A systematic review. Education and Information Technologies, 28 (1), 973–1018.
Kung, T. H., Cheatham, M., Medenilla, A., Sillos, C., De Leon, L., Elepaño, C., et al. (2023). Performance of ChatGPT on USMLE: Potential for AI-assisted medical education using large language models. PLoS Digital Health, 2 (2), e0000198.
Lally, A., & Fodor, P. (2011). Natural language processing with prolog in the ibm watson system. The Association for Logic Programming (ALP) Newsletter , 9 , 2011.
Latif, E., Mai, G., Nyaaba, M., Wu, X., Liu, N., Lu, G., ... & Zhai, X. (2023). Artificial general intelligence (AGI) for education. arXiv preprint arXiv:2304.12479.
Li, L., Ma, Z., Fan, L., Lee, S., Yu, H., & Hemphill, L. (2023). ChatGPT in education: A discourse analysis of worries and concerns on social media. ArXiv Preprint ArXiv:2305.02201.
Lo, C. K. (2023). What is the impact of ChatGPT on education? A rapid review of the literature. Education Sciences, 13 (4), 410.
Masters, K. (2023). Ethical use of artificial intelligence in health professions education: AMEE Guide No. 158. Medical Teacher , 45 (6), 574–584.
Miao, H., & Ahn, H. (2023). Impact of ChatGPT on interdisciplinary nursing education and research. Asian/pacific Island Nursing Journal, 7 (1), e48136.
Moppel, J. (2018). Socratic chatbot . University Of Tartu, Institute of Computer Science, Bachelor’s Thesis.
Okonkwo, C. W., & Ade-Ibijola, A. (2021). Chatbots applications in education: A systematic review. Computers and Education: Artificial Intelligence, 2 , 100033.
Pentina, I., Hancock, T., & Xie, T. (2023). Exploring relationship development with social chatbots: A mixed-method study of replika. Computers in Human Behavior, 140 , 107600.
Peredo, R., Canales, A., Menchaca, A., & Peredo, I. (2011). Intelligent Web-based education system for adaptive learning. Expert Systems with Applications, 38 (12), 14690–14702.
Pérez, J. Q., Daradoumis, T., & Puig, J. M. M. (2020). Rediscovering the use of chatbots in education: A systematic literature review. Computer Applications in Engineering Education, 28 (6), 1549–1565.
Qadir, J. (2023). Engineering education in the era of ChatGPT: Promise and pitfalls of generative AI for education. IEEE Global Engineering Education Conference (EDUCON), 2023 , 1–9.
Rahaman, M. S., Ahsan, M. M., Anjum, N., Rahman, M. M., & Rahman, M. N. (2023). The AI race is on! Google’s Bard and OpenAI’s ChatGPT head to head: An opinion article. Mizanur and Rahman, Md Nafizur, The AI Race Is On .
Rudolph, J., Tan, S., & Tan, S. (2023). War of the chatbots: Bard, Bing Chat, ChatGPT, Ernie and beyond. The new AI gold rush and its impact on higher education. Journal of Applied Learning and Teaching, 6 (1).
Ruthotto, I., Kreth, Q., Stevens, J., Trively, C., & Melkers, J. (2020). Lurking and participation in the virtual classroom: The effects of gender, race, and age among graduate students in computer science. Computers & Education, 151 , 103854.
de Sales, A. B., & Antunes, J. G. (2021). Evaluation of educational games usage satisfaction. 2021 16th Iberian Conference on Information Systems and Technologies (CISTI) , 1–6.
Schiff, D. (2021). Out of the laboratory and into the classroom: the future of artificial intelligence in education. AI & Society, 36 (1), 331–348.
Sedaghat, S. (2023). Success through simplicity: What other artificial intelligence applications in medicine should learn from history and ChatGPT. Annals of Biomedical Engineering , 1–2.
Sevgi, U. T., Erol, G., Doğruel, Y., Sönmez, O. F., Tubbs, R. S., & Güngor, A. (2023). The role of an open artificial intelligence platform in modern neurosurgical education: A preliminary study. Neurosurgical Review, 46 (1), 86.
Shidiq, M. (2023). The use of artificial intelligence-based chat-gpt and its challenges for the world of education; from the viewpoint of the development of creative writing skills. Proceeding of International Conference on Education, Society and Humanity, 1 (1), 353–357.
Shoufan, A. (2023). Exploring Students’ Perceptions of CHATGPT: Thematic Analysis and Follow-Up Survey. IEEE Access .
St-Hilaire, F., Vu, D. D., Frau, A., Burns, N., Faraji, F., Potochny, J., Robert, S., Roussel, A., Zheng, S., & Glazier, T. (2022). A new era: Intelligent tutoring systems will transform online learning for millions. ArXiv Preprint ArXiv:2203.03724.
Sullivan, M., Kelly, A., & McLaughlan, P. (2023). ChatGPT in higher education: Considerations for academic integrity and student learning .
Tahiru, F. (2021). AI in education: A systematic literature review. Journal of Cases on Information Technology (JCIT), 23 (1), 1–20.
Tate, T., Doroudi, S., Ritchie, D., & Xu, Y. (2023). Educational research and AI-generated writing: Confronting the coming tsunami .
Thurzo, A., Strunga, M., Urban, R., Surovková, J., & Afrashtehfar, K. I. (2023). Impact of artificial intelligence on dental education: A review and guide for curriculum update. Education Sciences, 13 (2), 150.
Wallace, R. (1995). Artificial linguistic internet computer entity (alice). City .
Wang, Q., Jing, S., Camacho, I., Joyner, D., & Goel, A. (2020). Jill Watson SA: Design and evaluation of a virtual agent to build communities among online learners. Extended Abstracts of the 2020 CHI Conference on Human Factors in Computing Systems , 1–8.
Warren, T. (2023). Microsoft is looking at OpenAI’s GPT for Word, Outlook, and PowerPoint. The Verge .
Weizenbaum, J. (1966). ELIZA—A computer program for the study of natural language communication between man and machine. Communications of the ACM, 9 (1), 36–45.
Williams, C. (2023). Hype, or the future of learning and teaching? 3 Limits to AI’s ability to write student essays .
Wollny, S., Schneider, J., Di Mitri, D., Weidlich, J., Rittberger, M., & Drachsler, H. (2021). Are we there yet?—A systematic literature review on chatbots in education. Frontiers in Artificial Intelligence, 4 , 654924.
Xie, T., & Pentina, I. (2022). Attachment theory as a framework to understand relationships with social chatbots: A case study of Replika .
Zhang, Q. (2023). Investigating the effects of gamification and ludicization on learning achievement and motivation: An empirical study employing Kahoot! and Habitica. International Journal of Technology-Enhanced Education (IJTEE), 2 (1), 1–19.
Download references
Acknowledgements
Not applicable.
The authors declare that this research paper did not receive any funding from external organizations. The study was conducted independently and without financial support from any source. The authors have no financial interests or affiliations that could have influenced the design, execution, analysis, or reporting of the research.
Author information
Authors and affiliations.
Finance Department, American University of the Middle East, Block 6, Building 1, Egaila, Kuwait
Lasha Labadze
Statistics Department, American University of the Middle East, Block 6, Building 1, Egaila, Kuwait
Maya Grigolia
Caucasus School of Business, Caucasus University, 1 Paata Saakadze St, 0102, Tbilisi, Georgia
Lela Machaidze
You can also search for this author in PubMed Google Scholar
Contributions
LL provided a concise overview of the existing literature and formulated the methodology. MG initiated the initial search process. LM authored the discussion section. All three authors collaborated on the selection of the final paper collection and contributed to crafting the conclusion. The final version of the paper received approval from all authors.
Corresponding author
Correspondence to Lasha Labadze .
Ethics declarations
Competing interests.
Authors have no competing interests to declare.
Additional information
Publisher's note.
Springer Nature remains neutral with regard to jurisdictional claims in published maps and institutional affiliations.
The original version of this article was revised: “A sentence has been added to the Methodology section of the article to acknowledge use of LLM”
Rights and permissions
Open Access This article is licensed under a Creative Commons Attribution 4.0 International License, which permits use, sharing, adaptation, distribution and reproduction in any medium or format, as long as you give appropriate credit to the original author(s) and the source, provide a link to the Creative Commons licence, and indicate if changes were made. The images or other third party material in this article are included in the article's Creative Commons licence, unless indicated otherwise in a credit line to the material. If material is not included in the article's Creative Commons licence and your intended use is not permitted by statutory regulation or exceeds the permitted use, you will need to obtain permission directly from the copyright holder. To view a copy of this licence, visit http://creativecommons.org/licenses/by/4.0/ .
Reprints and permissions
About this article
Cite this article.
Labadze, L., Grigolia, M. & Machaidze, L. Role of AI chatbots in education: systematic literature review. Int J Educ Technol High Educ 20 , 56 (2023). https://doi.org/10.1186/s41239-023-00426-1
Download citation
Received : 22 August 2023
Accepted : 18 October 2023
Published : 31 October 2023
DOI : https://doi.org/10.1186/s41239-023-00426-1
Share this article
Anyone you share the following link with will be able to read this content:
Sorry, a shareable link is not currently available for this article.
Provided by the Springer Nature SharedIt content-sharing initiative
- Systematic literature review
- Artificial intelligence
- AI chatbots
- Chatbots in education
Understanding user intent modeling for conversational recommender systems: a systematic literature review
- Open access
- Published: 06 June 2024
Cite this article
You have full access to this open access article
- Siamak Farshidi 1 , 2 ,
- Kiyan Rezaee 3 na1 ,
- Sara Mazaheri 3 na1 ,
- Amir Hossein Rahimi 3 na1 ,
- Ali Dadashzadeh 3 ,
- Morteza Ziabakhsh 3 ,
- Sadegh Eskandari 3 na1 &
- Slinger Jansen 1 , 4
User intent modeling in natural language processing deciphers user requests to allow for personalized responses. The substantial volume of research (exceeding 13,000 publications in the last decade) underscores the significance of understanding prevalent models in AI systems, with a focus on conversational recommender systems. We conducted a systematic literature review to identify models frequently employed for intent modeling in conversational recommender systems. From the collected data, we developed a decision model to assist researchers in selecting the most suitable models for their systems. Furthermore, we conducted two case studies to assess the utility of our proposed decision model in guiding research modelers in selecting user intent modeling models for developing their conversational recommender systems. Our study analyzed 59 distinct models and identified 74 commonly used features. We provided insights into potential model combinations, trends in model selection, quality concerns, evaluation measures, and frequently used datasets for training and evaluating these models. The study offers practical insights into the domain of user intent modeling, specifically enhancing the development of conversational recommender systems. The introduced decision model provides a structured framework, enabling researchers to navigate the selection of the most apt intent modeling methods for conversational recommender systems.
Avoid common mistakes on your manuscript.
1 Introduction
User intent modeling is a fundamental process within natural language processing models, with the primary aim of discerning a user’s underlying purpose or objective (Carmel et al. 2020 ). The comprehension and prediction of user goals and motivations through user intent modeling hold great significance in optimizing search engines and recommender systems (Zhang et al. 2019 ). This alignment of the user experience with preferences and needs contributes to enhanced user satisfaction and engagement (Oulasvirta and Blom 2008 ). Notably, state-of-the-art models such as ChatGPT have generated substantial interest for their potential in search engines and recommender systems (Cao et al. 2023 ), as they exhibit the capability to understand user intentions and engage in meaningful interactions.
The realm of user intent modeling finds extensive applications across diverse domains, spanning e-commerce, healthcare, education, and social media. In e-commerce, it plays a pivotal role in providing personalized product recommendations and detecting fraudulent product reviews (Tanjim et al. 2020 ; Wang et al. 2020 ; Guo et al. 2020 ; Paul and Nikolaev 2021 ). The healthcare sector leverages user intent modeling for delivering personalized health recommendations and interventions (Zhang et al. 2016 ; Wang et al. 2022 ). Similarly, within the educational sphere, it contributes to the tailoring of learning experiences to individual student goals and preferences (Liu et al. 2021 ; Bhaskaran and Santhi 2019 ). Furthermore, user intent modeling proves invaluable in comprehending user interests, preferences, and behaviors on social media, driving personalized content and targeted advertising delivery (Ding et al. 2015 ; Wang et al. 2019 ). Additionally, it plays a pivotal role in virtual assistants by aiding in the understanding of user queries and the provision of relevant responses (Penha and Hauff 2020 ; Hashemi et al. 2018 ).
User intent modeling approaches generally encompass a blend of models, including machine learning algorithms, to analyze various aspects of user input, such as words, phrases, and context. This approach enables the delivery of personalized responses within conversational recommender systems (Khilji et al. 2023 ). As a result, the domain of user intent modeling encompasses a diverse array of machine learning models, comprising Support Vector Machine (SVM) (Xia et al. 2018 ; Hu et al. 2017 ), Latent Dirichlet Allocation (LDA) (Chen et al. 2013 ; Weismayer and Pezenka 2017 ), Naive Bayes (Hu et al. 2018 ; Gu et al. 2016 ), as well as deep learning models like Bidirectional Encoder Representations from Transformers (BERT) (Yao et al. 2022 ), Word2vec(Da’u and Salim 2019 ; Ye et al. 2016 ), and Multilayer Perceptron (MLP) (Xu et al. 2022 ; Qu et al. 2016 ). A comprehensive examination of these models and their characteristics provides a holistic understanding of their advantages and limitations, thereby offering valuable insights for future research and development.
However, selecting the most suitable models within this domain presents challenges due to the multitude of available models and approaches (Zhang et al. 2019 ; Ricci et al. 2015 ). This challenge is further compounded by the absence of a clear classification scheme (Portugal et al. 2018 ), making it challenging for researchers and developers to navigate this diverse landscape and leading to uncertainties in model selection for specific requirements (Allamanis et al. 2018 ; Hill et al. 2016 ). Although user intent modeling in conversational recommender systems has been extensively explored, the research is dispersed across various sources, making it difficult to gain a cohesive understanding. The extensive array of machine learning models, concepts, datasets, and evaluation measures within this domain adds to the complexity.
To streamline and synthesize this wealth of information, we conducted a systematic literature review, adhering to established protocols set forth by Kitchenham et al. ( 2009 ), Xiao and Watson ( 2019 ), and Okoli and Schabram ( 2015 ). Furthermore, we developed a decision model derived from the literature review data, designed to assist in selecting user intent modeling methods. The utility of this decision model was evaluated through two academic case studies, structured in accordance with Yin’s guidelines (Yin 2009 ).
The study is structured as follows: we begin by defining the problem statement and research questions, followed by an outline of the employed research methods in Sect. 2 . Subsequently, we delve into the methodology of the Systematic Literature Review (SLR) in Sect. 3 , with findings and analysis presented in Sect. 4 . In Sect. 5 , we shift our focus to the practical application of the collected data through the introduced decision model. Furthermore, we present academic case studies validating our research in Sect. 6 . The outcomes, lessons learned, and implications of our findings are discussed in Sect. 7 , with a comparative analysis of related studies provided in Sect. 8 . Finally, Sect. 9 offers a summary of our contributions and outlines future research directions.
2 Research approach
This study uses a systematic research approach, combining an SLR and Case Study Research, to investigate approaches in user intent modeling for conversational recommended systems. The SLR was instrumental in collating relevant information from existing literature, and the case studies provided a means to evaluate the application of our findings.
2.1 Problem statement
Conversational recommender systems can be considered a multidisciplinary field, residing at the intersection of various domains including Human–Computer Interaction (HCI) (de Barcelos Silva et al. 2020 ; Rapp et al. 2021 ), Conversational AI (Zaib et al. 2022 ; Saka et al. 2023 ), Conversational Search Systems (Keyvan and Huang 2022 ; Yuan et al. 2020 ), User Preference Extraction & Prioritization (Pu et al. 2012 ; Liu et al. 2022 ), and Contextual Information Retrieval Systems (Tamine-Lechani et al. 2010 ; Chen et al. 2015 ). To develop an effective conversational recommender system empowered by user intent modeling, a comprehensive understanding of models and approaches recognized in other domains such as “topic modeling” (Vayansky and Kumar 2020 ), “user intent prediction” (Qu et al. 2019 ), “conversational search” (Zhang et al. 2018 ), “intent classification” (Larson et al. 2019 ), “intent mining” (Huang et al. 2018 ), “user response prediction” (Qu et al. 2016 ), “user behavior modeling” (Pi et al. 2019 ), and “concept discovery” (Sun et al. 2015 ) is essential. These concepts are discussed prominently within the context of search engines and recommender systems. This study acknowledges the evolving nature of modern search engines, increasingly incorporating conversational features, blurring the lines between traditional search engines and conversational recommender systems (Beel et al. 2016 ; Jain et al. 2015 ). Consequently, the research scope extends to encompass the analysis of search engines as conversational recommender systems, enabling exploration of how they engage with users, with a particular emphasis on accurately modeling and predicting user intent. It is essential to emphasize that developing conversational recommender systems requires a holistic understanding of key domains contributing to their efficacy and relevance. This study centers on integrating distinct yet interconnected fields pivotal for advancing conversational recommender systems, with each domain playing a unique role in shaping their design, functionality, and user engagement aspects.
An analysis of current approaches in user intent modeling reveals significant challenges, necessitating a systematic literature review and the development of a decision model:
Scattered knowledge: Concepts, models, and characteristics of intent modeling are widely dispersed in academic literature (Portugal et al. 2018 ), requiring systematic consolidation and categorization to advance conversational recommender systems.
Model integration: Combining different user intent modeling models is complex, demanding an analysis of their compatibility and synergistic potential (von Rueden et al. 2020 ).
Trends and emerging patterns: Understanding the evolving field of user intent modeling requires a comprehensive review of current trends and emerging patterns (Chen et al. 2015 ; Jordan and Mitchell 2015 ).
Assessment criteria: Selecting suitable evaluation measures for intent modeling is complex, necessitating tailored metrics for effective assessment (Telikani et al. 2021 ; Singh et al. 2016 ).
Dataset selection: Identifying appropriate datasets reflecting diverse intents and user behaviors for training and evaluating intent modeling approaches is a significant challenge (Yuan et al. 2020 ).
Decision-making framework: The absence of a decision model in current literature covering various intent modeling concepts and offering guidelines for model selection and evaluation highlights the critical need for such a model (Farshidi et al. 2020 ; Farshidi 2020 ).
These challenges form the basis of our research, leading to our systematic literature review and the development of a decision model to assist researchers in addressing these complexities in conversational recommender systems and user intent modeling.
2.2 Research questions
The research questions, formulated in response to the identified challenges, are as follows:
\(RQ_1\) : What models are commonly used in intent modeling approaches for conversational recommender systems?
\(RQ_2\) : What are the key characteristics and features supported by these models used in intent modeling?
\(RQ_3\) : What trends are observed in employing models for intent modeling in conversational recommender systems?
\(RQ_4\) : What evaluation measures and quality attributes are commonly used to assess the performance and efficacy of intent modeling approaches?
\(RQ_5\) : Which datasets are typically considered in the literature for training and evaluating machine learning models in intent modeling approaches?
\(RQ_6\) : How can a decision model be developed to assist researchers in selecting appropriate models for intent modeling approaches?
2.3 Research methods
A mixed research method was utilized to address these research questions, combining SLR and Case Study Research (Jansen 2009 ; Johnson and Onwuegbuzie 2004 ). The SLR provided an in-depth understanding of user intent modeling approaches, and the case studies evaluated the practical application of the proposed decision model.
The SLR followed guidelines by Kitchenham et al. ( 2009 ), Xiao and Watson ( 2019 ), and Okoli and Schabram ( 2015 ) to identify models, their definitions, combinations, supported features, potential evaluation measures, and relevant concepts. A decision model was developed from the SLR findings, influenced by previous studies on multi-criteria decision-making in software engineering (Farshidi 2020 ).
Two case studies were conducted to evaluate the decision model’s practicality, following Yin’s guidelines (Yin 2017 ). These studies tested whether the decision model effectively aided researchers in selecting models for their projects.
This mixed research method, encompassing SLR and case studies, provided insights and practical solutions for advancing intent modeling in conversational recommender systems.
2.4 Research methods
This mixed research method, encompassing both SLR and case studies, provided insights and practical solutions for advancing intent modeling in conversational recommender systems.
3 Systematic literature review methodology
This section outlines the review protocol employed in this study to systematically collect data from the literature on user intent modeling approaches for conversational recommender systems. The SLR review protocol, as depicted in Fig. 1 , systematically collects and analyzes data relevant to this area.
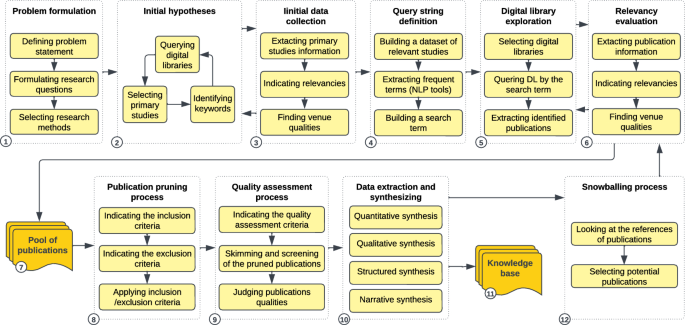
The review protocol used in this study, following the guidelines by Kitchenham et al. ( 2009 ), Xiao and Watson ( 2019 ), and Okoli and Schabram ( 2015 ). The protocol consists of 12 elements for systematically collecting and extracting data from relevant studies, ensuring rigorous investigation and adherence to scientific standards. For details on how the protocol aligns with the guidelines, see Appendix A
(1) Problem formulation: The review protocol began by defining the problem and formulating research questions, followed by identifying research methods suitable for these questions. The procedures of Xiao and Watson ( 2019 ) were followed for defining the problem statement and formulating research questions, as detailed in Sects. 2.1 and 2.2 . Analysis showed the first five research questions were suitable for exploration via an SLR. The outcomes of this SLR informed the development of a decision model. The final research question, focusing on the decision model’s development and application, was addressed through case study research.
(2) Initial hypotheses: A set of keywords was initially selected to locate primary studies relevant to the research questions. These keywords helped identify potential seed papers, marking the start of our literature review and facilitating a systematic exploration of relevant publications.
(3) Initial data collection: Primary studies’ characteristics, including source, URL, title, keywords, abstract, venue, venue quality, publication type, number of citations, publication year, and relevancy level, were manually collected. This process aided in focusing the review and establishing inclusion/exclusion criteria.
(4) Query string definition: The search query was developed by analyzing keywords, abstracts, and titles from primary studies, focusing on terms prevalent in relevant and high-quality papers. This method refined our search to include pertinent publications.
(5) Digital library exploration: Digital libraries such as ACM, ScienceDirect, and Elsevier were searched using the formulated query. This exploration ensured a thorough coverage of relevant publications.
(6) Relevancy Evaluation: Publications’ characteristics were evaluated for relevance to our research questions and challenges, confirming the inclusion of pertinent publications in our review.
(7) The pool of publications: The selected papers formed the basis of our review. This collection was expanded through the snowballing process, providing a thorough examination of the literature.
(8) Publication pruning process: Inclusion/exclusion criteria were strictly applied to the pool of publications, filtering out irrelevant content and focusing on relevant and high-quality studies.
(9) Quality assessment process: The quality of remaining publications was evaluated based on criteria like clarity of research questions and findings, ensuring the inclusion of only high-quality studies.
(10) Data extraction and synthesizing: Systematic data extraction from selected publications facilitated the identification and summarization of key information.
(11) Knowledge base: The final selection of publications formed a knowledge base, with extracted data linking findings and sources. This base serves as a resource for future research and further analysis.
(12) Snowballing process: Additional relevant papers were identified by reviewing references in selected publications, enhancing the review’s comprehensiveness.
This systematic review protocol ensured rigorous standards in collecting and analyzing literature on user intent modeling approaches, ensuring the validity and reliability of our study.
3.1 Review protocol
This section details the implementation of the review protocol, as depicted in Fig. 1 , for our SLR.
3.1.1 Pool of publications
In our systematic literature review, the manual search phase, comprising the Initial Hypothesis and Initial Data Collection stages, preceded the automatic search phase. During the manual phase, we initially collected publications and extracted keywords indicative of common terms in noteworthy high-quality papers. These keywords were subsequently used as the foundation for the automatic search phase, beginning with the Query String Definition step.
In the manual search phase, we initially gathered a set of primary studies using search terms to identify relevant publications addressing our research questions. These terms were refined based on our domain understanding, considering publication abstracts, keywords, and titles. This process led to the identification of 314 highly relevant and high-quality publications. A publication was deemed ’relevant’ if it addressed at least one of our research questions (Sect. 2.2 ). We evaluated quality based on criteria like publication venue reputation (CORE Rankings Portal Footnote 1 and Scimago Journal & Country Rank (SJR) Footnote 2 ), citation count, and recency.
