INTRUSION DETECTION SYSTEM
- International Journal of Technical Research and Applications 5(2):2320-8163
- 5(2):2320-8163

- Bharati Vidyapeeth College of Engineering, Delhi
- This person is not on ResearchGate, or hasn't claimed this research yet.
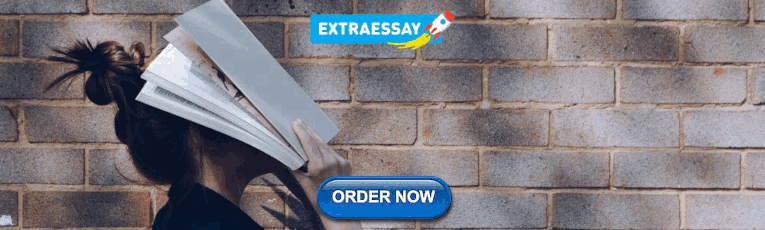
Abstract and Figures

Discover the world's research
- 25+ million members
- 160+ million publication pages
- 2.3+ billion citations

- C.Y.Daramola

- Vaishali Singh
- Shailendra Kumar Ravi
- Kriti Bhushan
- Swanand Arun Yamgar

- Himaathri Premakumar
- Amutha Kunarathinam

- Svetlana Artemova
- Konstantin Starodubov
- Almali Ahmed Adnan Lateef
- Alexander Mikhaylov

- Neha Choudhary

- Dietmar P. F. Möller

- B S Chaitanya
- Vamsee Pavan
- M Nalini Sri
- Jagadeep Vegunta

- Simone Teofili

- E. Lefebvre

- Daron Acemoglu

- Asuman Ozdaglar

- Recruit researchers
- Join for free
- Login Email Tip: Most researchers use their institutional email address as their ResearchGate login Password Forgot password? Keep me logged in Log in or Continue with Google Welcome back! Please log in. Email · Hint Tip: Most researchers use their institutional email address as their ResearchGate login Password Forgot password? Keep me logged in Log in or Continue with Google No account? Sign up
Smart Grid Intrusion Detection Based on Device Fingerprint Technology
New citation alert added.
This alert has been successfully added and will be sent to:
You will be notified whenever a record that you have chosen has been cited.
To manage your alert preferences, click on the button below.
New Citation Alert!
Please log in to your account
Information & Contributors
Bibliometrics & citations, recommendations, machine learning-based intrusion detection for smart grid computing: a survey.
Machine learning (ML)-based intrusion detection system (IDS) approaches have been significantly applied and advanced the state-of-the-art system security and defense mechanisms. In smart grid computing environments, security threats have been ...
Correlation of Intrusion Detection Information in Grid Environments
This paper presents a novel intrusion detection system for Grid Systems. It is intended to identify potential attackers who try to modify or compromise the applications sent to execution by various users or target different resource groups within the ...
An Intrusion Detection Scheme in TCP/IP Networks Based on Flow-Net and Fingerprint
Based on our previous work for a novel logging methodology, called flow-net, we propose an Intrusion Detection System (IDS) using Flow-Net Based Fingerprint (IDS-FF) in this paper. We apply the IDS-FF scheme in TCP/IP (Transmission Control Protocol/...
Information
Published in.