Subsequently, we employed Sketch Engine (Kilgarriff et al. 2014 ), a topic modeling tool, to extract frequently mentioned keywords from these 314 primary studies. We considered keywords that appeared at least three times and used them to formulate our search query for the automatic search phase, focusing on topics related to user intent modeling in search engines and recommender systems, including intent detection, prediction, interactive modeling, conversational search, classification, and user behavior modeling. Our search query combined keywords using logical operators “AND” and “OR,” resulting in the following query:
(“user intent” OR “user intent modeling” OR “topic model” OR “user intent detection” OR “user intent prediction” OR “interactive intent modeling” OR “conversational search” OR “intent classification” OR “intent mining” OR “conversational recommender system” OR “user response prediction” OR “user behavior modeling” OR “interactive user intent” OR “intent detection” OR “concept discovery”) AND (“search engine” OR “recommender system”)
In the automatic search phase, we assessed the relevance of these papers by examining their titles, abstracts, keywords, and conclusions, classifying them as ’highly relevant’ (addressing at least three research questions), ’medium relevant’ (addressing two questions), ’low relevant’ (addressing one question), or ’irrelevant’ (not addressing any questions). After this evaluation, we excluded irrelevant publications from the pool, leaving 3,828 relevant publications out of the initial 13,168 search results.
The publications underwent rigorous screening, adhering strictly to our predefined inclusion and exclusion criteria, ensuring the selection of only the most pertinent and high-quality publications for data extraction and analysis. We assessed the effectiveness of our search query by comparing results with those from a manual search, confirming the consistency and accuracy of our approach. This evaluation verified that our query included publications identified as high-quality and highly relevant during the manual phase, affirming the successful retrieval of publications relevant to user intent modeling in search engines and recommender systems.
3.1.2 Publication pruning process
In systematic literature reviews or meta-analyses, inclusion/exclusion criteria play a pivotal role as definitive guidelines for determining study relevance and eligibility. These criteria guarantee the selection of high-quality studies that directly address the research question.
For our study, we implemented stringent inclusion and exclusion criteria to eliminate irrelevant and low-quality publications. These criteria considered several factors, including the publication venue’s quality, publication year, citation counts, and relevance to our research topic. We precisely defined and consistently applied these criteria to include only high-quality and relevant publications.
We categorized publications based on their quality using assessments from the CORE Rankings Portal and SJR:
Publications with “ A* ” or “ Q1 ” indicators were classified as “ Excellent .”
Those with “ A ” or “ Q2 ” were deemed “ Good .”
“ B ” or “ Q3 ” were categorized as “ Average .”
“ C ” or “ Q4 ” were labeled as “ Poor .”
Publications without quality indicators on these platforms were marked as “ N/A .”
Publications classified as “ Poor ” or “ N/A ” were excluded from further consideration. Additional exclusion criteria encompassed publications with low citation counts, older publication dates, or classification as Gray literature (e.g., books, theses, reports, and short papers).
After applying our predefined inclusion/exclusion criteria, we identified and selected 1067 publications from the initial pool of 3828 publications.
3.1.3 Quality assessment process
During the SLR, we assessed the quality of the selected publications after applying the inclusion/exclusion criteria. Several factors were taken into consideration to evaluate the quality and suitability of the publications for our research:
Research method: We evaluated whether the chosen research method was appropriate for addressing the research question. The clarity and transparency of the research methodology were also assessed.
Research type: We considered whether the publication presented original research, a review article, a case study, or a meta-analysis. The relevance and scope of the research in the field of machine learning were also taken into account.
Data collection method: We evaluated the appropriateness of the data collection method in relation to the research question. The adequacy and clarity of the reported data collection process were also assessed.
Evaluation method: We assessed whether the chosen evaluation method was suitable for addressing the research question. The transparency and statistical significance of the reported results were considered.
Problem statement: We evaluated whether the publication identified the research problem and provided sufficient background information. The clarity and definition of the research question were also taken into account.
Research questions: We assessed the relevance, clarity, and definition of the research questions in relation to the research problem.
Research challenges: We considered whether the publication identified and acknowledged the challenges and limitations associated with the research.
Statement of findings: We evaluated whether the publication reported the research results and whether the findings were relevant to the research problem and questions.
Real-world use cases: We assessed whether the publication provided real-world use cases or applications for the proposed method or model.
Based on these assessment factors, a team of five researchers involved in the SLR evaluated the publications’ quality. Each researcher independently assessed the publications based on the established criteria. In cases where there were discrepancies or differences in evaluating a publication’s quality, the researchers engaged in discussions to reach a consensus and ensure a consistent assessment.
Through this collaborative evaluation process, a final selection of 791 publications was made from the initial pool of 1,067 publications. These selected publications demonstrated high quality and relevance to our research question, meeting the predefined inclusion/exclusion criteria. The consensus reached by the research team ensured a rigorous and reliable selection of publications for further analysis and data extraction in the SLR.
3.1.4 Data extraction and synthesizing
During the data extraction and synthesis phase of the SLR, our primary objective was to address the identified research questions and gain insights into the foundational models commonly employed by researchers in their intent modeling approaches. We aimed to understand the features of these models, the associated quality attributes, and the evaluation measures utilized by research modelers to assess their approaches. Furthermore, we explored the potential combinations of models that researchers incorporated into their research papers.
We extracted relevant data from the papers included in our review to achieve these objectives. In our perspective, evaluation measures encompassed a range of measurements and key performance indicators (KPIs) used to evaluate the performance of the models. Quality attributes represent the characteristics of models that are not easily quantifiable and are typically assigned values using Likert scales or similar approaches. For example, authors may assess the performance of a model as high or low compared to other models. On the other hand, features encompassed any characteristics of models that authors highlighted to demonstrate specific functionalities. These features played a role in the selection of models by research modelers. Examples of features include ranking and prediction capabilities.
In this study, ’models’ are conceptualized as structured, mathematical, or computational frameworks employed for simulating, predicting, or classifying phenomena within user intent modeling in conversational recommender systems. These models are organized into a variety of categories, reflecting diverse methodologies. This includes Supervised Learning, where models are trained on labeled data for accurate predictions; Unsupervised Learning, which uncovers patterns in unlabeled data; and Collaborative Filtering, among others, each offering unique insights into user interactions. Furthermore, the study emphasizes the critical role of development metrics such as Cosine similarity (Gunawan et al. 2018 ) and KL Divergence (Bigi 2003 ), which are not just evaluation tools but are fundamental in refining and optimizing the functionality of these models. Algorithmic and computational techniques like ALS (Takács and Tikk 2012 ) and BM25 (Robertson et al. 2004 ) also play an integral part in the implementation and efficacy of these categorized models (refer to Sect. 4.1 ).
By extracting and analyzing this data, we aimed to comprehensively understand the existing literature, including popular open-access datasets used for training and evaluating the models. This knowledge empowered us to contribute insights and recommendations to the academic community, supporting them in selecting appropriate models and approaches for their intent modeling research endeavors.
3.2 Search process
In this study, we followed the review protocol presented in this section (see Fig. 1 ) to gather relevant studies.
The search process involved an automated search phase, which utilized renowned digital libraries such as ACM DL, IEEE Xplore, ScienceDirect, and Springer. However, Google Scholar was excluded from the automated search due to its tendency to generate numerous irrelevant studies. Furthermore, Google Scholar significantly overlaps the other digital libraries considered in this SLR. Table 1 provides an overview of the sequential phases of the search process, outlining the number of studies encompassed within each stage. It provides insights into the search process conducted in four phases:
Phase 1 (Pool of Publications): We initially performed a manual search, resulting in 314 relevant publications from Google Scholar. Additionally, automated searches from ACM DL, IEEE Xplore, ScienceDirect, and Springer contributed to the pool of publications with 586, 82, 921, and 1,896 relevant papers, respectively.
Phase 2 (Publication pruning process): In this phase, the inclusion/exclusion criteria were applied to the collected publications, ensuring the selection of high-quality and relevant studies. The numbers were reduced to 311 in ACM DL, 9 in IEEE Xplore, 246 in ScienceDirect, and 379 in Springer.
Phase 3 (Quality assessment process): Quality assessment was conducted for the publications based on several criteria, resulting in a final selection of 1067 studies from all sources.
Phase 4 (Data extraction and synthesizing + Snowballing process): During this phase, data extraction and synthesis were performed to gain insights into foundational intent modeling models, quality attributes, evaluation measures, and potential combinations of models used by researchers. Additionally, snowballing, involving reviewing references of selected publications, led to an additional 20 relevant papers. Applying the review protocol and snowballing, we retrieved 791 high-quality studies for our comprehensive analysis and synthesis in this systematic literature review.
4 Findings and analysis
In this section, we present the SLR results and provide an overview of the collected data Footnote 3 , which were analyzed to address the research questions identified in our study.
This study defines a ’model’ as a structured, mathematical, or computational framework specifically designed for simulating, predicting, or classifying phenomena within user intent modeling in conversational recommender systems. These models have been organized into distinct categories, each representing a unique approach to comprehending and interpreting user interactions.
Model categories: Our categorization includes various methodologies such as Supervised Learning, Unsupervised Learning, Collaborative Filtering, and others. For instance, models under Supervised Learning rely on labeled data for training, enabling them to make informed predictions or classifications. Unsupervised Learning models, in contrast, derive insights autonomously from unlabeled data, revealing underlying patterns without explicit guidance.
Development metrics: To measure and refine model performance, development metrics like Cosine similarity (Gunawan et al. 2018 ) and Kullback–Leibler (KL) Divergence (Bigi 2003 ) are employed. These metrics are not just evaluative tools; they are pivotal in enhancing system functionality and optimization throughout the development process. In the development and assessment of conversational recommender systems, it is essential to differentiate between metrics used for system development and those applied for model evaluation. Metrics such as Cosine similarity and KL Divergence are integral during the development phase, where they contribute significantly to system functionality and optimization. These metrics help fine-tune the system by assessing similarity measures and information loss. Conversely, the evaluation of model performance relies on a distinct set of measures, which are crucial for understanding the efficacy and accuracy of models in real-world applications. These evaluation measures are detailed in Sect. 4.5 , providing insights into how well the models perform regarding user intent prediction and recommendation accuracy.
Algorithmic and computational techniques: The study also underscores the importance of various algorithmic and computational techniques, such as ALS (Takács and Tikk 2012 ) and BM25 (Robertson et al. 2004 ). These techniques are integral to the practical implementation of the categorized models, aiding in critical tasks like data processing and system optimization.
The SLR conducted reveals a multifaceted landscape of models used in user intent modeling, each marked by its distinct methodology and application. Detailed information about these models and their categorizations can be found in the appendix (Appendix C).
Key categories such as Classification (Qu et al. 2019 ; Zhang et al. 2016 ) and Clustering (Zhang et al. 2021 ; Agarwal et al. 2020 ) models, Convolutional Neural Network (CNN)(Wang et al. 2020 ; Zhang et al. 2016 ), Deep Belief Networks (DBN)(Zhang et al. 2018 ; Hu et al. 2017 ), and Graph Neural Networks (GNN) (Yu et al. 2022 ; Lin et al. 2021 ) are highlighted. These categories, detailed in our SLR, represent a spectrum of techniques and approaches within user intent modeling.
Table 2 presents an overview of the 59 most frequently mentioned models in the SLR on user intent modeling. The table showcases the models appearing in at least six publications (columns) and their corresponding 18 categories (rows). Each model in user intent modeling can often be categorized into multiple categories, highlighting their versatility and diverse functionalities. For example, GRU4Rec (Hidasi and Karatzoglou 2018 ), a widely recognized model in the field (cited in 10 publications included in our review), exhibits characteristics that align with various categories. GRU4Rec falls under Supervised Learning, as it uses labeled examples during training to predict user intent. Additionally, it incorporates Collaborative Filtering techniques by analyzing user behavior and preferences to generate personalized recommendations, associating it with the Collaborative Filtering category (Latifi et al. 2021 ). Moreover, GRU4Rec can be classified as a Classification model as it categorizes input data into specific classes or categories to predict user intent (Park et al. 2020 ). It also demonstrates traits of Regression models by estimating and predicting user preferences or ratings based on the available data. Considering its reliance on recurrent connections, GRU4Rec can be associated with the Recurrent Neural Networks (RNN) category, enabling it to process sequential data and capture temporal dependencies (Ludewig and Jannach 2018 ). Lastly, GRU4Rec’s ability to cluster similar users or items based on their behavior and preferences places it within the Clustering category. This clustering capability provides valuable insights and recommendations to users based on their respective clusters.
4.2 Features
In our research, we analyzed user intent modeling within conversational recommender systems. This involved the identification of 74 distinct features, each frequently mentioned in a minimum of six publications. These features provide an alternative means of categorizing models based on the specific functions they are designed to serve, as described by the authors in their studies. Subsequently, we categorized the models utilized in these systems based on the features they support, presenting the results systematically in Table 3 .
We grouped these features into 20 categories, each reflecting specific contexts and applications. Features such as historical data references (Zhou et al. 2020 ; White et al. 2013 ; Zou et al. 2022 ) enable models to leverage past interactions for future predictions, while algorithm-agnostic models (Zhou et al. 2019 ; Musto et al. 2019 ; Mandayam Comar and Sengamedu 2017 ) offer flexibility in selecting the most suitable algorithms for specific tasks. Model-based features (Ding et al. 2022 ; Pradhan et al. 2021 ; Yu et al. 2018 ), which rely on statistical methods (Schlaefer et al. 2011 ; Kim et al. 2017 ) and semantic analysis (Zhang and Zhong 2016 ; Xu et al. 2015 ), are used to provide predictions based on predefined models.
The categorization includes various focus areas: ’Rule-Based Approaches’ use pattern and template methods to interpret user intent, while ’Query Processing’ models specialize in refining user queries to improve interaction quality. In ’Predictive Modeling’, the focus is on forecasting user preferences using techniques such as Prediction and Ratings Prediction. ’Text Analytics’ involves models that perform Topic Modeling, Text Similarity, and Semantic Analysis, which are crucial for analyzing user dialogues. Personalization features, ranging from ’User-Based Personalization’ and ’Temporal Personalization’ to ’Content-Based Personalization’ and ’Interaction-Based Personalization’, adapt recommendations according to user activity, time factors, content characteristics, and user interactions. Finally, ’Recommendation Techniques’ cover a broad spectrum of models optimized for tasks like Item Recommendation, Hybrid Recommendation, and Ranking.
Table 3 not only illustrates the mapping of features to models in user intent modeling but also highlights the frequency of explicit mentions of these features in relevant publications. The color coding in each cell indicates the level of support for each feature by the models, with gray cells denoting an absence of evidence supporting the feature’s compatibility with a particular model, based on our comprehensive review of 791 papers. For example, LDA is frequently mentioned in the context of pattern-based approaches within rule-based methods (Tang et al. 2010 ; Li et al. 2014 ), underscoring its applicability in scenarios where patterns are analyzed to extract meaningful insights.
The process of mapping features to models in user intent modeling requires an in-depth understanding of the particular features and the capabilities of the available models. For instance, in text analysis and natural language processing, models like LDA, TF-IDF, and BERT are often chosen for their effectiveness in semantic analysis and topic modeling. Similarly, for predictive modeling tasks, SVM, Random Forest, and Gradient Boosted Decision Trees (GBDT) are preferred due to their accuracy in classification and regression tasks. In cases where temporal dynamics are significant, models like LSTM, GRU, and Markov Chains are utilized for their ability to handle sequential data effectively. Furthermore, for tasks involving recommendation systems, models like Matrix Factorization (MF), Collaborative Filtering (CF), and Neural Collaborative Filtering (NCF) are often employed for their efficiency in capturing user preferences and generating personalized recommendations.
4.3 Model combinations
The data extraction and synthesis phase of the SLR identified 59 models, each referenced in a minimum of six publications. These models were often integrated to address various research considerations, such as feature requirements, quality attributes, and evaluation measures, as illustrated in Fig. 3 . The selected publications discussed combinations of models based on the authors’ research and evaluated the outcomes of these combinations.
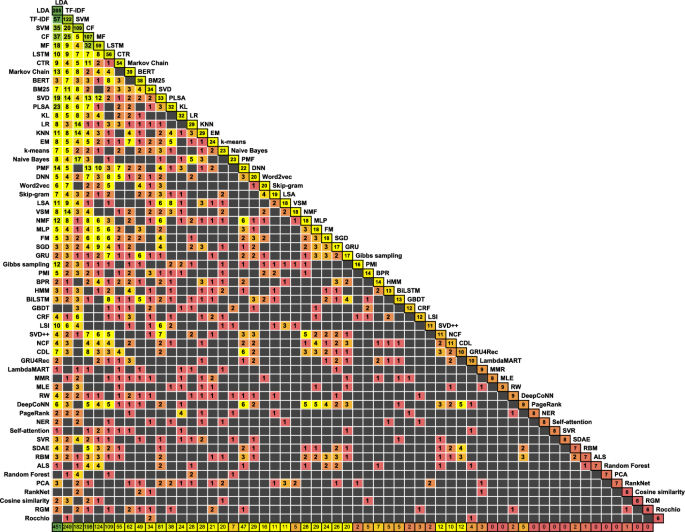
Shows the matrix representation of model combinations in user intent modeling research. The matrix shows the combinations of 59 models, with each cell indicating the number of publications discussing the model combination. Diagonal cells show the count of publications discussing each model individually. Green cells represent a higher number of research articles, yellow and red cells indicate a lower number, and gray cells show areas with no evidence of valid combinations. The last row indicates the frequency of publications where models were combined with others. For example, 451 publications mentioned LDA in combination with other models. This combination matrix offers insights into the frequency and popularity of model combinations, helping researchers identify existing combinations and potential research areas
To analyze model combinations, a matrix similar to a symmetric adjacency matrix was created, with models as nodes and combinations as edges in a graph. This matrix, shown in Fig. 2 , includes 59 models. Diagonal cells indicate the count of publications discussing each model independently, such as 205 papers on LDA (Chen et al. 2013 ; Weismayer and Pezenka 2017 ) and 122 on TF-IDF (Binkley et al. 2018 ; Izadi et al. 2022 ).
Matrix cells show the number of papers discussing model combinations. For instance, 57 papers explored the LDA and TF-IDF combination (Venkateswara Rao and Kumar 2022 ), and 35 examined SVM and LDA (Yu and Zhu 2015 ).
The matrix uses color coding to indicate the research volume associated with each combination. Green cells represent higher research volumes, yellow and red lower volumes, and gray cells indicate areas lacking evidence of valid combinations. These gray areas present opportunities for future research.
The combination matrix provides an overview of model combinations in user intent modeling research, highlighting the frequency of their use in literature and serving as a resource for identifying existing combinations and potential research areas.
Combining various models, often termed as ’ensemble’ or ’hybrid’ modeling (Sagi and Rokach 2018 ), can enhance the predictive power (Beemer et al. 2018 ) and accuracy of conversational recommender systems. However, this approach is subject to certain constraints and requires careful consideration.
Firstly, it’s crucial to acknowledge that while combining models is possible, it’s not always straightforward or advantageous. The feasibility of integrating multiple models depends on several factors:
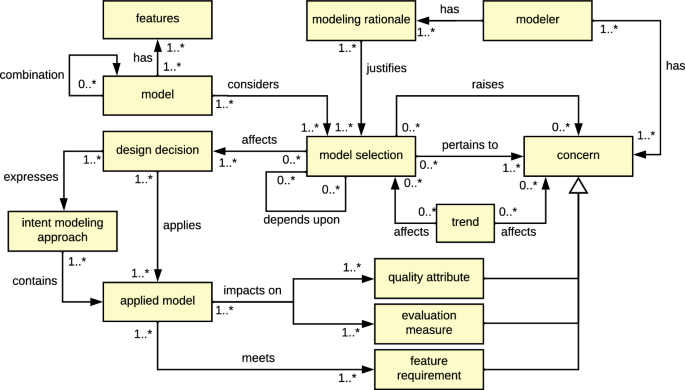
The decision-making process researchers employ in selecting intent modeling approaches within the academic literature
Compatibility : The models to be combined must be compatible in terms of input and output data formats, scale, and the nature of predictions they make (Srivastava et al. 2020 ). For instance, combining a probabilistic model with a neural network requires a harmonious interface where the output of one can effectively serve as the input for another.
Complexity and overfitting : Increasing model complexity can lead to overfitting, where the model performs well on training data but poorly on unseen data (Sagi and Rokach 2018 ). It is essential to balance the complexity with the generalizability of the model.
Computational resources : More complex ensembles demand greater computational power and resources. This can be a limiting factor, especially in real-time applications (Bifet et al. 2009 ).
Interpretability: Combining models can sometimes lead to a loss of interpretability, making it challenging to understand how predictions are made, which is crucial for certain applications (Wang and Lin 2021 ).
Regarding a more in-depth analysis, combining models indeed necessitates a thorough evaluation of their individual and collective performance. This includes assessing how they complement each other, their synergistic potential, and the trade-offs involved.
4.4 Model trends
In recent studies, machine learning models have witnessed significant advancements across various fields, leading to notable trends in their development and application. However, it is worth noting that our study goes beyond recent years. By using the term “models,” we refer to a wide range of models that research modelers can employ in user intent modeling.
To gain insights into the usage patterns of these models, we organized the 59 selected models (mentioned in at least six publications) based on the publication years of the studies that referenced them. The span of these publications ranges from 2002 to 2023. Table 4 provides an overview of these trends.
Among the selected models, LDA, TF-IDF, SVM, CF, and MF emerged as the top five most frequently mentioned models, appearing in over 500 papers. It is important to note that while some recently gained substantial attention, such as BERT (Yao et al. 2022 ), CF (Yadav et al. 2022 ), LSTM (Xu et al. 2022 ; Gozuacik et al. 2023 ), DNN (Yengikand et al. 2023 ), and GRU (Chen and Wong 2020 ; Elfaik 2023 ), our study encompasses models from various time periods.
These trends shed light on the popularity and usage patterns of different models in user intent modeling. By identifying frequently mentioned models and observing shifts in their prevalence over time, researchers and practitioners can stay informed about the evolving landscape of user intent modeling and make informed decisions when selecting models for their specific applications (Zaib et al. 2022 ; Ittoo and van den Bosch 2016 ).
4.5 Quality models and evaluation measures
In AI-based projects, selecting high-quality models and using evaluation measures is crucial. Quality attributes, defined in studies (de Barcelos Silva et al. 2020 ; Hernández-Rubio et al. 2019 ), reflect a model’s performance, effectiveness, and user-centric features in conversational recommender systems. These attributes are essential for a comprehensive evaluation but are not straightforward to measure empirically. They often require subjective assessment or indirect methods. “Novelty,” for example, relates to the uniqueness of recommendations (Cremonesi et al. 2011 ). Although challenging to quantify, methods like user studies or item distribution analysis can offer insights into a model’s novelty. Conversely, evaluation measures, as discussed in literature (Zaib et al. 2022 ), provide a quantitative assessment of model outputs. These attributes and measures are pivotal in delivering accurate and reliable results, as various studies demonstrate (Pan et al. 2022 ; Pu et al. 2012 ; Hernández-Rubio et al. 2019 ).
While accuracy is a commonly employed evaluation measure, it may not adequately represent the model’s performance, especially in imbalanced classes. Alternative measures such as precision (Salle et al. 2022 ; Baykan et al. 2011 ), recall (Wang et al. 2022 ; Phan et al. 2010 ), and F1-score (Yu et al. 2019 ; Ashkan et al. 2009 ) are used to evaluate model performance, particularly when dealing with imbalanced data. Additionally, evaluation measures like the area under the curve (AUC) (Xu et al. 2016 ; Liu et al. 2022 ) and receiver operating characteristic (ROC) (Wu et al. 2019 ; Wang et al. 2020 ) curve are frequently used to assess binary classifiers. These measures provide insights into the model’s ability to differentiate between positive and negative instances, particularly when the costs of false positives and false negatives differ.
For ranking problems, evaluation measures such as mean average precision (MAP) (Mao et al. 2019 ; Ni et al. 2012 ) and normalized discounted cumulative gain (NDCG) (Liu et al. 2020 ; Kaptein and Kamps 2013 ) are commonly employed. These measures evaluate the quality of the ranked lists generated by the model and estimate its effectiveness in predicting relevant instances.
When evaluating regression models, measures such as root mean squared error (RMSE) (Cai et al. 2014 ; Colace et al. 2015 ) and mean absolute error (MAE) (Yao et al. 2017 ; Yadav et al. 2022 ) are used to quantify the discrepancy between predicted values and actual values of the target variable.
The selection of appropriate evaluation measures is crucial to ensure the accuracy and reliability of machine learning models. The suitable measure(s) choice depends on the specific problem domain, data type, and project objectives. These factors are pivotal in selecting the most appropriate quality attributes and evaluation measures. Table 5 presents the quality attributes and evaluation measures identified in at least six publications. Performance, Effectiveness, Diversity, Usefulness, and Stability are among the top five quality attributes. Precision, recall, F1-score, accuracy, and NDCG are among the top five evaluation measures identified in the SLR. For detailed explanations of the identified quality attributes and evaluation measures, please refer to Appendix E.