Association for Computing Machinery
New York, NY, United States
Publication History
Permissions, check for updates, author tags.
- Deep learning
- Device fingerprint
- Intrusion detection
- Research-article
- Refereed limited
Contributors
Other metrics, bibliometrics, article metrics.
- 0 Total Citations
- 1 Total Downloads
- Downloads (Last 12 months) 1
- Downloads (Last 6 weeks) 1
View Options
Login options.
Check if you have access through your login credentials or your institution to get full access on this article.
Full Access
View options.
View or Download as a PDF file.
View online with eReader .
HTML Format
View this article in HTML Format.
Share this Publication link
Copying failed.
Share on social media
Affiliations, export citations.
- Please download or close your previous search result export first before starting a new bulk export. Preview is not available. By clicking download, a status dialog will open to start the export process. The process may take a few minutes but once it finishes a file will be downloadable from your browser. You may continue to browse the DL while the export process is in progress. Download
- Download citation
- Copy citation
We are preparing your search results for download ...
We will inform you here when the file is ready.
Your file of search results citations is now ready.
Your search export query has expired. Please try again.
- DOI: 10.1016/j.rineng.2024.102659
- Corpus ID: 271684117
An RFE/Ridge-ML/DL based anomaly intrusion detection approach for securing IoMT system
- Ghita Lazrek , Kaouthar Chetioui , +2 authors Moulhime El bekkali
- Published in Results in Engineering 1 August 2024
- Engineering, Computer Science
50 References
Meta-ids: meta-learning-based smart intrusion detection system for internet of medical things (iomt) network, deep learning-empowered intrusion detection framework for the internet of medical things environment, security information event management data acquisition and analysis methods with machine learning principles, twi-ftm: two-way iot-fog trust management scheme for task offloading in iot-fog networks, a framework for application-centric internet of things authentication, smarthealth: an intelligent framework to secure iomt service applications using machine learning, a secure ensemble learning-based fog-cloud approach for cyberattack detection in iomt, machine learning based intrusion detection system for iomt, cost-effective detection system of cross-site scripting attacks using hybrid learning approach, safetymed: a novel iomt intrusion detection system using cnn-lstm hybridization, related papers.
Showing 1 through 3 of 0 Related Papers
An Effective Ensemble Classification Algorithm for Intrusion Detection System
- Conference paper
- First Online: 13 August 2024
- Cite this conference paper
- Jun-Ping Wang 11 ,
- Ti-Ling Wang 11 ,
- Yu-Hsuan Wu 11 &
- Chun-Wei Tsai 11
Part of the book series: Communications in Computer and Information Science ((CCIS,volume 2144))
Included in the following conference series:
- Asian Conference on Intelligent Information and Database Systems
Using machine learning algorithm to build detection model of an intrusion detection system (IDS) to detect abnormal behaviors is an effective way that can be found in several studies; however, some different abnormal behaviors have similar characteristics that are quite difficult to be distinguished by using a single one detection model. To effectively identify such abnormal behaviors, the proposed method will construct a certain number of classifiers for different abnormal behaviors as a hierarchical and ensemble classification (detection) model. The proposed IDS will also adopt the domain adaptation method to remove the irrelevant augmented data because some of them may be assigned with incorrect labels during the augmentation process. Experimental results show that the proposed method can outperform other classification methods in terms of accuracy and recall such as machine learning, ensemble learning, and deep learning methods. It is shown that the proposed method can provide a promising design to detect different types of malicious intrusions.
This is a preview of subscription content, log in via an institution to check access.
Access this chapter
Subscribe and save.
- Get 10 units per month
- Download Article/Chapter or eBook
- 1 Unit = 1 Article or 1 Chapter
- Cancel anytime
- Available as PDF
- Read on any device
- Instant download
- Own it forever
- Available as EPUB and PDF
- Compact, lightweight edition
- Free shipping worldwide - see info
Tax calculation will be finalised at checkout
Purchases are for personal use only
Institutional subscriptions
Farnaaz, N., Jabbar, M.: Random forest modeling for network intrusion detection system. Procedia Comput. Sci. 89 , 213–217 (2016)
Article Google Scholar
Ahmad, Z., Shahid Khan, A., Wai Shiang, C., Abdullah, J., Ahmad, F.: Network intrusion detection system: a systematic study of machine learning and deep learning approaches. Trans. Emerg. Telecommun. Technol. 32 (1), 4150–4178 (2021)
Google Scholar
Selvakumar, B., Muneeswaran, K.: Firefly algorithm based feature selection for network intrusion detection. Comput. Secur. 81 , 148–155 (2019)
Li, X., Chen, W., Zhang, Q., Wu, L.: Building autoencoder intrusion detection system based on random forest feature selection. Comput. Secur. 95 , 101851–101865 (2020)
Gao, X., Shan, C., Hu, C., Niu, Z., Liu, Z.: An adaptive ensemble machine learning model for intrusion detection. IEEE Access 7 , 82512–82521 (2019)
Tama, B.A., Comuzzi, M., Rhee, K.-H.: TSE-IDS: a two-stage classifier ensemble for intelligent anomaly-based intrusion detection system. IEEE Access 7 , 94497–94507 (2019)
Yin, C., Zhu, Y., Fei, J., He, X.: A deep learning approach for intrusion detection using recurrent neural networks. IEEE Access 5 , 21954–21961 (2017)
Javaid, A., Niyaz, Q., Sun, W., Alam, M.: A deep learning approach for network intrusion detection system. In: Proceedings of the EAI International Conference on Bio-inspired Information and Communications Technologies, pp. 21–26 (2016)
Shone, N., Ngoc, T.N., Phai, V.D., Shi, Q.: A deep learning approach to network intrusion detection. IEEE Trans. Emerg. Top. Comput. Intell. 2 (1), 41–50 (2018)
Wang, M., Deng, W.: Deep visual domain adaptation: a survey. Neurocomputing 312 , 135–153 (2018)
Lu, C.-T., Tsai, C.-W.: An effective adaptive stacking ensemble algorithm for electricity theft detection. In: Proceedings of the ACM International Conference on Intelligent Computing and its Emerging Applications, pp. 22–27 (2021)
Shapoorifard, H., Shamsinejad, P.: Intrusion detection using a novel hybrid method incorporating an improved \(k\) NN. Int. J. Comput. Appl. 173 (1), 5–9 (2017)
Malik, A.J., Khan, F.A.: A hybrid technique using binary particle swarm optimization and decision tree pruning for network intrusion detection. Clust. Comput. 21 , 667–680 (2018)
Kumar, G., Thakur, K., Ayyagari, M.R.: MLEsIDSs: machine learning-based ensembles for intrusion detection systems–a review. J. Supercomput. 76 , 8938–8971 (2020)
Aburomman, A.A., Reaz, M.B.I.: A survey of intrusion detection systems based on ensemble and hybrid classifiers. Comput. Secur. 65 , 135–152 (2017)
Rajagopal, S., Kundapur, P.P., Hareesha, K.S.: A stacking ensemble for network intrusion detection using heterogeneous datasets. Secur. Commun. Netw. 2020 , 1–9 (2020)
He, K., Kim, D.D., Asghar, M.R.: Adversarial machine learning for network intrusion detection systems: a comprehensive survey. IEEE Commun. Surv. Tutor. 25 (1), 538–566 (2023)
Vinayakumar, R., Alazab, M., Soman, K.P., Poornachandran, P., Al-Nemrat, A., Venkatraman, S.: Deep learning approach for intelligent intrusion detection system. IEEE Access 7 , 41525–41550 (2019)
Rm, S.P., et al.: An effective feature engineering for DNN using hybrid PCA-GWO for intrusion detection in IoMT architecture. Comput. Commun. 160 , 139–149 (2020)
Xiao, Y., Xing, C., Zhang, T., Zhao, Z.: An intrusion detection model based on feature reduction and convolutional neural networks. IEEE Access 7 , 42210–42219 (2019)
Chawla, A., Lee, B., Fallon, S., Jacob, P.: Host based intrusion detection system with combined CNN/RNN model. In: Proceedings of the Joint European Conference on Machine Learning and Knowledge Discovery in Databases, pp. 149–158 (2019)
Li, P., Pei, Y., Li, J.: A comprehensive survey on design and application of autoencoder in deep learning. Appl. Soft Comput. 138 , 110176 (2023)
Motiian, S., Piccirilli, M., Adjeroh, D.A., Doretto, G.: Unified deep supervised domain adaptation and generalization. In: Proceedings of the IEEE International Conference on Computer Vision, pp. 5715–5725 (2017)
Liu, M.-Y., Tuzel, O.: Coupled generative adversarial networks. In: Proceedings of the Advances in Neural Information Processing Systems, pp. 469–477 (2016)
Ganin, Y., Lempitsky, V.: Unsupervised domain adaptation by backpropagation. In: Proceedings of the International Conference on Machine Learning, pp. 1180–1189 (2015)
Schuster, M., Paliwal, K.K.: Bidirectional recurrent neural networks. IEEE Trans. Signal Process. 45 (11), 2673–2681 (1997)
Dai, W., Yang, Q., Xue, G.-R., Yu, Y.: Boosting for transfer learning. In: Proceedings of the International Conference on Machine Learning, pp. 193–200 (2007)
Tavallaee, M., Bagheri, E., Lu, W., Ghorbani, A.A.: A detailed analysis of the KDD CUP 99 data set. In: Proceedings of the IEEE Symposium on Computational Intelligence for Security and Defense Applications, pp. 1–6 (2009)
Rao, B.B., Swathi, K.: Fast \(k\) NN classifiers for network intrusion detection system. Indian J. Sci. Technol. 10 (14), 1–10 (2017)
Rai, K., Devi, M.S., Guleria, A.: Decision tree based algorithm for intrusion detection. Int. J. Adv. Netw. Appl. 7 (4), 2828–2834 (2016)
Anton, S.D.D., Sinha, S., Schotten, H.D.: Anomaly-based intrusion detection in industrial data with SVM and random forests. In: Proceedings of the International Conference on Software, Telecommunications and Computer Networks, pp. 1–6 (2019)
Tang, X., Tan, S.X.-D., Chen, H.-B.: SVM based intrusion detection using nonlinear scaling scheme. In: Proceedings of the IEEE International Conference on Solid-state and Integrated Circuit Technology (ICSICT), pp. 1–4 (2018)
Hsu, C.-J.: An effective semi-supervised learning method for intrusion detection system. Master’s thesis, National Sun Yat-sen University, Taiwan (2021)
Xu, W., Jang-Jaccard, J., Singh, A., Wei, Y., Sabrina, F.: Improving performance of autoencoder-based network anomaly detection on NSL-KDD dataset. IEEE Access 9 , 140136–140146 (2021)
Chen, T., Guestrin, C.: XGBoost: a scalable tree boosting system. In: Proceedings of the ACM SIGKDD International Conference on Knowledge Discovery and Data Mining, pp. 785–794 (2016)
Tang, T.A., Mhamdi, L., McLernon, D., Zaidi, S.A.R., Ghogho, M.: Deep recurrent neural network for intrusion detection in SDN-based networks. In: Proceedings of the IEEE Conference on Network Softwarization and Workshops, pp. 202–206 (2018)
Laghrissi, F., Douzi, S., Douzi, K., Hssina, B.: IDS-attention: an efficient algorithm for intrusion detection systems using attention mechanism. J. Big Data 8 (1), 149–169 (2021)
He, H., Bai, Y., Garcia, E.A., Li, S.: ADASYN: adaptive synthetic sampling approach for imbalanced learning. In: Proceedings of the IEEE International Joint Conference on Neural Networks, pp. 1322–1328 (2008)
Tang, T.A., Mhamdi, L., McLernon, D., Zaidi, S.A.R., Ghogho, M.: Deep learning approach for network intrusion detection in software defined networking. In: Proceedings of the International Conference on Wireless Networks and Mobile Communications (WINCOM), pp. 258–263 (2016)
Download references
Acknowledgment
This research was partially supported by the National Science and Technology Council (NSTC) of Taiwan, R.O.C., under the grant numbers NSTC-111-2222-E-110-006-MY3, NSTC-112-2628-E-110-001-MY3, and NSTC-112-2634-F-110-001-MBK.
Author information
Authors and affiliations.
Computer Science and Engineering, National Sun Yat-sen University, Kaohsiung, Taiwan
Jun-Ping Wang, Ti-Ling Wang, Yu-Hsuan Wu & Chun-Wei Tsai
You can also search for this author in PubMed Google Scholar
Corresponding author
Correspondence to Chun-Wei Tsai .
Editor information
Editors and affiliations.
Wrocław University of Science and Technology, Wrocław, Poland
Ngoc Thanh Nguyen
University of Pau and Adour Countries, Pau, France
Richard Chbeir
Open University of Cyprus, Latsia, Cyprus
Yannis Manolopoulos
Iwate Prefectural University, Takizawa, Japan
Hamido Fujita
National University of Kaohsiung, Kaohsiung, Taiwan
Tzung-Pei Hong
Japan Advanced Institute of Science and Technology, Nomi, Japan
Le Minh Nguyen
University of Science and Technology, Wrocław, Poland
Krystian Wojtkiewicz
Rights and permissions
Reprints and permissions
Copyright information
© 2024 The Author(s), under exclusive license to Springer Nature Singapore Pte Ltd.
About this paper
Cite this paper.
Wang, JP., Wang, TL., Wu, YH., Tsai, CW. (2024). An Effective Ensemble Classification Algorithm for Intrusion Detection System. In: Nguyen, N.T., et al. Recent Challenges in Intelligent Information and Database Systems. ACIIDS 2024. Communications in Computer and Information Science, vol 2144. Springer, Singapore. https://doi.org/10.1007/978-981-97-5937-8_5
Download citation
DOI : https://doi.org/10.1007/978-981-97-5937-8_5
Published : 13 August 2024
Publisher Name : Springer, Singapore
Print ISBN : 978-981-97-5936-1
Online ISBN : 978-981-97-5937-8
eBook Packages : Computer Science Computer Science (R0)
Share this paper
Anyone you share the following link with will be able to read this content:
Sorry, a shareable link is not currently available for this article.
Provided by the Springer Nature SharedIt content-sharing initiative
- Publish with us
Policies and ethics
- Find a journal
- Track your research
Thank you for visiting nature.com. You are using a browser version with limited support for CSS. To obtain the best experience, we recommend you use a more up to date browser (or turn off compatibility mode in Internet Explorer). In the meantime, to ensure continued support, we are displaying the site without styles and JavaScript.
- View all journals
- Explore content
- About the journal
- Publish with us
- Sign up for alerts
- Open access
- Published: 01 August 2024
MPE-YOLO: enhanced small target detection in aerial imaging
- Yichang Qin 1 ,
- Ze Jia 1 &
- Ben Liang 1
Scientific Reports volume 14 , Article number: 17799 ( 2024 ) Cite this article
408 Accesses
Metrics details
- Aerospace engineering
- Electrical and electronic engineering
Aerial image target detection is essential for urban planning, traffic monitoring, and disaster assessment. However, existing detection algorithms struggle with small target recognition and accuracy in complex environments. To address this issue, this paper proposes an improved model based on YOLOv8, named MPE-YOLO. Initially, a multilevel feature integrator (MFI) module is employed to enhance the representation of small target features, which meticulously moderates information loss during the feature fusion process. For the backbone network of the model, a perception enhancement convolution (PEC) module is introduced to replace traditional convolutional layers, thereby expanding the network’s fine-grained feature processing capability. Furthermore, an enhanced scope-C2f (ES-C2f) module is designed, utilizing channel expansion and stacking of multiscale convolutional kernels to enhance the network’s ability to capture small target details. After a series of experiments on the VisDrone, RSOD, and AI-TOD datasets, the model has not only demonstrated superior performance in aerial image detection tasks compared to existing advanced algorithms but also achieved a lightweight model structure. The experimental results demonstrate the potential of MPE-YOLO in enhancing the accuracy and operational efficiency of aerial target detection. Code will be available online (https://github.com/zhanderen/MPE-YOLO).
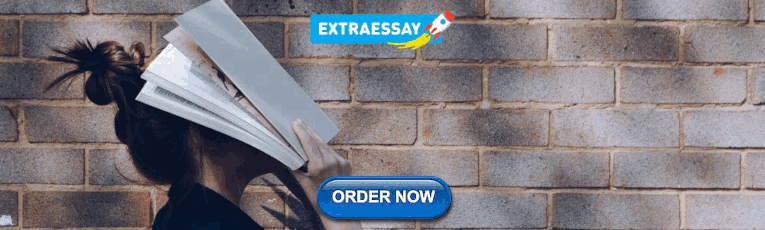
Similar content being viewed by others