4.6 Datasets
Datasets are fundamental to machine learning and data science research, as they provide the raw material for training and testing models and enable the development of solutions to complex problems. They come in various forms and sizes, ranging from small, well-curated collections to large, messy datasets with millions of records. The quality of datasets is crucial (Pan et al. 2022 ), as high-quality data ensure the accuracy and reliability of models, while poor-quality data can introduce biases and inaccuracies. Data quality encompasses completeness, accuracy, consistency, and relevance, and ensuring data quality involves cleaning, normalization, transformation, and validation.
The size and complexity of datasets pose challenges in terms of storage, processing, and analysis. Big datasets require specialized tools and infrastructure to handle the volume and velocity of data. On the other hand, complex datasets, such as graphs, images, and text, may require specialized techniques and models for extracting meaningful information and patterns.
Furthermore, the availability of datasets is a vital consideration in advancing machine learning research and applications. Open datasets that are freely accessible and well-documented foster collaboration and innovation, while proprietary datasets may restrict access and impede progress (Zhang et al. 2016 ; Teevan et al. 2008 ; Ittoo and van den Bosch 2016 ). Data sharing and ethical considerations in data use are increasingly recognized, leading to efforts to promote open-access and responsible data practices.
In this study, we identified 80 datasets that researchers have utilized in the context of intent modeling approaches, and these datasets were mentioned in at least two publications. Table 6 provides an overview of these datasets and their frequency of usage from 2005 to 2023. Notably, TREC, MovieLens, Amazon, Yelp, and AOL emerged as the top five datasets commonly used in evaluating intent modeling approaches for recommender systems (Wang et al. 2021 ; Papadimitriou et al. 2012 ; Wang et al. 2020 ) and search engines (Fan et al. 2022 ; Liu et al. 2022 ; Konishi et al. 2016 ). These datasets have been utilized in over 200 publications, highlighting their significance and wide adoption in the field.
The datasets selected for this study cover a broad range of scenarios in user intent modeling for conversational recommender systems. This diversity aligns with the comprehensive nature of the research. Each dataset contributes unique insights into user behaviors, preferences, and interactions, which are crucial for understanding and effectively modeling user intent within conversational interfaces.
The variety of datasets reflects the complexity of conversational recommender systems, which need to address varied user needs, contexts, and interaction modes. Including datasets that differ in size, structure, and origin ensures the study captures a broad spectrum of user interactions and system responses, providing a solid foundation for developing and evaluating intent modeling approaches.
5 Decision-making process
This section describes how researchers make decisions when selecting intent modeling approaches. It illustrates a systematic approach to choosing intent modeling methods based on academic literature.
5.1 Decision meta-model
Research modelers face the challenge of selecting the most suitable combination of models to develop an intent modeling approach for a conversational recommender system. In this section, we present a meta-model for the decision-making process in the context of intent modeling. Adopting this meta-model is based on the principles outlined in the ISO/IEC/IEEE standard 42010 (ISO 2011 ), which provides a framework for conceptual modeling of Architecture Description. This process requires a systematic approach to ensure that the chosen models effectively capture and understand users’ intentions. Let’s consider a scenario where research modelers encounter this challenge and go through the decision-making process:
Goal and concerns: The research modelers aim to build an intent modeling approach for a conversational recommender system. Their goal is to accurately determine the underlying purposes or goals behind users’ requests, enabling personalized and precise responses. The modelers have concerns regarding quality attributes and functional requirements, and they aim to achieve an acceptable level of quality based on their evaluation measures.
Identification of models and features: To address this problem, the modelers consider various models that can capture users’ intentions in the conversational context. They identify essential features, such as user intent prediction or context analysis based on their concerns. They explore the available models and techniques, such as Supervised Learning , Unsupervised Learning , Recurrent Neural Networks , Deep Belief Networks , Clustering , and Self-Supervised Learning Models . The modelers also consider the recent trends in employing models for intent modeling.
Evaluation of models: The modelers review the descriptions and capabilities of several models that align with capturing users’ intentions in conversational interactions. They analyze each model’s strengths, limitations, and applicability to the intent modeling problem. They consider factors such as the models’ ability to handle natural language input, understand context, and predict user intents accurately. This evaluation allows them to shortlist a set of candidate models that have the potential to address the intent modeling challenge effectively.
In-depth analysis: The research modelers conduct a more detailed analysis of the shortlisted models. They examine the associated techniques for each model to ensure their suitability in the conversational recommender system. They assess factors such as training data requirements, model complexity, interpretability, and scalability. Additionally, they explore the possibility of combining models to identify compatible combinations or evaluate the existing literature on such combinations. If necessary, further study may be conducted to assess the feasibility of model combinations. This step helps them identify the optimal combination of models that best capture users’ intentions in the conversational setting and address their concerns.
5.2 A decision model for intent modeling selection
Decision theories have wide-ranging applications in various fields, including e-learning (Garg et al. 2018 ) and software production (Xu and Brinkkemper 2007 ; Fitzgerald and Stol 2014 ; Rus et al. 2003 ). In the literature, decision-making is commonly defined as a process involving problem identification, data collection, defining alternatives and selecting feasible solutions with ranked preferences (Fitzgerald et al. 2017 ; Kaufmann et al. 2012 ; Garg 2020 ; Garg et al. 2017 ; Sandhya et al. 2018 ; Garg 2019 ). However, decision-makers approach decision problems differently, as they have their priorities, tacit knowledge, and decision-making policies (Doumpos and Grigoroudis 2013 ). These differences in judgment necessitate addressing them in decision models, which is a primary focus in the field of multiple-criteria decision-making (MCDM).
MCDM problems involve evaluating a set of alternatives and considering decision criteria (Farshidi et al. 2023 ). The challenge lies in selecting the most suitable alternatives based on decision-makers’ preferences and requirements (Majumder 2015 ). It is important to note that MCDM problems do not have a single optimal solution, and decision-makers’ preferences play a vital role in differentiating between solutions (Majumder 2015 ). In this study, we approach the problem of model selection as an MCDM problem within the context of intent modeling approaches for conversational recommender systems.
Let \(Models={m_1,m_2, \dots , m_{\Vert Models\Vert }}\) be a set of models found in the literature (decision space), such as LDA , SVM , and BERT . Let \(Features={f_1,f_2, \dots , f_{\Vert Features\Vert }}\) be a set of features associated with the models, such as ranking, prediction, and recommendation. Each model \(m \in Models\) supports a subset of the set Features and satisfies a set of evaluation measures ( \(Measures={e_1,e_2, \dots , e_{\Vert Measures\Vert }}\) ) and quality attributes ( \(Qualities={q_1,q_2, \dots , q_{\Vert Qualities\Vert }}\) ). The objective is to identify the most suitable models, or a combination of models, represented by the set \(Solutions \subset Models\) , that address the concerns of researchers denoted as Concerns , where \(Concerns \subseteq \{ Features \cup Measures \cup Qualities \}\) . Accordingly, research modelers can adopt a systematic strategy to select combinations of models by employing an MCDM approach. This approach involves taking Models and their associated Features as input and applying a weighted combination to prioritize the Features based on the preferences of decision-makers. Subsequently, the defined Concerns are considered, and an aggregation method is utilized to rank the Models and propose fitting Solutions . Consequently, the MCDM approach can be formally expressed as follows:
The decision model developed for intent modeling, using MCDM theory and depicted in Fig. 3 , is a valuable tool for researchers working on conversational recommender systems. This approach helps researchers explore options systematically, consider important factors for conversational interactions, and choose the best combination of models to create an effective intent modeling approach. The decision model suggests five steps for selecting a combination of models for conversational recommender systems:
Models: In this phase, researchers should gain insights into best practices and well-known models employed by other researchers in designing conversational recommender systems. Appendix B can be used to understand the definitions of models, while Appendix C can help become familiar with the categories used to classify these models. Table 2 illustrates the categorization of models in this study, and Table 4 presents the trends observed among research modelers in utilizing models to build their conversational recommender systems.
Feature requirements elicitation: In this step, researchers need to fully understand the core aspects of the intent modeling problem they are studying. They should carefully analyze their specific scenario to identify the key characteristics required in the models they seek, which may involve using a combination of models. For instance, researchers might consider prediction, ranking, and recommendation as essential feature requirements for their conversational recommender systems. Researchers can refer to Appendix D to gain a better understanding of feature definitions and model characteristics, which will help them select the most suitable features for their intent modeling project.
Finding feasible solutions: In this step, researchers should identify models that can feasibly fulfill all of their feature requirements. Table 3 can be used to determine which models support specific features. For example, the table shows that 99 publications explicitly mentioned Collaborative Filtering as a suitable model for applications requiring predictions, and 94 publications indicated CF’s applicability for ranking. Moreover, 46 studies employed CF for item recommendation. Based on these findings, if a conversational recommender system requires these three feature requirements, CF could be selected as one of the potential solutions. If the number of feature requirements increases, the selection problem can be converted into a set covering problem (Caprara et al. 2000 ) to identify the smallest sub-collection of models that collectively satisfy all feature requirements.
Selecting feasible combinations: In this phase, researchers need to assess whether the identified models can be integrated or combined. Figure 2 provides information on the feasibility of combining models based on the reviewed articles in this study. If the table does not indicate a potential combination, it does not necessarily imply that the combination is impossible. It means no evidence supports its feasibility, and researchers should investigate the combination independently.
Performance analysis: After identifying a set of feasible combinations, researchers should address their remaining concerns regarding quality attributes and evaluation measures. Table 5 and Appendix E can be used to understand the typical concerns other researchers in the field employ. Additionally, Table 6 provides insights into frequently used datasets across domains and applications. Researchers can then utilize off-the-shelf models from various libraries, such as TensorFlow Footnote 4 and scikit-learn, Footnote 5 to build their solutions (pipelines). These solutions can be evaluated using desired datasets to assess whether they meet all the specified concerns. This phase of the decision model differs from the previous four phases, as it requires significant ad-hoc efforts in developing, training, and evaluating the models. By employing this decision-making process, research modelers can develop an intent modeling approach that accurately captures and understands users’ intentions in the conversational recommender system. This enables personalized and precise responses, enhancing the overall user experience and satisfaction.
6 Evaluation of findings: case studies
In this section, we detail the evaluation of our proposed decision model (see Sect. 5 ) through two scientific case studies. These studies were conducted by a team of eight researchers from the University of California San Diego, USA, and the University of Klagenfurt, Austria. The primary goal was to test the decision model’s applicability in the participants’ projects and to understand their decision-making processes better.
During the case studies, participants specified their unique feature requirements, which we recorded in Table 3 . Essentially, after reviewing the features listed in the Table, the participants defined their requirements. Using these data, we pinpointed suitable models from the extensive information in Table 2 and Table 3 . We then examined potential combinations of these models, as depicted in Fig. 2 .
To evaluate the significance and recognition of the chosen models in academic circles, we undertook a detailed analysis, referencing Table 4 . This examination yielded insights into the models’ popularity and relevance over time within the research community. The most notable and trending combinations were then presented to the case study participants. Figure 3 provides a schematic of the typical decision-making process researchers follow when selecting models for intent modeling.
Table 7 offers a thorough summary of the conducted case studies. This table outlines the specific contexts of each study, the feature requirements identified by the participants, the model selections made by the researchers based on these requirements, and the outcomes from applying our decision model in each scenario. The following sections will delve deeper into these case studies, discussing the addressed concerns, the results achieved using the decision model, and the conclusions drawn from our comprehensive analysis.
6.1 Case study method
Case study research is an empirical research method (Jansen 2009 ) that investigates a phenomenon within a particular context in the domain of interest (Yin 2017 ). Case studies can be employed to describe, explain, and evaluate a hypothesis. They involve collecting data regarding a specific phenomenon and applying a tool to evaluate its efficiency and effectiveness, often through interviews. In our study, we followed the guidelines outlined by Yin ( 2009 ) to conduct and plan the case studies.
Objective: The main aim of this research was to conduct case studies to evaluate the effectiveness of the decision model and its applicability in the academic setting for supporting research modelers in selecting appropriate models for their intent modeling approaches.
The cases: We conducted two case studies within the academic domain to assess the practicality and usefulness of the proposed decision model. The case studies aimed to evaluate the decision model’s effectiveness in assisting research modelers and researchers in selecting models for their intent modeling tasks.
Methods: For the case studies, we engaged with research modelers and researchers actively involved in intent modeling approaches. We collected data through expert interviews and discussions to gain a comprehensive understanding of their specific requirements, preferences, and challenges when selecting models. The case study participants provided valuable insights into the decision-making process and offered feedback on the suitability of the decision model for their intent modeling needs.
Selection strategy: In line with our research objective, we employed a multiple case study approach (Yin 2009 ) to capture a diverse range of perspectives and scenarios within the academic domain. This selection strategy aimed to ensure the credibility and reliability of our findings. We deliberately selected two publications from highly regarded communities with an A* CORE rank. We verified the expertise of the authors, who actively engage in selecting and implementing intent modeling models. Their knowledge and experience allowed us to consider various factors in different application contexts, including quality attributes, evaluation measures, and feature requirements.
By conducting these case studies, our research aimed to validate the practicality of the decision model and demonstrate its value in supporting research modelers and researchers in their intent modeling endeavors. The insights gained from the case studies provided valuable feedback for refining the decision model and contributed to advancing the intent modeling field within the academic community.
6.2 Case study 1:
The first case study presented in our paper revolves around a research project conducted at the University of Klagenfurt in Austria. The study focused on investigating a retrieval-based approach for conversational recommender systems (CRS) (Manzoor and Jannach 2022 ). The primary objective of the researchers was to assess the effectiveness of this approach as an alternative or complement to language generation methods in CRS. They conducted user studies and carefully analyzed the results to understand the potential benefits of retrieval-based approaches in enhancing user intent modeling for conversational recommender systems.
Throughout the project, the case study participants made two important design decisions (models), TF-IDF and BERT, to develop the CRS. They evaluated their approach using MovieLens and ReDial datasets to measure its performance.
By applying the decision model presented in our paper (in Sect. 5.2 ), the case study participants identified six essential features that were crucial in guiding their decision-making process for selecting the most suitable models and datasets. These features provided valuable insights into designing and implementing an effective retrieval-based approach for conversational recommender systems, contributing to improving user intent modeling in this context.
6.2.1 Feature requirements
In this section, we outline the feature requirements that the case study participants considered during their decision-making process for the research project. Each feature requirement was carefully chosen based on its relevance and potential to enhance the retrieval-based approach for CRS. Below are the feature requirements and their rationale for selection:
Semantic analysis: The case study participants recognized the importance of analyzing the meaning and context of words and phrases in natural language data. Semantic analysis helps the model understand user intents more accurately, leading to more relevant and contextually appropriate recommendations.
Term weighting: Assigning numerical weights to terms or words in a document or dataset helps the machine learning model comprehend the significance of different terms in the data. The participants adopted term weighting to improve the model’s ability to identify relevant features and make better recommendations.
Content-based recommendations: This feature involves utilizing item characteristics or features to recommend similar items to users. The participants valued this approach, allowing the system to tailor recommendations based on users’ past interactions and preferences.
Ranking: The case study participants sought a model capable of ranking items or entities based on their relevance to specific queries or users. By incorporating ranking, the system ensures that the most relevant recommendations appear at the top, enhancing user satisfaction.
Transformer-based: Transformer-based models, such as neural networks, excel at learning contextual relationships in sequential data like natural language. The participants chose this approach to effectively leverage the model’s ability to understand and process conversational context.
End-to-end approach: The case study participants preferred an end-to-end modeling strategy, where a single model directly learns complex tasks from raw data inputs to desired outputs. By avoiding intermediate stages and hand-crafted features, the participants aimed to simplify the model and improve its performance in CRS tasks.
6.2.2 Results and analysis
During the expert interview session with the case study participants, we systematically followed the decision model presented in Sect. 5.2 to identify appropriate combinations of models that align with the defined feature requirements for their conversational recommender systems. In the initial steps (Steps 1 and 2), we collaboratively established the essential feature requirements for their CRS, carefully considering the critical aspects that would enhance their system’s performance. Subsequently, we referred to Table 3 (Steps 3 and 4) to evaluate which models could fulfill these specific feature requirements.
Upon analyzing the table, both the case study participants and we discovered that BERT offered support for Semantic Analysis, Content-Based Recommendations, Ranking, Transformer-Based, and End-To-End Approaches. Additionally, TF-IDF was found to be supportive of Term Weighting, Content-Based Recommendations, and Ranking. This insightful information made us realize that combining these two models would adequately address all six feature requirements for their CRS. Consequently, the case study participants confirmed that combining BERT and TF-IDF would be a suitable choice to fulfill their CRS needs. This combination was validated as a compatible and valid option, consistent with the guidance provided by the decision model.
The data presented in Table 4 further reinforce the popularity and relevance of BERT and TF-IDF as widely used models for conversational recommender systems. The case study participants were well aware of these trends and acknowledged that their model choices aligned with prevailing practices. This alignment provides additional validation to their model selections, demonstrating their dedication to adopting the latest technologies in their research project to create an effective CRS.
Furthermore, Table 6 provides valuable insights into the popularity and significance of various datasets, including MovieLens and ReDial. These datasets have been cited and utilized in over 50 publications, underscoring their recognition within the research community. The case study participants acknowledged the widespread use of these datasets by other researchers, reflecting an interesting trend in dataset selection. This awareness further highlights their commitment to utilizing well-established and reputable datasets in their research, contributing to the credibility and reliability of their study findings.
6.3 Case study 2
The second case study presented in our paper focuses on a research project conducted at the University of California San Diego in the United States (Tanjim et al. 2020 ). The study introduces the Attentive Sequential model of Latent Intent (ASLI) to enhance recommender systems by capturing users’ hidden intents from their interactions.
Understanding user intent is essential for delivering relevant recommendations in conventional recommender systems. However, user intents are often latent, meaning they are not directly observable from their interactions. ASLI addresses this challenge by uncovering and leveraging these latent user intents.
Using a self-attention layer, the researchers (case study participants) designed a model that initially learns item similarities based on users’ interaction histories. They incorporated a Temporal Convolutional Network (TCN) layer to derive latent representations of user intent from their actions within specific categories. ASLI employs an attentive model guided by the latent intent representation to predict the next item for users. This enables ASLI to capture the dynamic behavior and preferences of users, resulting in state-of-the-art performance on two major e-commerce datasets from Etsy and Alibaba.
By utilizing the decision model presented in our paper (in Sect. 5.2 ), the case study participants identified eight essential features crucial in guiding their decision-making process for selecting the most suitable models and datasets.
6.3.1 Feature requirements
In this section, we present the feature requirements that were crucial considerations for the case study participants during their decision-making process for the research project. The following are the feature requirements and the reasons behind their selection:
Pattern-based: In the case study, the researchers aimed to improve conversational recommender systems by capturing users’ hidden intents from their interactions. By identifying user interactions and behavior patterns, the ASLI model can make informed guesses about users’ intents and preferences, leading to more accurate and relevant recommendations.
Prediction: The ASLI model predicts the next item for users based on their latent intents derived from their historical interactions within specific categories. The model can deliver personalized and effective recommendations by predicting users’ preferences and future actions.
Historical data-driven recommendations: The researchers used previously collected data from users’ interactions to train the ASLI model. By analyzing historical data, the model can identify patterns, relationships, and trends in users’ behaviors, which inform its predictions and recommendations for future interactions.
Click-through recommendations: In the case study, the ASLI model considers users’ clicks on items to understand their preferences and improve the relevance and ranking of future recommendations. The model can adapt and refine its recommendations by utilizing click-through data to meet users’ needs better.
Item recommendation: The ASLI model suggests items to users based on their previous interactions, enabling it to offer personalized recommendations tailored to individual users’ preferences and behaviors.
Transformer-based: ASLI is a neural network model based on the Transformer architecture. Transformers are well-suited for learning context and meaning from sequential data, making them suitable for capturing the dynamic behavior and preferences of users in conversational recommender systems.
Network architecture: The ASLI model’s network architecture is crucial in guiding information flow through the model’s layers. By designing an effective network architecture, the researchers ensure that the model can capture and leverage users’ latent intents to make accurate recommendations.
Attentive: ASLI utilizes attention mechanisms to focus on the most relevant parts of users’ interactions and behaviors. The model can better understand users’ intents and preferences by paying attention to critical information, leading to more attentive and accurate recommendations.
6.3.2 Results and analysis
During the expert interview session with the case study participants, we used the decision model (outlined in Sect. 5.2 ) to identify suitable combinations of models that align with the defined feature requirements for their conversational recommender systems. In Steps 1 and 2, we collaboratively established the essential feature requirements for the ASLI, carefully considering critical aspects to enhance system performance. Then, in Steps 3 and 4, we referred to Table 3 to evaluate models that could fulfill these specific feature requirements.
According to the table, both the case study participants and ourselves found that the GRU model supports Prediction, Historical Data-Driven Recommendations, Click-Through Recommendations, Network Architecture, and Attentive features. Additionally, the LDA model supports Pattern-Based and Item Recommendation features. We also discovered that BERT is the only model in our list supporting Transformer-Based features, and the case study participants agreed with this combination, considering these models as the baseline of their approach. However, after performance analysis, they found that GRU’s performance was unsatisfactory in their setting. Consequently, they chose to develop their own model from scratch, modifying the self-attentive model. It’s worth noting that the Self-attentive model only supports Network Architecture and Attentive features, making it a suitable baseline in combination with other models for their solutions. The case study participants mentioned considering LDA and BERT as potential models for their upcoming research project due to their similar requirements, although they were not previously aware of this combination. As per Step 5 of the decision model, researchers should address any remaining concerns about quality attributes and evaluation measures after identifying feasible combinations. Thus, the decision model provided valid models in this case study, but in real-world scenarios, model combinations may be modified based on other researchers’ concerns, such as quality attributes and evaluation measures.
The case study participants emphasized the value of the data presented in Table 4 and their intention to incorporate it into their future design decisions. Understanding trends in model usage is crucial to identify models that may perform well in conversational recommender systems, considering similar concerns and requirements from other researchers.
Furthermore, Table 6 indicates that Etsy and Alibaba datasets are not widely known in the context of user intent modeling, although the case study participants clarified that these datasets are well-known in e-commerce services, aligning with their project’s specific domain of focus. Nonetheless, they expressed their intention to utilize the data presented in this table to explore potential datasets for evaluating their approach and comparing their work against other approaches in the literature.
7 Discussion
7.1 slr outcomes.
Code sharing: Our review of 791 publications revealed that only 68 (8.59%) explicitly shared their code repositories, such as GitHub. This observation underscores a significant gap in code sharing among researchers, posing challenges to replicating experiments and advancing scientific knowledge. Open access to code is imperative for ensuring transparency and reproducibility in machine learning research (Haefliger et al. 2008 ).
Singleton models: The systematic literature review yielded 600 models, with 352 (58.66%) being singletons. This trend indicates a preference for developing unique models tailored to specific research questions. However, an overreliance on singletons might hinder the generalizability of findings and the ability to compare different methods. Promoting the use of common models or establishing standard evaluation benchmarks is essential to enhance reproducibility and comparability in machine learning research (Amershi et al. 2019 ).
Model combination: The methodology for combining models in some publications was not clearly articulated, making it difficult to understand the techniques employed and their efficacy. Clear documentation of model combination techniques and their underlying rationale is crucial for ensuring transparency and facilitating the replication and extension of research findings (Kuwajima et al. 2020 ). The challenge lies in determining the effectiveness of integrating different models without extensive contextual information. The current approach, based on literature and general requirements, provides a foundational framework but may not capture the specific nuances needed for particular applications. Future research should involve detailed analyses of model combinations in specific scenarios, using case studies or empirical evaluations to provide insights into the interactions and complementarity of different models, thereby enhancing the practical applicability of intent modeling methods in conversational recommender systems.
Model variations: Our analysis identified a diverse range of model variations, such as BERT4Rec (Chen et al. 2022 ), SBERT (Garcia and Berton 2021 ), BERT-NeuQS (Hashemi et al. 2020 ), BioBERT (Carvallo et al. 2020 ), ELBERT (Gao and Lam 2022 ), and RoBERTa (Wu et al. 2021 ), primarily derived from BERT (Devlin et al. 2018 ). Despite the utility of these variations in addressing different tasks, their extensive use complicates model comparison and experiment replication. Establishing standardized categories for model variations would aid researchers in discerning model differences and similarities, thereby promoting model sharing, reuse, and collaborative progress in machine learning research (Sarker 2021 ).
Trends: As depicted in Fig. 2 , LDA is a predominant model in user intent modeling approaches (Table 4 ). Although traditional models like LDA have significantly contributed to the field and inspired the development of advanced models such as BERT (Devlin et al. 2018 ), the adoption of traditional models has possibly declined due to the emergence of sophisticated models like BERT. The bidirectional contextual embeddings and transformer architecture of BERT have demonstrated remarkable performance across various NLP tasks, attracting considerable attention from the research community. The preference for modern models is also influenced by the trade-off between the interpretability of traditional models and the complexity of advanced models like BERT, as well as the diversity of NLP applications (Ribeiro et al. 2016 ).
Datasets: Only 394 out of 791 publications (49.81%) utilized public, open-access datasets, indicating a reliance on proprietary datasets by more than half of the publications. This limitation hinders data reuse and poses challenges to research reproducibility and credibility. While 253 public open-access datasets were identified, 173 (68.37%) were mentioned in only one publication and not reused, highlighting deficiencies in dataset-sharing practices. The limited availability of datasets impedes the reproduction and validation of results, comparison, and benchmarking of models, and identification of state-of-the-art techniques. Moreover, the lack of diverse and openly accessible datasets may result in biased model development and evaluation, limiting the applicability of models to real-world scenarios and diverse user populations. Addressing these issues necessitates fostering a culture of openness and collaboration within the research community.
7.2 Case study participants
The case study participants showed a careful and thorough approach to decision-making by conducting extensive research and literature reviews. This method allowed them to select models for their research project carefully, showcasing the effectiveness of the decision model in helping researchers make well-informed and compatible model choices for developing conversational recommender systems.
Both case study participants emphasized the value of using the decision model and the knowledge gained during this study. They expressed their intention to use this information to make informed decisions when selecting the appropriate combinations of models for user intent modeling approaches.
Furthermore, the case study participants recognized that the decision model serves as a valuable tool for generating an initial list of models to develop their approaches. However, they acknowledged that Step 5 of the decision model highlights the importance of further analysis, such as performance testing, to identify the right combinations of models that work well for specific use cases. This recognition underscores the need for practical testing and validation to ensure the chosen model combinations are effective and suitable for their particular research goals.
The use of well-known datasets, such as MovieLens and ReDial in the first case study and Etsy and Alibaba datasets in the second case study, underlines the researchers’ commitment to using credible data sources for evaluation. The decision model allowed researchers to consider dataset popularity and relevance, enhancing the credibility and reliability of their study findings.
The decision model provided valuable insights into the trends in model usage, as presented in Table 4 . Both case study participants expressed interest in incorporating these trends into their future research decisions, ensuring they stay up-to-date with the latest advancements in intent modeling approaches.
Throughout the case studies, the discussion highlighted the dynamic nature of the decision-making process. While the decision model offered feasible model combinations based on feature requirements, the final choices were influenced by additional factors such as model performance, quality attributes, and evaluation measures. This adaptability showcased the decision model’s flexibility in accommodating researchers’ unique priorities and preferences.
Both case studies effectively demonstrated that the decision model offers a systematic approach to model selection and helps researchers explore various options and combinations of models. This exploratory nature allowed researchers to consider novel solutions and build upon existing models, creating innovative intent modeling approaches.
The success of the decision model in assisting researchers in their model selection process holds promising implications for the broader academic community. By providing a structured and comprehensive methodology, the decision model can streamline the development of conversational recommender systems with accurate intent modeling capabilities, ultimately enhancing user experience and satisfaction.
7.3 Threat to validity
Validity evaluation is essential in empirical studies, encompassing SLRs and case study research (Zhou et al. 2016 ). This paper’s validity assessment covers various dimensions, including Construct Validity, Internal Validity, External Validity, and Conclusion Validity. Although other types of validity, such as Theoretical Validity and Interpretive Validity, are relevant to intent modeling, they are not explicitly addressed in this context due to their relatively limited exploration.
Construct validity pertains to the accuracy of operational measures or tests used to investigate concepts. In this research, we developed a meta-model (refer to Fig. 3 ) based on the ISO/IEC/IEEE standard 42010 (ISO 2011 ) to represent the decision-making process in intent modeling for conversational recommender systems. We formulated comprehensive research questions by utilizing the meta-model’s essential elements, ensuring an exhaustive coverage of pertinent publications on intent modeling approaches.
Internal validity concerns verifying cause-effect relationships within the study’s scope and ensures the study’s robustness. We employed a rigorous quasi-gold standard (QGS) (Zhang et al. 2011 ) to minimize selection bias in paper inclusion. Combining manual and automated search strategies, the QGS provided an accurate evaluation of sensitivity and precision. Our search spanned four major online digital libraries, widely regarded to encompass a substantial portion of high-quality publications relevant to intent modeling for conversational recommender systems. Additionally, we used snowballing to complement our search and mitigate the risk of missing essential publications. The review process involved a team of researchers, including three principal investigators and five research assistants. Furthermore, the findings were validated by real-world researchers in intent modeling to ensure their practicality and effectiveness.
External validity pertains to the generalizability of research findings to real-world applications. This study considered publications discussing intent modeling approaches across multiple years. Although some exclusions and inaccessibility of studies may impact the generalizability of SLR and case study results, the proportion of inaccessible studies (less than 2%) is not expected to affect the overall findings significantly. The knowledge extracted from this research can be applied to support the development of new theories and methods for future intent modeling challenges, benefiting both academia and practitioners in this field.
Conclusion validity ensures that the study’s methods, including data collection and analysis, can be replicated to yield consistent results. We extracted knowledge from selected publications, encompassing various aspects such as Models , Datasets , Evaluation Metrics , Quality Attributes , Combinations , and Trends in intent modeling approaches. The accuracy of the extracted knowledge was safeguarded through a well-defined protocol governing the knowledge extraction strategy and format. The authors proposed and reviewed the review protocol, establishing a clear and consistent approach to knowledge extraction. A data extraction form was employed to ensure uniform extraction of relevant knowledge, and the acquired knowledge was validated against the research questions. All authors independently determined quality assessment criteria, and crosschecking was conducted among reviewers, with at least three researchers independently extracting data, thus enhancing the reliability of the results.
8 Related work
The development of conversational recommender systems is significantly influenced by the findings from SLRs in various related research domains, each contributing to the collective understanding of user intent modeling. These SLRs are pivotal in gathering and analyzing data to interpret user needs within conversational interfaces.
In the field of Human–Computer Interaction, key SLRs conducted by de Barcelos Silva et al. ( 2020 ), Rapp et al. ( 2021 ), Iovine et al. ( 2023 ), Jiang et al. ( 2013 ), and Jindal et al. ( 2014 ) have systematically collected and analyzed data to understand how user-friendly interfaces can enhance user engagement and satisfaction in conversational systems, a core aspect of user intent modeling.
Similarly, in Conversational AI, the SLRs by Zaib et al. ( 2022 ) and Saka et al. ( 2023 ) have aggregated research findings focusing on simulating natural, human-like interactions, a key component in understanding and modeling user intent in conversational recommender systems.
The research in Conversational Search Systems, notably synthesized by Keyvan and Huang ( 2022 ), and Yuan et al. ( 2020 ), represents comprehensive reviews of the dynamics of user-system interaction for information retrieval. These studies align with user intent modeling by providing insights into how conversational systems can better parse and understand user queries.
For User Preference Extraction & Prioritization, SLRs by Pu et al. ( 2012 ), Liu et al. ( 2022 ), Zhang et al. ( 2019 ), and Hernández-Rubio et al. ( 2019 ) have methodically reviewed the literature to inform how conversational recommender systems can more accurately and contextually tailor their recommendations.
In the realm of Contextual Information Retrieval Systems, the systematic reviews by Tamine-Lechani et al. ( 2010 ), Chen et al. ( 2015 ), and Latifi et al. ( 2021 ) have contributed to understanding the impact of explicit and implicit user queries and contextual factors, crucial for refining user intent modeling in conversational systems.
Our SLR encapsulates these efforts, covering a total of 791 publications. We highlight the collective contribution of these SLRs to the field of user intent modeling in conversational recommender systems. Table 8 summarizes these efforts, offering a comparative analysis and showcasing the contributions of our study. Notably, our review reveals that while there is a substantial amount of literature on individual aspects of user intent modeling, a comprehensive, integrated approach in the form of an SLR is less common. The synthesis of findings from HCI, Conversational AI, Conversational Search Systems, User Preference Analytics, and Contextual Information Retrieval forms the foundation for advancing user intent modeling in conversational recommender systems (Dodeja et al. 2024 ; Zhang et al. 2024 ).
In Table 8 : Column 1 shows the authors of the studies, Column 2 indicates the year of publication, and Column 3 indicates the type of publications, which could be either academic or gray literature. Column 4 highlights the research methods that the publications employed. Column 5 signifies the main focus of the topic of the publications, and Column 6 indicates the Application or Domain that the publication conducted research on. Column 7 (# Reviewed publications) indicates the number of publications that each study reviewed in its research. Column 8 (Decision model) indicates whether a selected publication offered a decision model based on its findings from the data captured in the literature. Column 9 (Trend) shows if the selected study reported on the trends in employing models that it found. Column 10 (Datasets) shows if the researchers reported on the training or evaluation datasets. Column 11 (Model categories) indicates whether the publications reported on categories of the models (if they categorized the models). Column 12 (Model combinations) indicates if they reported on model combinations and integration. Column 13 (Feature/Model Mapping) shows if they offered the features that the models support. The subsequent four columns (Columns 14, 15, 16, and 17) show the number of quality attributes, features, evaluation measures, and models that each study reported. Columns 18, 19, 20, and 21 indicate how many quality attributes, features, evaluation measures, and models that the selected publications reported are in common with the ones in our study. Finally, Column 22 (Coverage (%)) shows the percentage of common concepts between our study and each selected publication.
Academic literature reviews dominate the selected studies, representing over 80 percent of the reviewed literature, aligning with our primary focus on academic sources. The research methods in these studies include SLR, Case Study, Survey, and Review. However, none of the reviewed SLRs employed case studies to evaluate their findings, relying solely on the SLR process. Our study adopts a more comprehensive approach by incorporating case studies into our research methods, offering a holistic perspective on decision-making in user intent modeling.
Our study places a significant emphasis on decision-making processes and decision models. Among the reviewed SLRs, only one paper (Pu et al. 2012 ) focused on this aspect, while our study introduces a decision model based on existing literature. This model serves as a valuable tool for research modelers to make informed decisions and identify suitable models or combinations for specific scenarios.
In terms of trends within models, four studies (Zaib et al. 2022 ; Pu et al. 2012 ; Chen et al. 2015 ; Zhang et al. 2019 ) (23.52%) reported on this aspect. Additionally, seven studies (Latifi et al. 2021 ; Yuan et al. 2020 ; Jindal et al. 2014 ; Hernández-Rubio et al. 2019 ; Zaib et al. 2022 ; Keyvan and Huang 2022 ; Pan et al. 2022 ) (41.17%) provided insights into open-access datasets, valuable for training or evaluating models.
Furthermore, our study categorizes models similar to eight other SLRs (de Barcelos Silva et al. 2020 ; Rapp et al. 2021 ; Pan et al. 2022 ; Liu et al. 2022 ; Zhang et al. 2019 ; Hernández-Rubio et al. 2019 ; Jindal et al. 2014 ; Yuan et al. 2020 ) (47.05%). However, only two publications (Hernández-Rubio et al. 2019 ; Yuan et al. 2020 ) (11.76%) reported on model combinations, suggesting a research gap in effective model integration.
9 Conclusion and future work
In this paper, the investigation focused on the decision-making process involved in selecting intent modeling approaches for conversational recommender systems. The primary aim was to tackle the challenge encountered by research modelers in determining the most effective model combination for developing intent modeling approaches.
To ensure the credibility and reliability of our findings, we conducted a systematic literature review and carried out two academic case studies, meticulously examining various dimensions of validity, including Construct Validity, Internal Validity, External Validity, and Conclusion Validity.
Drawing inspiration from the ISO/IEC/IEEE standard 42010 (ISO 2011 ), we devised a meta-model as the foundational framework for representing the decision-making process in intent modeling. By formulating comprehensive research questions, we ensured the inclusion of relevant studies and achieved an exhaustive coverage of pertinent publications.
Our study offers a holistic understanding of user intent modeling within the context of conversational recommender systems. The SLR analyzed over 13,000 papers from the last decade, identifying 59 distinct models and 74 commonly used features. These analyses provide valuable insights into the design and implementation of user intent modeling approaches, contributing significantly to the advancement of the field.
Building on the findings from the SLR, we proposed a decision model to guide researchers and practitioners in selecting the most suitable models for developing conversational recommender systems. The decision model considers essential factors such as model characteristics, evaluation measures, and dataset requirements, facilitating informed decision-making and enhancing the development of more effective and efficient intent modeling approaches.
We demonstrated the practical applicability of the decision model through two case studies, showcasing its usefulness in real-world scenarios. The decision model aids researchers in identifying initial model sets and considering essential quality attributes and functional requirements, streamlining the process and enhancing its reliability.
The significance of contributions in user intent modeling cannot be overstated in the current landscape of scientific research. Whether actively advancing the fundamentals or exploring its applications within their respective domains, scientists are undeniably conscious of this field. Amidst this crucial juncture, our study is essential as it consolidates the field’s foundations. We envision our research to become an integral component of essential literature for newcomers, fostering the promotion of this vital field and streamlining researchers’ efforts in selecting suitable models and techniques. By solidifying the understanding and relevance of User Intent Modeling, we aim to facilitate future advancements and innovation in this study area.
To ensure the longevity and up-to-dateness of the knowledge base constructed from our SLR, we are enthusiastic about taking the necessary steps to maintain its relevance and value for future researchers embarking on similar projects. We plan to establish a collaborative platform or repository, inviting researchers to contribute their latest findings and studies pertaining to the addressed research challenges. By fostering a community-driven approach, we aim to create an engaging environment that encourages regular and meaningful contributions. To streamline the process, we intend to develop user-friendly interfaces and implement effective content moderation to ensure the knowledge base’s scientific integrity.
Additionally, we aim to extend the current methodology by introducing more detailed criteria and context-specific frameworks for the selection and integration of intent modeling methods in conversational recommender systems. This involves developing nuanced frameworks that assess model compatibility and integration potential, tailored to address the unique challenges and requirements of specific domains and conversational scenarios. By deepening the analysis of how different models interact and complement each other in varying contexts, future research will not only refine the decision-making process for method selection but also enhance the overall effectiveness and user-centricity of conversational recommender systems.
Moreover, we are excited to explore implementing an automated data crawling mechanism, periodically and systematically searching reputable literature sources and academic databases. This technology will enable seamless integration of the latest research into the knowledge base. Additionally, we are committed to maintaining a record of changes and updates to the knowledge base, including precise timestamps and new information sources. This transparent documentation will empower future researchers to follow the knowledge base’s evolution and confidently leverage it for their specific research needs. By embracing these proactive measures, we envision establishing a continuously updated and robust knowledge base that serves as a valuable resource for researchers in the dynamic domain of user intent modeling and recommender systems.
https://www.core.edu.au .
https://www.scimagojr.com/ .
For access to the complete lists of datasets, quality attributes and evaluation measures, models, and features related to this study, please refer to the supplementary materials available on Mendeley Data (Farshidi 2024 ).
https://www.tensorflow.org/ .
https://scikit-learn.org/ .
Agarwal, N., Sikka, G., Awasthi, L.K.: Evaluation of web service clustering using Dirichlet multinomial mixture model based approach for dimensionality reduction in service representation. Inf. Process. Manag. 57 (4), 102238 (2020)
Article Google Scholar
Allamanis, M., Barr, E.T., Devanbu, P., Sutton, C.: A survey of machine learning for big code and naturalness. ACM Computi. Surv. (CSUR) 51 (4), 1–37 (2018)
Google Scholar
Amershi, S., Begel, A., Bird, C., DeLine, R., Gall, H., Kamar, E., Nagappan, N., Nushi, B., Zimmermann, T.: Software engineering for machine learning: A case study. In: 2019 IEEE/ACM 41st International Conference on Software Engineering: Software Engineering in Practice (ICSE-SEIP), pp. 291–300. IEEE (2019)
Ashkan, A., Clarke, C.L., Agichtein, E., Guo, Q.: Classifying and characterizing query intent. In: Advances in Information Retrieval: 31th European Conference on IR Research, ECIR 2009, Toulouse, France, April 6–9, 2009. Proceedings 31, pp. 578–586. Springer (2009)
Baykan, E., Henzinger, M., Marian, L., Weber, I.: A comprehensive study of features and algorithms for URL-based topic classification. ACM Trans. Web (TWEB) 5 (3), 1–29 (2011)
Beel, J., Gipp, B., Langer, S., Breitinger, C.: Paper recommender systems: a literature survey. Int. J. Digit. Libr. 17 , 305–338 (2016)
Beemer, J., Spoon, K., He, L., Fan, J., Levine, R.A.: Ensemble learning for estimating individualized treatment effects in student success studies. Int. J. Artif. Intell. Educ. 28 , 315–335 (2018)
Bhaskaran, S., Santhi, B.: An efficient personalized trust based hybrid recommendation (TBHR) strategy for e-learning system in cloud computing. Clust. Comput. 22 , 1137–1149 (2019)
Bifet, A., Holmes, G., Pfahringer, B., Kirkby, R., Gavalda, R.: New ensemble methods for evolving data streams. In: Proceedings of the 15th ACM SIGKDD International Conference on Knowledge Discovery and Data Mining, pp. 139–148 (2009)
Bigi, B.: Using Kullback–Leibler distance for text categorization. In: European Conference on Information Retrieval, pp. 305–319. Springer (2003)
Binkley, D., Lawrie, D., Morrell, C.: The need for software specific natural language techniques. Empir. Softw. Eng. 23 , 2398–2425 (2018)
Cai, Y., Lau, R.Y., Liao, S.S., Li, C., Leung, H.-F., Ma, L.C.: Object typicality for effective web of things recommendations. Decis. Support Syst. 63 , 52–63 (2014)
Cao, Y., Li, S., Liu, Y., Yan, Z., Dai, Y., Yu, P.S., Sun, L.: A comprehensive survey of AI-generated content (AIGC): a history of generative AI from GAN to chatGPT. arXiv preprint arXiv:2303.04226 (2023)
Caprara, A., Toth, P., Fischetti, M.: Algorithms for the set covering problem. Ann. Oper. Res. 98 (1–4), 353–371 (2000)
Article MathSciNet Google Scholar
Carmel, D., Chang, Y., Deng, H., Nie, J.-Y.: Future directions of query understanding. Query Understanding for Search Engines, pp. 205–224 (2020)
Carvallo, A., Parra, D., Lobel, H., Soto, A.: Automatic document screening of medical literature using word and text embeddings in an active learning setting. Scientometrics 125 , 3047–3084 (2020)
Chen, Y., Liu, Z., Li, J., McAuley, J., Xiong, C.: Intent contrastive learning for sequential recommendation. In: Proceedings of the ACM Web Conference 2022, pp. 2172–2182 (2022)
Chen, L., Wang, Y., Yu, Q., Zheng, Z., Wu, J.: WT-LDA: user tagging augmented LDA for web service clustering. In: Service-Oriented Computing: 11th International Conference, ICSOC 2013, Berlin, Germany, December 2–5, 2013, Proceedings 11, pp. 162–176. Springer (2013)
Chen, T., Wong, R.C.-W.: Handling information loss of graph neural networks for session-based recommendation. In: Proceedings of the 26th ACM SIGKDD International Conference on Knowledge Discovery & Data Mining, pp. 1172–1180 (2020)
Chen, L., Chen, G., Wang, F.: Recommender systems based on user reviews: the state of the art. User Model. User-Adap. Inter. 25 , 99–154 (2015)
Colace, F., De Santo, M., Greco, L., Moscato, V., Picariello, A.: A collaborative user-centered framework for recommending items in online social networks. Comput. Hum. Behav. 51 , 694–704 (2015)
Cremonesi, P., Garzotto, F., Negro, S., Papadopoulos, A.V., Turrin, R.: Looking for “good” recommendations: a comparative evaluation of recommender systems. In: Human-Computer Interaction–INTERACT 2011: 13th IFIP TC 13 International Conference, Lisbon, Portugal, September 5–9, 2011, Proceedings, Part III 13, pp. 152–168. Springer (2011)
Da’u, A., Salim, N.: Sentiment-aware deep recommender system with neural attention networks. IEEE Access 7 , 45472–45484 (2019). https://doi.org/10.1109/ACCESS.2019.2907729
de Barcelos Silva, A., Gomes, M.M., da Costa, C.A., da Rosa Righi, R., Barbosa, J.L.V., Pessin, G., De Doncker, G., Federizzi, G.: Intelligent personal assistants: a systematic literature review. Expert Syst. Appl. 147 , 113193 (2020)
Devlin, J., Chang, M.-W., Lee, K., Toutanova, K.: Bert: pre-training of deep bidirectional transformers for language understanding. arXiv preprint arXiv:1810.04805 (2018)
Ding, X., Liu, T., Duan, J., Nie, J.-Y.: Mining user consumption intention from social media using domain adaptive convolutional neural network. In: Proceedings of the AAAI Conference on Artificial Intelligence, vol. 29 (2015)
Ding, H., Liu, Q., Hu, G.: TDTMF: a recommendation model based on user temporal interest drift and latent review topic evolution with regularization factor. Inf. Process. Manag. 59 (5), 103037 (2022)
Dodeja, L., Tambwekar, P., Hedlund-Botti, E., Gombolay, M.: Towards the design of user-centric strategy recommendation systems for collaborative human-AI tasks. Int. J. Hum Comput Stud. 184 , 103216 (2024)
Doumpos, M., Grigoroudis, E.: Multicriteria Decision Aid and Artificial Intelligence. Whiley, UK (2013)
Book Google Scholar
Elfaik, H., et al.: Leveraging feature-level fusion representations and attentional bidirectional RNN-CNN deep models for Arabic affect analysis on Twitter. J. King Saud Univ. Comput. Inf. Sci. 35 (1), 462–482 (2023)
Fan, L., Li, Q., Liu, B., Wu, X.-M., Zhang, X., Lv, F., Lin, G., Li, S., Jin, T., Yang, K.: Modeling user behavior with graph convolution for personalized product search. In: Proceedings of the ACM Web Conference 2022, pp. 203–212 (2022)
Farshidi, S., Kwantes, I.B., Jansen, S.: Business process modeling language selection for research modelers. Softw Syst Model 1–26 (2023)
Farshidi, S.: Multi-criteria decision-making in software production. PhD thesis, Utrecht University (2020)
Farshidi, S.: Understanding user intent: a systematic literature review of modeling techniques. Mendeley Data (2024). https://doi.org/10.17632/zcbh9r37rc.1
Farshidi, S., Jansen, S., van der Werf, J.M.: Capturing software architecture knowledge for pattern-driven design. J. Syst. Softw. 169 , 110714 (2020)
Fitzgerald, B., Stol, K.-J.: Continuous software engineering and beyond: trends and challenges. In: Proceedings of the 1st International Workshop on Rapid Continuous Software Engineering, pp. 1–9 (2014)
Fitzgerald, D.R., Mohammed, S., Kremer, G.O.: Differences in the way we decide: the effect of decision style diversity on process conflict in design teams. Pers. Individ. Differ. 104 , 339–344 (2017)