Improved GBS-YOLOv5 algorithm based on YOLOv5 applied to UAV intelligent traffic
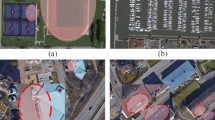
Centralised visual processing center for remote sensing target detection
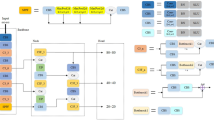
Lightweight aerial image object detection algorithm based on improved YOLOv5s
Introduction.
Aerial images, acquired through aerial photography technology, feature high-resolution and extensive area coverage, providing critical support to fields such as traffic monitoring 1 and disaster relief 2 through the automated extraction and analysis of geographic information. With continuous advancements in remote sensing technology, aerial image detection offers valuable data support for geographic information systems and related applications, playing a significant role in enhancing the identification and monitoring of surface objects and the development of geographic information technology.
Aerial images are characterized by complex terrain, varying light conditions, and difficulties in data acquisition and storage. However, the high-dimensionality and massive volume of aerial image data pose numerous challenges to image detection, particularly because aerial images often contain small targets, making detection even more challenging 3 . In light of these issues, target detection algorithms are increasingly vital as the core technology for aerial image analysis.
Traditional object detection algorithms often rely on manually designed feature extraction methods such as scale-invariant feature transform (SIFT), and speeded up robust feature (SURF). These methods represent targets by extracting local features from images but might fail to capture higher-level semantic information. Machine learning approaches such as support vector machines (SVMs) 4 , random forests 5 , etc., have effectively improved the accuracy and efficiency of aerial detection, but struggle with the detection of complex backgrounds. With the rapid development of deep learning technology, neural network-based image object detection methods have become mainstream. The end-to-end learning capability of deep learning allows algorithms to automatically learn and extract more abstract and higher-level semantic features, replacing traditionally manually designed features.
Deep learning-based object detection algorithms can be divided into single-stage and two-stage algorithms. The two-stage algorithms are represented by the R-CNN 6 , 7 , 8 series, which adopts a two-stage detection process; First candidate regions are created via the region proposal network (RPN), and then the location and classification are fine-tuned through classifiers and regressors. Such algorithms can precisely locate and identify various complex land objects, especially when dealing with small or densely arranged targets, and have received widespread attention and application. However, two-stage detection algorithms still have room for improvement in terms of speed and efficiency. Single-stage detection algorithms, represented by SSD 9 and YOLO 10 , 11 , 12 , 13 , 14 , 15 , 16 , 17 series, approach object detection as a regression problem and predict the categories and locations of targets directly from the global image, enabling real-time detection. These algorithms offer good real-time performance and accuracy, and are particularly suitable for processing large-scale aerial image data. They hold significant application prospects for quickly obtaining geographic information, monitoring urban changes, and natural disasters. However, single-stage object detection algorithms still face challenges in the accurate detection and positioning of small targets.
In the context of UAV aerial imagery, object detection encounters several specific challenges:
Dense small objects and occlusion Images captured from low altitudes often contain a large number of dense small objects, particularly in urban or complex terrains. Due to the considerable distance, these objects appear smaller in the images and are prone to occlusion. For instance, buildings might obscure each other, or trees might cover parked vehicles. Such occlusion leads to partial hiding of target object features, thereby affecting the performance of detection algorithms. Even advanced detection algorithms struggle to accurately identify and locate all objects in highly dense and severely occluded environments.
Real-time requirements vs. accuracy trade-off UAV aerial image object detection must meet real-time requirements, particularly in monitoring and emergency response scenarios. Achieving real-time detection necessitates a reduction in algorithmic computational complexity, which frequently conflicts with detection accuracy. High-accuracy detection algorithms typically require substantial computational resources and time, whereas real-time demands necessitate algorithms that can process vast amounts of data swiftly. The challenge lies in maintaining high detection accuracy while ensuring real-time performance. This requires optimization in network architecture to balance the number of parameters and accuracy effectively.
Complex backgrounds Aerial images often include a significant amount of irrelevant background information like buildings, trees, and roads. The complexity and diversity of background information can interfere with the correct detection of small objects. Moreover, the features of small objects are inherently less pronounced. Traditional single-stage and two-stage algorithms primarily focus on global features and may overlook the fine-grained features crucial for detecting small objects. These algorithms often fail to capture the details of small objects, resulting in lower detection accuracy. Therefore, there is a pressing need for more advanced deep learning models and algorithms that can handle these subtle features, thereby enhancing the accuracy of small object detection.
To address the aforementioned issues, this study proposes an algorithm called MPE-YOLO, which is based on the YOLOv8 model, and enhances the detection accuracy of small objects while maintaining a lightweight model. The main contributions of this study are as follows.
We developed a multilevel feature integrator (MFI) module with a hierarchical structure to merge image features at different levels, enhancing scene comprehension and boosting object detection accuracy.
A perception enhancement convolution (PEC) module is proposed, which uses multislice operations and channel dimension concatenation to expand the receptive field, thereby improving the model’s ability to capture detailed target information.
By incorporating the proposed enhanced scope-C2f (ES-C2f) operation and introducing an efficient feature selection and utilization mechanism, the selective use of features is further enhanced, effectively improving the accuracy and robustness of small object detection.
After comprehensive comparative experiments with various other object detection models, MPE-YOLO has demonstrated a significant improvement in performance , proving its effectiveness.
The rest of this paper includes the following content: Section 2 briefly introduces the recent research results on aerial image detection and the main idea of YOLOv8. Section 3 introduces the innovations of this paper. Section 4 describes the experimental setup, including the experimental environment, parameter configuration, datasets used, and performance evaluation metrics, and presents detailed experimental steps and results, verifying the effectiveness of the improvement strategies. Section 5 summarizes the main contributions of this research and discusses future directions of work.
Background and related works
Related works.
Deep learning-based object detection algorithms are widely applied in fields such as aerial image detection, medical image processing, precision agriculture, and robotics due to their high detection accuracy and inference speed. The following are some algorithms used in aerial image detection: Cheng et al. 18 proposed a method combining cross-scale feature fusion to enhance the network’s ability to distinguish similar objects in aerial images. Guo et al. 19 presented a novel object detection algorithm that improves the accuracy and efficiency of highway intrusion detection by refining feature extraction, feature fusion, and computational complexity methods. Sahin et al. 20 introduced YOLODrone, an improved version of the YOLOv3 algorithm that increases the number of detection layers to enhance the model’s capability to detect objects of various sizes, although this adds to the model’s complexity. Chen et al. 21 enhanced the feature extraction capability of the model by optimizing residual blocks in the multi-level local structure of DW-YOLO and improved accuracy by increasing the number of convolution kernels. Zhu et al. 22 incorporated the CBAM attention mechanism into the YOLOv5 model to address the issue of blurred objects in aerial images. Additionally, Yang 23 enhanced small object detection capability by adding upsampling in the neck part of the YOLOv5 network. And integrated an image segmentation layer into the detection network. Lin et al. 24 proposed GDRS-YOLO, which first constructs multi-scale features through deformable convolution and gathering-dispersing mechanisms, and then introduces normalized Wasserstein distance for mixed loss training, effectively improving the accuracy of object detection in remote sensing images. Jin et al. 25 improved the robustness and generalization of UAV image detection under different shooting conditions by decomposing domain-invariant features, domain-specific features, and using balanced sampling data augmentation techniques. Bai et al.’s CCNet 26 suppresses interference in deep feature maps using high-level RGB feature maps while achieving cross-modality interaction, enhancing salient object detection.
In the field of medical image processing, typical object detection algorithms include: Pacal et al. 27 demonstrated that by improving the YOLO algorithm and using the latest data augmentation and transfer learning techniques, the efficiency and accuracy of polyp detection could be significantly enhanced. Xu et al. 28 showed that the improved Faster R-CNN model exhibited excellent performance in lung nodule detection, particularly in small object detection capability and overall detection accuracy.Xi et al. 29 improved the sensitivity of small object detection by introducing a super-resolution reconstruction branch and an attention fusion module in the MSP-YOLO network. In the agricultural field, Zhu et al. 30 demonstrated how to achieve high-precision drone control systems through a combination of hardware and software. Its application in agricultural spraying provides a reference for the performance of automated control systems in practical applications. In the field of robotics, Wang et al. 31 researched robotic mechanical models and optimized jumping behavior through bionic methods. This combination of biological observation and mechanical modeling can inspire the development of other robots or systems that require motion optimization, using bionic mechanisms to achieve efficient and reliable motion control.