Gao, C., Lam, W.: Search clarification selection via query-intent-clarification graph attention. In: European Conference on Information Retrieval, pp. 230–243. Springer (2022)
Garcia, K., Berton, L.: Topic detection and sentiment analysis in twitter content related to COVID-19 from Brazil and the USA. Appl. Soft Comput. 101 , 107057 (2021)
Garg, R.: Parametric selection of software reliability growth models using multi-criteria decision-making approach. Int. J. Reliab. Saf. 13 (4), 291–309 (2019)
Garg, R.: MCDM-based parametric selection of cloud deployment models for an academic organization. IEEE Trans. Cloud Comput. 10 , 863–871 (2020)
Garg, R., Sharma, R., Sharma, K.: MCDM based evaluation and ranking of commercial off-the-shelf using fuzzy based matrix method. Decis. Sci. Lett. 6 (2), 117–136 (2017)
Garg, R., Kumar, R., Garg, S.: MADM-based parametric selection and ranking of E-learning websites using fuzzy COPRAS. IEEE Trans. Educ. 62 (1), 11–18 (2018)
Gozuacik, N., Sakar, C.O., Ozcan, S.: Technological forecasting based on estimation of word embedding matrix using LSTM networks. Technol. Forecast. Soc. Change 191 , 122520 (2023)
Gu, Y., Zhao, B., Hardtke, D., Sun, Y.: Learning global term weights for content-based recommender systems. In: Proceedings of the 25th International Conference on World Wide Web. WWW ’16, pp. 391–400. International World Wide Web Conferences Steering Committee, Republic and Canton of Geneva, CHE (2016). https://doi.org/10.1145/2872427.2883069
Gunawan, D., Sembiring, C., Budiman, M.A.: The implementation of cosine similarity to calculate text relevance between two documents. In: Journal of Physics: Conference Series, vol. 978, p. 012120. IOP Publishing (2018)
Guo, L., Hua, L., Jia, R., Fang, F., Zhao, B., Cui, B.: EdgeDIPN: a unified deep intent prediction network deployed at the edge. Proc. VLDB Endowm. 14 (3), 320–328 (2020)
Haefliger, S., Von Krogh, G., Spaeth, S.: Code reuse in open source software. Manag. Sci. 54 (1), 180–193 (2008)
Hashemi, S.H., Williams, K., El Kholy, A., Zitouni, I., Crook, P.A.: Measuring user satisfaction on smart speaker intelligent assistants using intent sensitive query embeddings. In: Proceedings of the 27th ACM International Conference on Information and Knowledge Management, pp. 1183–1192 (2018)
Hashemi, H., Zamani, H., Croft, W.B.: Guided transformer: leveraging multiple external sources for representation learning in conversational search. In: Proceedings of the 43rd International ACM SIGIR Conference on Research and Development in Information Retrieval, pp. 1131–1140 (2020)
Hernández-Rubio, M., Cantador, I., Bellogín, A.: A comparative analysis of recommender systems based on item aspect opinions extracted from user reviews. User Model. User-Adap. Inter. 29 (2), 381–441 (2019)
Hidasi, B., Karatzoglou, A.: Recurrent neural networks with top-k gains for session-based recommendations. In: Proceedings of the 27th ACM International Conference on Information and Knowledge Management, pp. 843–852 (2018)
Hill, C., Bellamy, R., Erickson, T., Burnett, M.: Trials and tribulations of developers of intelligent systems: a field study. In: 2016 IEEE Symposium on Visual Languages and Human-Centric Computing (VL/HCC), pp. 162–170. IEEE (2016)
Hu, Y., Da, Q., Zeng, A., Yu, Y., Xu, Y.: Reinforcement learning to rank in e-commerce search engine: formalization, analysis, and application. In: Proceedings of the 24th ACM SIGKDD International Conference on Knowledge Discovery & Data Mining. KDD ’18, pp. 368–377. Association for Computing Machinery, New York (2018). https://doi.org/10.1145/3219819.3219846
Hu, Z., Zhang, Z., Yang, H., Chen, Q., Zuo, D.: A deep learning approach for predicting the quality of online health expert question-answering services. J. Biomed. Inform. 71 , 241–253 (2017)
Huang, Q., Xia, X., Lo, D., Murphy, G.C.: Automating intention mining. IEEE Trans. Softw. Eng. 46 (10), 1098–1119 (2018)
Iovine, A., Narducci, F., Musto, C., de Gemmis, M., Semeraro, G.: Virtual customer assistants in finance: from state of the art and practices to design guidelines. Comput. Sci. Rev. 47 , 100534 (2023)
ISO: IEC/IEEE systems and software engineering: Architecture description. ISO/IEC/IEEE 42010: 2011 (E)(Revision of ISO/IEC 42010: 2007 and IEEE Std 1471-2000) (2011)
Ittoo, A., van den Bosch, A., et al.: Text analytics in industry: challenges, desiderata and trends. Comput. Ind. 78 , 96–107 (2016)
Izadi, M., Akbari, K., Heydarnoori, A.: Predicting the objective and priority of issue reports in software repositories. Empir. Softw. Eng. 27 (2), 50 (2022)
Jain, S., Grover, A., Thakur, P.S., Choudhary, S.K.: Trends, problems and solutions of recommender system. In: International Conference on Computing, Communication & Automation, pp. 955–958 (2015)
Jansen, S.: Applied multi-case research in a mixed-method research project: customer configuration updating improvement. In: Information Systems Research Methods, Epistemology, and Applications, pp. 120–139. IGI Global (2009)
Jiang, D., Pei, J., Li, H.: Mining search and browse logs for web search: a survey. ACM Trans. Intell. Syst. Technol. (TIST) 4 (4), 1–37 (2013)
Jindal, V., Bawa, S., Batra, S.: A review of ranking approaches for semantic search on web. Inform. Process. Manag. 50 (2), 416–425 (2014)
Johnson, R.B., Onwuegbuzie, A.J.: Mixed methods research: a research paradigm whose time has come. Educ. Res. 33 (7), 14–26 (2004)
Jordan, M.I., Mitchell, T.M.: Machine learning: trends, perspectives, and prospects. Science 349 (6245), 255–260 (2015)
Kaptein, R., Kamps, J.: Exploiting the category structure of wikipedia for entity ranking. Artif. Intell. 194 , 111–129 (2013)
Kaufmann, L., Kreft, S., Ehrgott, M., Reimann, F.: Rationality in supplier selection decisions: the effect of the buyer’s national task environment. J. Purch. Supply Manag. 18 (2), 76–91 (2012)
Keyvan, K., Huang, J.X.: How to approach ambiguous queries in conversational search: a survey of techniques, approaches, tools, and challenges. ACM Comput. Surv. 55 (6), 1–40 (2022)
Khilji, A.F.U.R., Sinha, U., Singh, P., Ali, A., Dadure, P., Manna, R., Pakray, P.: Multimodal recipe recommendation system using deep learning and rule-based approach. SN Comput. Sci. 4 (4), 421 (2023)
Kilgarriff, A., Baisa, V., Bušta, J., Jakubíček, M., Kovář, V., Michelfeit, J., Rychlỳ, P., Suchomel, V.: The sketch engine: ten years on. Lexicography 1 (1), 7–36 (2014)
Kim, D., Park, C., Oh, J., Yu, H.: Deep hybrid recommender systems via exploiting document context and statistics of items. Inf. Sci. 417 , 72–87 (2017)
Kitchenham, B., Brereton, O.P., Budgen, D., Turner, M., Bailey, J., Linkman, S.: Systematic literature reviews in software engineering-a systematic literature review. Inf. Softw. Technol. 51 (1), 7–15 (2009)
Konishi, T., Ohwa, T., Fujita, S., Ikeda, K., Hayashi, K.: Extracting search query patterns via the pairwise coupled topic model. In: Proceedings of the Ninth ACM International Conference on Web Search and Data Mining, pp. 655–664 (2016)
Kuwajima, H., Yasuoka, H., Nakae, T.: Engineering problems in machine learning systems. Mach. Learn. 109 (5), 1103–1126 (2020)
Larson, S., Mahendran, A., Peper, J.J., Clarke, C., Lee, A., Hill, P., Kummerfeld, J.K., Leach, K., Laurenzano, M.A., Tang, L. et al.: An evaluation dataset for intent classification and out-of-scope prediction. arXiv preprint arXiv:1909.02027 (2019)
Latifi, S., Mauro, N., Jannach, D.: Session-aware recommendation: a surprising quest for the state-of-the-art. Inf. Sci. 573 , 291–315 (2021)
Li, L., Deng, H., Dong, A., Chang, Y., Zha, H.: Identifying and labeling search tasks via query-based hawkes processes. In: Proceedings of the 20th ACM SIGKDD International Conference on Knowledge Discovery and Data Mining, pp. 731–740 (2014)
Lin, H., Liu, G., Li, F., Zuo, Y.: Where to go? predicting next location in IoT environment. Front. Comput. Sci. 15 , 1–13 (2021)
Liu, Z., Chen, H., Sun, F., Xie, X., Gao, J., Ding, B., Shen, Y.: Intent preference decoupling for user representation on online recommender system. In: Proceedings of the Twenty-Ninth International Conference on International Joint Conferences on Artificial Intelligence, pp. 2575–2582 (2021)
Liu, J., Dou, Z., Zhu, Q., Wen, J.-R.: A category-aware multi-interest model for personalized product search. In: Proceedings of the ACM Web Conference 2022, pp. 360–368 (2022)
Liu, P., Liao, D., Wang, J., Wu, Y., Li, G., Xia, S.-T., Xu, J.: Multi-task ranking with user behaviors for text-video search. In: Companion Proceedings of the Web Conference 2022, pp. 126–130 (2022)
Liu, P., Zhang, L., Gulla, J.A.: Dynamic attention-based explainable recommendation with textual and visual fusion. Inf. Process. Manag. 57 (6), 102099 (2020)
Liu, T., Wu, Q., Chang, L., Gu, T.: A review of deep learning-based recommender system in e-learning environments. Artif. Intell. Rev. 55 (8), 5953–5980 (2022)
Ludewig, M., Jannach, D.: Evaluation of session-based recommendation algorithms. User Model. User-Adap. Inter. 28 , 331–390 (2018)
Majumder, M.: Multi criteria decision making. In: Impact of Urbanization on Water Shortage in Face of Climatic Aberrations, pp. 35–47. Springer (2015)
Mandayam Comar, P., Sengamedu, S.H.: Intent based relevance estimation from click logs. In: Proceedings of the 2017 ACM on Conference on Information and Knowledge Management, pp. 59–66 (2017)
Manzoor, A., Jannach, D.: Towards retrieval-based conversational recommendation. Inf. Syst. 109 , 102083 (2022). https://doi.org/10.1016/j.is.2022.102083
Mao, M., Lu, J., Han, J., Zhang, G.: Multiobjective e-commerce recommendations based on hypergraph ranking. Inf. Sci. 471 , 269–287 (2019)
Musto, C., Narducci, F., Lops, P., de Gemmis, M., Semeraro, G.: Linked open data-based explanations for transparent recommender systems. Int. J. Hum. Comput. Stud. 121 , 93–107 (2019)
Ni, X., Lu, Y., Quan, X., Wenyin, L., Hua, B.: User interest modeling and its application for question recommendation in user-interactive question answering systems. Inf. Process. Manag. 48 (2), 218–233 (2012)
Okoli, C., Schabram, K.: A guide to conducting a systematic literature review of information systems research (2015)
Oulasvirta, A., Blom, J.: Motivations in personalisation behaviour. Interact. Comput. 20 (1), 1–16 (2008)
Pan, R., Bagherzadeh, M., Ghaleb, T.A., Briand, L.: Test case selection and prioritization using machine learning: a systematic literature review. Empir. Softw. Eng. 27 (2), 29 (2022)
Papadimitriou, A., Symeonidis, P., Manolopoulos, Y.: A generalized taxonomy of explanations styles for traditional and social recommender systems. Data Min. Knowl. Discov. 24 , 555–583 (2012)
Park, C., Kim, D., Yang, M.-C., Lee, J.-T., Yu, H.: Click-aware purchase prediction with push at the top. Inf. Sci. 521 , 350–364 (2020)
Paul, H., Nikolaev, A.: Fake review detection on online e-commerce platforms: a systematic literature review. Data Min. Knowl. Discov. 35 (5), 1830–1881 (2021)
Penha, G., Hauff, C.: What does BERT know about books, movies and music? probing BERT for conversational recommendation. In: Proceedings of the 14th ACM Conference on Recommender Systems, pp. 388–397 (2020)
Phan, X.-H., Nguyen, C.-T., Le, D.-T., Nguyen, L.-M., Horiguchi, S., Ha, Q.-T.: A hidden topic-based framework toward building applications with short web documents. IEEE Trans. Knowl. Data Eng. 23 (7), 961–976 (2010)
Pi, Q., Bian, W., Zhou, G., Zhu, X., Gai, K.: Practice on long sequential user behavior modeling for click-through rate prediction. In: Proceedings of the 25th ACM SIGKDD International Conference on Knowledge Discovery & Data Mining, pp. 2671–2679 (2019)
Portugal, I., Alencar, P., Cowan, D.: The use of machine learning algorithms in recommender systems: a systematic review. Expert Syst. Appl. 97 , 205–227 (2018)
Pradhan, T., Kumar, P., Pal, S.: CLAVER: an integrated framework of convolutional layer, bidirectional LSTM with attention mechanism based scholarly venue recommendation. Inf. Sci. 559 , 212–235 (2021)
Pu, P., Chen, L., Hu, R.: Evaluating recommender systems from the user’s perspective: survey of the state of the art. User Model. User-Adap. Inter. 22 (4), 317–355 (2012)
Qu, Y., Cai, H., Ren, K., Zhang, W., Yu, Y., Wen, Y., Wang, J.: Product-based neural networks for user response prediction. In: 2016 IEEE 16th International Conference on Data Mining (ICDM), pp. 1149–1154 (2016). https://doi.org/10.1109/ICDM.2016.0151
Qu, Y., Cai, H., Ren, K., Zhang, W., Yu, Y., Wen, Y., Wang, J.: Product-based neural networks for user response prediction. In: 2016 IEEE 16th International Conference on Data Mining (ICDM), pp. 1149–1154. IEEE (2016)
Qu, C., Yang, L., Croft, W.B., Zhang, Y., Trippas, J.R., Qiu, M.: User intent prediction in information-seeking conversations. In: Proceedings of the 2019 Conference on Human Information Interaction and Retrieval, pp. 25–33 (2019)
Rapp, A., Curti, L., Boldi, A.: The human side of human-chatbot interaction: a systematic literature review of ten years of research on text-based chatbots. Int. J. Hum. Comput. Stud. 151 , 102630 (2021)
Ribeiro, M.T., Singh, S., Guestrin, C.: “why should i trust you?” explaining the predictions of any classifier. In: Proceedings of the 22nd ACM SIGKDD International Conference on Knowledge Discovery and Data Mining, pp. 1135–1144 (2016)
Ricci, F., Rokach, L., Shapira, B.: Recommender systems: introduction and challenges. Recom. Syst. Handb. 1–34 (2015)
Robertson, S., Zaragoza, H., Taylor, M.: Simple bm25 extension to multiple weighted fields. In: Proceedings of the Thirteenth ACM International Conference on Information and Knowledge Management, pp. 42–49 (2004)
Rus, I., Halling, M., Biffl, S.: Supporting decision-making in software engineering with process simulation and empirical studies. Int. J. Softw. Eng. Knowl. Eng. 13 (05), 531–545 (2003)
Sagi, O., Rokach, L.: Ensemble learning: a survey. Wiley Interdiscip. Rev. Data Min. Knowl. Discov. 8 (4), 1249 (2018)
Saka, A.B., Oyedele, L.O., Akanbi, L.A., Ganiyu, S.A., Chan, D.W., Bello, S.A.: Conversational artificial intelligence in the AEC industry: a review of present status, challenges and opportunities. Adv. Eng. Inform. 55 , 101869 (2023)
Salle, A., Malmasi, S., Rokhlenko, O., Agichtein, E.: COSEARCHER: studying the effectiveness of conversational search refinement and clarification through user simulation. Inf. Retr. J. 25 (2), 209–238 (2022)
Sandhya, Garg, R., Kumar, R.: Computational MADM evaluation and ranking of cloud service providers using distance-based approach. Int J Inf Decis. Sci. 10 (3), 222–234 (2018)
Sarker, I.H.: Machine learning: algorithms, real-world applications and research directions. SN Comput. Sci. 2 (3), 160 (2021)
Schlaefer, N., Chu-Carroll, J., Nyberg, E., Fan, J., Zadrozny, W., Ferrucci, D.: Statistical source expansion for question answering. In: Proceedings of the 20th ACM International Conference on Information and Knowledge Management, pp. 345–354 (2011)
Singh, A., Thakur, N., Sharma, A.: A review of supervised machine learning algorithms. In: 2016 3rd International Conference on Computing for Sustainable Global Development (INDIACom), pp. 1310–1315. IEEE (2016)
Srivastava, M., Nushi, B., Kamar, E., Shah, S., Horvitz, E.: An empirical analysis of backward compatibility in machine learning systems. In: Proceedings of the 26th ACM SIGKDD International Conference on Knowledge Discovery & Data Mining, pp. 3272–3280 (2020)
Sun, C., Gan, C., Nevatia, R.: Automatic concept discovery from parallel text and visual corpora. In: Proceedings of the IEEE International Conference on Computer Vision, pp. 2596–2604 (2015)
Takács, G., Tikk, D.: Alternating least squares for personalized ranking. In: Proceedings of the Sixth ACM Conference on Recommender Systems, pp. 83–90 (2012)
Tamine-Lechani, L., Boughanem, M., Daoud, M.: Evaluation of contextual information retrieval effectiveness: overview of issues and research. Knowl. Inf. Syst. 24 , 1–34 (2010)
Tang, J., Yao, L., Zhang, D., Zhang, J.: A combination approach to web user profiling. ACM Trans. Knowl. Discov. Data (TKDD) 5 (1), 1–44 (2010)
Tanjim, M.M., Su, C., Benjamin, E., Hu, D., Hong, L., McAuley, J.: Attentive sequential models of latent intent for next item recommendation. In: Proceedings of The Web Conference 2020, pp. 2528–2534 (2020)
Tanjim, M.M., Su, C., Benjamin, E., Hu, D., Hong, L., McAuley, J.: Attentive sequential models of latent intent for next item recommendation. In: Proceedings of The Web Conference 2020. WWW ’20, pp. 2528–2534. Association for Computing Machinery, New York (2020). https://doi.org/10.1145/3366423.3380002
Teevan, J., Dumais, S.T., Liebling, D.J.: To personalize or not to personalize: modeling queries with variation in user intent. In: Proceedings of the 31st Annual International ACM SIGIR Conference on Research and Development in Information Retrieval, pp. 163–170 (2008)
Telikani, A., Tahmassebi, A., Banzhaf, W., Gandomi, A.H.: Evolutionary machine learning: a survey. ACM Comput. Surv. (CSUR) 54 (8), 1–35 (2021)
Vayansky, I., Kumar, S.A.: A review of topic modeling methods. Inf. Syst. 94 , 101582 (2020)
Venkateswara Rao, P., Kumar, A.S.: The societal communication of the Q &A community on topic modeling. J. Supercomput. 78 (1), 1117–1143 (2022)
von Rueden, L., Mayer, S., Sifa, R., Bauckhage, C., Garcke, J.: Combining machine learning and simulation to a hybrid modelling approach: current and future directions. In: Advances in Intelligent Data Analysis XVIII: 18th International Symposium on Intelligent Data Analysis, IDA 2020, Konstanz, Germany, April 27–29, 2020, Proceedings 18, pp. 548–560. Springer (2020)
Wang, J., Ding, K., Hong, L., Liu, H., Caverlee, J.: Next-item recommendation with sequential hypergraphs. In: Proceedings of the 43rd International ACM SIGIR Conference on Research and Development in Information Retrieval, pp. 1101–1110 (2020)
Wang, W., Hosseini, S., Awadallah, A.H., Bennett, P.N., Quirk, C.: Context-aware intent identification in email conversations. In: Proceedings of the 42nd International ACM SIGIR Conference on Research and Development in Information Retrieval, pp. 585–594 (2019)
Wang, Y., Wang, S., Li, Y., Dou, D.: Recognizing medical search query intent by few-shot learning. In: Proceedings of the 45th International ACM SIGIR Conference on Research and Development in Information Retrieval, pp. 502–512 (2022)
Wang, T., Lin, Q.: Hybrid predictive models: when an interpretable model collaborates with a black-box model. J. Mach. Learn. Res. 22 (1), 6085–6122 (2021)
MathSciNet Google Scholar
Wang, H.-C., Jhou, H.-T., Tsai, Y.-S.: Adapting topic map and social influence to the personalized hybrid recommender system. Inf. Sci. 575 , 762–778 (2021)
Wang, X., Li, Q., Yu, D., Cui, P., Wang, Z., Xu, G.: Causal disentanglement for semantics-aware intent learning in recommendation. IEEE Trans. Knowl. Data Eng. (2022). https://doi.org/10.1109/TKDE.2022.3159802
Weismayer, C., Pezenka, I.: Identifying emerging research fields: a longitudinal latent semantic keyword analysis. Scientometrics 113 (3), 1757–1785 (2017)
White, R.W., Chu, W., Hassan, A., He, X., Song, Y., Wang, H.: Enhancing personalized search by mining and modeling task behavior. In: Proceedings of the 22nd International Conference on World Wide Web, pp. 1411–1420 (2013)
Wu, L., Quan, C., Li, C., Wang, Q., Zheng, B., Luo, X.: A context-aware user-item representation learning for item recommendation. ACM Trans. Inf. Syst. (TOIS) 37 (2), 1–29 (2019)
Wu, Z., Liang, J., Zhang, Z., Lei, J.: Exploration of text matching methods in Chinese disease Q &A systems: a method using ensemble based on BERT and boosted tree models. J. Biomed. Inform. 115 , 103683 (2021)
Xia, C., Zhang, C., Yan, X., Chang, Y., Yu, P.S.: Zero-shot user intent detection via capsule neural networks. arXiv preprint arXiv:1809.00385 (2018)
Xiao, Y., Watson, M.: Guidance on conducting a systematic literature review. J. Plan. Educ. Res. 39 (1), 93–112 (2019)
Xu, P., Sugano, Y., Bulling, A.: Spatio-temporal modeling and prediction of visual attention in graphical user interfaces. In: Proceedings of the 2016 CHI Conference on Human Factors in Computing Systems, pp. 3299–3310 (2016)
Xu, L., Brinkkemper, S.: Concepts of product software. Eur. J. Inf. Syst. 16 (5), 531–541 (2007)
Xu, Z., Chen, L., Chen, G.: Topic based context-aware travel recommendation method exploiting geotagged photos. Neurocomputing 155 , 99–107 (2015)
Xu, H., Ding, W., Shen, W., Wang, J., Yang, Z.: Deep convolutional recurrent model for region recommendation with spatial and temporal contexts. Ad Hoc Netw. 129 , 102545 (2022)
Xu, H., Ding, W., Shen, W., Wang, J., Yang, Z.: Deep convolutional recurrent model for region recommendation with spatial and temporal contexts. Ad Hoc Netw. 129 , 102545 (2022). https://doi.org/10.1016/j.adhoc.2021.102545
Yadav, N., Pal, S., Singh, A.K., Singh, K.: Clus-DR: cluster-based pre-trained model for diverse recommendation generation. J. King Saud Univ. Comput. Inf. Sci. 34 (8), 6385–6399 (2022)
Yao, S., Tan, J., Chen, X., Zhang, J., Zeng, X., Yang, K.: ReprBERT: distilling BERT to an efficient representation-based relevance model for e-commerce. In: Proceedings of the 28th ACM SIGKDD Conference on Knowledge Discovery and Data Mining, pp. 4363–4371 (2022)
Yao, Y., Zhao, W.X., Wang, Y., Tong, H., Xu, F., Lu, J.: Version-aware rating prediction for mobile app recommendation. ACM Trans. Inf. Syst. (TOIS) 35 (4), 1–33 (2017)
Ye, Q., Wang, F., Li, B.: Starrysky: A practical system to track millions of high-precision query intents. In: Proceedings of the 25th International Conference Companion on World Wide Web. WWW ’16 Companion, pp. 961–966. International World Wide Web Conferences Steering Committee, Republic and Canton of Geneva, CHE (2016). https://doi.org/10.1145/2872518.2890588
Yengikand, A.K., Meghdadi, M., Ahmadian, S.: DHSIRS: a novel deep hybrid side information-based recommender system. Multimed Tools Appl 1–27 (2023)
Yin, R.K.: Case Study Research: Design and Methods, vol. 5. Sage, Thousand Oaks (2009)
Yin, R.K.: Case Study Research and Applications: Design and Methods. Sage publications, New York (2017)
Yu, Z., Lian, J., Mahmoody, A., Liu, G., Xie, X.: Adaptive user modeling with long and short-term preferences for personalized recommendation. In: IJCAI, pp. 4213–4219 (2019)
Yu, J., Zhu, T.: Combining long-term and short-term user interest for personalized hashtag recommendation. Front. Comput. Sci. 9 , 608–622 (2015)
Yu, S., Liu, J., Yang, Z., Chen, Z., Jiang, H., Tolba, A., Xia, F.: Pave: Personalized academic venue recommendation exploiting co-publication networks. J. Netw. Comput. Appl. 104 , 38–47 (2018)
Yu, B., Zhang, R., Chen, W., Fang, J.: Graph neural network based model for multi-behavior session-based recommendation. GeoInformatica 26 (2), 429–447 (2022)
Yuan, S., Zhang, Y., Tang, J., Hall, W., Cabotà, J.B.: Expert finding in community question answering: a review. Artif. Intell. Rev. 53 , 843–874 (2020)
Zaib, M., Zhang, W.E., Sheng, Q.Z., Mahmood, A., Zhang, Y.: Conversational question answering: a survey. Knowl. Inf. Syst. 64 (12), 3151–3195 (2022)
Zhang, Y., Chen, X., Ai, Q., Yang, L., Croft, W.B.: Towards conversational search and recommendation: System ask, user respond. In: Proceedings of the 27th ACM International Conference on Information and Knowledge Management, pp. 177–186 (2018)
Zhang, C., Fan, W., Du, N., Yu, P.S.: Mining user intentions from medical queries: A neural network based heterogeneous jointly modeling approach. In: Proceedings of the 25th International Conference on World Wide Web, pp. 1373–1384 (2016)
Zhang, H., Xu, H., Lin, T.-E., Lyu, R.: Discovering new intents with deep aligned clustering. In: Proceedings of the AAAI Conference on Artificial Intelligence, vol. 35, pp. 14365–14373 (2021)
Zhang, Y., Yin, H., Huang, Z., Du, X., Yang, G., Lian, D.: Discrete deep learning for fast content-aware recommendation. In: Proceedings of the Eleventh ACM International Conference on Web Search and Data Mining, pp. 717–726 (2018)
Zhang, H., Zhong, G.: Improving short text classification by learning vector representations of both words and hidden topics. Knowl.-Based Syst. 102 , 76–86 (2016)
Zhang, H., Babar, M.A., Tell, P.: Identifying relevant studies in software engineering. Inf. Softw. Technol. 53 (6), 625–637 (2011)
Zhang, S., Yao, L., Sun, A., Tay, Y.: Deep learning based recommender system: a survey and new perspectives. ACM Comput Surv. (CSUR) 52 (1), 1–38 (2019)
Zhang, C., Huang, X., An, J., Zou, S.: Improving conversational recommender systems via multi-preference modeling and knowledge-enhanced. Knowl. Based Syst. 286 , 111361 (2024)
Zhou, X., Jin, Y., Zhang, H., Li, S., Huang, X.: A map of threats to validity of systematic literature reviews in software engineering. In: 2016 23rd Asia-Pacific Software Engineering Conference (APSEC), pp. 153–160. IEEE (2016)
Zhou, K., Zhao, W.X., Wang, H., Wang, S., Zhang, F., Wang, Z., Wen, J.-R.: Leveraging historical interaction data for improving conversational recommender system. In: Proceedings of the 29th ACM International Conference on Information & Knowledge Management, pp. 2349–2352 (2020)
Zhou, X., Qin, D., Chen, L., Zhang, Y.: Real-time context-aware social media recommendation. VLDB J. 28 , 197–219 (2019)
Zou, J., Kanoulas, E., Ren, P., Ren, Z., Sun, A., Long, C.: Improving conversational recommender systems via transformer-based sequential modelling. In: Proceedings of the 45th International ACM SIGIR Conference on Research and Development in Information Retrieval, pp. 2319–2324 (2022)
Download references
Acknowledgements
We extend our sincere gratitude to the domain experts who actively participated in and contributed to this research project. Their valuable insights and expertise have significantly enriched the quality of this study. We would like to express our appreciation to Sjaak Brinkkemper, Fabiano Dalpiaz, Gerard Wagenaar, Fernando Castor de Lima Filho, and Sergio Espana Cubillo for their invaluable feedback, which has helped us in presenting the results of this study more effectively. We are also deeply thankful to all the participants of the case studies for their cooperation and willingness to share their valuable publications, which served as essential resources in evaluating and validating the proposed decision model. Their contributions have been pivotal in ensuring the practical applicability and effectiveness of the decision model in real-world scenarios. Finally, we extend our appreciation to the journal editors and reviewers for their meticulous review of this manuscript and their constructive feedback. Their efforts have played a crucial role in enhancing the quality and clarity of this research, making it a more valuable contribution to the scientific community.
Author information
Kiyan Rezaee, Sara Mazaherim, Amir Hossein Rahimi and Sadegh Eskandari have contributed equally to this work.
Authors and Affiliations
Department of Information and Computer Science, Utrecht University, Utrecht, The Netherlands
Siamak Farshidi & Slinger Jansen
Informatics Institute, University of Amsterdam, Amsterdam, The Netherlands
Siamak Farshidi
Department of Computer Science, University of Guilan, Rasht, Iran
Kiyan Rezaee, Sara Mazaheri, Amir Hossein Rahimi, Ali Dadashzadeh, Morteza Ziabakhsh & Sadegh Eskandari
Lappeenranta University of Technology, Lappeenranta, Finland
Slinger Jansen
You can also search for this author in PubMed Google Scholar
Corresponding authors
Correspondence to Siamak Farshidi , Sadegh Eskandari or Slinger Jansen .
Additional information
Publisher's note.
Springer Nature remains neutral with regard to jurisdictional claims in published maps and institutional affiliations.
A Review protocol
See Table 9 .
See Table 10 .
C Categories
See Table 11 .
See Table 12 .
E Quality attributes and evaluation measures
See Table 13 .
Rights and permissions
Open Access This article is licensed under a Creative Commons Attribution 4.0 International License, which permits use, sharing, adaptation, distribution and reproduction in any medium or format, as long as you give appropriate credit to the original author(s) and the source, provide a link to the Creative Commons licence, and indicate if changes were made. The images or other third party material in this article are included in the article's Creative Commons licence, unless indicated otherwise in a credit line to the material. If material is not included in the article's Creative Commons licence and your intended use is not permitted by statutory regulation or exceeds the permitted use, you will need to obtain permission directly from the copyright holder. To view a copy of this licence, visit http://creativecommons.org/licenses/by/4.0/ .
Reprints and permissions
About this article
Farshidi, S., Rezaee, K., Mazaheri, S. et al. Understanding user intent modeling for conversational recommender systems: a systematic literature review. User Model User-Adap Inter (2024). https://doi.org/10.1007/s11257-024-09398-x
Download citation
Received : 06 August 2023
Accepted : 17 May 2024
Published : 06 June 2024
DOI : https://doi.org/10.1007/s11257-024-09398-x
Share this article
Anyone you share the following link with will be able to read this content:
Sorry, a shareable link is not currently available for this article.
Provided by the Springer Nature SharedIt content-sharing initiative
- User intent modeling
- User behavior
- Query intent
- Conversational recommender systems
- Personalized recommendation
- Machine learning models
Advertisement
- Find a journal
- Publish with us
- Track your research
Loading metrics
Open Access
Peer-reviewed
Research Article
Functional connectivity changes in the brain of adolescents with internet addiction: A systematic literature review of imaging studies
Roles Conceptualization, Data curation, Formal analysis, Investigation, Methodology, Project administration, Software, Validation, Visualization, Writing – original draft, Writing – review & editing
Affiliation Child and Adolescent Mental Health, Department of Brain Sciences, Great Ormond Street Institute of Child Health, University College London, London, United Kingdom
Roles Conceptualization, Supervision, Validation, Writing – review & editing
* E-mail: [email protected]
Affiliation Behavioural Brain Sciences Unit, Population Policy Practice Programme, Great Ormond Street Institute of Child Health, University College London, London, United Kingdom

- Max L. Y. Chang,
- Irene O. Lee
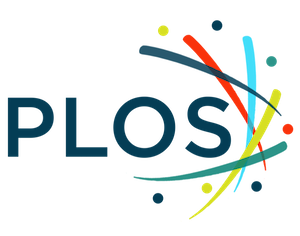
- Published: June 4, 2024
- https://doi.org/10.1371/journal.pmen.0000022
- Peer Review
- Reader Comments
Internet usage has seen a stark global rise over the last few decades, particularly among adolescents and young people, who have also been diagnosed increasingly with internet addiction (IA). IA impacts several neural networks that influence an adolescent’s behaviour and development. This article issued a literature review on the resting-state and task-based functional magnetic resonance imaging (fMRI) studies to inspect the consequences of IA on the functional connectivity (FC) in the adolescent brain and its subsequent effects on their behaviour and development. A systematic search was conducted from two databases, PubMed and PsycINFO, to select eligible articles according to the inclusion and exclusion criteria. Eligibility criteria was especially stringent regarding the adolescent age range (10–19) and formal diagnosis of IA. Bias and quality of individual studies were evaluated. The fMRI results from 12 articles demonstrated that the effects of IA were seen throughout multiple neural networks: a mix of increases/decreases in FC in the default mode network; an overall decrease in FC in the executive control network; and no clear increase or decrease in FC within the salience network and reward pathway. The FC changes led to addictive behaviour and tendencies in adolescents. The subsequent behavioural changes are associated with the mechanisms relating to the areas of cognitive control, reward valuation, motor coordination, and the developing adolescent brain. Our results presented the FC alterations in numerous brain regions of adolescents with IA leading to the behavioural and developmental changes. Research on this topic had a low frequency with adolescent samples and were primarily produced in Asian countries. Future research studies of comparing results from Western adolescent samples provide more insight on therapeutic intervention.
Citation: Chang MLY, Lee IO (2024) Functional connectivity changes in the brain of adolescents with internet addiction: A systematic literature review of imaging studies. PLOS Ment Health 1(1): e0000022. https://doi.org/10.1371/journal.pmen.0000022
Editor: Kizito Omona, Uganda Martyrs University, UGANDA
Received: December 29, 2023; Accepted: March 18, 2024; Published: June 4, 2024
Copyright: © 2024 Chang, Lee. This is an open access article distributed under the terms of the Creative Commons Attribution License , which permits unrestricted use, distribution, and reproduction in any medium, provided the original author and source are credited.
Data Availability: All relevant data are within the paper and its Supporting information files.
Funding: The authors received no specific funding for this work.
Competing interests: The authors have declared that no competing interests exist.
Introduction
The behavioural addiction brought on by excessive internet use has become a rising source of concern [ 1 ] since the last decade. According to clinical studies, individuals with Internet Addiction (IA) or Internet Gaming Disorder (IGD) may have a range of biopsychosocial effects and is classified as an impulse-control disorder owing to its resemblance to pathological gambling and substance addiction [ 2 , 3 ]. IA has been defined by researchers as a person’s inability to resist the urge to use the internet, which has negative effects on their psychological well-being as well as their social, academic, and professional lives [ 4 ]. The symptoms can have serious physical and interpersonal repercussions and are linked to mood modification, salience, tolerance, impulsivity, and conflict [ 5 ]. In severe circumstances, people may experience severe pain in their bodies or health issues like carpal tunnel syndrome, dry eyes, irregular eating and disrupted sleep [ 6 ]. Additionally, IA is significantly linked to comorbidities with other psychiatric disorders [ 7 ].
Stevens et al (2021) reviewed 53 studies including 17 countries and reported the global prevalence of IA was 3.05% [ 8 ]. Asian countries had a higher prevalence (5.1%) than European countries (2.7%) [ 8 ]. Strikingly, adolescents and young adults had a global IGD prevalence rate of 9.9% which matches previous literature that reported historically higher prevalence among adolescent populations compared to adults [ 8 , 9 ]. Over 80% of adolescent population in the UK, the USA, and Asia have direct access to the internet [ 10 ]. Children and adolescents frequently spend more time on media (possibly 7 hours and 22 minutes per day) than at school or sleeping [ 11 ]. Developing nations have also shown a sharp rise in teenage internet usage despite having lower internet penetration rates [ 10 ]. Concerns regarding the possible harms that overt internet use could do to adolescents and their development have arisen because of this surge, especially the significant impacts by the COVID-19 pandemic [ 12 ]. The growing prevalence and neurocognitive consequences of IA among adolescents makes this population a vital area of study [ 13 ].
Adolescence is a crucial developmental stage during which people go through significant changes in their biology, cognition, and personalities [ 14 ]. Adolescents’ emotional-behavioural functioning is hyperactivated, which creates risk of psychopathological vulnerability [ 15 ]. In accordance with clinical study results [ 16 ], this emotional hyperactivity is supported by a high level of neuronal plasticity. This plasticity enables teenagers to adapt to the numerous physical and emotional changes that occur during puberty as well as develop communication techniques and gain independence [ 16 ]. However, the strong neuronal plasticity is also associated with risk-taking and sensation seeking [ 17 ] which may lead to IA.
Despite the fact that the precise neuronal mechanisms underlying IA are still largely unclear, functional magnetic resonance imaging (fMRI) method has been used by scientists as an important framework to examine the neuropathological changes occurring in IA, particularly in the form of functional connectivity (FC) [ 18 ]. fMRI research study has shown that IA alters both the functional and structural makeup of the brain [ 3 ].
We hypothesise that IA has widespread neurological alteration effects rather than being limited to a few specific brain regions. Further hypothesis holds that according to these alterations of FC between the brain regions or certain neural networks, adolescents with IA would experience behavioural changes. An investigation of these domains could be useful for creating better procedures and standards as well as minimising the negative effects of overt internet use. This literature review aims to summarise and analyse the evidence of various imaging studies that have investigated the effects of IA on the FC in adolescents. This will be addressed through two research questions:
- How does internet addiction affect the functional connectivity in the adolescent brain?
- How is adolescent behaviour and development impacted by functional connectivity changes due to internet addiction?
The review protocol was conducted in line with the Preferred Reporting Items for Systematic Reviews and Meta-Analyses (PRISMA) guidelines (see S1 Checklist ).
Search strategy and selection process
A systematic search was conducted up until April 2023 from two sources of database, PubMed and PsycINFO, using a range of terms relevant to the title and research questions (see full list of search terms in S1 Appendix ). All the searched articles can be accessed in the S1 Data . The eligible articles were selected according to the inclusion and exclusion criteria. Inclusion criteria used for the present review were: (i) participants in the studies with clinical diagnosis of IA; (ii) participants between the ages of 10 and 19; (iii) imaging research investigations; (iv) works published between January 2013 and April 2023; (v) written in English language; (vi) peer-reviewed papers and (vii) full text. The numbers of articles excluded due to not meeting the inclusion criteria are shown in Fig 1 . Each study’s title and abstract were screened for eligibility.
- PPT PowerPoint slide
- PNG larger image
- TIFF original image
https://doi.org/10.1371/journal.pmen.0000022.g001
Quality appraisal
Full texts of all potentially relevant studies were then retrieved and further appraised for eligibility. Furthermore, articles were critically appraised based on the GRADE (Grading of Recommendations, Assessment, Development, and Evaluations) framework to evaluate the individual study for both quality and bias. The subsequent quality levels were then appraised to each article and listed as either low, moderate, or high.
Data collection process
Data that satisfied the inclusion requirements was entered into an excel sheet for data extraction and further selection. An article’s author, publication year, country, age range, participant sample size, sex, area of interest, measures, outcome and article quality were all included in the data extraction spreadsheet. Studies looking at FC, for instance, were grouped, while studies looking at FC in specific area were further divided into sub-groups.
Data synthesis and analysis
Articles were classified according to their location in the brain as well as the network or pathway they were a part of to create a coherent narrative between the selected studies. Conclusions concerning various research trends relevant to particular groupings were drawn from these groupings and subgroupings. To maintain the offered information in a prominent manner, these assertions were entered into the data extraction excel spreadsheet.
With the search performed on the selected databases, 238 articles in total were identified (see Fig 1 ). 15 duplicated articles were eliminated, and another 6 items were removed for various other reasons. Title and abstract screening eliminated 184 articles because they were not in English (number of article, n, = 7), did not include imaging components (n = 47), had adult participants (n = 53), did not have a clinical diagnosis of IA (n = 19), did not address FC in the brain (n = 20), and were published outside the desired timeframe (n = 38). A further 21 papers were eliminated for failing to meet inclusion requirements after the remaining 33 articles underwent full-text eligibility screening. A total of 12 papers were deemed eligible for this review analysis.
Characteristics of the included studies, as depicted in the data extraction sheet in Table 1 provide information of the author(s), publication year, sample size, study location, age range, gender, area of interest, outcome, measures used and quality appraisal. Most of the studies in this review utilised resting state functional magnetic resonance imaging techniques (n = 7), with several studies demonstrating task-based fMRI procedures (n = 3), and the remaining studies utilising whole-brain imaging measures (n = 2). The studies were all conducted in Asiatic countries, specifically coming from China (8), Korea (3), and Indonesia (1). Sample sizes ranged from 12 to 31 participants with most of the imaging studies having comparable sample sizes. Majority of the studies included a mix of male and female participants (n = 8) with several studies having a male only participant pool (n = 3). All except one of the mixed gender studies had a majority male participant pool. One study did not disclose their data on the gender demographics of their experiment. Study years ranged from 2013–2022, with 2 studies in 2013, 3 studies in 2014, 3 studies in 2015, 1 study in 2017, 1 study in 2020, 1 study in 2021, and 1 study in 2022.
https://doi.org/10.1371/journal.pmen.0000022.t001
(1) How does internet addiction affect the functional connectivity in the adolescent brain?
The included studies were organised according to the brain region or network that they were observing. The specific networks affected by IA were the default mode network, executive control system, salience network and reward pathway. These networks are vital components of adolescent behaviour and development [ 31 ]. The studies in each section were then grouped into subsections according to their specific brain regions within their network.
Default mode network (DMN)/reward network.
Out of the 12 studies, 3 have specifically studied the default mode network (DMN), and 3 observed whole-brain FC that partially included components of the DMN. The effect of IA on the various centres of the DMN was not unilaterally the same. The findings illustrate a complex mix of increases and decreases in FC depending on the specific region in the DMN (see Table 2 and Fig 2 ). The alteration of FC in posterior cingulate cortex (PCC) in the DMN was the most frequently reported area in adolescents with IA, which involved in attentional processes [ 32 ], but Lee et al. (2020) additionally found alterations of FC in other brain regions, such as anterior insula cortex, a node in the DMN that controls the integration of motivational and cognitive processes [ 20 ].
https://doi.org/10.1371/journal.pmen.0000022.g002
The overall changes of functional connectivity in the brain network including default mode network (DMN), executive control network (ECN), salience network (SN) and reward network. IA = Internet Addiction, FC = Functional Connectivity.
https://doi.org/10.1371/journal.pmen.0000022.t002
Ding et al. (2013) revealed altered FC in the cerebellum, the middle temporal gyrus, and the medial prefrontal cortex (mPFC) [ 22 ]. They found that the bilateral inferior parietal lobule, left superior parietal lobule, and right inferior temporal gyrus had decreased FC, while the bilateral posterior lobe of the cerebellum and the medial temporal gyrus had increased FC [ 22 ]. The right middle temporal gyrus was found to have 111 cluster voxels (t = 3.52, p<0.05) and the right inferior parietal lobule was found to have 324 cluster voxels (t = -4.07, p<0.05) with an extent threshold of 54 voxels (figures above this threshold are deemed significant) [ 22 ]. Additionally, there was a negative correlation, with 95 cluster voxels (p<0.05) between the FC of the left superior parietal lobule and the PCC with the Chen Internet Addiction Scores (CIAS) which are used to determine the severity of IA [ 22 ]. On the other hand, in regions of the reward system, connection with the PCC was positively connected with CIAS scores [ 22 ]. The most significant was the right praecuneus with 219 cluster voxels (p<0.05) [ 22 ]. Wang et al. (2017) also discovered that adolescents with IA had 33% less FC in the left inferior parietal lobule and 20% less FC in the dorsal mPFC [ 24 ]. A potential connection between the effects of substance use and overt internet use is revealed by the generally decreased FC in these areas of the DMN of teenagers with drug addiction and IA [ 35 ].
The putamen was one of the main regions of reduced FC in adolescents with IA [ 19 ]. The putamen and the insula-operculum demonstrated significant group differences regarding functional connectivity with a cluster size of 251 and an extent threshold of 250 (Z = 3.40, p<0.05) [ 19 ]. The molecular mechanisms behind addiction disorders have been intimately connected to decreased striatal dopaminergic function [ 19 ], making this function crucial.
Executive Control Network (ECN).
5 studies out of 12 have specifically viewed parts of the executive control network (ECN) and 3 studies observed whole-brain FC. The effects of IA on the ECN’s constituent parts were consistent across all the studies examined for this analysis (see Table 2 and Fig 3 ). The results showed a notable decline in all the ECN’s major centres. Li et al. (2014) used fMRI imaging and a behavioural task to study response inhibition in adolescents with IA [ 25 ] and found decreased activation at the striatum and frontal gyrus, particularly a reduction in FC at inferior frontal gyrus, in the IA group compared to controls [ 25 ]. The inferior frontal gyrus showed a reduction in FC in comparison to the controls with a cluster size of 71 (t = 4.18, p<0.05) [ 25 ]. In addition, the frontal-basal ganglia pathways in the adolescents with IA showed little effective connection between areas and increased degrees of response inhibition [ 25 ].
https://doi.org/10.1371/journal.pmen.0000022.g003
Lin et al. (2015) found that adolescents with IA demonstrated disrupted corticostriatal FC compared to controls [ 33 ]. The corticostriatal circuitry experienced decreased connectivity with the caudate, bilateral anterior cingulate cortex (ACC), as well as the striatum and frontal gyrus [ 33 ]. The inferior ventral striatum showed significantly reduced FC with the subcallosal ACC and caudate head with cluster size of 101 (t = -4.64, p<0.05) [ 33 ]. Decreased FC in the caudate implies dysfunction of the corticostriatal-limbic circuitry involved in cognitive and emotional control [ 36 ]. The decrease in FC in both the striatum and frontal gyrus is related to inhibitory control, a common deficit seen with disruptions with the ECN [ 33 ].
The dorsolateral prefrontal cortex (DLPFC), ACC, and right supplementary motor area (SMA) of the prefrontal cortex were all found to have significantly decreased grey matter volume [ 29 ]. In addition, the DLPFC, insula, temporal cortices, as well as significant subcortical regions like the striatum and thalamus, showed decreased FC [ 29 ]. According to Tremblay (2009), the striatum plays a significant role in the processing of rewards, decision-making, and motivation [ 37 ]. Chen et al. (2020) reported that the IA group demonstrated increased impulsivity as well as decreased reaction inhibition using a Stroop colour-word task [ 26 ]. Furthermore, Chen et al. (2020) observed that the left DLPFC and dorsal striatum experienced a negative connection efficiency value, specifically demonstrating that the dorsal striatum activity suppressed the left DLPFC [ 27 ].
Salience network (SN).
Out of the 12 chosen studies, 3 studies specifically looked at the salience network (SN) and 3 studies have observed whole-brain FC. Relative to the DMN and ECN, the findings on the SN were slightly sparser. Despite this, adolescents with IA demonstrated a moderate decrease in FC, as well as other measures like fibre connectivity and cognitive control, when compared to healthy control (see Table 2 and Fig 4 ).
https://doi.org/10.1371/journal.pmen.0000022.g004
Xing et al. (2014) used both dorsal anterior cingulate cortex (dACC) and insula to test FC changes in the SN of adolescents with IA and found decreased structural connectivity in the SN as well as decreased fractional anisotropy (FA) that correlated to behaviour performance in the Stroop colour word-task [ 21 ]. They examined the dACC and insula to determine whether the SN’s disrupted connectivity may be linked to the SN’s disruption of regulation, which would explain the impaired cognitive control seen in adolescents with IA. However, researchers did not find significant FC differences in the SN when compared to the controls [ 21 ]. These results provided evidence for the structural changes in the interconnectivity within SN in adolescents with IA.
Wang et al. (2017) investigated network interactions between the DMN, ECN, SN and reward pathway in IA subjects [ 24 ] (see Fig 5 ), and found 40% reduction of FC between the DMN and specific regions of the SN, such as the insula, in comparison to the controls (p = 0.008) [ 24 ]. The anterior insula and dACC are two areas that are impacted by this altered FC [ 24 ]. This finding supports the idea that IA has similar neurobiological abnormalities with other addictive illnesses, which is in line with a study that discovered disruptive changes in the SN and DMN’s interaction in cocaine addiction [ 38 ]. The insula has also been linked to the intensity of symptoms and has been implicated in the development of IA [ 39 ].
“+” indicates an increase in behaivour; “-”indicates a decrease in behaviour; solid arrows indicate a direct network interaction; and the dotted arrows indicates a reduction in network interaction. This diagram depicts network interactions juxtaposed with engaging in internet related behaviours. Through the neural interactions, the diagram illustrates how the networks inhibit or amplify internet usage and vice versa. Furthermore, it demonstrates how the SN mediates both the DMN and ECN.
https://doi.org/10.1371/journal.pmen.0000022.g005
(2) How is adolescent behaviour and development impacted by functional connectivity changes due to internet addiction?
The findings that IA individuals demonstrate an overall decrease in FC in the DMN is supported by numerous research [ 24 ]. Drug addict populations also exhibited similar decline in FC in the DMN [ 40 ]. The disruption of attentional orientation and self-referential processing for both substance and behavioural addiction was then hypothesised to be caused by DMN anomalies in FC [ 41 ].
In adolescents with IA, decline of FC in the parietal lobule affects visuospatial task-related behaviour [ 22 ], short-term memory [ 42 ], and the ability of controlling attention or restraining motor responses during response inhibition tests [ 42 ]. Cue-induced gaming cravings are influenced by the DMN [ 43 ]. A visual processing area called the praecuneus links gaming cues to internal information [ 22 ]. A meta-analysis found that the posterior cingulate cortex activity of individuals with IA during cue-reactivity tasks was connected with their gaming time [ 44 ], suggesting that excessive gaming may impair DMN function and that individuals with IA exert more cognitive effort to control it. Findings for the behavioural consequences of FC changes in the DMN illustrate its underlying role in regulating impulsivity, self-monitoring, and cognitive control.
Furthermore, Ding et al. (2013) reported an activation of components of the reward pathway, including areas like the nucleus accumbens, praecuneus, SMA, caudate, and thalamus, in connection to the DMN [ 22 ]. The increased FC of the limbic and reward networks have been confirmed to be a major biomarker for IA [ 45 , 46 ]. The increased reinforcement in these networks increases the strength of reward stimuli and makes it more difficult for other networks, namely the ECN, to down-regulate the increased attention [ 29 ] (See Fig 5 ).
Executive control network (ECN).
The numerous IA-affected components in the ECN have a role in a variety of behaviours that are connected to both response inhibition and emotional regulation [ 47 ]. For instance, brain regions like the striatum, which are linked to impulsivity and the reward system, are heavily involved in the act of playing online games [ 47 ]. Online game play activates the striatum, which suppresses the left DLPFC in ECN [ 48 ]. As a result, people with IA may find it difficult to control their want to play online games [ 48 ]. This system thus causes impulsive and protracted gaming conduct, lack of inhibitory control leading to the continued use of internet in an overt manner despite a variety of negative effects, personal distress, and signs of psychological dependence [ 33 ] (See Fig 5 ).