The aforementioned methods face challenges such as the limitations of the receptive field and insufficient feature fusion in highly complex backgrounds or dense small object scenes, resulting in poor performance in low-resolution and densely occluded situations. Driven by these motivations, we propose an algorithm called MPE-YOLO that improves the detection accuracy of small objects while maintaining a lightweight model. Numerous experiments have demonstrated that by integrating multilevel features and strengthening detail information perception modules, we can achieve higher detection accuracy across different datasets.
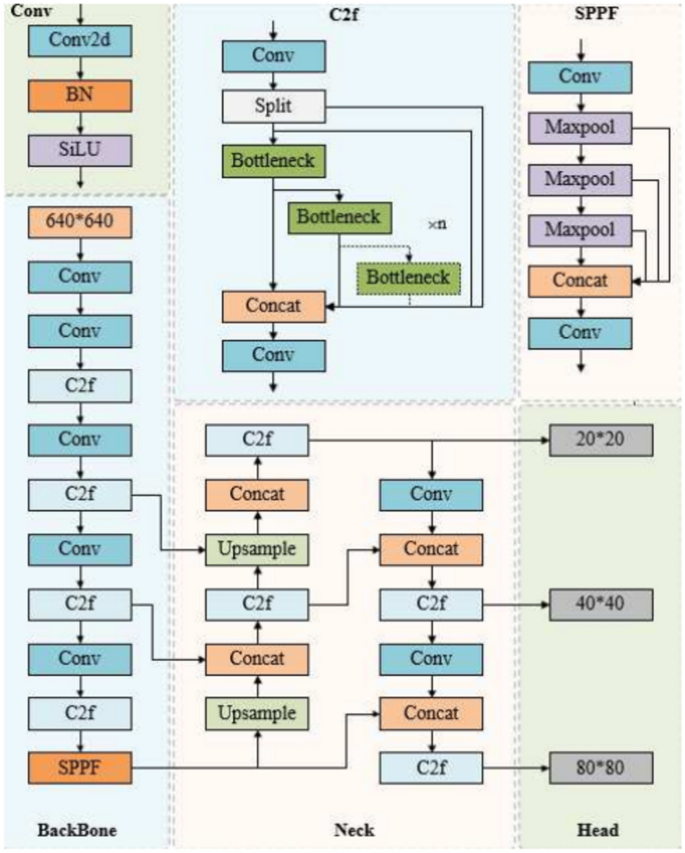
YOLOv8 network structure.
YOLOv8 is the latest generation of object detection algorithms developed by Ultralytics, and officially released on January 10, 2023. YOLOv8 improves upon YOLOv5 by replacing the C3 module with the C2f module. The head utilizes a contemporary decoupled head structure, separating classification and detection heads, and transitions from an anchor-based to an anchor-free approach, resulting in higher detection accuracy and speed. The YOLOv8 model comprises an input layer, a backbone network, a neck network, and a head network, as shown in Fig. 1 . The input image is first resized to 640x640 to meet the size requirements of the input layer, and the backbone network achieves downsampling and feature extraction via multiple convolutional operations, with each convolutional layer equipped with batch normalization and SiLU 32 activation functions. To improve the network’s gradient flow and feature extraction capacity, the C2f block was introduced, drawing on the E-ELAN structure from YOLOv7, and employing multilayer branch connections. Furthermore, the SPPF 33 block is positioned at the end of the backbone network and combines multiscale feature processing to enhance the feature abstraction capability. The neck network adopts the FPN 34 and PAN 35 structures for effective fusion of different scale feature maps, which are then passed on to the head network. The head network is designed in a decoupled manner, including two parallel convolutional branches that handle regression and classification tasks separately to improve focus and performance on each task. The YOLOv8 series offers five different scaled models for users to choose from, including YOLOv8n, YOLOv8s, YOLOv8m, YOLOv8l, and YOLOv8x. Compared to other models, YOLOv8s strikes a balance between accuracy and model complexity. Therefore, this study chooses YOLOv8s as the baseline network.
Methodology
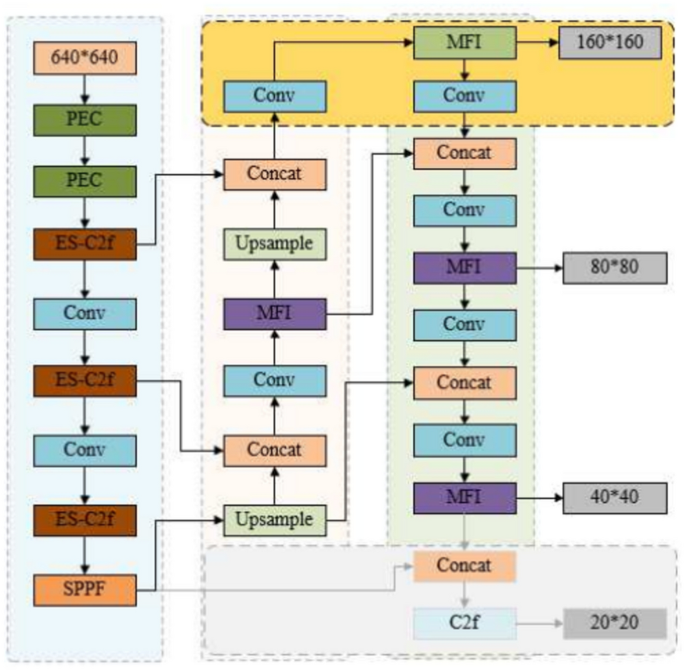
MPE-YOLO network structure.
In response to the need for detecting small objects in aerial and drone imagery, we propose the MPE-YOLO algorithm to adjust the structure of the original YOLOv8 components. As shown in Fig. 2 , by designing the multilevel feature integrator (MFI) module, the representation and information fusion of small target features are optimized, so as to reduce the information loss in the process of feature fusion. The introduction of the perception enhancement convolution (PEC) module replaces the traditional convolutional layer, expands the ability of fine-grained feature processing of the network, and significantly improves the recognition accuracy of small targets in complex backgrounds. We replaced the last two downsampling layers and the detection layer for 20*20 size targets in the backbone network with a detection layer for small 160*160 size targets. This enables the model to focus more on the details of small targets. Finally, through the enhanced scope-C2f (ES-C2f) module, the feature extraction efficiency and operation efficiency of the model are further improved by using channel expansion and the stacking of multi-scale convolution kernels. Combining these improvements, MPE-YOLO performs well in small object detection tasks in complex environments, and significantly improves the accuracy and performance of the model. To differentiate from the baseline model, MPE-YOLO marks the improved modules with darker colors. The gray area at the bottom represents the removal of the 20*20 detection head, while the yellow area at the top represents the addition of the 160*160 detection head.
Multilevel feature integrator
In object detection tasks, the feature representation of small objects is often unclear due to size restrictions, which can lead to them being overlooked or lost in the feature fusion process, resulting in decreased detection performance. To effectively address this issue, we adopted the structure of Res2Net 36 and designed an innovative multilevel feature integrator (MFI). The structure of the MFI module, as shown in Fig. 3 , aims to optimize the feature representation and information fusion of small objects through a series of detailed strategies, reducing the loss of feature information and suppressing redundancy and noise.
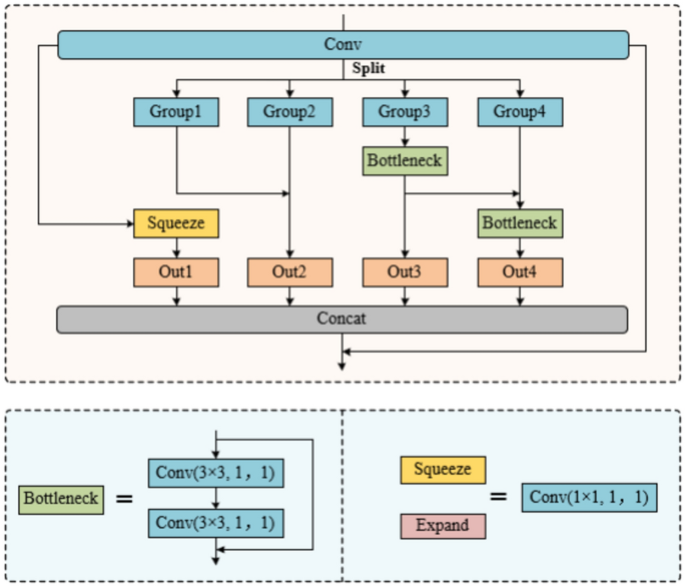
Multilevel feature integrator structure.
First, the MFI module uses convolutional operations to reduce the channel dimensions of the input feature maps, simplifying the subsequent computation process. Immediately following, the reduced feature maps are uniformly divided into four groups (Group 1 to Group 4), with each group containing 25% of the total number of original feature maps. This partition is not random, but a uniform segmentation of the number of channels based on the feature map, aiming to optimize the computational efficiency and the subsequent feature fusion effect. We use a squeeze convolution layer to shape and compress the feature maps from all groups, resulting in output Out1, which aims to focus on key target features, reduce feature redundancy, and preserve details helpful for small object detection. Second, by performing proportional feature fusion of Group 1 and Group 2, we construct complex low-level feature representations, forming the output part Out2, and enhancing the feature details of small objects. Additionally, the bottleneck module 17 is applied to Group 3 to refine high-level semantic information, and produce Out3. This advanced feature output helps capture richer contextual information, improving the detection efficiency of small objects.
Out4 is obtained by fusing the high-level features from Out3 with the Group4 features and then processing them again through the bottleneck module. The purpose of this step is to integrate the low-level features with the high-level features, enabling the model to understand the characteristics of small objects more comprehensively. Then by concatenating and integrating the four different levels of outputs-Out1, Out2, Out3, and Out4-in the channel direction, the features of all the scales are fully utilized, thereby improving the overall performance of the model in small object detection tasks.
Ultimately, MFI module adopts a channel-wise feature integration approach to aggregate features from various levels, enhancing the ability to recognize different target behaviors, particularly improving the accuracy of capturing small object behaviors and interactions in dynamic scenes.
Perception enhancement convolution
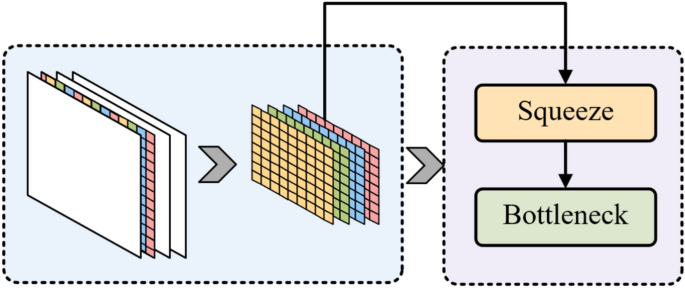
Perception enhancement convolution structure.
When dealing with multiscale object detection tasks, traditional convolutional neural networks typically face challenges such as fixed receptive fields 37 , insufficient use of context information, and limited environmental perception. In particular, in the detection of small objects, these limitations can significantly suppress the performance of the model. To overcome these issues, we introduce Perception-Enhanced Convolution (PEC), as shown in Fig. 4 , which is a module specifically designed for the backbone network and intended to replace traditional convolutional layers. The main advantage of PEC is that it introduces a new dimension during the phase of extracting primary features in the model, which can significantly expand the receptive field and more effectively integrate context information, thus further deepening the model’s understanding of small objects and their environment.
In detail, the PEC module begins by precisely cutting the input feature map into four smaller feature map blocks, each of which is reduced in size by half in the spatial dimension. This cutting process involves the selection of specific pixels, ensuring that representative information from the top-left, top-right, bottom-left, and bottom-right of the original feature map is captured separately in each channel. Through such a meticulous division of the spatial dimension, the resulting small blocks retain important spatial information while ensuring even coverage of information. Subsequently, these small blocks are concatenated in the channel dimension to form a new feature map, with an increased number of channels but reduced spatial resolution, thus significantly reducing the computational burden while maintaining a large receptive field.
To further enhance feature expressiveness and computational efficiency, a squeeze layer is integrated into the PEC, which reduces model parameters by compressing feature dimensions while ensuring that key features are emphasized even as the model is simplified. For deeper feature extraction, we apply the classic bottleneck structure, which not only refines the hierarchical representation of features but also significantly enhances the model’s sensitivity and cognitive ability for small objects, further boosting the computational efficiency of features.
Overall, through the PEC module, the model is endowed with stronger environmental adaptability and understanding of object relations. The innovative design of the PEC enables feature maps to obtain more comprehensive and detailed information on targets and the environment while expanding the receptive field. This is particularly crucial in areas such as traffic monitoring for object classification and behavior prediction, as these aeras greatly depend on accurate interpretations of subtle changes and complex scenes.
Enhanced Scope-C2f
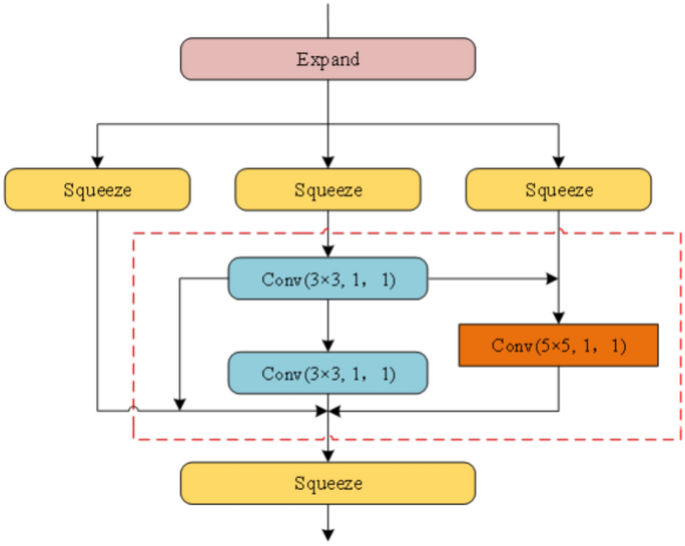
Enhanced Scope-C2f structure.
In the YOLOv8 model, researchers designed the C2f module 17 to maintain a lightweight network while obtaining richer gradient flow information. However, when dealing with small targets or low-contrast targets in aerial images, this module does not sufficiently express fine features, affecting the detection accuracy of targets with complex scales. To address this issue, this study proposes an improved module called Enhanced Scope-C2f (ES-C2f), as shown in Fig. 5 , which focuses on improving the network’s ability to capture details and feature utilization efficiency, especially in expressing small targets and low-contrast targets.