Wang et al. (2017) report that disruptions in cognitive control networks within the ECN are frequently linked to characteristics of substance addiction [ 24 ]. With samples that were addicted to heroin and cocaine, previous studies discovered abnormal FC in the ECN and the PFC [ 49 ]. Electronic gaming is known to promote striatal dopamine release, similar to drug addiction [ 50 ]. According to Drgonova and Walther (2016), it is hypothesised that dopamine could stimulate the reward system of the striatum in the brain, leading to a loss of impulse control and a failure of prefrontal lobe executive inhibitory control [ 51 ]. In the end, IA’s resemblance to drug use disorders may point to vital biomarkers or underlying mechanisms that explain how cognitive control and impulsive behaviour are related.
A task-related fMRI study found that the decrease in FC between the left DLPFC and dorsal striatum was congruent with an increase in impulsivity in adolescents with IA [ 26 ]. The lack of response inhibition from the ECN results in a loss of control over internet usage and a reduced capacity to display goal-directed behaviour [ 33 ]. Previous studies have linked the alteration of the ECN in IA with higher cue reactivity and impaired ability to self-regulate internet specific stimuli [ 52 ].
Salience network (SN)/ other networks.
Xing et al. (2014) investigated the significance of the SN regarding cognitive control in teenagers with IA [ 21 ]. The SN, which is composed of the ACC and insula, has been demonstrated to control dynamic changes in other networks to modify cognitive performance [ 21 ]. The ACC is engaged in conflict monitoring and cognitive control, according to previous neuroimaging research [ 53 ]. The insula is a region that integrates interoceptive states into conscious feelings [ 54 ]. The results from Xing et al. (2014) showed declines in the SN regarding its structural connectivity and fractional anisotropy, even though they did not observe any appreciable change in FC in the IA participants [ 21 ]. Due to the small sample size, the results may have indicated that FC methods are not sensitive enough to detect the significant functional changes [ 21 ]. However, task performance behaviours associated with impaired cognitive control in adolescents with IA were correlated with these findings [ 21 ]. Our comprehension of the SN’s broader function in IA can be enhanced by this relationship.
Research study supports the idea that different psychological issues are caused by the functional reorganisation of expansive brain networks, such that strong association between SN and DMN may provide neurological underpinnings at the system level for the uncontrollable character of internet-using behaviours [ 24 ]. In the study by Wang et al. (2017), the decreased interconnectivity between the SN and DMN, comprising regions such the DLPFC and the insula, suggests that adolescents with IA may struggle to effectively inhibit DMN activity during internally focused processing, leading to poorly managed desires or preoccupations to use the internet [ 24 ] (See Fig 5 ). Subsequently, this may cause a failure to inhibit DMN activity as well as a restriction of ECN functionality [ 55 ]. As a result, the adolescent experiences an increased salience and sensitivity towards internet addicting cues making it difficult to avoid these triggers [ 56 ].
The primary aim of this review was to present a summary of how internet addiction impacts on the functional connectivity of adolescent brain. Subsequently, the influence of IA on the adolescent brain was compartmentalised into three sections: alterations of FC at various brain regions, specific FC relationships, and behavioural/developmental changes. Overall, the specific effects of IA on the adolescent brain were not completely clear, given the variety of FC changes. However, there were overarching behavioural, network and developmental trends that were supported that provided insight on adolescent development.
The first hypothesis that was held about this question was that IA was widespread and would be regionally similar to substance-use and gambling addiction. After conducting a review of the information in the chosen articles, the hypothesis was predictably supported. The regions of the brain affected by IA are widespread and influence multiple networks, mainly DMN, ECN, SN and reward pathway. In the DMN, there was a complex mix of increases and decreases within the network. However, in the ECN, the alterations of FC were more unilaterally decreased, but the findings of SN and reward pathway were not quite clear. Overall, the FC changes within adolescents with IA are very much network specific and lay a solid foundation from which to understand the subsequent behaviour changes that arise from the disorder.
The second hypothesis placed emphasis on the importance of between network interactions and within network interactions in the continuation of IA and the development of its behavioural symptoms. The results from the findings involving the networks, DMN, SN, ECN and reward system, support this hypothesis (see Fig 5 ). Studies confirm the influence of all these neural networks on reward valuation, impulsivity, salience to stimuli, cue reactivity and other changes that alter behaviour towards the internet use. Many of these changes are connected to the inherent nature of the adolescent brain.
There are multiple explanations that underlie the vulnerability of the adolescent brain towards IA related urges. Several of them have to do with the inherent nature and underlying mechanisms of the adolescent brain. Children’s emotional, social, and cognitive capacities grow exponentially during childhood and adolescence [ 57 ]. Early teenagers go through a process called “social reorientation” that is characterised by heightened sensitivity to social cues and peer connections [ 58 ]. Adolescents’ improvements in their social skills coincide with changes in their brains’ anatomical and functional organisation [ 59 ]. Functional hubs exhibit growing connectivity strength [ 60 ], suggesting increased functional integration during development. During this time, the brain’s functional networks change from an anatomically dominant structure to a scattered architecture [ 60 ].
The adolescent brain is very responsive to synaptic reorganisation and experience cues [ 61 ]. As a result, one of the distinguishing traits of the maturation of adolescent brains is the variation in neural network trajectory [ 62 ]. Important weaknesses of the adolescent brain that may explain the neurobiological change brought on by external stimuli are illustrated by features like the functional gaps between networks and the inadequate segregation of networks [ 62 ].
The implications of these findings towards adolescent behaviour are significant. Although the exact changes and mechanisms are not fully clear, the observed changes in functional connectivity have the capacity of influencing several aspects of adolescent development. For example, functional connectivity has been utilised to investigate attachment styles in adolescents [ 63 ]. It was observed that adolescent attachment styles were negatively associated with caudate-prefrontal connectivity, but positively with the putamen-visual area connectivity [ 63 ]. Both named areas were also influenced by the onset of internet addiction, possibly providing a connection between the two. Another study associated neighbourhood/socioeconomic disadvantage with functional connectivity alterations in the DMN and dorsal attention network [ 64 ]. The study also found multivariate brain behaviour relationships between the altered/disadvantaged functional connectivity and mental health and cognition [ 64 ]. This conclusion supports the notion that the functional connectivity alterations observed in IA are associated with specific adolescent behaviours as well as the fact that functional connectivity can be utilised as a platform onto which to compare various neurologic conditions.
Limitations/strengths
There were several limitations that were related to the conduction of the review as well as the data extracted from the articles. Firstly, the study followed a systematic literature review design when analysing the fMRI studies. The data pulled from these imaging studies were namely qualitative and were subject to bias contrasting the quantitative nature of statistical analysis. Components of the study, such as sample sizes, effect sizes, and demographics were not weighted or controlled. The second limitation brought up by a similar review was the lack of a universal consensus of terminology given IA [ 47 ]. Globally, authors writing about this topic use an array of terminology including online gaming addiction, internet addiction, internet gaming disorder, and problematic internet use. Often, authors use multiple terms interchangeably which makes it difficult to depict the subtle similarities and differences between the terms.
Reviewing the explicit limitations in each of the included studies, two major limitations were brought up in many of the articles. One was relating to the cross-sectional nature of the included studies. Due to the inherent qualities of a cross-sectional study, the studies did not provide clear evidence that IA played a causal role towards the development of the adolescent brain. While several biopsychosocial factors mediate these interactions, task-based measures that combine executive functions with imaging results reinforce the assumed connection between the two that is utilised by the papers studying IA. Another limitation regarded the small sample size of the included studies, which averaged to around 20 participants. The small sample size can influence the generalisation of the results as well as the effectiveness of statistical analyses. Ultimately, both included study specific limitations illustrate the need for future studies to clarify the causal relationship between the alterations of FC and the development of IA.
Another vital limitation was the limited number of studies applying imaging techniques for investigations on IA in adolescents were a uniformly Far East collection of studies. The reason for this was because the studies included in this review were the only fMRI studies that were found that adhered to the strict adolescent age restriction. The adolescent age range given by the WHO (10–19 years old) [ 65 ] was strictly followed. It is important to note that a multitude of studies found in the initial search utilised an older adolescent demographic that was slightly higher than the WHO age range and had a mean age that was outside of the limitations. As a result, the results of this review are biased and based on the 12 studies that met the inclusion and exclusion criteria.
Regarding the global nature of the research, although the journals that the studies were published in were all established western journals, the collection of studies were found to all originate from Asian countries, namely China and Korea. Subsequently, it pulls into question if the results and measures from these studies are generalisable towards a western population. As stated previously, Asian countries have a higher prevalence of IA, which may be the reasoning to why the majority of studies are from there [ 8 ]. However, in an additional search including other age groups, it was found that a high majority of all FC studies on IA were done in Asian countries. Interestingly, western papers studying fMRI FC were primarily focused on gambling and substance-use addiction disorders. The western papers on IA were less focused on fMRI FC but more on other components of IA such as sleep, game-genre, and other non-imaging related factors. This demonstrated an overall lack of western fMRI studies on IA. It is important to note that both western and eastern fMRI studies on IA presented an overall lack on children and adolescents in general.
Despite the several limitations, this review provided a clear reflection on the state of the data. The strengths of the review include the strict inclusion/exclusion criteria that filtered through studies and only included ones that contained a purely adolescent sample. As a result, the information presented in this review was specific to the review’s aims. Given the sparse nature of adolescent specific fMRI studies on the FC changes in IA, this review successfully provided a much-needed niche representation of adolescent specific results. Furthermore, the review provided a thorough functional explanation of the DMN, ECN, SN and reward pathway making it accessible to readers new to the topic.
Future directions and implications
Through the search process of the review, there were more imaging studies focused on older adolescence and adulthood. Furthermore, finding a review that covered a strictly adolescent population, focused on FC changes, and was specifically depicting IA, was proven difficult. Many related reviews, such as Tereshchenko and Kasparov (2019), looked at risk factors related to the biopsychosocial model, but did not tackle specific alterations in specific structural or functional changes in the brain [ 66 ]. Weinstein (2017) found similar structural and functional results as well as the role IA has in altering response inhibition and reward valuation in adolescents with IA [ 47 ]. Overall, the accumulated findings only paint an emerging pattern which aligns with similar substance-use and gambling disorders. Future studies require more specificity in depicting the interactions between neural networks, as well as more literature on adolescent and comorbid populations. One future field of interest is the incorporation of more task-based fMRI data. Advances in resting-state fMRI methods have yet to be reflected or confirmed in task-based fMRI methods [ 62 ]. Due to the fact that network connectivity is shaped by different tasks, it is critical to confirm that the findings of the resting state fMRI studies also apply to the task based ones [ 62 ]. Subsequently, work in this area will confirm if intrinsic connectivity networks function in resting state will function similarly during goal directed behaviour [ 62 ]. An elevated focus on adolescent populations as well as task-based fMRI methodology will help uncover to what extent adolescent network connectivity maturation facilitates behavioural and cognitive development [ 62 ].
A treatment implication is the potential usage of bupropion for the treatment of IA. Bupropion has been previously used to treat patients with gambling disorder and has been effective in decreasing overall gambling behaviour as well as money spent while gambling [ 67 ]. Bae et al. (2018) found a decrease in clinical symptoms of IA in line with a 12-week bupropion treatment [ 31 ]. The study found that bupropion altered the FC of both the DMN and ECN which in turn decreased impulsivity and attentional deficits for the individuals with IA [ 31 ]. Interventions like bupropion illustrate the importance of understanding the fundamental mechanisms that underlie disorders like IA.
The goal for this review was to summarise the current literature on functional connectivity changes in adolescents with internet addiction. The findings answered the primary research questions that were directed at FC alterations within several networks of the adolescent brain and how that influenced their behaviour and development. Overall, the research demonstrated several wide-ranging effects that influenced the DMN, SN, ECN, and reward centres. Additionally, the findings gave ground to important details such as the maturation of the adolescent brain, the high prevalence of Asian originated studies, and the importance of task-based studies in this field. The process of making this review allowed for a thorough understanding IA and adolescent brain interactions.
Given the influx of technology and media in the lives and education of children and adolescents, an increase in prevalence and focus on internet related behavioural changes is imperative towards future children/adolescent mental health. Events such as COVID-19 act to expose the consequences of extended internet usage on the development and lifestyle of specifically young people. While it is important for parents and older generations to be wary of these changes, it is important for them to develop a base understanding of the issue and not dismiss it as an all-bad or all-good scenario. Future research on IA will aim to better understand the causal relationship between IA and psychological symptoms that coincide with it. The current literature regarding functional connectivity changes in adolescents is limited and requires future studies to test with larger sample sizes, comorbid populations, and populations outside Far East Asia.
This review aimed to demonstrate the inner workings of how IA alters the connection between the primary behavioural networks in the adolescent brain. Predictably, the present answers merely paint an unfinished picture that does not necessarily depict internet usage as overwhelmingly positive or negative. Alternatively, the research points towards emerging patterns that can direct individuals on the consequences of certain variables or risk factors. A clearer depiction of the mechanisms of IA would allow physicians to screen and treat the onset of IA more effectively. Clinically, this could be in the form of more streamlined and accurate sessions of CBT or family therapy, targeting key symptoms of IA. Alternatively clinicians could potentially prescribe treatment such as bupropion to target FC in certain regions of the brain. Furthermore, parental education on IA is another possible avenue of prevention from a public health standpoint. Parents who are aware of the early signs and onset of IA will more effectively handle screen time, impulsivity, and minimize the risk factors surrounding IA.
Additionally, an increased attention towards internet related fMRI research is needed in the West, as mentioned previously. Despite cultural differences, Western countries may hold similarities to the eastern countries with a high prevalence of IA, like China and Korea, regarding the implications of the internet and IA. The increasing influence of the internet on the world may contribute to an overall increase in the global prevalence of IA. Nonetheless, the high saturation of eastern studies in this field should be replicated with a Western sample to determine if the same FC alterations occur. A growing interest in internet related research and education within the West will hopefully lead to the knowledge of healthier internet habits and coping strategies among parents with children and adolescents. Furthermore, IA research has the potential to become a crucial proxy for which to study adolescent brain maturation and development.
Supporting information
S1 checklist. prisma checklist..
https://doi.org/10.1371/journal.pmen.0000022.s001
S1 Appendix. Search strategies with all the terms.
https://doi.org/10.1371/journal.pmen.0000022.s002
S1 Data. Article screening records with details of categorized content.
https://doi.org/10.1371/journal.pmen.0000022.s003
Acknowledgments
The authors thank https://www.stockio.com/free-clipart/brain-01 (with attribution to Stockio.com); and https://www.rawpixel.com/image/6442258/png-sticker-vintage for the free images used to create Figs 2 – 4 .
- View Article
- PubMed/NCBI
- Google Scholar
- 2. Association AP. Diagnostic and statistical manual of mental disorders: DSM-5. 5 ed. Washington, D.C.: American Psychiatric Publishing; 2013.
- 10. Stats IW. World Internet Users Statistics and World Population Stats 2013 [ http://www.internetworldstats.com/stats.htm .
- 11. Rideout VJR M. B. The common sense census: media use by tweens and teens. San Francisco, CA: Common Sense Media; 2019.
- 37. Tremblay L. The Ventral Striatum. Handbook of Reward and Decision Making: Academic Press; 2009.
- 57. Bhana A. Middle childhood and pre-adolescence. Promoting mental health in scarce-resource contexts: emerging evidence and practice. Cape Town: HSRC Press; 2010. p. 124–42.
- 65. Organization WH. Adolescent Health 2023 [ https://www.who.int/health-topics/adolescent-health#tab=tab_1 .
Log in using your username and password
- Search More Search for this keyword Advanced search
- Latest content
- Current issue
- BMJ Journals More You are viewing from: Google Indexer
You are here
- Online First
- Assessing health outcomes: a systematic review of electronic patient-reported outcomes in oncology
- Article Text
- Article info
- Citation Tools
- Rapid Responses
- Article metrics

- http://orcid.org/0000-0003-1880-5731 Mikel Urretavizcaya 1 ,
- Karen Álvarez 2 ,
- Olatz Olariaga 1 ,
- Maria Jose Tames 1 ,
- Ainhoa Asensio 1 ,
- Gerardo Cajaraville 1 ,
- http://orcid.org/0000-0002-8986-0247 Ana Cristina Riestra 1 , 3
- 1 Pharmacy Department , Onkologikoa , San Sebastian , País Vasco , Spain
- 2 Pharmacy Department , Nuestra Senora de la Candelaria University Hospital , Santa Cruz de Tenerife , Canarias , Spain
- 3 Medicine Department , University of Deusto , Bilbao , País Vasco , Spain
- Correspondence to Mikel Urretavizcaya, Pharmacy Department, Onkologikoa, San Sebastian, País Vasco, Spain; urretabizkaia.mikel{at}gmail.com
Purpose This study investigates the clinical impact of electronic patient-reported outcome (ePRO) monitoring apps/web interfaces, aimed at symptom-management, in cancer patients undergoing outpatient systemic antineoplastic treatment. Additionally, it explores the advantages offered by these applications, including their functionalities and healthcare team-initiated follow-up programmes.
Methods A systematic literature review was conducted using a predefined search strategy in MEDLINE. Inclusion criteria encompassed primary studies assessing symptom burden through at-home ePRO surveys in adult cancer patients receiving outpatient systemic antineoplastic treatment, whenever health outcomes were evaluated. Exclusion criteria excluded telemedicine-based interventions other than ePRO questionnaires and non-primary articles or study protocols. To evaluate the potential bias in the included studies, an exhaustive quality assessment was conducted, as an additional inclusion filter.
Results Among 246 identified articles, 227 were excluded for non-compliance with inclusion/exclusion criteria. Of the remaining 19 articles, only eight met the rigorous validity assessment and were included for detailed examination and data extraction, presented in attached tables.
Conclusion This review provides compelling evidence of ePRO monitoring’s positive clinical impact across diverse cancer settings, encompassing various cancer types, including early and metastatic stages. These systems are crucial in enabling timely interventions and reducing communication barriers, among other functionalities. While areas for future ePRO innovation are identified, the primary limitation lies in comparing clinical outcomes of reviewed articles, due to scale variability and study population heterogeneity. To conclude, our results reaffirm the transformative potential of ePRO apps in oncology and their pivotal role in shaping the future of cancer care.
- Patient Reported Outcomes
- Adverse Drug Reaction Reporting Systems
- Antineoplastic agents
- MEDICAL ONCOLOGY
- Quality of Life
- Outcome Assessment, Health Care
Data availability statement
All data relevant to the study are included in the article or uploaded as supplementary information. As this study is a systematic review, no new data were generated or analysed. All data used in this review are publicly available in the cited sources, and the comprehensive list of references is provided in the manuscript. The authors confirm that they had full access to all the data in the study and take responsibility for the integrity of the data and the accuracy of the data analysis.
https://doi.org/10.1136/ejhpharm-2023-004072
Statistics from Altmetric.com
Request permissions.
If you wish to reuse any or all of this article please use the link below which will take you to the Copyright Clearance Center’s RightsLink service. You will be able to get a quick price and instant permission to reuse the content in many different ways.
WHAT IS ALREADY KNOWN ON THIS TOPIC
Previous studies acknowledge that ePRO monitoring holds promise for timely symptom care and improved health-related quality of life (HRQL) in cancer treatment. However, its widespread adoption remains limited.
WHAT THIS STUDY ADDS
Our review offers compelling evidence supporting the increasingly apparent clinical benefits of ePRO monitoring, including reduced symptom burden, enhanced HRQL and even overall survival benefits. Additionally, we introduce a comprehensive checklist for evaluating diverse ePRO app functionalities, identifying areas for improvement and emphasising opportunities for innovation.
HOW THIS STUDY MIGHT AFFECT RESEARCH, PRACTICE OR POLICY
This review highlights the transformative potential of ePRO apps in oncology, entailing a step forward in implementing ePRO monitoring in clinical practice.
Introduction
Symptoms experienced by cancer patients are a common and complex facet of their journey, stemming from various sources, including the cancer itself, treatment-related side effects, and coexisting conditions. 1 Alarmingly, these symptoms often evade detection by healthcare professionals in up to half of the cases. 2 Managing symptoms presents a significant challenge to the well-being and treatment outcomes of cancer patients. Uncontrolled symptoms can result in impaired health-related quality of life (HRQL), treatment adherence issues, chemotherapy delays or dose reductions, and distressing visits to emergency departments, ultimately affecting health outcomes and even mortality. 3–6
Traditionally, the reporting of symptoms has relied on patients' retrospective recall and self-identification of severe symptoms, leading to uncertainties and delayed reporting, and often, the inability to access timely care. 6 7 This delay places patients' safety at risk and necessitates the exploration of alternative approaches.
One promising avenue is the integration of electronic systems that facilitate patient-reported outcome (PRO) surveys, enabling early symptom detection and timely clinician intervention. 8 The systematic collection of symptom information through standardised PRO questionnaires has shown potential in improving symptom control. 9 10 Numerous web-based systems exist 11 12 and have demonstrated their ability to prompt clinicians to intensify symptom management, 13 14 enhance patient-clinician communication, and improve patient satisfaction and well-being. 15–21
Despite the compelling evidence supporting the benefits of electronic symptom monitoring through PROs, its widespread adoption in cancer treatment has been limited. 8 The hesitance to adopt these systems may stem from the ongoing debate regarding whether the clinical benefits of integrating electronic patient-reported outcomes (ePRO) into routine oncology practice outweigh the associated costs and burdens. 12 15 16 This integration demands technological resources, patient engagement, staff effort, and a reconfiguration of information flow. 22
In this context, the future of ePRO monitoring depends on evidence that it actually produces clinical benefits. To gain a clearer understanding of its value, it is imperative to identify which components of web-based support systems are most beneficial for patients. Unfortunately, this attempt is complicated by the fact that it remains unclear which specific eHealth functions are responsible for the reported clinical benefits. 23 This introduces an additional layer of complexity when comparing the health results obtained from different articles that use various apps, because, as the intervention (the components of web-based support systems) varies, it is to be expected that the obtained clinical benefits do too.
In light of these challenges and opportunities, this review explores the potential of electronic PRO systems to revolutionise the management of cancer-related symptoms, with a particular focus on their impact on health outcomes in the broadest sense. We also examine the functionalities and advantages offered by each PRO monitoring app and web interface, aiming to provide insights to the components included by web-based support systems that have demonstrated actual health benefits. Through an in-depth analysis of the existing literature, we seek to shed light on the role of electronic PRO systems in shaping the future of symptom management in cancer care.
We conducted a systematic review following the Preferred Reporting Items for Systematic Reviews and Meta-Analyses (PRISMA) statement guidelines to ensure methodological quality.
Research questions
The PICO (Population, Intervention, Comparison and Outcome) model was employed to frame our clinical research questions. The following were proposed:
RQ1. Have ePRO monitoring apps/web interfaces, aimed at symptom assessment, demonstrated positive health results when applied to adult cancer outpatients receiving systemic antineoplastic agents?
RQ2. What kind of advantages do these applications offer to patients, both through their functionalities and through the follow-up programmes that the healthcare team offers?
Search strategy
The search was conducted in March 2023. The MEDLINE Public Library of Medicine (PubMed) database was explored for relevant studies using the following search terms, limited to the period from 2010 to present:
‘Patient Reported Outcome Measures’ OR ‘Patient Outcome Assessment’ OR ‘Healthcare Surveys’
‘Mobile Applications’ OR ‘Telemedicine’ OR ‘Tablet’
‘Neoplasms’
‘Drug-Related Side Effects and Adverse Reactions’ OR ‘Quality of Life’ OR ‘Self Care’ OR ‘Patient Satisfaction’
All search terms were combined using the Boolean operator ‘AND,’ resulting in the following search expression: A AND B AND C AND D.
The search was replicated in May 2024 to update the results in case of finding relevant articles.
Inclusion and exclusion criteria
Titles and abstracts were independently reviewed by two researchers to ensure eligibility to the predefined criteria. In case of disagreement, a third author reviewed to confirm inclusion or exclusion.
Studies meeting the following criteria were included: (i) primary studies assessing symptom burden, at least weekly, through at-home ePRO questionnaires; (ii) developed with adult cancer outpatients receiving systemic antineoplastic treatment; and (iii) whenever health outcomes were evaluated. The exclusion criteria were: (i) studies with telemedicine-based interventions other than the use of electronic questionnaires for the PRO registration; and (ii) articles describing study protocols, systematic reviews, editorials, doctoral theses, opinion articles, abstracts, posters or conference presentations.
Validity assessment
The extent to which a review can draw meaningful conclusions about the effects of an intervention depends on whether the data and results from the included studies are valid. Hence, we developed a novel method to systematically evaluate the validity of each individual study chosen based on our inclusion and exclusion criteria. We established seven quality criteria (QC) to create a scoring system for assessing both internal and external validity.
External validity refers to whether the study is asking an appropriate research question. To assess this, three predefined QC were employed to evaluate if the research questions in the reviewed studies were pertinent to answering the research questions posed in our review (QC5 to QC7).
Internal validity refers to whether a study appropriately answers its research question, which is closely linked to its methodological quality. Given that this review aimed to compile information from articles assessing the impact on health outcomes, it was assumed that the research questions should be related to health outcomes. Therefore, we evaluated their methodological ability to demonstrate valid and reliable differences in health outcomes, where present.
This capacity depends on various factors, including the use of appropriate methods for quantifying health outcomes, presenting them in comparison to a control group, and conducting statistical analyses to verify the statistical significance of observed differences. These principles, along with whether the study’s limitations were acknowledged, constituted the internal validity quality criteria (QC1 to QC4).
These QC were used to form a scoring system to determine the inclusion or exclusion of articles in this review, based on their validity. We set a minimum threshold of 3 out of 4 points for internal validity and 2 out of 3 points for external validity as the criteria for inclusion.
Quality criteria compliance was independently assessed by two researchers. In cases of disagreement, a third author reviewed the articles to confirm inclusion or exclusion.
Internal validity criteria
QC1 . Are health outcome obtaining methods well described?
1 point if well described, using validated scales.
0.5 points if adequately described but not using validated scales.
0 points if poorly described.
QC2 . Are health outcomes expressed relative to a control group with no ePRO symptom assessment (pre-post or parallel control group)?
1 point if affirmative.
0 points if negative.
QC3 . Is a statistical significance analysis performed for the obtained health outcomes?
QC4 . Are the study limitations presented?
1 point if the study limitations were detailed.
0.5 points if approached superficially.
0 points if not addressed.
External validity criteria
QC5 . Do the research objectives explicitly include patient-reported health outcomes as either primary or secondary measures?
1 point if main objectives involve patient-reported health outcome measures.
0.5 points if secondary objectives involve patient-reported health outcome measures.
QC6 . Does it address the methods by which patients receive advice for managing their symptoms?
1 point if well addressed.
0.5 points if superficially addressed.
QC7 . Does the article provide information that answers the proposed research questions?
1 point if the article contributed to answering the research questions.
0.5 points if it partially contributed.
0 points if it did not contribute to solving the research questions.
Study variables and data extraction
Data extraction, which served as the basis for our discussion and results, was carried out using a comparative table that included various variables: author name, publication year, study population, sample size, used app/web-interface, intended health-related endpoints, health results obtained by the intervention group compared with the control group, used scales and subscales, mean differences and statistical data.
Additionally, the authors collaboratively established a set of features that, in their collective perspective, an ideal symptom-management-focused Patient-Reported Outcome Measure (PROM) app/web interface should encompass. These features were documented in a 21-item checklist, and the extent to which the reviewed articles' apps complied with these criteria was assessed.
All authors collectively reviewed the data contained in these tables through group discussions and individual reviews.
Out of the initial 246 articles identified through our search strategy, 227 were excluded for not meeting the predetermined inclusion and exclusion criteria based on the title and abstract review. Subsequently, the remaining 19 articles underwent a thorough full-text evaluation and validity assessment (see figure 1 ). Among these, 11 were discarded as they did not meet our specified validity criteria, primarily due to external validity. 24–34 In other words, they did not contribute to addressing the research questions outlined in our review and did not meet the minimum external validity threshold (refer to table 1 for details). In no way does this imply that the scientific merit of the excluded studies is being questioned. They were excluded solely based on our predetermined criteria, which were established with the aim of providing an objective method for assessing validity.
- Download figure
- Open in new tab
- Download powerpoint
Quantitative results of the search protocol and study selection.
- View inline
Quality criteria compliance degree
Consequently, the remaining eight articles were included in our review, being subjected to detailed examination and data extraction. 1 7 8 35–39 A search update conducted in May 2024 confirmed that no further articles meeting the pre-specified inclusion and exclusion criteria had been found up to that date.
Attending to the design, all studies were controlled clinical trials including patients on a voluntary basis. None of the authors described a widespread adoption of ePRO monitoring apps in the routine oncology practice; hence, giving access to these apps was the cornerstone of the initiative carried out in the intervention group, as compared with the control group, who continued with the usual clinical care.
Table 2 and the online supplemental table encapsulate the information gleaned from these articles. The online supplemental table contains essential data to address the first research question concerning the health outcomes demonstrated through ePRO monitoring. It is evident that these studies consistently demonstrate improvements in symptom burden and overall symptom distress. 7 8 35 38 Notably, enhancements in HRQL and their impact on process of care measures are also noteworthy. 7 8 37 The most remarkable results, however, pertain to the improvements in overall survival. 37 40
Supplemental material
Included apps′ functionalities and healthcare team-initiated follow-up programmes
Table 2 compiles pertinent information to answer the second research question regarding the advantages provided by these applications and monitoring programmes to patients, and their contributions to improved health outcomes. The collected data suggests that these systems play a pivotal role in enabling timely interventions, more frequent than conventional practices, and in reducing communication barriers. 7 8 35 38 39
Notably, the reviewed literature did not provide information regarding the approval status of these apps by national regulatory agencies in their respective countries.
Through a meticulous analysis of existing literature, this review contributes valuable insights to the ongoing debate surrounding the integration of ePROs into routine oncology practice.
In this review, we pose two significant research queries regarding the clinical impact and operational capabilities of ePRO monitoring apps and web interfaces. Next, we delve into a comprehensive discussion of the insights gathered from the systematic review, structured in alignment with the research questions specified in the study protocol.
Research query 1: Have ePRO monitoring apps/web interfaces, aimed at symptom assessment, demonstrated positive health results when applied to adult cancer outpatients receiving systemic antineoplastic agents?
Our review of the literature indicates that ePRO monitoring apps designed for symptom control show positive impacts on health-related outcomes when applied to cancer patients undergoing systemic antineoplastic treatment on an outpatient basis. The reviewed data encompass studies involving a wide range of cancer types, including breast, colorectal, genitourinary, gynaecological, pancreatic, Hodgkin’s lymphoma, and non-Hodgkin's lymphoma, spanning both early and metastatic stages.
✓ Early or non-metastatic stage
Consistent improvements in symptom burden and overall symptom distress are observed in various studies, as measured by the Memorial Symptom Assessment Scale. 7 35 38 Enhancements in physical well-being are reported within a few weeks after the initiation of chemotherapy 1 and a reduction in the prevalence of symptoms is observed in both neoadjuvant 38 and adjuvant settings. 39 Some studies also note a reduction in anxiety and depression symptoms over time 35 ; other, however, fail to observe significant differences in symptom severity and interference. 36
HRQL outcomes are less consistent. Larger studies, such as the one conducted by Maguire et al. , report significant improvements in global HRQL across all cycles, as well as in physical and functional domains, as measured by the FACT-G scale. 7 In contrast, medium-sized and smaller studies do not consistently observe significant differences in global HRQL. 1 36 38 39 However, these studies do identify enhancements in specific HRQL domains, such as the QLQ-C30 emotional functioning subscale 38 39 and favourable changes on the EuroQol EQ-5D Visual Analogue Scale. 1
Additional outcomes include improvements in various supportive care needs 7 and self-efficacy domains. 1 7 39 Furthermore, a noteworthy finding is an enhanced adherence rate to oral therapies among patients who reported baseline adherence issues and elevated anxiety symptoms. 36
✓ Metastatic setting
Basch et al, demonstrate that ePRO monitoring leads to improvement in physical function, symptom control and HRQL in the metastatic setting. It also impacts on process of care measures, reducing emergency department visits, hospitalisations, and prolonging the time patients remain on chemotherapy. 8 37
The most significant results, however, are those that reveal an increase in overall survival (OS). In the study by Basch et al., the percentage of patients alive at 1 year increased by 6%. 37 Furthermore, a post-hoc analysis confirms that these benefits are sustained over time, with a median 7 year follow-up showing a mean 5 month OS improvement, 40 underscoring the durability of the initially observed benefits.
Research query 2. What kind of advantages do these applications offer to patients, both through their functionalities and through the follow-up programmes that the healthcare team offers?
Most ePRO monitoring apps facilitate real-time symptom reporting to patients through standardised questionnaires, thereby enabling the healthcare team to continuously monitor the severity of symptoms over time. 1 7 35 37–39 Patient reports are linked to stratified alert systems, that trigger contact protocols with the healthcare team when symptom thresholds are exceeded. 1 7 8 37–39 Additionally, these apps typically provide automated and personalised feedback to patients, utilising clinical algorithms to provide specific recommendations based on reported symptom levels. 1 7 8 35 38 39
The enhancement of health outcomes is closely related to the reduction of communication barriers and the more frequent and timely interventions provided by the healthcare team, as compared with conventional practices. 7 8 35 38 39 Some studies even establish maximum response times to alerts by the healthcare team, which proves to be an interesting approach. 7 35 38 39 Equally important is the provision of hospital contact instructions to patients for urgent needs outside of alert handling hours. 1 37–39
Regular reminders sent to patients represent a widely adopted strategy aimed at motivating app usage and enhancing adherence to symptom reporting. 1 8 36–39 The reported data not only contribute to improved symptom management but also enable healthcare teams to address patients' physical and psychological needs, 1 7 8 35 38 39 and emotional and wellness support. 7 8 35 36
For patients undergoing oral therapies, some apps send regular reminders with each oral antineoplastic agent (OAA) intake, enabling patients to track OAA consumption, note any omissions, and provide reasons for non-adherence. 36 Ideally, such a system should alert the healthcare team in case of poor adherence to OAA.
The findings presented in our review offer compelling evidence that ePRO monitoring yields tangible improvements in health outcomes across diverse cancer settings, spanning both early and metastatic stages. Clinical benefits on symptom burden, HRQL, and even overall survival are becoming increasingly evident. This reinforces the idea that the clinical advantages of these systems may outweigh any associated costs or logistical challenges.
In the context of identifying the most beneficial components of web-based support systems for patients, the currently available evidence suggests that the key role played by these systems is in facilitating timely interventions, more frequent than in conventional practices, and reducing communication barriers. Additionally, these systems empower patients to actively engage in their self-care, fostering a sense of ownership and involvement in their health management.
However, our review also highlights areas for potential innovation within the ePRO landscape. It is noteworthy that several features identified by the authors as ideal for inclusion in a symptom-management-focused PROM app were not commonly found in the articles under review. These particular features have been recognised as potential areas for improvement in future apps and encompass the following.
First, among the apps examined, none have yet embraced the concept of tailoring personalised symptom-related surveys through predictive symptom and adverse effect analysis, specifically customised for distinct cancer types and treatments. This innovative approach not only streamlines the questionnaire, focusing on the most pertinent and anticipated aspects but also avoids overburdening patients with less relevant inquiries. Moreover, the potential for integrating artificial intelligence and real-world data to develop predictive, personalised, and targeted interventions represents an exciting avenue for future research, promising to further enhance patient outcomes and care efficiency.
Second, the inclusion of clinical parameter monitoring within ePRO apps has the potential to broaden their utility, facilitating a comprehensive approach to cancer care management. Wearable devices, such as smartwatches with capabilities to measure vital signs such as blood pressure, heart rate, and body temperature, could emerge as valuable components in advancing this approach.
Thirdly, a critical factor in promoting the integration of ePROs into routine clinical practice is to ensure their incorporation into electronic medical records. This would enable healthcare teams to access patient-reported outcomes effortlessly, reducing administrative burdens and consolidating all of the patient’s clinical information within a unified platform. Regrettably, our review reveals that progress in this area has been modest, indicating a clear need for streamlining these processes.
Finally, patients would highly appreciate the integration of a calendar feature that seamlessly synchronises with electronic medical records, automatically presenting all their medical appointments. The expedited processing of appointment requests for clinical consultations through the ePROs monitoring app would also be valued by patients. These enhancements have the potential to greatly enhance the overall patient experience and convenience.
Study limitations
The search strategy was limited to PubMed, potentially excluding relevant studies from other databases.
The analysis of data in this systematic review poses difficulties in making direct comparisons among the clinical outcomes reported in the reviewed articles. These challenges stem from two main factors: the significant variability in the measurement scales used to assess health outcomes and the observed heterogeneity in the study populations. The studies included in this review cover a diverse spectrum of neoplastic conditions and various stages of cancer, adding complexity to the task of drawing direct comparisons.
Furthermore, based on the findings, we are unable to provide insights into which specific components of web-based support systems offer the greatest benefits to patients. Future research should aim to explore these specific components in greater detail, seeking to establish direct connections between them and measurable health outcomes. It is important to recognise the inherent complexity in demonstrating such associations, making this a challenging endeavour for future investigations.
To conclude, our review reaffirms the transformative potential of ePRO systems in oncology. It calls for ongoing research and innovation to unlock the full spectrum of their capabilities and underscores the imperative of leveraging these technologies to enhance patient care, improve health outcomes, and drive efficiency in healthcare delivery.
Moreover, the COVID-19 pandemic has emphasised the urgency of accelerating the adoption of e-PROMs and eHealth interventions for the safe and efficient delivery of cancer care. Fortunately, governments and healthcare organisations are recognising the disruptive potential of digital health technologies and are actively adapting to this ever-evolving landscape, further highlighting that ePROs are poised to play a pivotal role in the future of cancer care.
Ethics statements
Patient consent for publication.
Not applicable.
Ethics approval
Acknowledgments.
We extend our gratitude to the scientific community for their valuable contributions to electronic symptom monitoring across different settings. Their prior work has been pivotal in shaping the context for this review.
- Absolom K ,
- Warrington L ,
- Hudson E , et al
- Pakhomov S ,
- Jacobsen SJ ,
- Chute CG , et al
- McKenzie H ,
- White K , et al
- Visvanathan K ,
- Thorner E , et al
- Dunbar A , et al
- Coolbrandt A ,
- Van den Heede K ,
- Vanhove E , et al
- Maguire R ,
- Kotronoulas G , et al
- Henson S , et al
- Snyder CF ,
- Aaronson NK ,
- Choucair AK , et al
- Bennett AV ,
- Jensen RE ,
- Abernethy AP , et al
- Sussman J ,
- Martelli-Reid L , et al
- Kroenke K ,
- Wu J , et al
- Valderas JM ,
- Kotzeva A ,
- Espallargues M , et al
- Kotronoulas G ,
- Kearney N ,
- Maguire R , et al
- Detmar SB ,
- Muller MJ ,
- Schornagel JH , et al
- Dulko D , et al
- Velikova G ,
- Smith AB , et al
- Blumenstein BA ,
- Halpenny B , et al
- Stover AM ,
- Schrag D , et al
- Hawkins R ,
- Pingree S , et al
- Mohamed A ,
- Corbett C , et al
- McKillop CN ,
- Stepanski E , et al
- Richardson D ,
- Mahtani R , et al
- Connaghan J ,
- Arber A , et al
- Lanzola G ,
- Quaglini S , et al
- Dudley WN ,
- Dudley M , et al
- Innominato PF ,
- Komarzynski S ,
- Mohammad-Djafari A , et al
- App R , et al
- Meghiref Y ,
- Duverger C , et al
- Alt-Epping B , et al
- Børøsund E ,
- Cvancarova M ,
- Moore SM , et al
- Jacobs JM ,
- Pensak N , et al
- Kris MG , et al
- Langius-Eklöf A ,
- Nilsson M , et al
- Gustavell T ,
- Sundberg K ,
- Segersvärd R , et al
- Dueck AC , et al
EAHP Statement 5: Patient Safety and Quality Assurance.
Contributors All authors contributed to the study conception and design.The process of reviewing abstracts and titles to determine their inclusion or exclusion in this study, as well as the subsequent full-text evaluation of potentially eligible articles for quality assessment, was conducted by MUA and KAT. In case of disagreement, ACRA reviewed to confirm inclusion or exclusion. Data collection and analysis were performed by MUA, KAT and ACRA. The first draft of the manuscript was written by MUA and all authors commented on previous versions of the manuscript. All authors read and approved the final manuscript. ChatGPT 3.5 was used to translate content from Spanish to English and to help restructure some paragraphs, but not for creating new content.
Funding The authors have not declared a specific grant for this research from any funding agency in the public, commercial or not-for-profit sectors.
Competing interests None declared.
Provenance and peer review Not commissioned; externally peer reviewed.
Supplemental material This content has been supplied by the author(s). It has not been vetted by BMJ Publishing Group Limited (BMJ) and may not have been peer-reviewed. Any opinions or recommendations discussed are solely those of the author(s) and are not endorsed by BMJ. BMJ disclaims all liability and responsibility arising from any reliance placed on the content. Where the content includes any translated material, BMJ does not warrant the accuracy and reliability of the translations (including but not limited to local regulations, clinical guidelines, terminology, drug names and drug dosages), and is not responsible for any error and/or omissions arising from translation and adaptation or otherwise.
Read the full text or download the PDF:
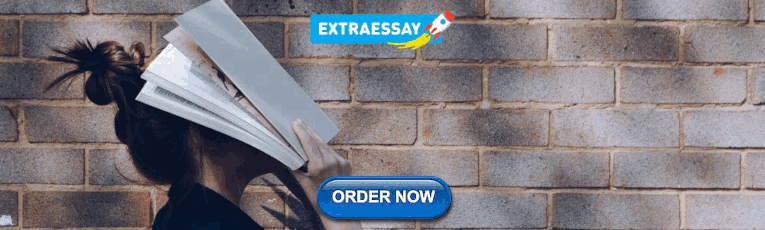
IMAGES
VIDEO
COMMENTS
Rayyan Enterprise and Rayyan Teams+ make it faster, easier and more convenient for you to manage your research process across your organization. Accelerate your research across your team or organization and save valuable researcher time. Build and preserve institutional assets, including literature searches, systematic reviews, and full-text ...
Background Systematic reviews provide a structured overview of the available evidence in medical-scientific research. However, due to the increasing medical-scientific research output, it is a time-consuming task to conduct systematic reviews. To accelerate this process, artificial intelligence (AI) can be used in the review process. In this communication paper, we suggest how to conduct a ...
A study evaluating the screening process in 25 'classic' systematic reviews showed that approximately 18% was labelled relevant and 5% was actually included in the reviews. 32 This difference is probably due to more narrow literature searches in 'classic' reviews for feasibility purposes compared with AI-supported reviews, resulting in ...
In contrast, a systematic literature review, through content analysis of research articles, can delve into research nuances that are of interest to researchers ... Literature review. AI is a subfield of computer science dedicated to understanding human thought processes and recreating their effects through information systems.
It is a challenging task for any research field to screen the literature and determine what needs to be included in a systematic review in a transparent way. A new open source machine learning ...
Artificial Intelligence for Literature Reviews: Opportunities and Challenges. This manuscript presents a comprehensive review of the use of Artificial Intelligence (AI) in Systematic Literature Reviews (SLRs). A SLR is a rigorous and organised methodology that assesses and integrates previous research on a given topic.
AI provides methods to represent and infer knowledge, efficiently manipulate texts and learn from vast amount of data. ... A systematic literature review (SLR) is a secondary study that follows a well-established methodology to find relevant papers, extract information from them and properly present their key findings . The literature review is ...
Artificial Intelligence (AI) is increasingly adopted by organizations to innovate, and this is ever more reflected in scholarly work. To illustrate, assess and map research at the intersection of AI and innovation, we performed a Systematic Literature Review (SLR) of published work indexed in the Clarivate Web of Science (WOS) and Elsevier Scopus databases (the final sample includes 1448 ...
This review delineated automated tools and platforms that employ artificial intelligence (AI) approaches and evaluated the reported benefits and challenges in using such methods. A search was conducted in 4 databases (Medline, Embase, CDSR, and Epistemonikos) up to April 2021 for systematic reviews and other related reviews implementing AI methods.
An ongoing umbrella review (a review of reviews) has found that nearly 7,000 reviews (systematic and non-systematic) have been published on AI in the category 'medicine'.
It has been widely adopted as a key source of data for systematic literature review in AI-related fields (Spanaki et al., 2022; Verma et al., 2021). Scopus was selected as the primary source for data collection. The study analyses a corpus of 1455 records from Scopus, a prominent database encompassing abstracts and citations of peer-reviewed ...
The systematic literature review (SLR) is the gold standard in providing research a firm evidence foundation to support decision-making. ... (AI) and Machine Learning Techniques (MLTs) developed with computer programming languages can provide methods to increase the speed, rigour, transparency, and repeatability of SLRs. Aimed towards ...
Follows the Reproducibility and Data Storage Checklist for AI-Aided Systematic Reviews; Download ASReview LAB. In 2 minutes up and running. With the smart project setup features, you can start a new project in minutes. Ready, set, start screening! Create as many projects as you want;
The effective integration of AI tools into the systematic review process has considerable potential to significantly improve efficiency and streamline the research workflow, while accelerating development of new, more targeted tools. ... A comprehensive literature review is a key component of any systematic review, and must be complete and ...
Systematic review is an indispensable tool for optimal evidence collection and evaluation in evidence-based medicine. However, the explosive increase of the original literatures makes it difficult to accomplish critical appraisal and regular update. Artificial intelligence (AI) algorithms have been applied to automate the literature screening procedure in medical systematic reviews.
The purpose of this systematic literature review was to identify and analyze quantitative studies utilizing or integrating AI to address and report clinical-level patient safety outcomes. Methods We restricted our search to the PubMed, PubMed Central, and Web of Science databases to retrieve research articles published in English between ...
Use AI to search, summarize, extract data from, and chat with over 125 million papers. ... Speed up literature review; Find papers they couldn't find elsewhere; Automate systematic reviews and meta-analyses; Learn about a new domain; Elicit tends to work best for empirical domains that involve experiments and concrete results. This type of ...
Ethics in AI becomes a global topic of interest for both policymakers and academic researchers. In the last few years, various research organizations, lawyers, think tankers and regulatory bodies get involved in developing AI ethics guidelines and principles. However, there is still debate about the implications of these principles. We conducted a systematic literature review (SLR) study to ...
This systematic literature review has identified 23 empirical studies which investigate how user trust is defined, factors influencing user trust, and methods for measuring user trust in AI-enabled systems. This section will discuss each research question separately. 4.1.
Over the last four decades, studies have investigated the incorporation of Artificial Intelligence (AI) into education. A recent prominent AI-powered technology that has impacted the education sector is ChatGPT. This article provides a systematic review of 14 empirical studies incorporating ChatGPT into various educational settings, published in 2022 and before the 10th of April 2023—the ...
Table 1 Systematic literature reviews on AI Chatbots in Education. Full size table. It is evident that chatbot technology has a significant impact on overall learning outcomes. Specifically, chatbots have demonstrated significant enhancements in learning achievement, explicit reasoning, and knowledge retention. The integration of chatbots in ...
Utilising a systematic literature review, we examined 64 studies focusing on both statistical and qualitative aspects of AI risk perceptions. This research shows that current publications focus on Asia and North America, with the number of publications increasing significantly over the last three years.
A Systematic Literature Review on Transparencyand Interpretability of AI models in Healthcare:Taxonomies, Tools, Techniques, Datasets, OpenResearch Challenges, and Future Trends June 2024 DOI: 10. ...
Applying the rules and guidelines of systematic reviews is crucial for researchers who undertake this approach (Kitchenham and Charters, 2007).Commencing the review process using a protocol to identify, select, and assess the relevant literature will make the systematic review highly efficient (Tranfield et al., 2003).The systematic process should be reproducible, objective, transparent ...
User intent modeling in natural language processing deciphers user requests to allow for personalized responses. The substantial volume of research (exceeding 13,000 publications in the last decade) underscores the significance of understanding prevalent models in AI systems, with a focus on conversational recommender systems. We conducted a systematic literature review to identify models ...
This systematic literature review addresses the current understanding of AI's potential to optimize energy efficiency and minimize environmental impact in building design, construction, and operation. A comprehensive literature review and synthesis were conducted to identify AI technologies applicable to sustainable building practices, examine ...
GUANGTIAN, Zhang et al. Conceptualizing the Dimensions of Digital Leadership towards Academicians' Competencies in AI: A Systematic Literature Review for Instrument Design. Asian Journal of Research in Education and Social Sciences , [S.l.], v. 6, n. 2, p. 116-132, june 2024.
Internet usage has seen a stark global rise over the last few decades, particularly among adolescents and young people, who have also been diagnosed increasingly with internet addiction (IA). IA impacts several neural networks that influence an adolescent's behaviour and development. This article issued a literature review on the resting-state and task-based functional magnetic resonance ...
Methods A systematic literature review was conducted using a predefined search strategy in MEDLINE. Inclusion criteria encompassed primary studies assessing symptom burden through at-home ePRO surveys in adult cancer patients receiving outpatient systemic antineoplastic treatment, whenever health outcomes were evaluated.
DOI: 10.1080/2050571x.2024.2359274 Corpus ID: 270238629; AI-based automated speech therapy tools for persons with speech sound disorder: a systematic literature review @article{Deka2024AIbasedAS, title={AI-based automated speech therapy tools for persons with speech sound disorder: a systematic literature review}, author={Chinmoy Deka and Abhishek Shrivastava and Ajish K. Abraham and Saurabh ...