The ES-C2f module enhances the network’s representation capability for targets by expanding the channel capacity of feature maps, enabling the model to capture more subtle feature variations. This strategy is dedicated to enhancing the network’s sensitivity to small target details and improving the adaptability to low-contrast target environments through a wider range of feature representations.
To expand the channel capacity while considering computational efficiency, the ES-C2f module cleverly integrates a series of squeeze layers. These layers perform intelligent selection and compression of feature channels, not only streamlining feature representations but also preserving the capture of key information. The design of this feature operation fully considers the need to enhance identification capabilities while reducing model complexity and computational load. ES-C2f further employs a strategy of stacking multiscale convolutional kernels as well as combining local and global features. This provides an effective means to integrate features at different levels, enabling the model to make decisions on a richer feature dimension. Deep semantic information is cleverly woven with shallow texture details, enhancing the perception of scale diversity.
An optimized squeeze layer is introduced at the end of the module to further refine the essence of the features and adapt to the needs of subsequent processing layers. This engineering not only enhances the feature representation capacity but also improves the information decoding efficiency of subsequent layers, allowing the model to detect and recognize targets with greater precision. With the improvements made to the original C2f module in the YOLOv8 architecture, the proposed ES-C2f module provides a more effective solution for small targets and low-contrast scenes. The ES-C2f module not only maintains the lightweight structure and response speed of the model in extremely challenging scenarios but also significantly improves the overall recognition ability for complex-scale target detection.
Experiments
Experimental setup.
The batch size was set to 4 to avoid memory overflow, the learning rate was set to 0.01, the learning rate was adjusted by the cosine annealing algorithm, the momentum of the stochastic gradient descent (SGD) was set to 0.937, and the mosaic method was used for data augmentation. The resolution of the input graphics is uniformly set to 640 \(\times \) 640. A total of 200 epochs were trained on all models, and no pretrained models were used in training to ensure the fairness of the experiment. We opted for random weight initialization, ensuring that the initial weights of each model originate from the same distribution. Although the specific initial values differ, this guarantees that all models start from a fair and balanced point, enabling comparison under identical training conditions without the influence of historical biases from pretrained models. Pretrained models are typically trained on large datasets that may not align with our target dataset distribution, potentially introducing unforeseen biases. Therefore, we decided against using pretrained models. To mitigate the impact of randomness in weight initialization, we conducted multiple independent experiments and averaged the results. Table 1 lists the training environment configurations.
To ensure the rationality of the experimental data, this article selected three representative public datasets for experiments, namely VisDrone2019 38 , RSOD 39 , and AI-TOD 40 . VisDrone2019, as the main dataset of this experiment, was subjected to very detailed comparative and ablation studies. To validate the generalizability and universality of the model, experiments were conducted on the RSOD and AI-TOD datasets.
Considering the consistency of the dataset and the continuity of the study, we selected the VisDrone2019 dataset, it collected and released by Tianjin University’s Machine Learning and Data Mining Lab, comprises a total of 8629 images. Among them, 6471 images were used for training, 548 images were used for validation, and 1610 images were used for testing. The dataset encompasses 10 categories from daily scenes-pedestrian, person, bicycle, car, van, truck, tricycle, awning tricycle, bus, and motorcycle. In this dataset, the proportion of categories is unbalanced, and most images contain small targets, making detection difficult.
The RSOD dataset is a public dataset released by Wuhan University in 2017, it consists of 976 optical remote sensing images taken from Google Earth and Tianditu, and is composed of four object classes: aircraft, oiltank, overpass, and playground, totalling 6950 targets. To increase the number of samples, the dataset was expanded by means of rotation, translation, and splicing, increasing the total to 2000 images. To avoid data leakage issues, data augmentation is performed only on the training set, and the validation and test sets remain in their original state. Then randomly split it into training, validation, and test sets at a ratio of 8:1:1, with the training set comprising 1,600 images and both the validation and test sets containing 200 images each.
The AI-TOD dataset is a specialized remote sensing image dataset focused on tiny objects, consisting of 28,036 images and 700,621 targets. These targets are divided into eight categories: bridge, ship, vehicle, storage-tank, person, swimming-pool, wind-mill, and airplane. Compared to other aerial remote sensing datasets, the average size of targets in AI-TOD is approximately 12.8 pixels, which is significantly smaller than that in other datasets, increasing the difficulty of detection. The dataset is divided into training, validation, and test sets at a ratio of 6:1:3.
Evaluation criteria
We selected mAP0.5, mAP0.5:0.95, and APs as indicators to measure the model’s accuracy in small target detection. To evaluate the model’s efficiency, we used the number of parameters and model size as indicators of its lightweight nature. Additionally, latency was chosen to assess the model’s real-time detection performance.
Precision is the ratio of the number of samples correctly predicted as positive to the number of all samples predicted as positive. The formula is as follows:
Recall is the ratio of the number of samples correctly predicted as positive to the number of samples of all true cases. The formula is as follows:
TP (true positives) represents the number of correctly identified positive instances, FP (false positives) represents the number of incorrectly identified negative instances as positive, and FN (false negatives) represents the number of incorrectly identified positive instances as negative.
mAP refers to the average AP of all defect categories, AP refers to the area of the curve below the precision recall curve, and the formula for AP and mAP is as follows, the greater the mAP is, the better the comprehensive detection performance of the model in all categories, the specific formula is as follows:
The APs metric is the average accuracy of calculating the detection results of small objects, and this metric can help us understand how well the model performs when detecting small objects. The number of parameters represents the number of the parameters used by the model, measured in millions. The number of parameters provides a direct indicator of the complexity of the model, a greater number of parameters usually means greater representation power, but can likewise lead to longer training times and the risk of overfitting. Model size usually refers to the size of the model file stored on disk and is usually quantified in megabytes (MB). Model size reflects the amount of storage space the model occupies, which is especially important in resource-constrained environments such as mobile devices or where the model needs to be deployed to embedded devices. Latency refers to the time it takes to process a frame in object detection, and is one of the metrics to measure whether a model can meet real-time detection.
Ablation atudy
To validate the effectiveness of the proposed module in aerial image detection, we conducted ablation studies for each module, using the YOLOv8s model as the baseline. The experimental results are shown in Table 2 , where ✓ indicates the addition of the module to the model, A represents adding the MFI module, B represents improving the network structure, C represents adding the PEC module, and D represents adding the ES-C2f module.
By incorporating the multilevel feature integrator (MFI) module, experiments demonstrate a notable enhancement in small object detection performance, notably reflected in a 1.6% increase in mean average precision ([email protected]) and a 0.9% increase in [email protected]:0.95. Simultaneously, the total number of model parameters is reduced by 0.8 million, and the model size decreases by 1.6 megabytes. Additionally, it reduces latency to 8.5 milliseconds, indicating that the MFI module has optimized the model’s computational efficiency and feature extraction capabilities, particularly in integrating multi-level semantic information and reducing redundant calculations.
By optimizing the network structure, removing redundant deep feature mappings, and introducing detection heads optimized for small object detection, the precision of the model is significantly enhanced, as is the model’s ability to capture low-frequency detail information. These changes resulted in an improvement of 1.8% in mAP0.5 and 1.3% in mAP0.5:0.95. By compressing the number of channels and reducing the number of network layers, the model can abstractly extract semantic information from deeper feature maps, further enhancing the recognition of small objects. The simplification of the structure not only reduced the parameter count by 7.2 M but also reduced the model size to 6.3 MB. However, an increase in latency to 12ms suggests that the addition of a specific small object detection head has led to an increase in latency.
Subsequently, by introducing the PEC module, the feature maps are finely sliced and fused along the channel dimension, enhancing the spatial integrity and richness of the features. At the same time, with the introduction of squeeze layers, we compress key information while reducing computational complexity, thus improving the efficiency of feature processing. By using the bottleneck structure for deep feature processing, the small object detection and processing capabilities of the module are enhanced, and the complexity of the model increases only slightly compared to that of the baseline model, maintaining the latency at 12.5 ms, resulting in a 1.2% improvement in the mAP0.5 and a 0.7% improvement in the mAP0.5:0.95. This result shows that even with a slight increase in complexity, the PEC module achieves a significant improvement in the accuracy of small object detection, especially in complex scenarios, where the model’s performance has been effectively improved.
Finally, by integrating the ES-C2f module, the model can combine the advantages of \(3 \times 3\) and \(5 \times 5\) convolutional kernels to capture local detail features of the target more efficiently than the traditional C2f module while integrating a wider range of contextual information. This module not only improves computational efficiency but also enhances the model’s representational capacity through internal feature channel transformation and information compression. This allows the model to more comprehensively analyze the image content and accurately capture the details of small objects. As a result, the model’s mAP0.5 and mAP0.5:0.95 increased by approximately 1.1% and 0.6%, respectively, while the number of parameters and the model size were reduced by 6.7 M and 12.7 MB compared to the baseline, and then seeing an increase to 14 ms, still ensures a reasonable latency time.
These results validate our improvement strategy, which effectively enhances the accuracy of target detection in aerial images while ensuring that the model is lightweight, demonstrating the profound significance of the research.
Compared with the baseline model, MPE-YOLO shows a significant improvement in the detection accuracy of all categories. As shown in Table 3 , the accuracy of both the pedestrian and people categories is improved by more than 8 points, which indicates that the MPE-YOLO model has a strong detail capture ability for small-scale targets. Overall, the average accuracy of the MPE-YOLO model (mAP0.5) reached 37.0%, which is nearly 6% higher than that of YOLOv8, proving the effectiveness of MPE-YOLO.
Comparative experiments
To validate the effectiveness of the model. we selected the most popular object detection algorithms to compare with MPE-YOLO, including YOLOv5, YOLOv6, YOLOv7, YOLOv8, YOLOX 41 , RT-DETR 42 , and Gold-YOLO 43 ,ASF-YOLO 44 , two of the recent research results, as shown in Table 4 .
The test results on the VisDrone2019 dataset show the differences in the performances of different object detection algorithms. First, we observed that the performances of the most classical YOLOv5s model was 26.8% on mAP0.5 and 7.0% on APs for small target detection. This result reflects the challenges of the basic YOLO model for small target detection on aerial image datasets. In comparison, YOLOv6s performed slightly worse, with mAP0.5 at 26.6% and APs at 6.7%, but despite this, the performances of the two methods were not very different. The model size and the number of parameters significantly differ, with the model size of YOLOv6s being nearly three times larger than that of YOLOv5s, and the number of parameters being more than doubled. YOLOX-s increased mAP0.5 to 29.5% and APs to 8.8%, indicating a significant improvement in the detection effect. However, this improvement comes at the cost of an increased model size (50.4 MB) and a larger number of parameters (8.9 M).
We then analyzed more advanced models - YOLOv8s and YOLOv8m. The YOLOv8s model achieves 31.3% on mAP0.5 and 8.2% APs, indicating that structural optimization has led to significant improvements. The YOLOv8m model achieves 35.4% and 9.8% on mAP0.5 and APs, respectively, which further proves that larger models may have better accuracy, especially for the more complex task of small object detection.
The RT-DETR-R18 model has a high score (35.9% vs. 10.2%) on both mAP0.5 and APs compared to the traditional architecture of the YOLO series, and it uses the DETR architecture, indicating the potential of the attention mechanism for more accurate object detection, and its model size and number of parameters are also lower than YOLOv8m.
To further validate the superiority of the MPE-YOLO model, we included two advanced models from existing literature, Gold-YOLO and X-YOLO, for comparative experiments. The experimental results show that Gold-YOLO achieved mAP0.5 and APs of 33.2 % and 9.5% respectively, with a model size of 26.3 MB and 13.4 million parameters. X-YOLO achieved mAP0.5 and APs of 34.0% and 9.6% respectively, with a model size of 22.8 MB and 11.3 million parameters. Both models showed significant improvements in performance and small object detection compared to the early YOLO series.
In the end, the MPE-YOLO model achieved the highest mAP0.5 of 37.0% and APs of 10.8%, while maintaining a model size of only 11.5 MB and 4.4 million parameters. This demonstrates that MPE-YOLO not only outperforms other current models in terms of performance but also achieves low resource consumption through its lightweight design, making it highly practical and attractive for real-world applications.
Visual analytics
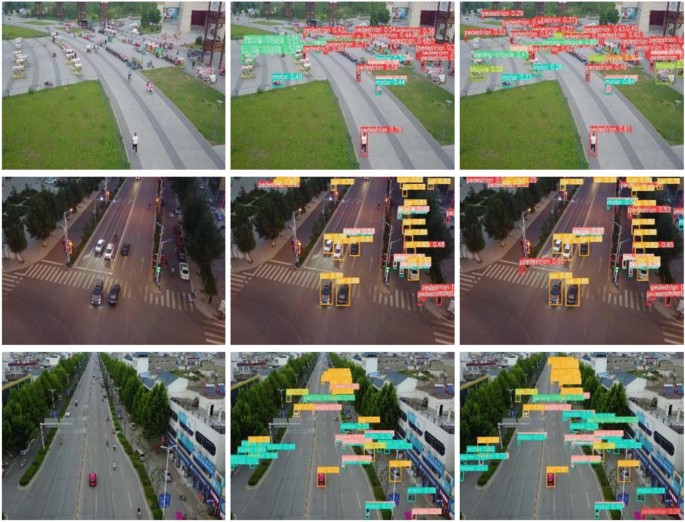
Comparison of YOLOv8(mid)and MPE-YOLO(right)on the RSOD VisDrone dataset.
By carefully selecting image samples, we applied the baseline model and the MPE-YOLO model for object detection. This allowed us to compare and analyze the detection performances of the two models. As shown in Fig. 6 , the detection confidence of the MPE-YOLO model is significantly better than that of the baseline model under multiple scenarios and challenging conditions. This is manifested in the fact that the target bounding boxes it identifies have higher confidence scores, and these scores are more consistent with the actual target. More importantly, MPE-YOLO also shows significant improvements in reducing false positives and false negatives, accurately identifying and identifying most targets, while minimizing misidentification of non-target areas. Moreover, even under suboptimal shading or lighting conditions, MPE-YOLO achieved a low missed detection rate. These comparison results highlight the effectiveness of the enhanced feature extraction network in MPE-YOLO in dealing with overlapping, size changes and complex backgrounds between targets, indicating that it has more robust feature learning and more accurate target prediction capabilities.
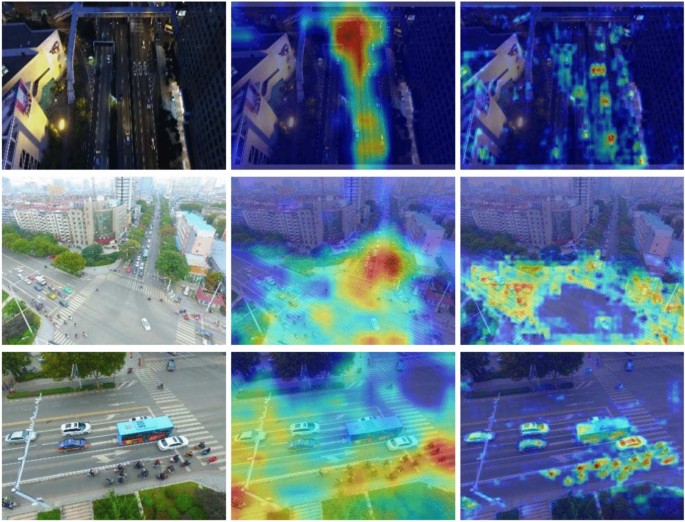
In Fig. 7 , the improved MPE-YOLO model demonstrates its superior feature extraction and targeting capabilities. This is evident by the more concentrated and reinforced high-response regions it reflects. This feature is presented as a brighter area on the heat map, closely following the actual position and contour of the target, demonstrating that the MPE-YOLO model can effectively focus on important signals. In addition, compared with the baseline model, the heat map generated by the improved model shows fewer scattered hot spots around the target, which reduces the possibility of false detection and false alarms, demonstrating the precision and robustness of MPE-YOLO in small target detection tasks. First, the heat map of the night scene in the first row reveals the recognition ability of MPE-YOLO under low-light conditions, in which areas with strong brightness are accurately mapped to the target location, indicating that the model still has efficient feature capture capabilities at low lighting levels. Then, in the second row, when faced with a complex background scene, the heat map generated by MPE-YOLO maintained the ability to accurately identify the target without being affected by the complex environment. The model’s clear positioning of the target verifies its effectiveness in distinguishing the target from the cluttered background in the actual environment. Finally, in the case of dense small targets in the third row, the MPE-YOLO heat map shows excellent discrimination, even when the targets are very close to each other. The highlights of the heat map correspond densely and distinctly to the contours of each small target, showing the model’s ability to accurately locate multiple targets.
These visual evidences are consistent with the increase in mAP0.5 and mAP0.5:0.95 in the experiment, which provides intuitive and strong support for our research.
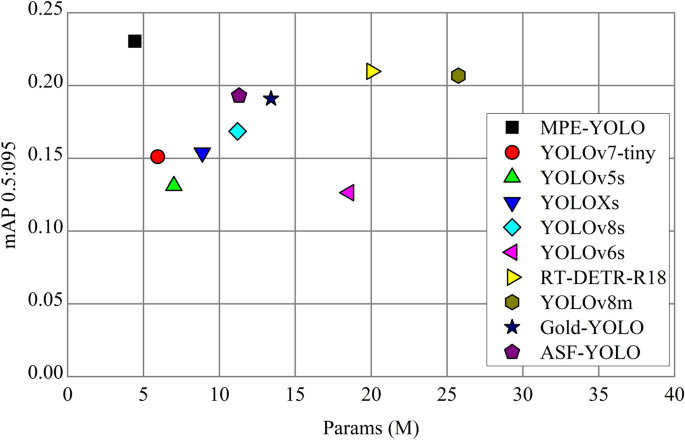
Relationships between the AP50:95 and model parameter count for different models.
Figure 8 shows the relationship between mAP0.5:0.95 and the parameters of each model, where the x-axis represents the parameters of the model and the y-axis represents the detection performance index. As can be seen from the figure, MPE-YOLO achieves an improvement in detection accuracy while maintaining a low weight. Compared to all the comparison models, our model is best suited for drone vehicle inspection tasks.
Generalization study
Through comprehensive comparative tests on two different remote sensing image datasets RSOD and AI-TOD in Table 5 , our MPE-YOLO model demonstrates its superior generalizability. According to these tests, the MPE-YOLO model showed high accuracy in the two key performance indicators of mAP0.5 and mAP0.5:0.95 compared with several existing advanced object detection models, especially on the AI-TOD dataset, for which the average target size was only 12.8 pixels.
The experimental results reveal the strong detection ability of MPE-YOLO, which maintains high accuracy even in small target detection scenarios, confirming its practicability and effectiveness in the field of remote sensing image analysis. These conclusions support the use of the MPE-YOLO model as a remote sensing target detection algorithm with strong adaptability and generalizability, and indicate its broad potential for future practical applications.
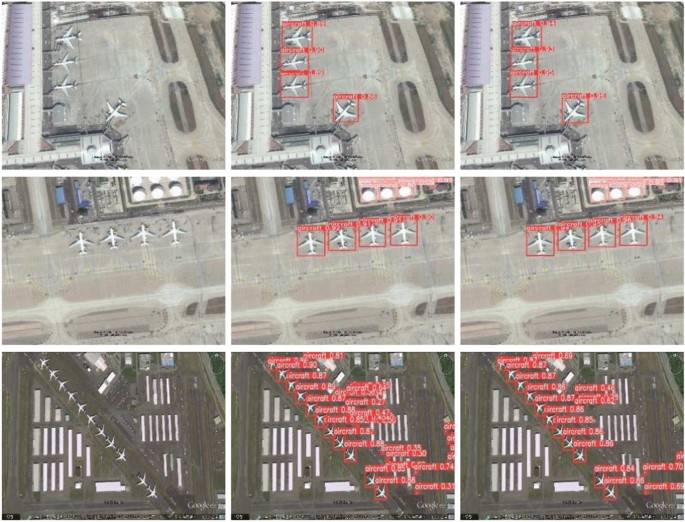
Comparison of YOLOv8(mid)and MPE-YOLO(right)on the RSOD dataset.
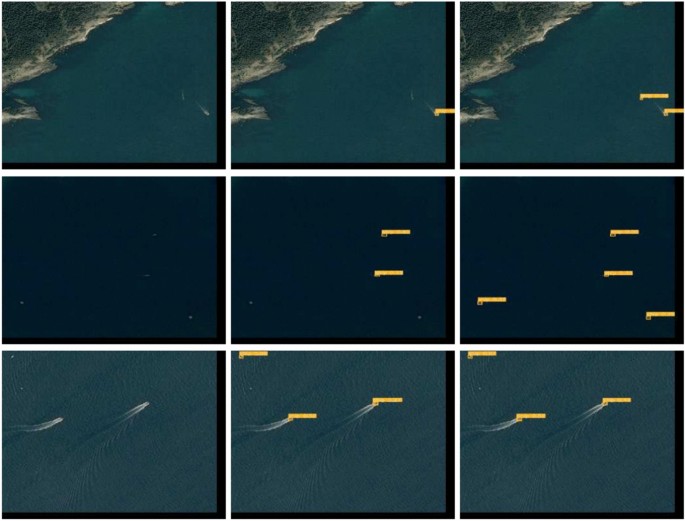
Comparison of YOLOv8(mid)and MPE-YOLO(right)on the AI-TOD dataset.
To more clearly demonstrate the strength of our algorithm in detecting small-sized targets, we selected several representative photographs from both the RSOD and AI-TOD datasets. Figures 9 and 10 show that YOLOv8 has a great number of missed detections on smaller targets than MPE-YOLO, which has significantly fewer missed cases. Additionally, MPE-YOLO shows a general improvement in detection precision. These comparative visuals underscore that MPE-YOLO is a more suitable model for practical detection in aerial imagery applications.
Upon examining these sets of illustrations, it becomes evident that our MPE-YOLO outperforms YOLOv8, especially in scenarios with smaller and easily overlooked targets, reinforcing its efficacy and reliability for deployment in aerial target detection tasks.
Conclusions
In this study, we propose the MPE-YOLO model, which effectively improves the accuracy of small and medium-sized object detection in aerial images, and optimizes the object detection performance in complex environments. First, the MFI module is proposed to effectively improve the efficiency of feature fusion, reduce information loss, and qualitatively improve the detection characteristics of small targets. The PEC module enhances the ability of the network to capture the detailed features of the target, which has a significant effect on the object detection in complex backgrounds. The ES-C2f module further strengthens the feature representation ability of small targets by optimizing the sensing range. The model has been tested on multiple aerial image datasets to confirm its excellent performance, especially in terms of real-time processing power and detection accuracy. Future work will focus on improving the generalization ability of the model and optimizing the operational efficiency, with a view to deploying it in a wider range of practical applications.
Data availability
All the images and experimental test images in this paper were from the open source VisDrone dataset, RSOD dataset and AI-TOD dataset. These datasets analyzed during the current research period can be found at the following website.Visdrone: (https://github.com/VisDrone/VisDrone-Dataset), RSOD: (https://github.com/RSIA-LIESMARS-WHU/RSOD-Dataset-) and AI-TOD :( https://github.com/jwwangchn/AI-TOD).
Liu, H. et al. Improved gbs-yolov5 algorithm based on yolov5 applied to uav intelligent traffic. Sci. Rep. 13 , 9577 (2023).
Article ADS CAS PubMed PubMed Central Google Scholar
Bravo, R. Z. B., Leiras, A. & CyrinoOliveira, F. L. The use of uav s in humanitarian relief. An application of pomdp-based methodology for finding victims. Prod. Oper. Manag. 28 , 421–440 (2019).
Article Google Scholar
Suthaharan, S. & Suthaharan, S. Support vector machine. Machine Learning Models and Algorithms for Big Data Classification: Thinking with Examples for Effective Learning 207–235 (2016).
Biau, G. & Scornet, E. A random forest guided tour. TEST 25 , 197–227 (2016).
Article MathSciNet Google Scholar
Dalal, N. & Triggs, B. Histograms of oriented gradients for human detection. In 2005 IEEE Computer Society Conference on Computer Vision and Pattern Recognition (CVPR’05) Vol. 1, 886–893 (IEEE, 2005).
Girshick, R., Donahue, J., Darrell, T. & Malik, J. Rich feature hierarchies for accurate object detection and semantic segmentation. In Proceedings of the IEEE Conference on Computer Vision and Pattern Recognition 580–587 (2014).
Girshick, R. Fast r-cnn. In Proceedings of the IEEE International Conference on Computer Vision 1440–1448 (2015).
Ren, S., He, K., Girshick, R. & Sun, J. Faster r-cnn: Towards real-time object detection with region proposal networks. Adv. Neural Inform. Process. Syst. 28 (2015).
Liu, W. et al. Ssd: Single shot multibox detector. In Computer Vision-ECCV 2016: 14th European Conference, Amsterdam, The Netherlands, October 11–14, 2016, Proceedings, Part I 14 21–37 (Springer, 2016).
Redmon, J., Divvala, S., Girshick, R. & Farhadi, A. You only look once: Unified, real-time object detection. In Proceedings of the IEEE Conference on Computer Vision and Pattern Recognition 779–788 (2016).
Redmon, J. & Farhadi, A. Yolo9000: Better, faster, stronger. In Proceedings of the IEEE Conference on Computer Vision and Pattern Recognition 6517–6525 (2017).
Redmon, J. & Farhadi, A. Yolov3: An incremental improvement. arXiv preprint arXiv:1804.02767 (2018).
Bochkovskiy, A., Wang, C.-Y. & Liao, H.-Y. M. Yolov4: Optimal speed and accuracy of object detection. arXiv preprint arXiv:2004.10934 (2020).
Glenn, J. Ultralytics yolov5 (2022).
Li, C. et al. Yolov6: A single-stage object detection framework for industrial applications. arXiv preprint arXiv:2209.02976 (2022).
Wang, C.-Y., Bochkovskiy, A. & Liao, H.-Y. M. Yolov7: Trainable bag-of-freebies sets new state-of-the-art for real-time object detectors. In Proceedings of the IEEE/CVF Conference on Computer Vision and Pattern Recognition 7464–7475 (2023).
Glenn, J. Ultralytics yolov8 (2023).
Cheng, G., Si, Y., Hong, H., Yao, X. & Guo, L. Cross-scale feature fusion for object detection in optical remote sensing images. IEEE Geosci. Remote Sens. Lett. 18 , 431–435 (2020).
Article ADS Google Scholar
Guo, J. et al. A new detection algorithm for alien intrusion on highway. Sci. Rep. 13 , 10667 (2023).
Sahin, O. & Ozer, S. Yolodrone: Improved yolo architecture for object detection in drone images. In 2021 44th International Conference on Telecommunications and Signal Processing (TSP) , 361–365 (IEEE, 2021).
Chen, Y., Zheng, W., Zhao, Y., Song, T. H. & Shin, H. Dw-yolo: An efficient object detector for drones and self-driving vehicles. Arab. J. Sci. Eng. 48 , 1427–1436 (2023).
Zhu, X., Lyu, S., Wang, X. & Zhao, Q. Tph-yolov5: Improved yolov5 based on transformer prediction head for object detection on drone-captured scenarios. In Proceedings of the IEEE/CVF International Conference on Computer Vision 2778–2788 (2021).
Yang, Y. Drone-view object detection based on the improved yolov5. In 2022 IEEE International Conference on Electrical Engineering, Big Data and Algorithms (EEBDA) 612–617 (IEEE, 2022).
Lin, Y., Li, J., Shen, S., Wang, H. & Zhou, H. In GDRS-YOLO: More Efficient Multiscale Features Fusion Object Detector for Remote Sensing Images 21 , 1–5 (2024).
Jin, R., Jia, Z., Yin, X., Niu, Y. & Qi., Y. In Domain Feature Decomposition for Efficient Object Detection in Aerial Images Vol. 16, 1626 (2024).
Bai, Z., Liu, Z., Li, G., Ye, L. & Wang, Y. Circular Complement Network for RGB-D Salient Object Detection Vol. 451, 95–106 (Elsevier, 2021).
Google Scholar
Pacal, I. et al. In An efficient real-time colonic polyp detection with YOLO algorithms trained by using negative samples and large datasets 141 , 105031 (2022).
Xu, J., Ren, H., Cai, S. & Zhang, X. An Improved faster R-CNN Algorithm for Assisted Detection of Lung Nodules Vol. 153, 106470 (Elsevier, 2023).
Chen, X., Zheng, H., Tang, H. & Li, F. Multi-Scale Perceptual YOLO for Automatic Detection of Clue Cells and Trichomonas in Fluorescence Microscopic Images 108500 (Elsevier, 2024).
Zhu, H. et al. Development of a PWM Precision Spraying Controller for Unmanned Aerial Vehicles Vol. 7, 276–283 (Elsevier, 2010).
Wang, M., Zang, X.-Z., Fan, J.-Z. & Zhao, J. Biological Jumping Mechanism Analysis and Modeling for Frog Robot Vol. 5, 181–188 (Elsevier, 2008).
Nishiyama, T., Kumagai, A., Kamiya, K. & Takahashi, K. Silu: Strategy involving large-scale unlabeled logs for improving malware detector. In 2020 IEEE Symposium on Computers and Communications (ISCC) 1–7 (IEEE, 2020).
He, K., Zhang, X., Ren, S. & Sun, J. Spatial pyramid pooling in deep convolutional networks for visual recognition. IEEE Trans. Pattern Anal. Mach. Intell. 37 , 1904–1916 (2015).
Article PubMed Google Scholar
Lin, T.-Y. et al. Feature pyramid networks for object detection. In Proceedings of the IEEE Conference on Computer Vision and Pattern Recognition 2117–2125 (2017).
Liu, S., Qi, L., Qin, H., Shi, J. & Jia, J. Path aggregation network for instance segmentation. In Proceedings of the IEEE Conference on Computer Vision and Pattern Recognition 8759–8768 (2018).
Gao, S.-H. et al. Res2net: A new multi-scale backbone architecture. IEEE Trans. Pattern Anal. Mach. Intell. 43 , 652–662 (2019).
Luo, W., Li, Y., Urtasun, R. & Zemel, R. Understanding the effective receptive field in deep convolutional neural networks. Adv. Neural Inform. Process. Syst. 29 (2016).
Du, D. et al. Visdrone-det2019: The vision meets drone object detection in image challenge results. In Proceedings of the IEEE/CVF international conference on computer vision workshops (2019).
Long, Y., Gong, Y., Xiao, Z. & Liu, Q. Accurate object localization in remote sensing images based on convolutional neural networks. IEEE Trans. Geosci. Remote Sens. 55 , 2486–2498 (2017).
Wang, J., Yang, W., Guo, H., Zhang, R. & Xia, G.-S. Tiny object detection in aerial images. In 2020 25th International Conference on Pattern Recognition (ICPR) 3791–3798 (IEEE, 2021).
Ge, Z., Liu, S., Wang, F., Li, Z. & Sun, J. Yolox: Exceeding yolo series in 2021. arXiv preprint arXiv:2107.08430 (2021).
Lv, W. et al. Detrs beat yolos on real-time object detection. arXiv preprint arXiv:2304.08069 (2023).
Wang, C. et al. Gold-yolo: Efficient object detector via gather-and-distribute mechanism. Adv. Neural Inform. Process. Syst. 36 (2024).
Kang, M., Ting, C.-M., Ting, F. & Phan, R. Asf-yolo: A novel yolo model with attentional scale sequence fusion for cell instance segmentation. Image Vis. Comput. 147 , 105057 (2024).
Download references
Acknowledgements
This work was supported by a Grant from the National Natural Science Foundation of China (No.62105093)
Author information
Authors and affiliations.
College of Information Science and Engineering, Hebei University of Science and Technology, Shijiazhuang, 050018, China
Jia Su, Yichang Qin, Ze Jia & Ben Liang
You can also search for this author in PubMed Google Scholar
Contributions
J.S. conceived the experiments, J.S. and Y.Q. conducted the experiments, Z.J. and B.L. analysed the results. Y.Q. wrote the main manuscript text. All authors reviewed the manuscript.
Corresponding author
Correspondence to Yichang Qin .
Additional information
Publisher's note.
Springer Nature remains neutral with regard to jurisdictional claims in published maps and institutional affiliations.
Rights and permissions
Open Access This article is licensed under a Creative Commons Attribution-NonCommercial-NoDerivatives 4.0 International License, which permits any non-commercial use, sharing, distribution and reproduction in any medium or format, as long as you give appropriate credit to the original author(s) and the source, provide a link to the Creative Commons licence, and indicate if you modified the licensed material. You do not have permission under this licence to share adapted material derived from this article or parts of it. The images or other third party material in this article are included in the article’s Creative Commons licence, unless indicated otherwise in a credit line to the material. If material is not included in the article’s Creative Commons licence and your intended use is not permitted by statutory regulation or exceeds the permitted use, you will need to obtain permission directly from the copyright holder. To view a copy of this licence, visit http://creativecommons.org/licenses/by-nc-nd/4.0/ .
Reprints and permissions
About this article
Cite this article.
Su, J., Qin, Y., Jia, Z. et al. MPE-YOLO: enhanced small target detection in aerial imaging. Sci Rep 14 , 17799 (2024). https://doi.org/10.1038/s41598-024-68934-2
Download citation
Received : 29 February 2024
Accepted : 30 July 2024
Published : 01 August 2024
DOI : https://doi.org/10.1038/s41598-024-68934-2
Share this article
Anyone you share the following link with will be able to read this content:
Sorry, a shareable link is not currently available for this article.
Provided by the Springer Nature SharedIt content-sharing initiative
- Object detection
- Aerial image
- Small target
- Model lightweight
By submitting a comment you agree to abide by our Terms and Community Guidelines . If you find something abusive or that does not comply with our terms or guidelines please flag it as inappropriate.
Quick links
- Explore articles by subject
- Guide to authors
- Editorial policies
Sign up for the Nature Briefing newsletter — what matters in science, free to your inbox daily.

IEEE Account
- Change Username/Password
- Update Address
Purchase Details
- Payment Options
- Order History
- View Purchased Documents
Profile Information
- Communications Preferences
- Profession and Education
- Technical Interests
- US & Canada: +1 800 678 4333
- Worldwide: +1 732 981 0060
- Contact & Support
- About IEEE Xplore
- Accessibility
- Terms of Use
- Nondiscrimination Policy
- Privacy & Opting Out of Cookies
A not-for-profit organization, IEEE is the world's largest technical professional organization dedicated to advancing technology for the benefit of humanity. © Copyright 2024 IEEE - All rights reserved. Use of this web site signifies your agreement to the terms and conditions.
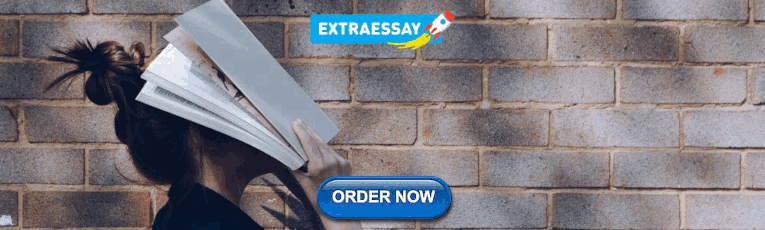
IMAGES
COMMENTS
Network threats and hazards are evolving at a high-speed rate in recent years. Many mechanisms (such as firewalls, anti-virus, anti-malware, and spam filters) are being used as security tools to protect networks. An intrusion detection system (IDS) is also an effective and powerful network security system to detect unauthorized and abnormal network traffic flow. This article presents a review ...
In order to examine malicious activity that occurs in a network or a system, intrusion detection system is used. Intrusion Detection is software or a device that scans a system or a network for a distrustful activity. Due to the growing connectivity between computers, intrusion detection becomes vital to perform network security. Various machine learning techniques and statistical ...
Due to the widespread use of the internet, computer networks are vulnerable to cyber-attacks, prompting various researchers to suggest intrusion detection systems (IDSs). Detecting intrusions is one of the important research topics in network security. As a precaution to guarantee the network's security, it aids in the detection of unwanted usage and assaults. This study summarizes significant ...
This paper proposes the use of deep learning architectures to develop an adaptive and resilient network intrusion detection system (IDS) to detect and classify network attacks. The emphasis is how deep learning or deep neural networks (DNNs) can facilitate flexible IDS with learning capability to detect recognized and new or zero-day network ...
Table 2 presents a comparison of unsupervised ML-based intrusion detection systems (IDS) that do not require labeled data. The table lists the architecture used in the study, the dataset employed, the corresponding research article, the attacks addressed, feature reduction or dimensionality reduction techniques used, and the performance metrics achieved.
Hence, the main objective of this paper is to present a survey for supervised learning algorithms and intrusion detection systems. We review necessary concepts that are related to IDS, The concept of IDS is first presented, where we discuss its definition, types, and importance. Then, we review popular supervised learning algorithms and popular ...
It employs tools like firewall, antivirus software, and intrusion detection system (IDS) to ensure the security of the network and all its associated assets within a cyberspace. 1 Among these, network-based intrusion detection system (NIDS) is the attack detection mechanism that provides the desired security by constantly monitoring the network ...
unauthorized and malicious activities. Intrusion is the breach o f. security policy with malicious i ntent. Therefore, intrusion. detection system monitors traffic flowing on a network through ...
IEEE: (("Hybrid" OR "Integrated" OR "Cascaded") AND ("Intrusion detection System" OR "IDS") AND (Artificial Intelligence OR Machine Learning)) ... 17 were short papers, 1 was non-English paper, and 1 was incomplete paper. In this research 111 papers were selected for the review as shown in Table 5. 5. Selected papers per ...
Existing research often focuses on individual attacks, utilizing various mitigation strategies, including machine learning and deep learning for detection. This paper proposes an Intrusion Detection System (IDS) using the ROUT-4-2023 dataset, which encompasses Black Hole, Flooding, DODAG Version Number, and Decreased Rank attacks.
A. CAN Intrusion Detection The research on IDS development for IoV and connected vehicles has been considered critical in recent years. Many research works have a focus on detecting attacks on CAN-based intra-vehicle networks. Alshammari et al. [12] proposed an intrusion classification model to identify CAN intrusions on in-vehicle networks ...
classification of the attacks in case of intrusion detection within the system. However, due to the large number of algorithms available, the selection of the right method is a challenging task. To resolve this issue, this paper analyses some of the current state of the art intrusion detection methods and discusses their pros and cons.
The use of deep learning models for the network intrusion detection task has been an active area of research in cybersecurity. Although several excellent surveys cover the growing body of research on this topic, the literature lacks an objective comparison of the different deep learning models within a controlled environment, especially on recent intrusion detection datasets.
A thorough evaluation was carried out by [] on IDS with a focus on the signature- and anomaly-based approaches; however, a systematic literature review approach was not followed, and there was also no in-depth analysis or comparisons of the methods, datasets, attacks, or performances of the techniques.In the same vein, [] followed a systematic approach to reviewing hybrid-based IDS; however ...
The IDS can be categorized into three categories on the installation basis in the system- Host-based IDS (HIDS), Network-based IDS (NIDS), and Hybrid IDS. HIDS are deployed on a single host. In ...
An Intrusion Detection and Prevention System (IDS & IPS) have a vital influence in finding and restricting various intrusion attempts and gives a positive secure framework. The expanding sensors in an IoT framework accompany constraints, for example, interoperability, adaptability, and capacity.
An intrusion detection system is a security system that monitors and analyzes network traffic and computer systems to detect potential attacks originating from either outside or inside the ...
An Intrusion Detection Scheme in TCP/IP Networks Based on Flow-Net and Fingerprint ACM SE '17: Proceedings of the SouthEast Conference Based on our previous work for a novel logging methodology, called flow-net, we propose an Intrusion Detection System (IDS) using Flow-Net Based Fingerprint (IDS-FF) in this paper.
DOI: 10.1016/j.rineng.2024.102659 Corpus ID: 271684117; An RFE/Ridge-ML/DL based anomaly intrusion detection approach for securing IoMT system @article{Lazrek2024AnRB, title={An RFE/Ridge-ML/DL based anomaly intrusion detection approach for securing IoMT system}, author={Ghita Lazrek and Kaouthar Chetioui and Younes Balboul and Said Mazer and Moulhime El bekkali}, journal={Results in ...
NIST ( Bace and Mell, 2001) describes the intrusion as an attempt to compromise CIA, or to bypass the security mechanisms of a computer or network, intrusion detection is the process of monitoring the events occurring in a computer system or network, and analyzing them for signs of intrusions. Especially, wireless networks have recently been ...
Computer networks are exposed to cyber related attacks due to the common usage of internet, as the result of such, several intrusion detection systems (IDSs) were proposed by several researchers. Among key research issues in securing network is detecting intrusions. It helps to recognize unauthorized usage and attacks as a measure to ensure the secure the network's security. Various ...
2.1 Problem Definition of Intrusion Detection Systems. Let us assume that the input data D are divided into two subsets, namely, training data \(D^t\) and test data \(D^v\), and that \(\mathcal {A}()\) is the accuracy function. An intrusion detection problem can be regarded as an extended classification problem [], defined as follows:Phase 1: Suppose input data D can be divided into n classes.
Effectively detecting intrusions in the computer networks still remains problematic. This is because cyber attackers are changing packet contents to disguise the intrusion detection system (IDS) recently. Besides, everyday a lot of new devices are added to the computer networks. These new devices are also raising security issues in the computer networks. To effectively manage the computer ...
Therefore, how to improve the security and privacy challenges of IoT remains an important problem in the computer security field. This paper proposes a machine learning-based intrusion detection system (ML-IDS) for detecting IoT network attacks. The primary objective of this research focuses on applying ML-supervised algorithm-based IDS for IoT.
The rest of this paper includes the following content: Section 2 briefly introduces the recent research results on aerial image detection and the main idea of YOLOv8. Section 3 introduces the ...
This paper proposes two types of Network-based Intrusion Detection Systems (NIDSs): promiscuous and network system modes. This Intrusion Detection System (IDS) reacts just to the mark-based identified assaults, which is a significant disadvantage of this strategy, and yet, the user is expected to understand the issue.
The main aim for this review paper is that to provide information about the need of intrusion detection systems in day to day life. Published in: 2020 IEEE International Conference for Innovation in Technology (INOCON) Date of Conference: 06-08 November 2020. Date Added to IEEE Xplore: 01 January 2021. ISBN Information: