Rewrite your text with precision and ease
Transform your writing with DeepL’s AI-powered paraphraser and grammar checker. Offering unparalleled accuracy and versatility in rewriting, experience the future of paraphrasing today.
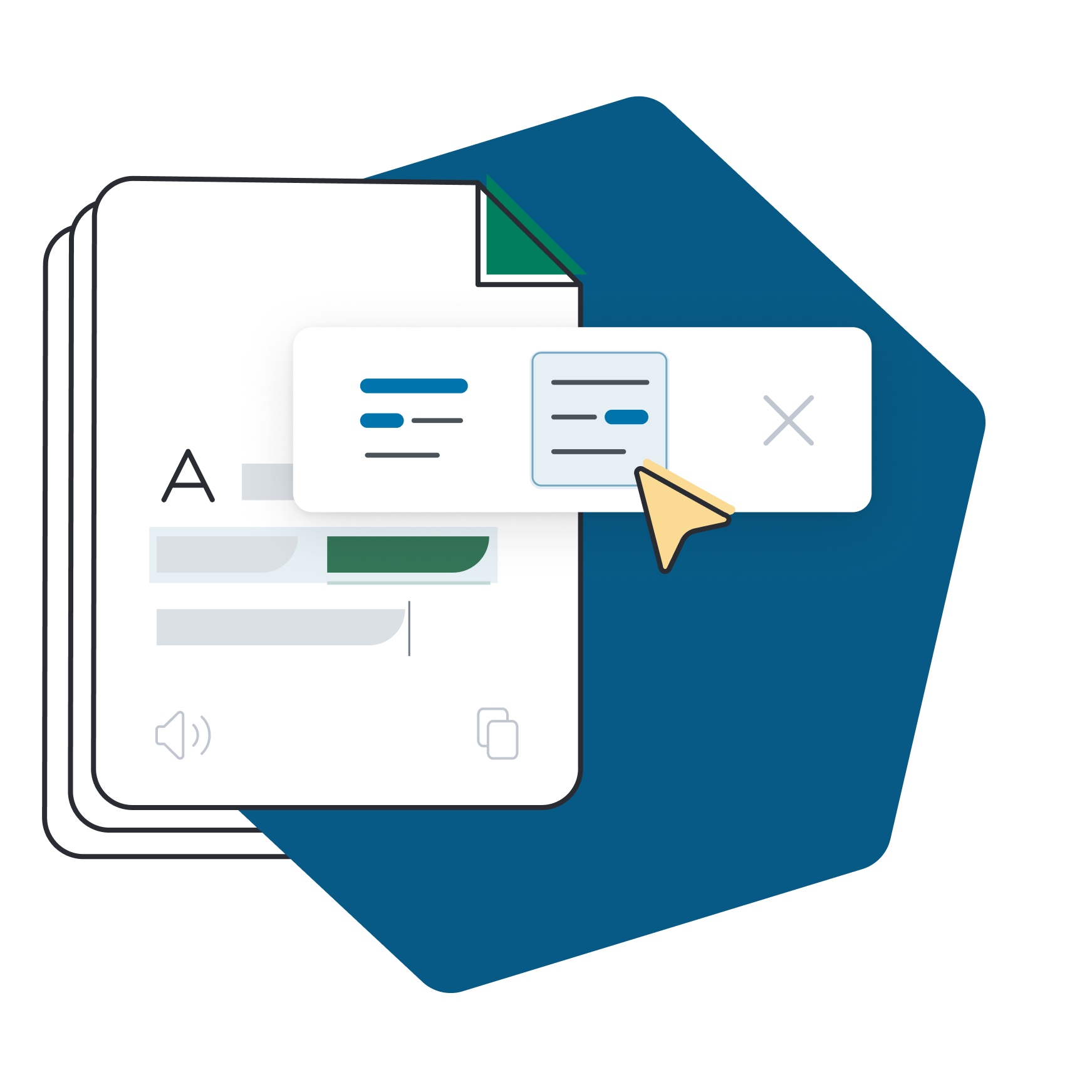
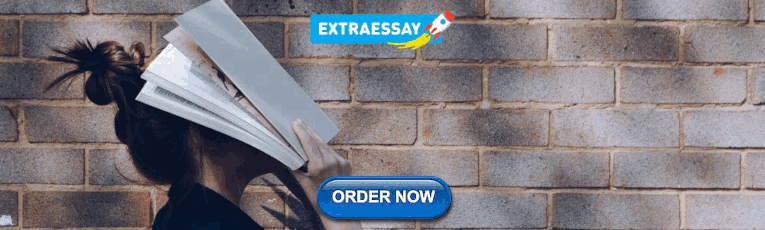
Revolutionize your writing with our advanced AI paraphraser
Embrace the power of DeepL’s cutting-edge AI to transform your writing. Our paraphrasing tool goes beyond simple synonym replacement, using a sophisticated language model to capture and convey the nuances of your text.
With our paraphraser, you'll not only retain the essence of your original content, but also enhance its clarity.
We currently offer text rewriting only in English and German. In the future, we'll release new languages gradually to ensure we deliver texts that are not just rewritten, but elevated.
Why use DeepL’s paraphrasing tool?
With our AI writing assistant, you can:
Improve your writing
Enhance the clarity, tone, and grammar of your text, especially in professional contexts.
Avoid errors
Forgo errors and present your ideas concisely for more polished writing.
Speed up writing
Expedite the writing process with suggestions for more formal, refined language.
Express yourself clearly
Perfect sentences and express yourself clearly—particularly for non-native English and German speakers.
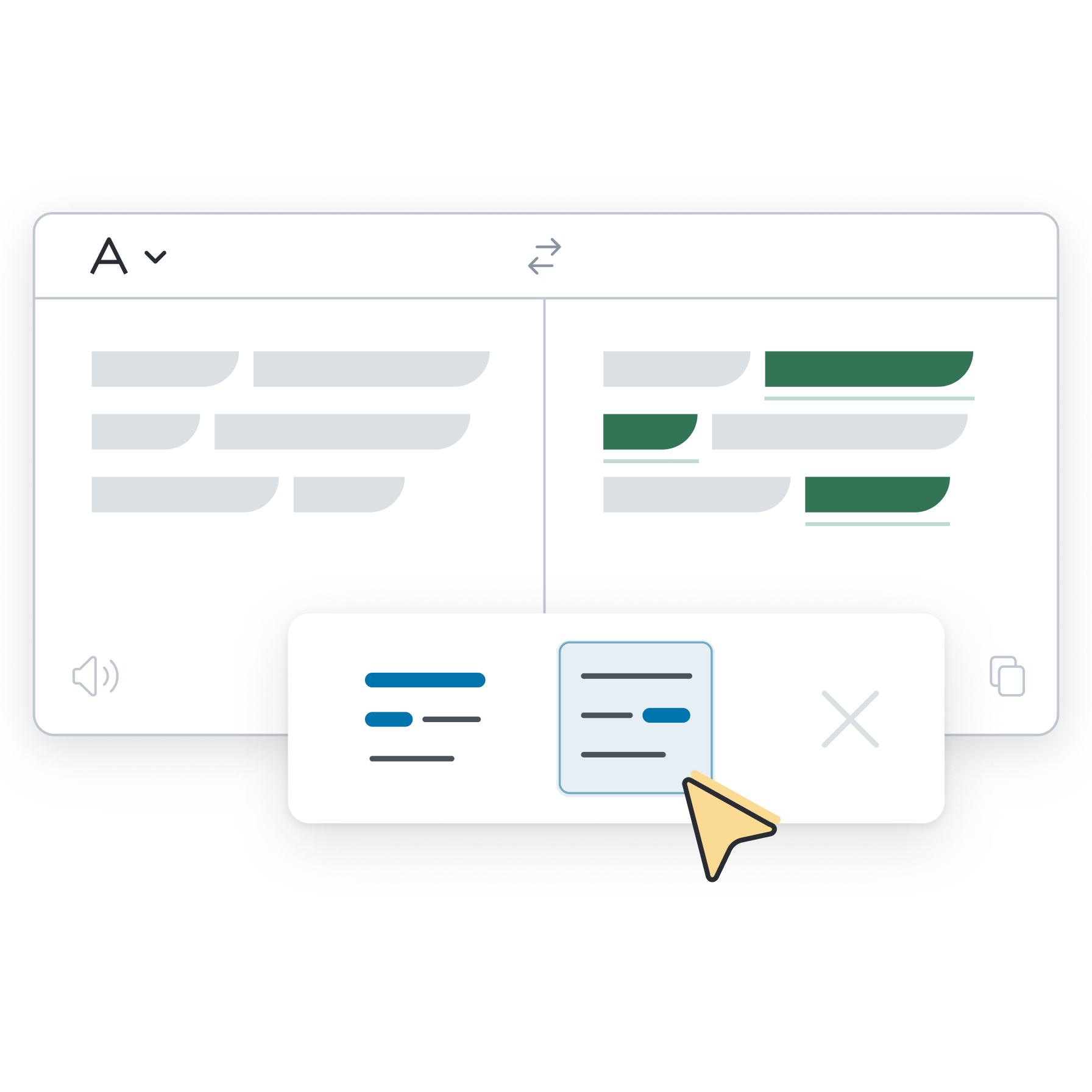
Here's what you can do with our paraphraser
For business use:
- Great for short-form writing, like emails or messages, and long-form content, like PowerPoint presentations, essays, or scientific papers.
For personal use:
- Improve your writing and vocabulary, generate ideas, and express your thoughts more clearly.
DeepL’s paraphraser is also helpful for language learners. For example, you can memorize suggested vocabulary and phrases.
Try our paraphrasing tool to improve your writing instantly
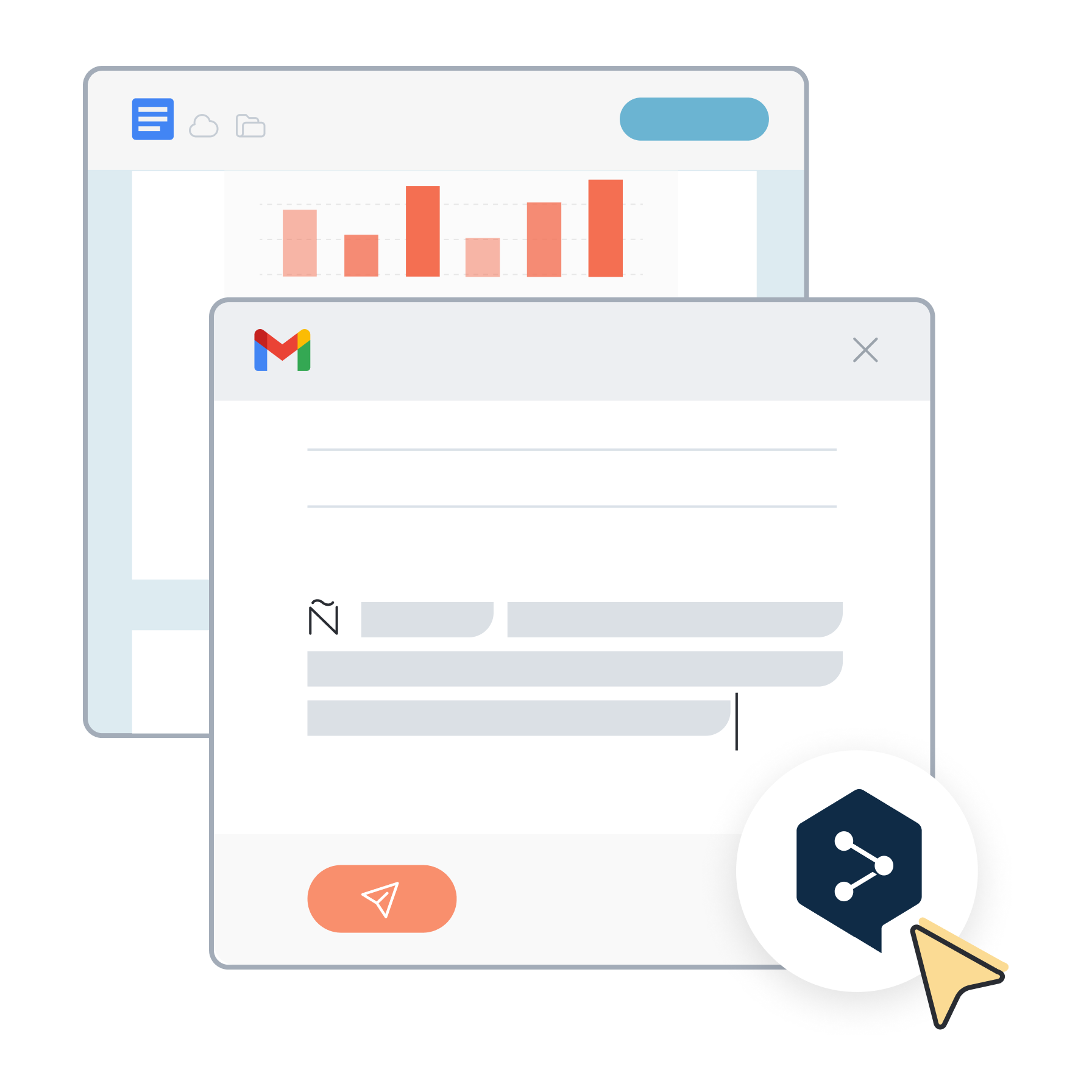
Key features of our AI paraphrasing tool
- Incorporated into translator: Translate your text into English or German, and click "Improve translation" to explore alternate versions of your translation. No more copy/paste between tools.
- Easy-to-see changes: When you insert the text to be rewritten, activate "Show changes" to see suggested edits.
- AI-powered suggestions: By deactivating "Show changes", you can click on any word to see suggestions and refine your writing.
- Grammar and spell checker: Our paraphrasing tool is all-in-one, helping you correct grammar, spelling, and punctuation errors.
- Helpful integrations: Access our paraphrasing tool in Gmail, Google Slides, or Google Docs via our browser extension or in Microsoft Word via add-ins .
"The fastest, easiest, and most efficient translation tool I've ever used."
" You can easily modify the translation to use the vocabulary you want and make it sound natural. "
Still have questions about DeepL’s paraphrasing tool?
1. what makes our paraphrasing tool unique.
DeepL uses advanced AI to provide high-quality, context-aware paraphrasing in English and German. Our tool intelligently restructures and rephrases text, preserving the original meaning and enhancing your writing.
2. How do you use DeepL’s paraphrasing tool?
To accomplish writing tasks, you can:
- Paste your existing text into the tool
- Compose directly in the tool
- Use DeepL Translator before refining your writing with our paraphraser
3. Can the tool paraphrase complex academic texts?
Absolutely. DeepL's paraphraser is designed to handle complex sentence structures, making it useful for academic writing.
4. How does DeepL's paraphraser support language learners?
By making suggestions, the tool enables you to learn new phrases or words to incorporate into your vocabulary.
5. Is the paraphrasing tool free to use?
For now, the tool is completely free to use.
Explore the capabilities of our tool
Paraphrasing Tool
Paraphrasing Tool in partnership with QuillBot. Paraphrase everywhere with the free Chrome Extension .
Try our other writing services

Avoid plagiarism in your paraphrased text
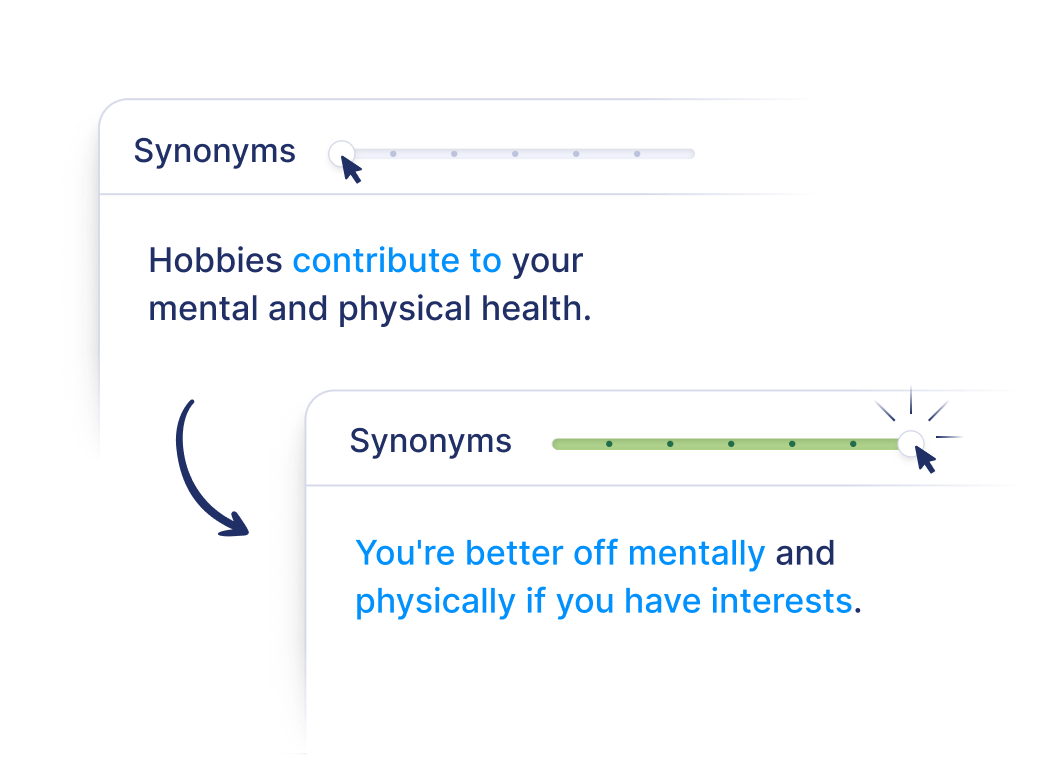
What is a paraphrasing tool?
This AI-powered paraphrasing tool lets you rewrite text in your own words. Use it to paraphrase articles, essays, and other pieces of text. You can also use it to rephrase sentences and find synonyms for individual words. And the best part? It’s all 100% free!
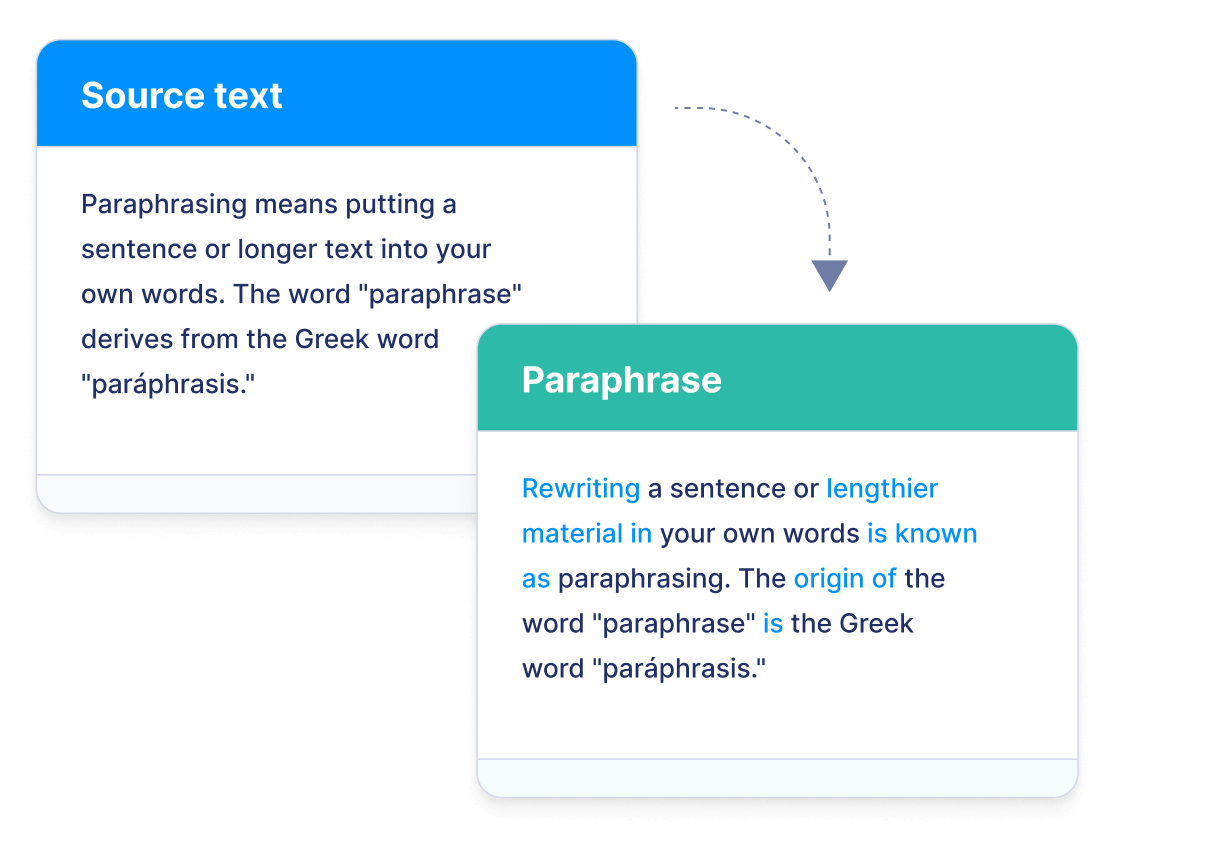
What is paraphrasing?
Paraphrasing involves expressing someone else’s ideas or thoughts in your own words while maintaining the original meaning. Paraphrasing tools can help you quickly reword text by replacing certain words with synonyms or restructuring sentences. They can also make your text more concise, clear, and suitable for a specific audience. Paraphrasing is an essential skill in academic writing and professional communication.
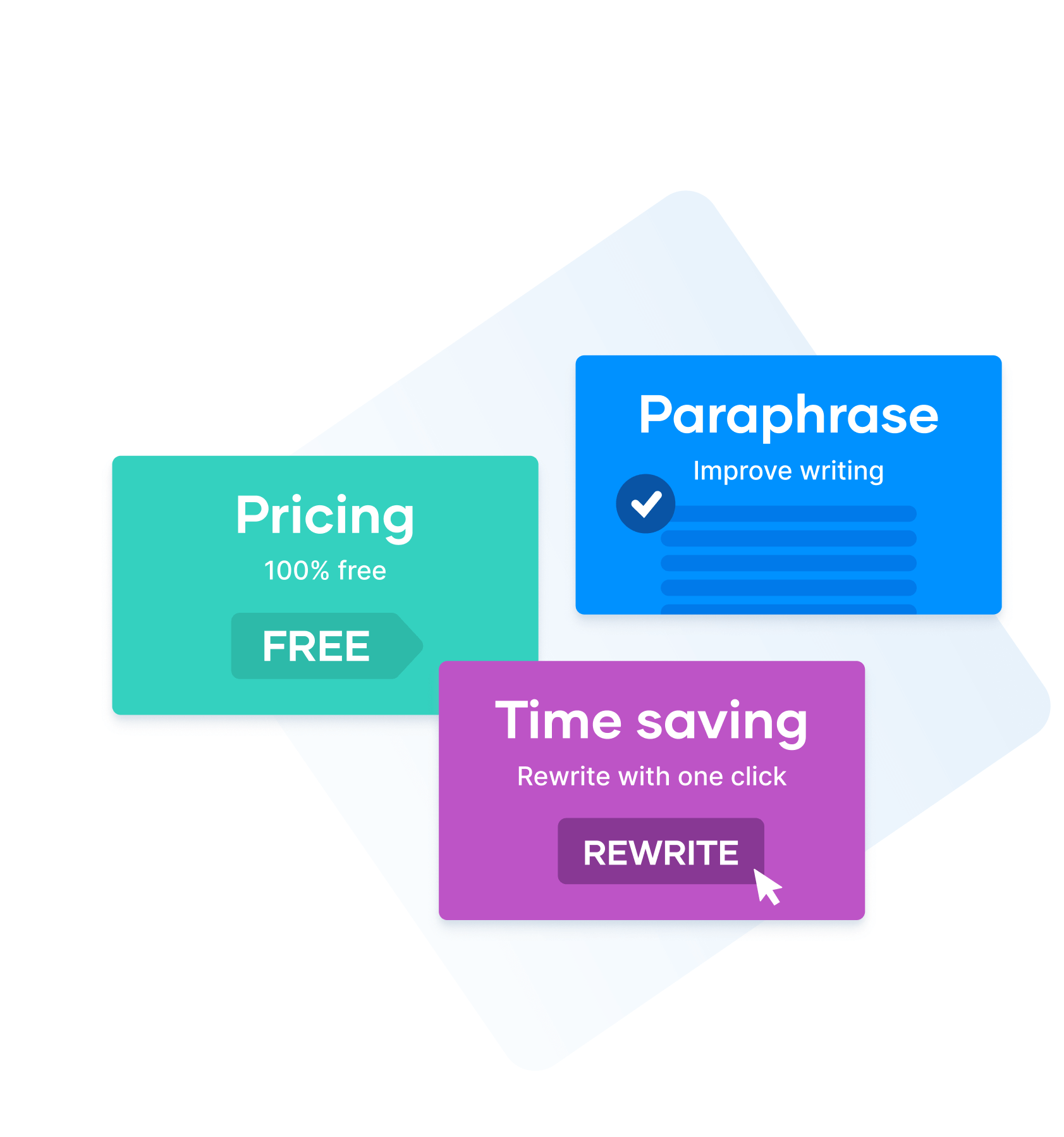
Why use this paraphrasing tool?
- Save time: Gone are the days when you had to reword sentences yourself; now you can rewrite an individual sentence or a complete text with one click.
- Improve your writing: Your writing will always be clear and easy to understand. Automatically ensure consistent language throughout.
- Preserve original meaning: Paraphrase without fear of losing the point of your text.
- No annoying ads: We care about the user experience, so we don’t run any ads.
- Accurate: Reliable and grammatically correct paraphrasing.
- No sign-up required: We don’t need your data for you to use our paraphrasing tool.
- Super simple to use: A simple interface even your grandma could use.
- It’s 100% free: No hidden costs, just unlimited use of a free paraphrasing tool.
People are in love with our paraphrasing tool
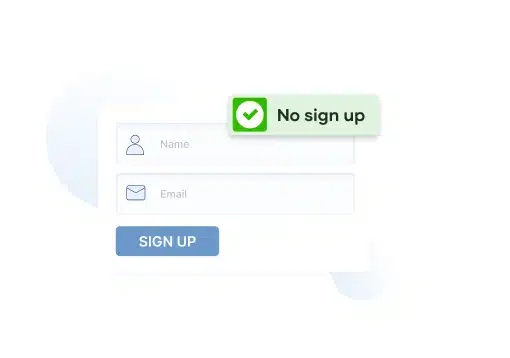
No Signup Needed
You don’t have to register or sign up. Insert your text and get started right away.
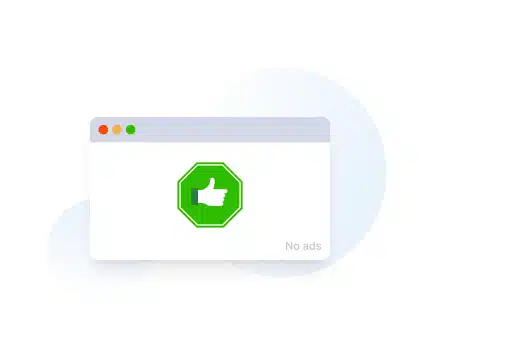
The Paraphraser is Ad-Free
Don’t wait for ads or distractions. The paraphrasing tool is ad-free!
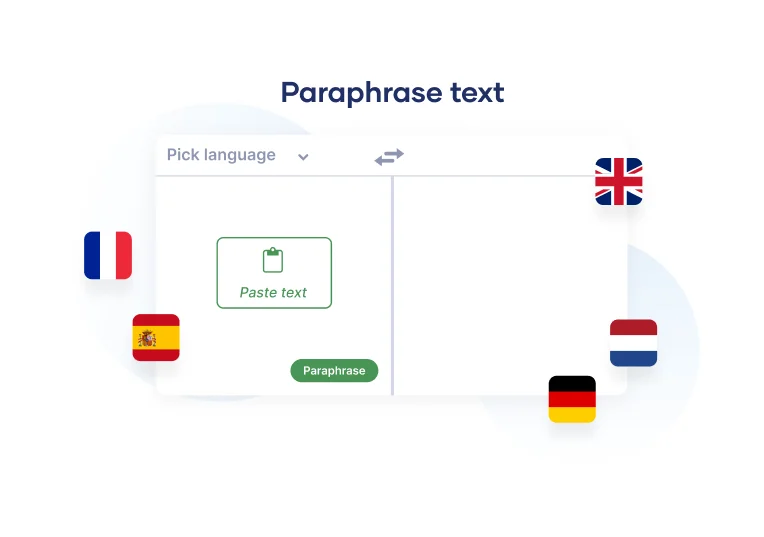
Multi-lingual
Use our paraphraser for texts in different languages.
Features of the paraphrasing tool
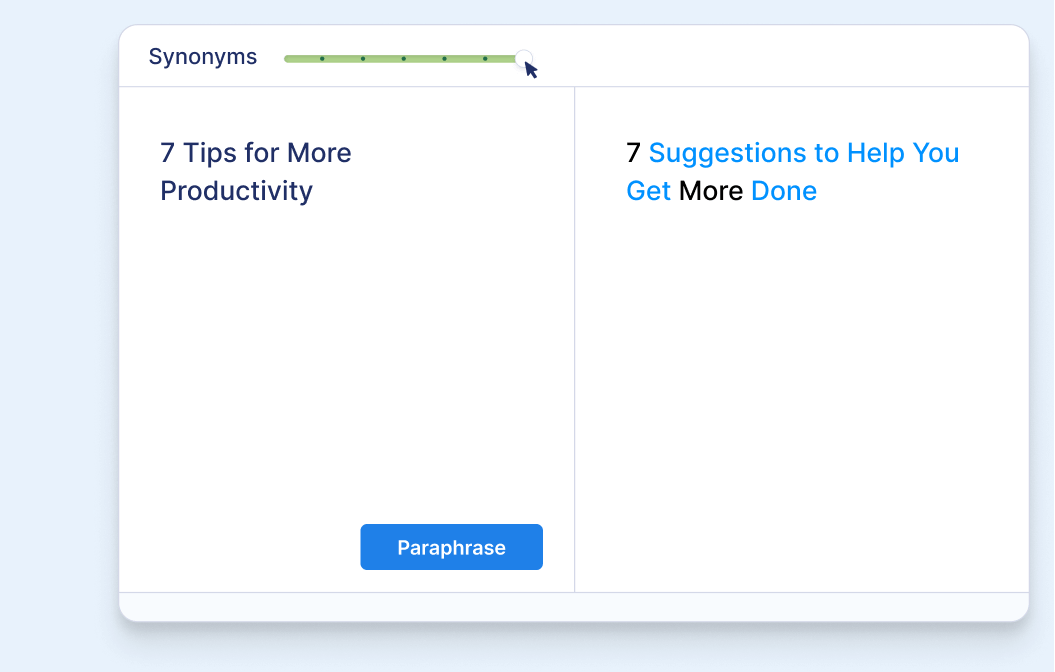
Rephrase individual sentences
With the Scribbr Paraphrasing Tool, you can easily reformulate individual sentences.
- Write varied headlines
- Rephrase the subject line of an email
- Create unique image captions
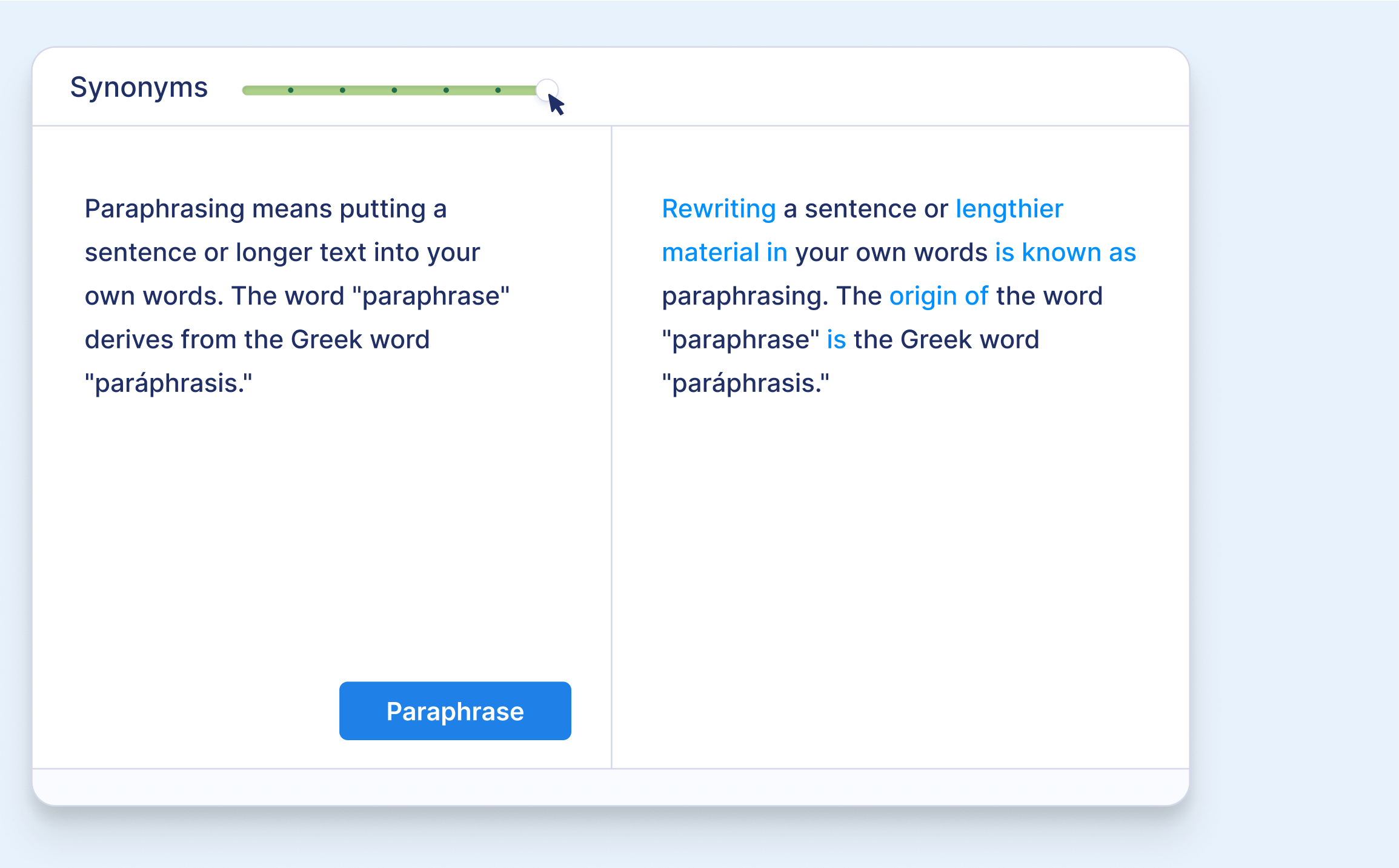
Paraphrase a whole text
Our paraphraser can also help with longer passages (up to 125 words per input). Upload your document or copy your text into the input field.
With one click, you can reformulate the entire text.
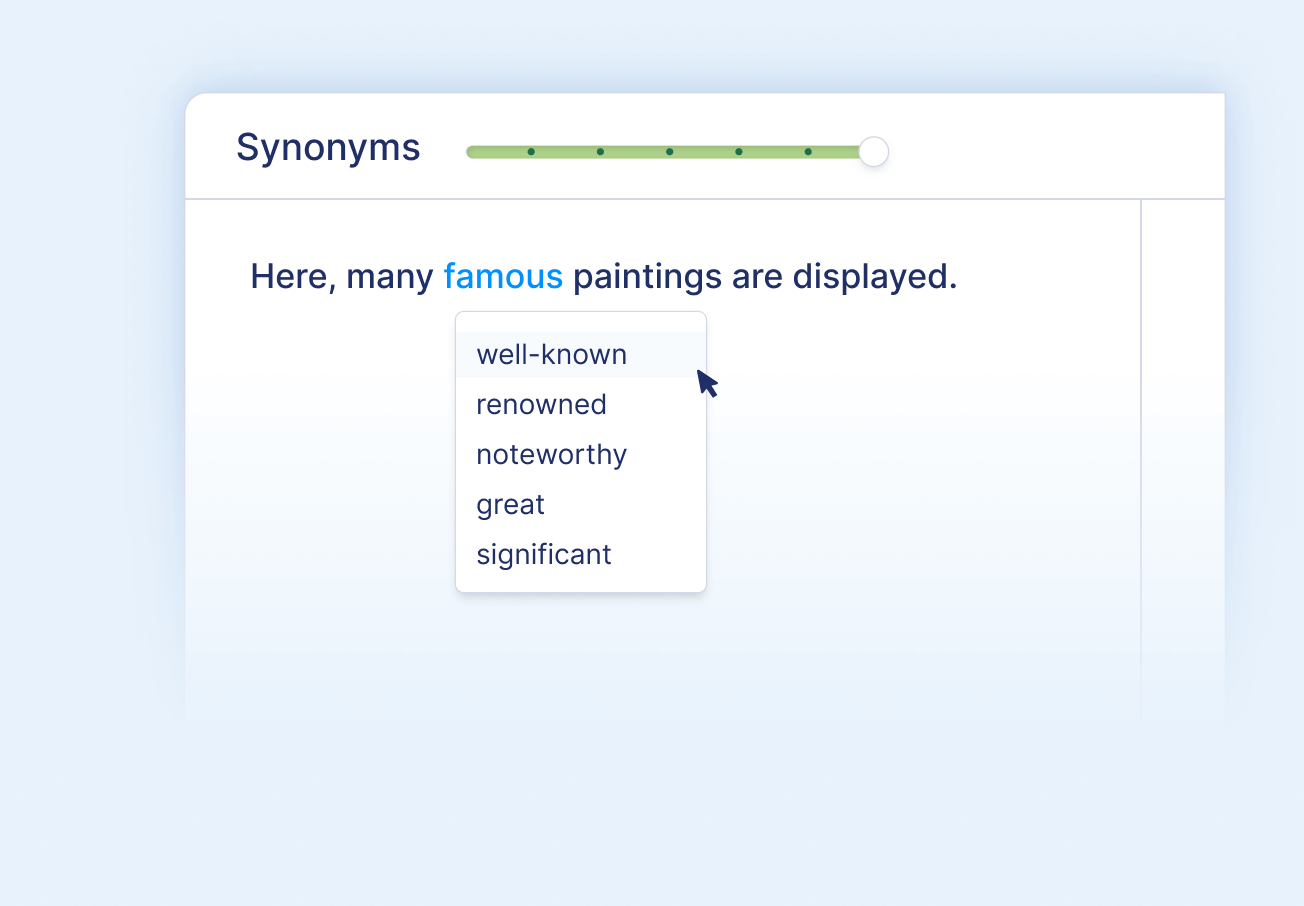
Find synonyms with ease
Simply click on any word to open the interactive thesaurus.
- Choose from a list of suggested synonyms
- Find the synonym with the most appropriate meaning
- Replace the word with a single click
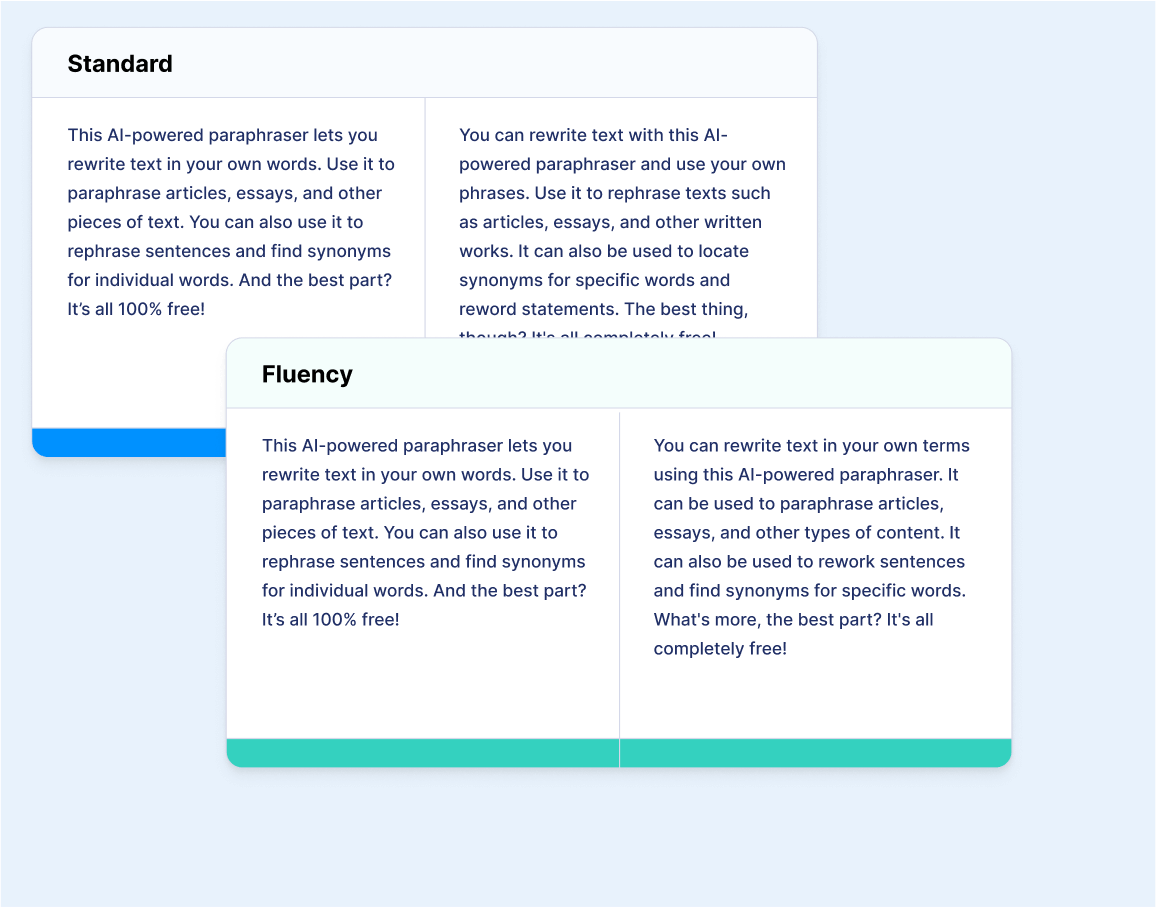
Paraphrase in two ways
- Standard: Offers a compromise between modifying and preserving the meaning of the original text
- Fluency: Improves language and corrects grammatical mistakes
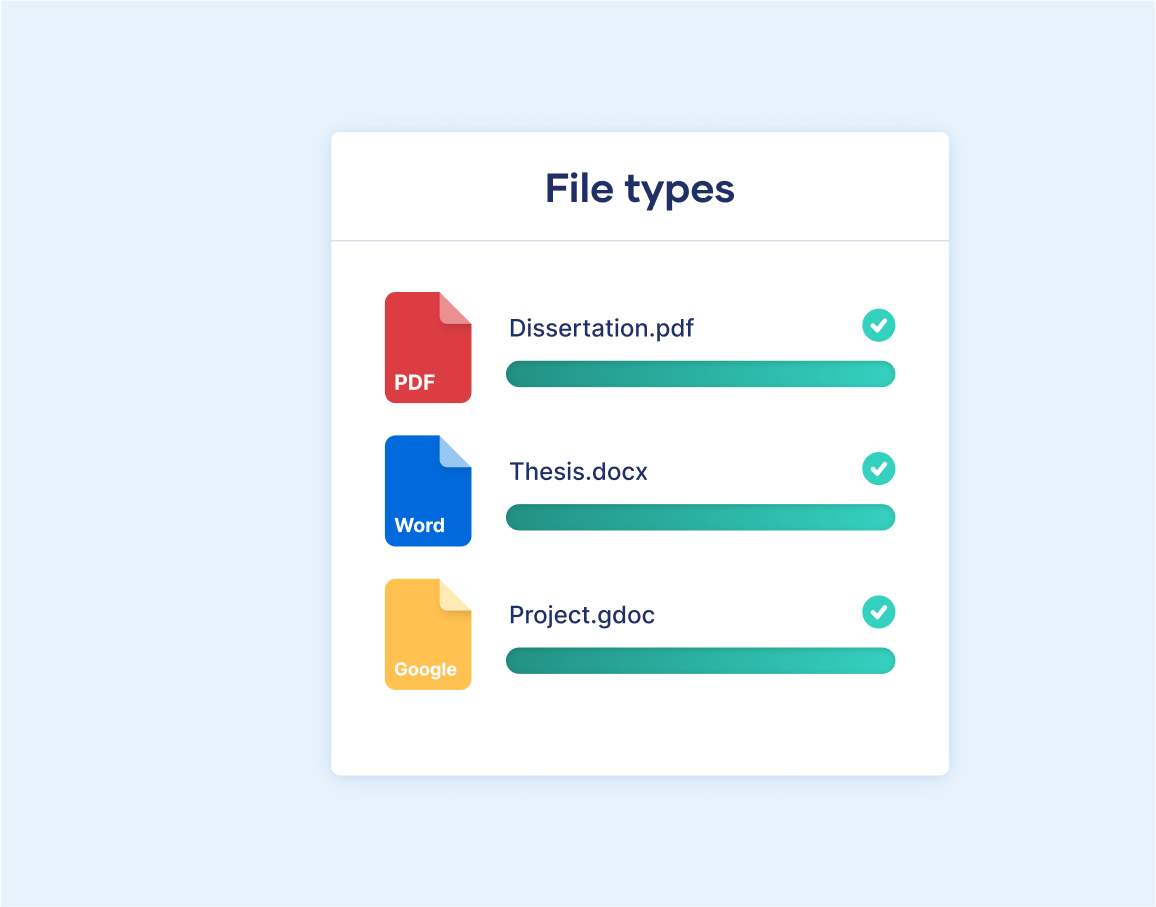
Upload different types of documents
Upload any Microsoft Word document, Google Doc, or PDF into the paraphrasing tool.
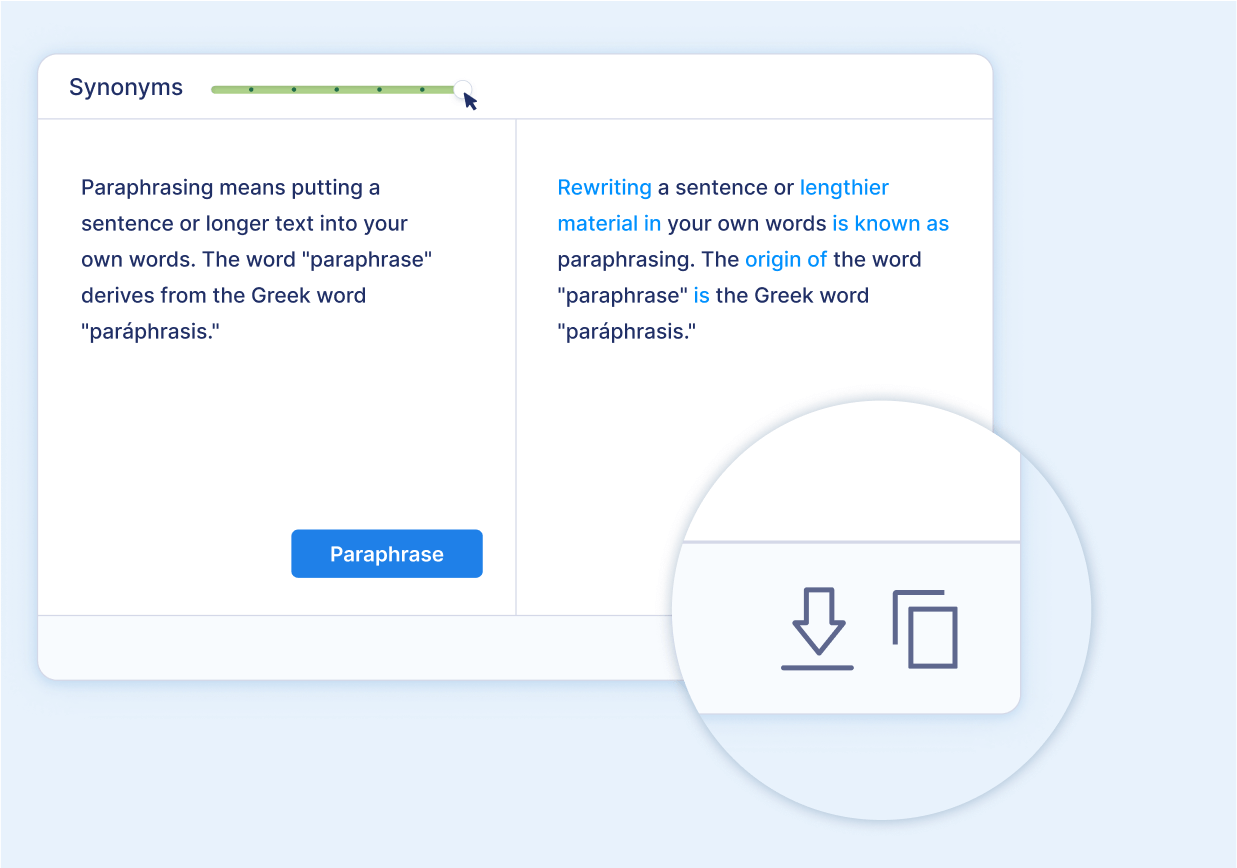
Download or copy your results
After you’re done, you can easily download or copy your text to use somewhere else.
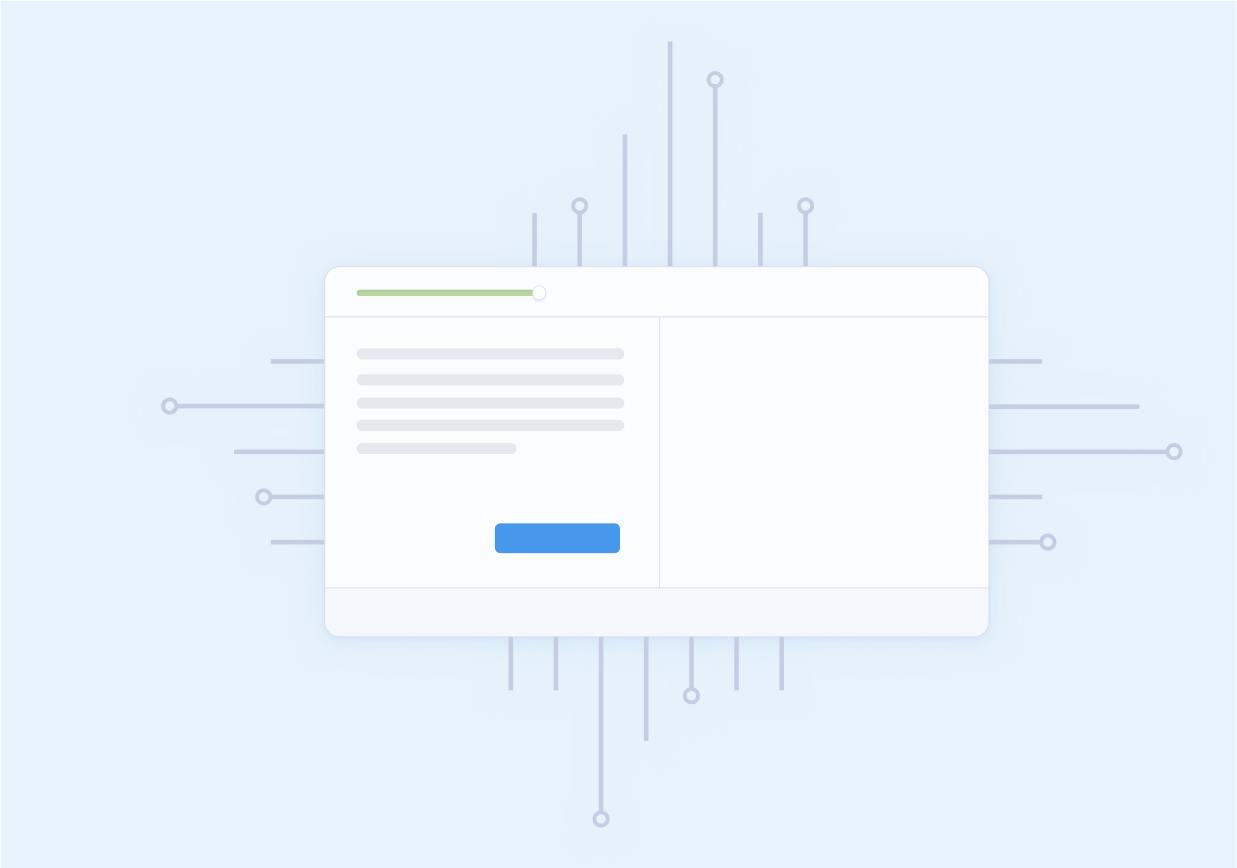
Powered by AI
The paraphrasing tool uses natural language processing to rewrite any text you give it. This way, you can paraphrase any text within seconds.
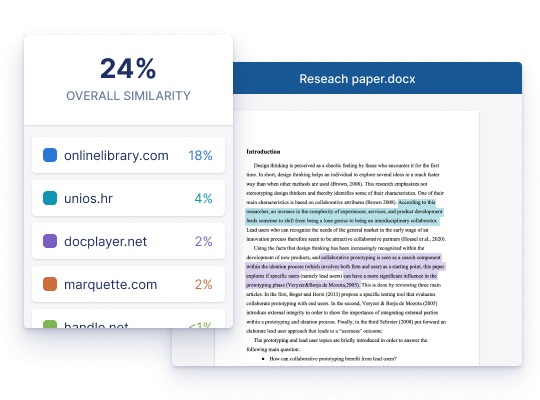
Avoid accidental plagiarism
Want to make sure your document is plagiarism-free? In addition to our paraphrasing tool, which will help you rephrase sentences, quotations, or paragraphs correctly, you can also use our anti-plagiarism software to make sure your document is unique and not plagiarized.
Scribbr’s anti-plagiarism software enables you to:
- Detect plagiarism more accurately than other tools
- Ensure that your paraphrased text is valid
- Highlight the sources that are most similar to your text
Start for free
How does this paraphrasing tool work?
1. put your text into the paraphraser, 2. select your method of paraphrasing, 3. select the quantity of synonyms you want, 4. edit your text where needed, who can use this paraphrasing tool.
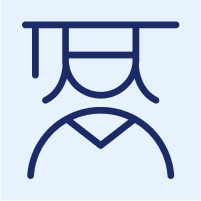
Paraphrasing tools can help students to understand texts and improve the quality of their writing.
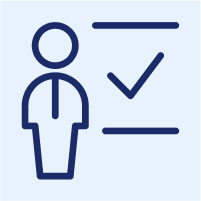
Create original lesson plans, presentations, or other educational materials.
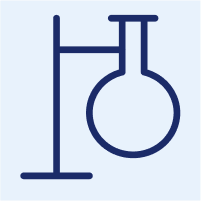
Researchers
Explain complex concepts or ideas to a wider audience.
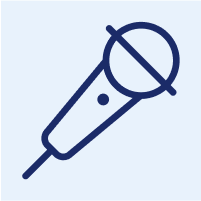
Journalists
Quickly and easily rephrase text to avoid repetitive language.
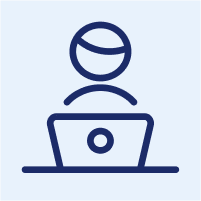
Copywriters
By using a paraphrasing tool, you can quickly and easily rework existing content to create something new and unique.
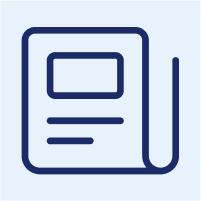
Bloggers can rewrite existing content to make it their own.
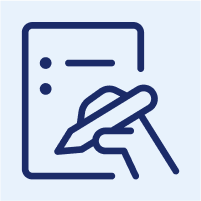
Writers who need to rewrite content, such as adapting an article for a different context or writing content for a different audience.
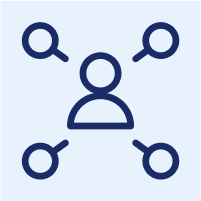
A paraphrasing tool lets you quickly rewrite your original content for each medium, ensuring you reach the right audience on each platform.
The all-purpose paraphrasing tool
The Scribbr Paraphrasing Tool is the perfect assistant in a variety of contexts.
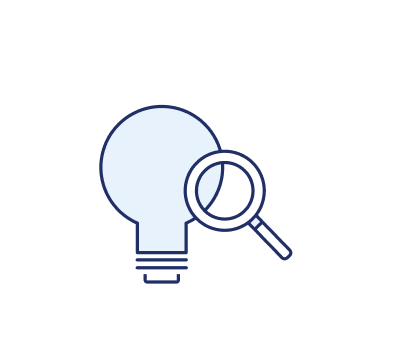
Brainstorming
Writer’s block? Use our paraphraser to get some inspiration.
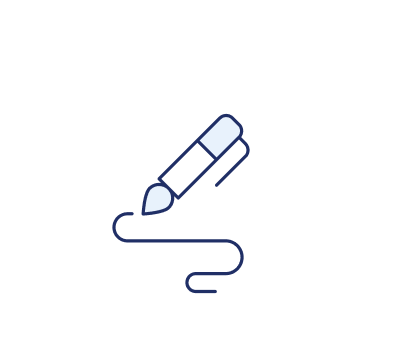
Professional communication
Produce creative headings for your blog posts or PowerPoint slides.
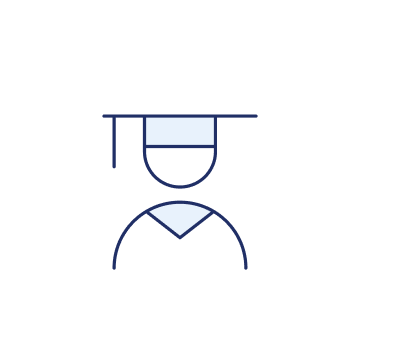
Academic writing
Paraphrase sources smoothly in your thesis or research paper.
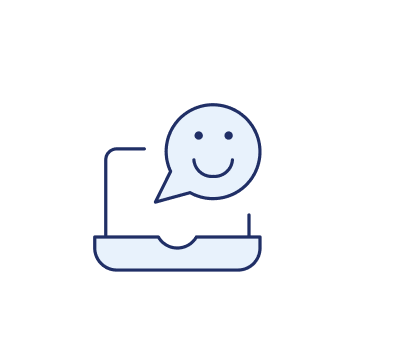
Social media
Craft memorable captions and content for your social media posts.
Paraphrase text online, for free
The Scribbr Paraphrasing Tool lets you rewrite as many sentences as you want—for free.
💶 100% free | Rephrase as many texts as you want |
---|---|
🟢 No login | No registration needed |
📜 Sentences & paragraphs | Suitable for individual sentences or whole paragraphs |
🖍️ Choice of writing styles | For school, university, or work |
⭐️ Rating | based on 13,101 reviews |
Write with 100% confidence 👉
Scribbr & academic integrity.
Scribbr is committed to protecting academic integrity. Our plagiarism checker , AI Detector , Citation Generator , proofreading services , paraphrasing tool, grammar checker , summarizer , and free Knowledge Base content are designed to help students produce quality academic papers.
Ask our team
Want to contact us directly? No problem. We are always here for you.
- Email [email protected]
- Start live chat
- Call +1 (510) 822-8066
- WhatsApp +31 20 261 6040
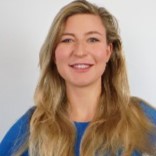
Frequently asked questions
The act of putting someone else’s ideas or words into your own words is called paraphrasing, rephrasing, or rewording. Even though they are often used interchangeably, the terms can mean slightly different things:
Paraphrasing is restating someone else’s ideas or words in your own words while retaining their meaning. Paraphrasing changes sentence structure, word choice, and sentence length to convey the same meaning.
Rephrasing may involve more substantial changes to the original text, including changing the order of sentences or the overall structure of the text.
Rewording is changing individual words in a text without changing its meaning or structure, often using synonyms.
It can. One of the two methods of paraphrasing is called “Fluency.” This will improve the language and fix grammatical errors in the text you’re paraphrasing.
Paraphrasing and using a paraphrasing tool aren’t cheating. It’s a great tool for saving time and coming up with new ways to express yourself in writing. However, always be sure to credit your sources. Avoid plagiarism.
If you don’t properly cite text paraphrased from another source, you’re plagiarizing. If you use someone else’s text and paraphrase it, you need to credit the original source. You can do that by using citations. There are different styles, like APA, MLA, Harvard, and Chicago. Find more information about citing sources here.
Paraphrasing without crediting the original author is a form of plagiarism , because you’re presenting someone else’s ideas as if they were your own.
However, paraphrasing is not plagiarism if you correctly cite the source . This means including an in-text citation and a full reference, formatted according to your required citation style .
As well as citing, make sure that any paraphrased text is completely rewritten in your own words.
Plagiarism means using someone else’s words or ideas and passing them off as your own. Paraphrasing means putting someone else’s ideas in your own words.
So when does paraphrasing count as plagiarism?
- Paraphrasing is plagiarism if you don’t properly credit the original author.
- Paraphrasing is plagiarism if your text is too close to the original wording (even if you cite the source). If you directly copy a sentence or phrase, you should quote it instead.
- Paraphrasing is not plagiarism if you put the author’s ideas completely in your own words and properly cite the source .
Try our services
Monolingual Machine Translation for Paraphrase Generation
- Chris Quirk ,
- Chris Brockett ,
Published by Association for Computational Linguistics
This version corrects an editing error in the text.
We apply statistical machine translation (SMT) tools to generate novel paraphrases of input sentences in the same language. The system is trained on large volumes of sentence pairs automatically extracted from clustered news articles available on the World Wide Web. Alignment Error Rate (AER) is measured to gauge the quality of the resulting corpus. A monotone phrasal decoder generates contextual replacements. Human evaluation shows that this system outperforms baseline paraphrase generation techniques and, in a departure from previous work, offers better coverage and scalability than the current best-of-breed paraphrasing approaches.
- Follow on Twitter
- Like on Facebook
- Follow on LinkedIn
- Subscribe on Youtube
- Follow on Instagram
- Subscribe to our RSS feed
Share this page:
- Share on Twitter
- Share on Facebook
- Share on LinkedIn
- Share on Reddit
The ParaBank project consists of a series of efforts exploring the potential for guided backtranslation for the purpose of paraphrasing with constraints. This work is spiritually connected to prior efforts at JHU in paraphrasing, in particular projects surrounding the ParaPhrase DataBase (PPDB) .
The following are brief descriptions of projects under ParaBank, along with associated artifacts.
ParaBank: Monolingual Bitext Generation and Sentential Paraphrasing via Lexically-constrained Neural Machine Translation
Abstract: We present ParaBank, a large-scale English paraphrase dataset that surpasses prior work in both quantity and quality. Following the approach of ParaNMT, we train a Czech-English neural machine translation (NMT) system to generate novel paraphrases of English reference sentences. By adding lexical constraints to the NMT decoding procedure, however, we are able to produce multiple high-quality sentential paraphrases per source sentence, yielding an English paraphrase resource with more than 4 billion generated tokens and exhibiting greater lexical diversity. Using human judgments, we also demonstrate that ParaBank's paraphrases improve over ParaNMT on both semantic similarity and fluency. Finally, we use ParaBank to train a monolingual NMT model with the same support for lexically-constrained decoding for sentence rewriting tasks.
arXiv: https://arxiv.org/abs/1901.03644
ParaBank v1.0 Full (~9 GB)
ParaBank v1.0 Large, 50m pairs (~3 GB)
ParaBank v1.0 Small Diverse, 5m pairs
ParaBank v1.0 Large Diverse, 50m pairs
Improved Lexically Constrained Decoding for Translation and Monolingual Rewriting
Abstract: Lexically-constrained sequence decoding allows for explicit positive or negative phrasebased constraints to be placed on target output strings in generation tasks such as machine translation or monolingual text rewriting. We describe vectorized dynamic beam allocation, which extends work in lexically-constrained decoding to work with batching, leading to a five-fold improvement in throughput when working with positive constraints. Faster decoding enables faster exploration of constraint strategies: we illustrate this via data augmentation experiments with a monolingual rewriter applied to the tasks of natural language inference, question answering and machine translation, showing improvements in all three.
https://www.aclweb.org/anthology/N19-1090
pMNLI : Paraphrase Augmentation of MNLI
Large-scale, Diverse, Paraphrastic Bitexts via Sampling and Clustering
Abstract: Producing diverse paraphrases of a sentence is a challenging task. Natural paraphrase corpora are scarce and limited, while existing large-scale resources are automatically generated via back-translation and rely on beam search, which tends to lack diversity. We describe ParaBank 2, a new resource that contains multiple diverse sentential paraphrases, produced from a bilingual corpus using negative constraints, inference sampling, and clustering.We show that ParaBank 2 significantly surpasses prior work in both lexical and syntactic diversity while being meaning-preserving, as measured by human judgments and standardized metrics. Further, we illustrate how such paraphrastic resources may be used to refine contextualized encoders, leading to improvements in downstream tasks.
https://www.aclweb.org/anthology/K19-1005
ParaBank v2.0 (~2.3 GB)
Iterative Paraphrastic Augmentation with Discriminative Span Alignment
Abstract: We introduce a novel paraphrastic augmentation strategy based on sentence-level lexically constrained paraphrasing and discriminative span alignment. Our approach allows for the large-scale expansion of existing resources, or the rapid creation of new resources from a small, manually-produced seed corpus. We illustrate our framework on the Berkeley FrameNet Project, a large-scale language understanding effort spanning more than two decades of human labor. Based on roughly four days of collecting training data for the alignment model and approximately one day of parallel compute, we automatically generate 495,300 unique (Frame, Trigger) combinations annotated in context, a roughly 50x expansion atop FrameNet v1.7.
TACL: https://direct.mit.edu/tacl/article/doi/10.1162/tacl_a_00380/100783/Iterative-Paraphrastic-Augmentation-with
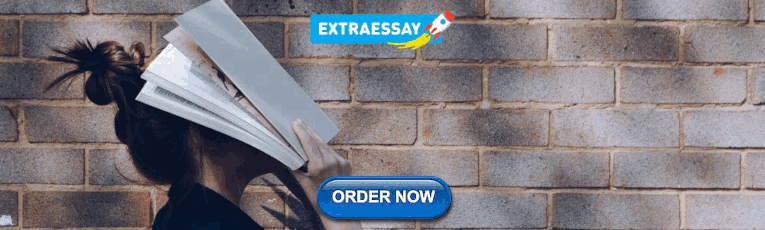
Augmented FrameNet
Name: framenet-expanded-vers2.0.jsonlines.gz
This file contains an expanded 1,983,680-sentence version of FrameNet generated by applying 10 rounds of iterative paraphrastic augmentation to (almost all) of the roughly 200,000 sentences in the original resource. Each line is a JSON object with the following attributes:
- frame_name : The frame to which this sentence belongs.
- lexunit_compound_name : The lexical unit in the form of lemma.POS e.g. increase.n .
- original_string : The raw FrameNet sentence.
- original_trigger_offset : The character-level offset into the raw FrameNet sentence representing the trigger.
- original_trigger : The string value of the trigger.
- frame_id : The associated frame ID from FrameNet data release v1.7.
- lexunit_id : The associated lexical unit ID from FrameNet data release v1.7.
- exemplar_id : The associated exemplar ID from FrameNet data release v1.7.
- annoset_id : The associated annotation set ID from FrameNet data release v1.7.
- outputs : A list containing 10 items, each representing an automatically paraphrased and aligned sentence corresponding to the original FrameNet source sentence.
Each such item is of the form:
- output_string : The tokenized automatically-generated paraphrase.
- output_trigger_offset : The offset into the paraphrase representing the automatically aligned trigger.
- output_trigger : The string value of the automatically aligned trigger in the paraphrase.
- pbr_score : The negative log-likelihood of this paraphrase under the paraphrase model.
- aligner_score : The probability of this alignment under the alignment model.
- iteration : The iteration in which this output was generated (ranges between 1 and 10).
- pclassifier_score : Probability of this output under a classifier trained to optimize for high precision of acceptable outputs.
- rclassifier_score : Probability of this output under a classifier trained to optimize for high recall of acceptable outputs.
The pclassifier_score may be used to select a smaller, higher quality subset of the full dataset whereas the rclassifier_score may be used to obtain a larger but slightly lower quality subset.
Alignment Dataset
Name: alignment-release.jsonlines.gz
This file contains a 36,417-instance manually annotated dataset for monolingual span alignment. Each data point consists of a natural-language sentence (the source), a span in that sentence, an automatically generated paraphrase (the reference), and a span in the reference with the same meaning as the source-side span. All source sentences are taken from FrameNet v1.7.
Each line is a JSON object with the following attributes:
- source_bert_toks : The tokenized source sentence.
- source_bert_span : Offset into the source sentence representing a span.
- `reference spacy tokens``: The tokenized reference sentence.
- reference_span : Offset into the reference sentence representing a span.
- has_corres : Boolean value representing whether the reference sentence contains a span that corresponds in meaning to the source-side span.
- Corpus ID: 13043395
Monolingual Machine Translation for Paraphrase Generation
- Chris Quirk , Chris Brockett , W. Dolan
- Published in Conference on Empirical… 1 July 2004
- Computer Science, Linguistics
Figures and Tables from this paper
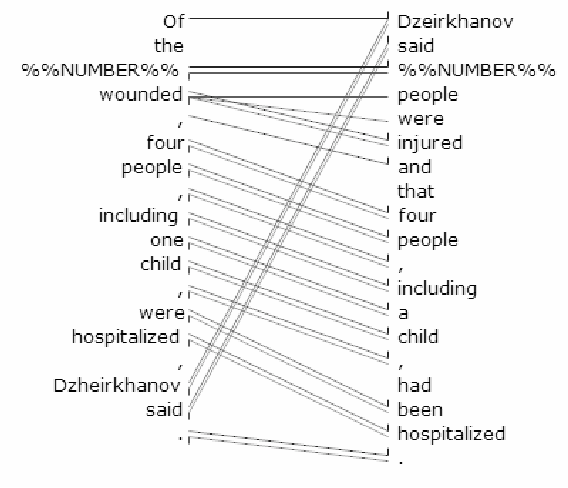
354 Citations
Paraphrase generation as monolingual translation: data and evaluation, neural paraphrase generation using transfer learning, paraphrasing headlines by machine translation sentential paraphrase acquisition and generation using google news, creating and using large monolingual parallel corpora for sentential paraphrase generation.
- Highly Influenced
Neural Machine Translation for Malayalam Paraphrase Generation
Comparing phrase-based and syntax-based paraphrase generation, neural machine translation for paraphrase generation, paraphrase generation as zero-shot multilingual translation: disentangling semantic similarity from lexical and syntactic diversity, support vector machines for paraphrase identification and corpus construction, constructing corpora for the development and evaluation of paraphrase systems, 27 references, extracting structural paraphrases from aligned monolingual corpora, learning to paraphrase: an unsupervised approach using multiple-sequence alignment.
- Highly Influential
Automatic paraphrase acquisition from news articles
Extracting paraphrases from a parallel corpus, extracting paraphrases from aligned corpora, syntax-based alignment of multiple translations: extracting paraphrases and generating new sentences, unsupervised construction of large paraphrase corpora: exploiting massively parallel news sources, example-based machine translation using dp-matching between work sequences, the cmu statistical machine translation system, statistical phrase-based translation, related papers.
Showing 1 through 3 of 0 Related Papers
- Original article
- Open access
- Published: 26 January 2017
Using Internet based paraphrasing tools: Original work, patchwriting or facilitated plagiarism?
- Ann M. Rogerson 1 &
- Grace McCarthy 1
International Journal for Educational Integrity volume 13 , Article number: 2 ( 2017 ) Cite this article
77k Accesses
61 Citations
167 Altmetric
Metrics details
A casual comment by a student alerted the authors to the existence and prevalence of Internet-based paraphrasing tools. A subsequent quick Google search highlighted the broad range and availability of online paraphrasing tools which offer free ‘services’ to paraphrase large sections of text ranging from sentences, paragraphs, whole articles, book chapters or previously written assignments. The ease of access to online paraphrasing tools provides the potential for students to submit work they have not directly written themselves, or in the case of academics and other authors, to rewrite previously published materials to sidestep self-plagiarism. Students placing trust in online paraphrasing tools as an easy way of complying with the requirement for originality in submissions are at risk in terms of the quality of the output generated and possibly of not achieving the learning outcomes as they may not fully understand the information they have compiled. There are further risks relating to the legitimacy of the outputs in terms of academic integrity and plagiarism. The purpose of this paper is to highlight the existence, development, use and detection of use of Internet based paraphrasing tools. To demonstrate the dangers in using paraphrasing tools an experiment was conducted using some easily accessible Internet-based paraphrasing tools to process part of an existing publication. Two sites are compared to demonstrate the types of differences that exist in the quality of the output from certain paraphrasing algorithms, and the present poor performance of online originality checking services such as Turnitin® to identify and link material processed via machine based paraphrasing tools. The implications for student skills in paraphrasing, academic integrity and the clues to assist staff in identifying the use of online paraphrasing tools are discussed.
Introduction
A casual question from a student regarding another student’s contribution to a group work assignment inadvertently led to an explanation of some unusual text submitted for assessment in a previous session. The student queried whether the use of a paraphrasing tool was acceptable in the preparation of a written submission for assessment. Discussing the matter further, the student revealed that they had queried the writing provided by one member of the group as their contribution to the report “did not make sense”. When asked, the group member stated that they had taken material from a journal article and used a fee free Internet paraphrasing tool “so that the words were not the same as the original to avoid plagiarism”. After the clarification, the group did not accept the submission from their team member and instead worked with them to develop an original submission. The group were thanked for their approach to the situation; however this revelation provided a potential explanation for some analogous submissions for previous subjects.
One particular submission from a previous subject instance had phrasing that included “constructive employee execution” and “worker execution audits” for an assessment topic on employee performance reviews. The student was interviewed at the time about why they had submitted work relating the words execution and employees and no satisfactory or plausible explanation was provided. With a new awareness of paraphrasing tools, a Google search revealed in excess of 500,000 hits and a simple statement was entered into one tool to test this connection. Testing the phrase ‘employee performance reviews’ via the top search response revealed an explanation for the unusual student submission as the paraphrase was returned as ‘representative execution surveys’. Choosing to use output generated by these tools begs the question – is it original work, patchwriting or facilitated plagiarism?
Having had our attention drawn to the existence and use of paraphrasing tools it was decided to investigate the phenomenon. What became apparent was that the ease of access to and use of such tools was greater than first thought. Consequently it is important to bring the use and operation of paraphrasing tools to a wider audience to encourage discussion about developing individual writing skills and improve the detection of these emerging practices, thereby raising awareness for students, teachers and institutions.
Paraphrasing and patchwriting
Academic writing is largely reliant on the skill of paraphrasing to demonstrate that the author can capture the essence of what they have read, they understand what they have read and can use the appropriately acknowledged evidence in support of their responses (Fillenbaum, 1970 ; Keck, 2006 , 2014 ; Shi, 2012 ). In higher education a student’s attempts at paraphrasing can provide “insight into how well students read as well as write” (Hirvela & Du, 2013 , p.88). While there appears to be an underlying assumption that students and researchers understand and accept that there is a standard convention about how to paraphrase and appropriately use and acknowledge source texts (Shi, 2012 ), there can be inconsistencies between underlying assumptions in how paraphrases are identified, described and assessed (Keck, 2006 ). Poorer forms of paraphrasing tend to use a simplistic approach where some words are simply replaced with synonyms found through functionality available in word processing software or online dictionaries. This is a form of superficial paraphrasing or ‘close paraphrasing’ (Keck, 2010 ) or ‘patchwriting’ (Howard, 1995 ). The question as to “the exact degree to which text must be modified to be classified as correctly paraphrased” (Roig, 2001 , p.309) is somewhat vague, although Keck ( 2006 ) outlined a Taxonomy of Paraphrase Types where paraphrases are classified in four categories ranging from near copy to substantial revision based on the number of unique links or strings of words.
Research in this area appears to concentrate more specifically on second language (L2) students rather than students per se (For a review see Cumming et al. 2016 ) although many native English writers may also lack the language skills to disseminate academic discourse in their own voice (Bailey & Challen, 2015 ). Paraphrasing is a skill that transcends the written form as it is actually a communication strategy required for all language groups in interpersonal or intergroup interactions and includes oral (Rabab’ah, 2016 ) and visual forms (Chen et al. 2015a ). Paraphrasing allows the same idea to be expressed in different ways as appropriate for the intended audience. It can also be used for persuasion (Suchan, 2014 ), explanations (Patil & Karekatti, 2015 ) and support (Bodie et al. 2016 ). In coaching, paraphrasing is used to ensure that the coach has correctly understood what the coachee is saying, thus allowing the coachee to further clarify their meaning (McCarthy, 2014 ).
Online writing tools
The prevalence and easy access to digital technologies and Internet-based sources have shifted “the way knowledge is constructed, shared and evaluated” (Evering & Moorman, 2012 , p.36). However the quality, efficacy, validity and reliability of some Internet-based material is questionable from an educational standpoint (Niño, 2009 ). Internet-based paraphrasing tools are text processing applications and associated with the same approaches used for machine translation (MT). While MT usually focusses on the translation of one language to another, the broader consideration of text processing can operate between or within language corpuses (Ambati et al. 2010 ).
Internet-based conversion and translation tools are easily accessible, and a number of versions are available to all without cost (Somers, 2012 ). Developments in the treatment of translating natural language as a machine learning problem (known as statistical machine translation - SMT) are leading to continual improvements in this field although the linguistic accuracy varies based on the way each machine ‘learns’ (Lopez, 2008 ). The free tools available via the Internet lack constant updates and improvements as the code is controlled by webmasters and not by experts in MT (Carter & Inkpen, 2012 ). This means advances in methods and algorithms are not always available to individuals relying on free Internet based tools. Consequently there are issues with the quality of MT which may require a level of post-editing to correct the raw output so that it is fit for purpose (Inaba et al. 2007 ).
Post-editing of an online output may be problematic or difficult for an individual with a low level of proficiency in the language they are being taught or assessed in as grammatical inaccuracies and awkward phrasing cannot be easily identified and therefore corrected (Niño, 2009 ). Where a student is considered to lack the necessary linguistic skills, the errors or inaccuracies may be interpreted by assessors as a student having a poor understanding of academic writing conventions rather than recognising that a student may not have written the work themselves. Where an academic is working in an additional language, they may find the detection of the errors or inaccuracies more difficult to identify.
Nor is the issue of paraphrasing or article spinning tool use confined to students. Automated article spinners perform the same way as paraphrasing tools, where text is entered into one field with a ‘spun’ output provided on the same webpage. They were initially developed for re-writing web content to maximise exposure and links to particular sites, without being detected as a duplicate of original content (Madera et al. 2014 ). The underlying purpose appears to allow website owners to “make money from the new, but not strictly original, article” (Lancaster & Clarke, 2009 ). These sites are freely available to students leading to a new label covering the use of these tools as ‘essay spinning’ (Lancaster & Clarke, 2009 , p.26). However, these spinning tools are equally available to academics who may be enticed with the notion of repurposing already published content as a way of increasing research output.
Although the quality levels of MT output varies widely, careful editing and review can address the errors further disguising the original source material (Somers, 2012 ). Roig ( 2016 ) highlights that some forms of text recycling are normal in academic life such as converting conference presentations and theses to journal articles and the textual reuse between editions of books, as long as there is appropriate acknowledgement of the original source. However Roig also points out that authors should be concerned about reusing previous work as with technological advances it will not be long before all forms of academic written work can “be easily identified, retrieved, stored and processed in ways that are inconceivable at the present time” (Roig, 2016 , p.665).
The fact remains that taking another author’s work, processing it through an online paraphrasing tool then submitting that work as ‘original’ is not original work where it involves the use of source texts and materials without acknowledgement. The case of a student submitting work generated by an online tool without appropriate acknowledgement could be considered as a form of plagiarism, and the case of academics trying to reframe texts for alternate publications could be considered as a form of self-plagiarism. Both scenarios could be considered as ‘facilitated plagiarism’ where an individual actively seeks to use some form of easily accessible Internet-based source to prepare or supplement submission material for assessment by others (Granitz, 2007 ; Scanlon & Neumann, 2002 ; Stamatatos, 2011 ). Applying technology to identify where the paraphrasing tools have been used is difficult as detection moves beyond text summarisation and matching to comparison of meaning and evaluation of machine translation (Socher et al. 2011 ).
Furthermore, students using an online paraphrasing system fail to demonstrate their understanding of the assessment task and hence fail to provide evidence of achieving learning outcomes. If they do not acknowledge the source of the text which they have put through the paraphrasing tool, they are also guilty of academic misconduct. On both counts, they would not merit a pass in the subject for which they submit such material.
Methodology
In order to test the quality of output generated by some free Internet based paraphrasing tools and how the originality of the output is assessed by Turnitin®, the following experiment was conducted. A paragraph from an existing publication by this article’s authors from a prior edition of the International Journal of Educations Integrity (IJEI) was selected to be the original source material (McCarthy & Rogerson, 2009 , p.49). To assess how a paraphrasing tool processes an in-text citation, one in-text citation was included (Thatcher, 2008 ). A set of three bibliographic entries from the reference list of the same article were also selected to test how references are interpreted.
As students are more likely to use Google as the Internet search engine of choice and rely on results near the top of page (Spievak & Hayes-Bohanan, 2016 ), this approach was used to identify and select some online paraphrasing tools for testing. The selected paragraph (including the in-text citation), and the selected references were entered into the first two hits on a Google search on www.google.com.au for ‘ paraphrasing tools ’. Consequently the sites used for the experiment were www.paraphrasing-tool.com (Tool 1) and www.goparaphrase.com (Tool 2).
The next step was to compare the outputs from the original journal article material to the outputs of Tool 1 and Tool 2. Exact matches to the original text were observed, tagged and highlighted in grey. Matches between the two paraphrasing outputs that did not match the original source were highlighted by placing the relevant text in a box. Contractions and unusual matches were highlighted by double underlining the text. For the first set of comparisons (paragraph with an in-text citation) the following summary characteristics were calculated: total word counts, total word matches and percentage of similarity to the original paragraph.
In order to identify how Turnitin® interpreted the paragraph and bibliographic outputs from the paraphrasing tools, the original source material and two paraphrasing outputs were uploaded to Turnitin® to check whether the journal publication could be identified. Turnitin® comprises a suite of online educative writing and evaluation tools where assessment tasks can be uploaded, checked and assessed ( www.turnitin.com ). It can be accessed via the Internet or through an interface with an institutional learning management system (LMS). The originality checking area compares a submission against a range of previously published materials and a database of previously submitted assignments. The system generates an originality report where text that matches closely to a previously published or submitted source is highlighted by colour and number with links provided to publicly accessible materials. Matches to papers submitted at other institutions cannot be accessed without the express permission of the owning institution. As Baggaley and Spencer note ( 2005 ) Turnitin® originality reports require careful analysis, for the reports identify text “which may or may not have been correctly attributed” (Baggaley & Spencer, 2005 , p. 56) and cannot be used as the sole determinant of whether or not a work is plagiarised or if source materials have been inappropriately used (Rogerson, 2014 ).
A separate Turnitin® assessment file was created for the experiment on an institutional academic integrity LMS site (Moodle) where a bank of dummy student profiles is available for testing purposes. Three dummy student accounts were used to load the individual ‘outputs’ under two assignment parts. The uploads included one instance of the source material in order to generate comparative originality reports for both the paragraph outputs (loaded under part 1) and the reference list outputs (loaded under part 2). For both sets of outputs the overall Turnitin® similarity percentages and document matches were reviewed for comparison purposes.
The highlighted comparisons of the paragraph outputs are presented in Fig. 1 (comparing Tool 1) and Fig. 2 (comparing Tool 2). The summary characteristics for the paragraph outputs are presented in Table 1 .
Comparison with output from www.paraphrasing-tool.com . Original source materials from McCarthy and Rogerson ( 2009 , p.49) and citing Thatcher ( 2008 )
Comparison with www.goparaphrase.com . Original source materials from McCarthy and Rogerson ( 2009 , p.49) and citing Thatcher ( 2008 )
There are obvious differences in how the online paraphrasing tools have reengineered the original work based on the number of identifiable matches between the original and output texts. For example there are differences in how words such as plagiarism are expressed (Original source: plagiarism ; Tool 1: copyright infringement ; Tool 2: counterfeit ). Both tools have used additional words (Tool 1: additional five words; Tool 2: additional 20 words). The output from Tool 1 has used 77 words or 50% of the words in the original paragraph but these were predominately coordinating conjunctions. Tool 1 has followed the correct use of capitalisations in all words and sentences, however Tool 2 has not capitalised words such as English, and Chinese, but did capitalise seven random words mid-sentence ( Audit, Numerous, Concerning, Likewise, Taking, and What’s ). In addition Tool 2 used contractions ( doesn’t ) and the words ‘ can have ’ in the original have been reprocessed to ‘ camwood ’.
The highlighted comparisons of the reference section outputs are presented in Fig. 3 (comparing the original source with Tool 1 and Tool 2). The summary characteristics of the Turnitin® results for the reference section outputs are presented in Table 2 .
Comparison with three reference list entries. Original source materials from McCarthy and Rogerson ( 2009 , p.56) and citing Carroll and Appleton ( 2005 ), Crisp ( 2007 ), and Dahl ( 2007 )
The Turnitin® results for both the paragraph and reference list uploads identified the original source as 100% match to the online location of the journal supporting Turnitin’s® claim in relation to identifying legitimate academic resources. What is of concern is Turnitin’s® apparent inability to identify the similarities evident by a manual comparison of the source and outputs. Figures 1 and 2 demonstrate the similarities between the original source materials and the output of the tools yet the similarity percentages noted in Table 2 indicate that the re-engineered paragraphs are not detected. One of the current limitations of Turnitin® is that it can detect some but not all cases of synonym replacement (Menai, 2012 ). Despite the patterned nature of the text matching identified through a visual examination of the output, the machine-based originality similarity checking software continues to have limitations in identifying materials that appear to be plagiarised through the use of an online paraphrasing tool or language translation application.
Turnitin® was more successful in matching up bibliographic data to the original source. This was likely due to the fact that the paraphrasing tools did not alter (or barely altered) long strings of numbers, letters and website URLs. The higher Turnitin® match to the output from Tool 1 (72% similarity) was due to the retention of most of the journal name ( International replaced with Global ) however the author name ‘ Crisp’ was altered to ‘ Fresh’ . The output from Tool 2 retained the authors’ last names, but added in 11 additional words to replace author Dahl’s first initial of ‘S’ which would have affected the calculation of similarity percentage. It is interesting that the change to lower case for authors’ initials appeared to impact on Turnitin’s® capacity to identify the authors in the first reference and missed the end of the journal details in the third reference, which also would have contributed to the lower similarity percentage. This led to Turnitin® overlooking 15 word matches and 13 other number and character matches in the Tool 2 submission that were identified as direct matches in the Tool 1 output.
A further examination of both sets of outputs from the paraphrasing tools identified that the tools appear to retain most words and formatting close to punctuation. For example both tools retained [ , policed, ], and the name and intext citation [Thatcher ( 2008 )] in the paragraph comparison, and a string in the reference section comparison [ Integrity, 3, 3–15, from http://www . ]. Without knowing the algorithms for the paraphrasing tools or Turnitin®, patterns such as these can only be observed rather than analysed.
The outputs and comparisons presented in Figs. 1 and 2 appear more like patchwriting rather than paraphrasing. Li and Casanave ( 2012 ) argue that patchwriting is an indication that the student is a novice writer still learning how to write and understand the “complexities of appropriate textual borrowing” (Li & Casanave, 2012 , p.177) although their study was confined to L2 students submitting assessment material in English. They further argue that deeming text as patchwriting does not attract the same negative connotations of plagiarism nor would it attract the same penalties. In our examples the patterns of text, language and phrasing can identify a student requiring learning support. This determination is likely due to the presence of poor expression, grammatical errors and areas of confused meaning which are sometimes referred to as a ‘word salad’. The term word salad is drawn from psychology but has been adopted in areas such as MT to classify unintelligible and random collections of words and phrases (Definition:word salad, 2016 ). Word salads are produced by MT “when translation engines fail to do a complete analysis of their input” (Callison-Burch & Flournoy, 2001 , p.1).
While the output from Tool 1 is mainly intelligible, some of the results from Tool 2 could be classified as word salads, for example in the last line the following string of words was produced ‘ duplicating Likewise an approach about Taking in starting with What's more paying admiration to previous aces’ . If an unintelligible string of words was submitted as part of an assessment task it may be a reason to have a conversation with a student to understand how they are going about their writing, and to determine if paraphrasing tools or article spinners have contributed. Where a citation is provided, it may be a case of a student having a poor understanding of academic writing conventions. Where there is no citation or any reference to the original source the situation may warrant investigation under academic integrity institutional policies and procedures.
If the percentage calculations presented in Fig. 1 are compared with Kecks ( 2006 ) Taxonomy of Paraphrase Types , the outputs from the online tools would fall into the category of paraphrases with minimal revision when compared to the original text (Keck, 2014 , p.9). The manual comparison of documents in this experiment indicates a level of patchwriting, however Turnitin® could not establish a relationship between the original source paragraph and the machine generated paraphrasing-tool outputs. It is more akin to some of the plagiarism behaviours described by Walker ( 1998 , p.103) such as “illicit paraphrasing” where material is reused without any source acknowledgement or even “sham paraphrasing” where text is directly copied but includes a source acknowledgement. This is a cause for concern as the comparison with the online paraphrasing tool output was only possible as the original source was known. It is not just a question of percentages but in the patterns clearly visible in Figs. 1 , 2 and 3 . Consequently, this set of experiments indicates a level of similarity that is concerning in two key areas, firstly where the original source is not acknowledged or identifiable, and secondly if this level of similarity were found in student work, it would suggest that the student may not have understood the material, or at least that he/she has not demonstrated their understanding.
Manual analysis and academic judgement are integral parts of the process of detection of plagiarised materials (Bretag & Mahmud, 2009b ), and are heavily reliant on the level of experience an assessor has in identifying clues, markers and textual patterns (Rogerson & Bassanta, 2016 ). In this experiment the original source of the plagiarised materials would be difficult to identify, however the presence of clues and patterns may be sufficient to motivate a lecturer or tutor to initiate an initial conversation with a student to determine whether the work is actually the student’s own (Somers et al. 2006 ).
A further investigation of the results from the Google search on ‘paraphrasing tools’ identified that many of the sites have multiple public faces—that is that there are additional URLs that direct users back to the same paraphrasing machine. The purpose behind the existence of the sites is not clear. The sites do carry Internet advertising so their existence and multiple faces may be related to a way to generate income. Alarmingly the sites examined in this study showed advertisements for higher education institutions which could be misinterpreted by users as tacit approval for the sites and their output. Other sites highlight that rudimentary paraphrasing tools are highly inaccurate but promote their paid services to correct the output—i.e. a process that could be interpreted as another form of contracted plagiarism (Clarke & Lancaster, 2013 ).
One of the questions that arises in assessing work as plagiarised is associated with intentionality—that is, did the person intend to deceive another about the originality of work (Lee, 2016 ). In the case of students “it is the inappropriate research and writing practices and the resulting misappropriate or misuse of information that leads students to breach academic integrity expectations” (Pfannenstiel, 2010 , p.43). Pfannenstiel’s use of the word ‘expectations’ is both interesting and enlightening as it is probable that differences in expectations is what is at the crux of the issue with online paraphrasing or article spinning tools. Expectations can be influenced by cultural and educational backgrounds, a lack of understanding or skills in paraphrasing and linguistic and language resources (Cumming et al., 2016 ; Sun, 2012 ). For example: a student may sincerely believe that as they have not submitted an exact copy of the original source, and that there is no evidence of match to the original source via online originality checking software that they have met the objective of submitting original work. Conversely, an academic may reasonably consider this to be direct plagiarism as the student copied the original work of someone else and reused it without any acknowledgement (Davis & Morley, 2015 ). This area of confusion was noted in Shi’s ( 2012 ) study where a student stated that using a translation of an original text did not require acknowledgment of the original source as the translation was not directly the original source. (Shi, 2012 , p.140).
While Turnitin® cannot currently connect the writing and the paraphrases in this experiment, it and other MT tools are in a constant state of evolution and their ability to identify poor quality machine translated text will continue to improve over time (Carter & Inkpen, 2012 ). In order to test the progress, Carter and Inkpen ( 2012 ) suggest that multiple tests of the same piece of text be conducted over a period of years to measure both the quality of output and the ability to detect their use. The literature reviewed in this area focusses on the detection of phrases and sentences, with Socher et al. ( 2011 ) noting that once detection switches from phrases to full sentences a comparison of meaning is more difficult for a machine to learn.
This article does not attempt to outline all the work being undertaken in this area, instead it highlights that there is research being undertaken to develop and further enhance MT (encoding and decoding) and detection of MT use. This includes computers learning computational semantics and managing expanded vocabularies to move beyond recognition of specific tasks (Kiros et al., 2015 ). Turnitin®’s ability to match large sections of text outside of their own repository of previously submitted assessment tasks is very useful because the majority of academic materials that can be plagiarised are text based (Bretag & Mahmud, 2009a ). Using text-matching as a basis for detection instead of semantic matching means that uses of online paraphrasing tools and article spinners continues to be difficult for technology to detect at this time. Therefore for the foreseeable future the onus of detection of unoriginal material remains with academics, lecturers and teachers (Rogerson, 2014 ).
Further confusion arises when institutions develop computer based paraphrasing tools as a way of developing English language writing skills for L2 students. Aware of the difficulties that L2 learners have with paraphrasing tasks, Chen, Huang, Chang and Liou developed a web and corpus based ‘paraphrasing assistant system’ designed to suggest paraphrases with corresponding Chinese translations (Chen et al. 2015b , p.23). Students familiar with using such a system in their home country may seek similar assistance if studying abroad. Without access to an approved technology they may seek to discover similar assistance tools on the Internet—where they can easily locate the paraphrasing tools identified in this experiment. These same students may also lack the judgement skills to discern the difference between the output from approved and poor quality online tools whether they are paraphrasing tools, article spinners or language translators.
Implications for practice: working with students
One way of confronting or approaching this issue is to openly demonstrate to students the errors and inaccuracies that can result in using online tools (Niño, 2009 ). Communicating proactively about the issue provides students with a greater awareness of the problems that can result from using online paraphrasing sites as well as ensuring that students understand that they should not expect to graduate unless they can demonstrate they understand the course material. Their current and future employers have the right to expect that for example, a student graduating with a degree in marketing will be able to articulate their understanding of marketing concepts. Proactive approaches can also promote learning development and support services offered by the educational institution providing students with advice about paraphrasing and strategies for improving their writing skills and therefore avoiding problematic practices. This educates students about alternatives to using online machine text generation tools.
Some students have expressed concerns that other students will continue to take advantage of technology based aids even though they had been told not to use them and knowing that to do so could be classified as cheating (Burnett et al. 2016 ). Students who do not cheat but put in the effort themselves are usually outraged if fellow students get away with cheating and may even bring cases they notice to the institutions’ attention (Warnock, 2006 ). This was the case with the casual comment by the student who brought the online paraphrasing tools to our attention. The actions of our students working with their group member to develop their own work also demonstrates how honest students can be allies in upholding the academic standards of the institution (Bretag & Mahmud, 2016 ). If the benefits of learning and developing individual paraphrasing skills are linked to the broader benefits of effective interpersonal and intergroup communication, the open approach to confronting and discussing the issue may be more successful.
Implications for practice: working with staff
The development of reading, summarising and paraphrasing skills are not the sole responsibility of learning developers. Educators need to embed academic skills in lectures and tutorials and provide feedback on student progress measured through effective assessment (Sambell et al. 2013 ). Clear assessment requirements and use of rubrics indicate the importance and differences to grades for the various levels of academic skills (Atkinson & Lim, 2013 ) providing students with a reason to develop their skills. Effective feedback assists students in identifying where they have achieved certain levels of academic skills and which skills require further development (Evans, 2013 ).
A further approach to tackling the issue is to re-design assessment tasks to include an oral component where the student has to present a summary of their argument and answer questions. This approach can ensure that the student understands and has achieved the learning outcomes, although it is no guarantee of the student’s academic integrity in preparing for their presentation. Finally, academics can also be trained to look for linguistic markers indicating the possibility of the use of such online paraphrasing tools so that they can investigate cases appropriately. Such markers include sentences that do not make sense, odd use of capitalisations in the middle of sentences, unusual phrases and, in the case where students have reprocessed work from old textbooks, out of date and superseded reference material.
Conclusion and recommendations for further research
This study has demonstrated that students can use online paraphrasing tools or article spinners in ways that avoid detection by originality checking software such as Turnitin®. Whether or not it is the student’s intent to avoid plagiarism is not the issue examined here. Rather, the intent of this paper is to ensure that those involved in teaching and learning are aware of the practice, can detect its use and initiate meaningful conversations with students about the perils of using such tools. There is a fine line between use of paraphrasing tools and the use of tools to plagiarise, however it is only through open discussion that students will learn to appreciate the benefits of articulating their understanding in their own words with the appropriate acknowledgement of sources.
Paraphrasing is a skill that transcends an ability to interpret and restate an idea or concept in writing. It is an important skill that needs to be introduced and developed in terms of written, visual and oral forms. The capacity of students and academics to rephrase, frame and restate the ideas and intentions of original authors themselves with appropriate acknowledgements of sources is fundamental to the principles of academic integrity and personal development. The proliferation of fee-based and free Internet-based tools designed to re-engineer text is a concern. Of greater concern is that tools contracted to identify original source materials cannot necessarily be used at this time to identify where writing has been repurposed. Regardless of the ease of access to online text regeneration tools and the work being done to try to electronically detect their use, individuals should be encouraged to improve their own paraphrasing expertise as an essential part of individual skill development in and beyond educational institutions.
Further work is needed to identify linguistic markers indicating use of online paraphrasing tools such as those identified in this study. Academics are already time poor and while they may be strongly in favour of upholding academic standards, they may also be reluctant to undertake time-consuming investigations into possible misconduct. They need encouragement to integrate the observation of textual patterns and markers into their grading and assessment practice. Research is also needed in exploring the most effective techniques or combination of educational, deterrent and punitive techniques and machine detection tools to combat the use of online paraphrasing tools and article spinners and other forms of academic malpractice. Such developments will assist in directing the focus of writing efforts back to where it should be – which is individuals writing and submitting their own work with appropriate acknowledgements.
Ambati V, Vogel S, and Carbonell JG (2010). Active learning and crowd-sourcing for machine translation. Paper presented at the Proceedings of the Seventh International Conference on Language Resources and Evaluation (LREC’10), Malta.
Atkinson D, Lim SL (2013) Improving assessment processes in Higher Education: Student and teacher perceptions of the effectiveness of a rubric embedded in a LMS. Australas J Educ Technol 29(5):651–666
Google Scholar
Baggaley J, Spencer B (2005) The mind of a plagiarist. Learning, Media and Technology 30(1):55–62. doi: 10.1080/13581650500075587
Article Google Scholar
Bailey C, Challen R (2015) Student perceptions of the value of Turnitin text-matching software as a learning tool. Practitioner Research in Higher Education 9(1):38–51
Bodie GD, Cannava KE, Vickery AJ (2016) Supportive communication and the adequate paraphrase. Commun Res Rep 33(2):166–172. doi: 10.1080/08824096.2016.1154839
Bretag T, and Mahmud S (2009a). A model for determining student plagiarism: Electronic detection and academic judgement. Paper presented at the 4APFEI Asia Pacific Conference on Education Integrity APFEI, Wollongong.
Bretag T, Mahmud S (2009b) Self-plagiarism or appropriate textual re-use? J Academic Ethics 7(3):193–205. doi: 10.1007/s10805-009-9092-1
Bretag T, Mahmud S (2016) A conceptual framework for implementing exemplary academic integrity policy in Australian higher education. In: Bretag T (ed) Handbook of Academic Integrity. Springer, Singapore, pp 463–480
Chapter Google Scholar
Burnett AJ, Enyeart Smith TM, Wessel MT (2016) Use of the Social Cognitive Theory to Frame University Students’ Perceptions of Cheating. J Academic Ethics 14(1):49–69. doi: 10.1007/s10805-015-9252-4
Callison-Burch C, Flournoy RS (2001) A program for automatically selecting the best output from multiple machine translation engines, Paper presented at the Proceedings of the Machine Translation Summit VIII
Carroll J, Appleton J (2005). Towards consistent penalty decisions for breaches of academic regulations in one UK university. Int J Educ Integr 1(1):1–11
Carter D, Inkpen D (2012) Searching for poor quality machine translated text : Learning the difference between human writing and machine translations, Paper presented at the Advances in Artificial Intelligence: 25th Canadian Conference on Artificial Intelligence, Canadian AI 2012, 28–30 May 2012., Toronto, Ontario, Canada
Chen J, Kuznetsova P, Warren D, and Choi Y (2015). Déja image-captions: A corpus of expressive descriptions in repetition. Paper presented at the Proceedings of the 2015 Conference of the North American Chapter of the Association for Computational Linguistics: Human Language Technologies.
Chen MH, Huang ST, Chang JS, Liou HC (2015b) Developing a corpus-based paraphrase tool to improve EFL learners’ writing skills. Comput Assist Lang Learn 28(1):22–40. doi: 10.1080/09588221.2013.783873
Clarke R, and Lancaster T (2013). Commercial aspects of contract cheating. Paper presented at the Proceedings of the 18th ACM Conference on Innovation and Technology in Computer Science Education.
Cumming A, Lai C, Cho H (2016) Students’ writing from sources for academic purposes: A synthesis of recent research. J Engl Acad Purp 23:47–58, http://dx.doi.org/10.1016/j.jeap.2016.06.002
Crisp G (2007). Staff attitudes to dealing with plagiarism issues: Perspectives from one Australian university. Int J Educ Integr 3(1):3–15
Dahl S (2007). Turnitin®: The student perspective on using plagiarism detection software. Act Learn Higher
Davis M, Morley J (2015) Phrasal intertextuality: The responses of academics from different disciplines to students’ re-use of phrases. J Second Lang Writ 28:20–35, http://dx.doi.org/10.1016/j.jslw.2015.02.004
Evans C (2013) Making sense of assessment feedback in higher education. Rev Educ Res 83(1):70–120. doi: 10.3102/0034654312474350
Evering LC, Moorman G (2012) Rethinking plagiarism in the digital age. J Adolesc Adult Lit 56(1):35–44. doi: 10.1002/JAAL.00100
Fillenbaum S (1970) A note on the “Search after meaning”: Sensibleness of paraphrases of well formed and malformed expressions. Psychon Sci 18(2):67–68. doi: 10.3758/bf03335699
Granitz N (2007) Applying ethical theories: Interpreting and responding to student plagiarism. J Bus Ethics 72(3):293–306. doi: 10.1007/s10551-006-9171-9
Hirvela A, Du Q (2013) “Why am I paraphrasing?” Undergraduate ESL writers’ engagement with source-based academic writing and reading. J Engl Acad Purp 12(2):87–98, http://dx.doi.org/10.1016/j.jeap.2012.11.005
Howard RM (1995) Plagiarisms, authorships, and the academic death penalty. Coll Engl 57(7):788–806. doi: 10.2307/378403
Inaba R, Murakami Y, Nadamoto A, and Ishida T (2007). Multilingual communication support using the language grid Intercultural Collaboration. Springer, Berlin Heidelberg, p 118–132
Keck C (2006) The use of paraphrase in summary writing: A comparison of L1 and L2 writers. J Second Lang Writ 15(4):261–278
Keck C (2010). How do university students attempt to avoid plagiarism? A grammatical analysis of undergraduate paraphrasing strategies. Writing & Pedagogy 2(2):192-222
Keck C (2014) Copying, paraphrasing, and academic writing development: A re-examination of L1 and L2 summarization practices. J Second Lang Writ 25:4–22, http://dx.doi.org/10.1016/j.jslw.2014.05.005
Kiros R, Zhu Y, Salakhutdinov R, Zemel RS, Torralba A, Urtasun R, Fidler S (2015) Skip-Thought Vectors, Paper presented at the Neural Information Processing Systems 2015, Montreal, Canada
Lancaster T, Clarke R (2009) Automated essay spinning–an initial investigation, Paper presented at the 10 th Annual Conference of the Subject Centre for Information and Computer Sciences
Lee A (2016) Student perspectives on plagiarism. In: Bretag T (ed) Handbook of Academic Integrity. Springer, Singapore, pp 519–535
Li Y, Casanave CP (2012) Two first-year students’ strategies for writing from sources: Patchwriting or plagiarism? J Second Lang Writ 21(2):165–180, http://dx.doi.org/10.1016/j.jslw.2012.03.002
Lopez A (2008) Statistical machine translation. ACM Computing Survey 40(3):1–49. doi: 10.1145/1380584.1380586
Madera Q, García-Valdez M, and Mancilla A (2014). Ad text optimization using interactive evolutionary computation techniques Recent Advances on Hybrid Approaches for Designing Intelligent Systems. Springer, Heidelberg, pp 671–680
McCarthy G (2014). Coaching and mentoring for business: Sage, London
McCarthy G, Rogerson AM (2009) Links are not enough: using originality reports to improve academic standards, compliance and learning outcomes among postgraduate students. Int J Educ Integr 5(2):47–57
Menai MEB (2012) Detection of plagiarism in Arabic documents. Int J Inf Technol Comput Sci 4(10):80–89
Niño A (2009) Machine translation in foreign language learning: language learners’ and tutors’ perceptions of its advantages and disadvantages. ReCALL 21(02):241–258
Patil S, Karekatti T (2015) The use of communication strategies in oral communicative situations by engineering students. Language in India 15(3):214–238
Pfannenstiel AN (2010) Digital literacies and academic integrity. Int J Educ Integr 6(2):41–49
Rabab’ah G (2016) The effect of communication strategy training on the development of EFL learners’ strategic competence and oral communicative ability. J Psycholinguist Res 45(3):625–651. doi: 10.1007/s10936-015-9365-3
Rogerson AM (2014). Detecting the work of essay mills and file swapping sites: some clues they leave behind. Paper presented at the 6th International Integrity and Plagiarism Conference Newcastle-on-Tyne.
Rogerson AM, Bassanta G (2016) Peer-to-peer file sharing and academic integrity in the Internet age. In: Bretag T (ed) Handbook of Academic Integrity. Springer, Singapore, pp 273–285
Roig M (2001) Plagiarism and paraphrasing criteria of college and university professors. Ethics & Behavior 11(3):307–323
Roig M (2016) Recycling our own work in the digital age. In: Bretag T (ed) Handbook of Academic Integrity. Springer, Singapore, pp 655–669
Sambell K, McDowell L, Montgomery C (2013) Assessment for learning in higher education. Routledge, Abingdon, Oxon
Scanlon PM, Neumann DR (2002) Internet plagiarism among college students. J Coll Stud Dev 43(3):374–385
Shi L (2012) Rewriting and paraphrasing source texts in second language writing. J Second Lang Writ 21:134–148. doi: 10.1016/j.jslw.2012.03.003
Socher R, Huang EH, Pennin J, Manning CD, Ng AY (2011) Dynamic pooling and unfolding recursive autoencoders for paraphrase detection, Paper presented at the Advances in Neural Information Processing Systems (NIPS), Granada, Spain
Somers H (2012). Computer-assisted language learning and machine translation. The Encyclopedia of Applied Linguistics. Blackwell Publishing Ltd, Hoboken, p 1-9
Somers H, Gaspari F, Niño A (2006) Detecting inappropriate use of free online machine-translation by language students-A special case of plagiarism detection, Paper presented at the 11th Annual Conference of the European Association for Machine Translation–Proceedings, Oslo, Norway
Spievak ER, Hayes-Bohanan P (2016) Creating order: The role of heuristics in website selection. Internet Reference Services Quarterly 21(1–2):23–46. doi: 10.1080/10875301.2016.1149541
Stamatatos E (2011) Plagiarism and authorship analysis: Introduction to the special issue. Lang Resour Eval 45(1):1–4. doi: 10.1007/s10579-011-9136-1
Suchan J (2014) Toward an understanding of Arabic persuasion: A western perspective. Int J Bus Commun 51(3):279–303. doi: 10.1177/2329488414525401
Sun Y-C (2012) Does text readability matter? A study of paraphrasing and plagiarism in English as a foreign language writing context. Asia-Pacific Education Researcher 21(2):296–306
Thatcher SG (2008) China’s copyright dilemma. Learned Publishing 21(4):278–284
Walker J (1998) Student plagiarism in universities: What are we doing about it? Higher Education Research & Development 17(1):89–106
Warnock S (2006) “Awesome job!”—Or was it? The “many eyes” of asynchronous writing environments and the implications on plagiarism. Plagiary: Cross-Disciplinary Studies in Plagiarism, Fabrication, and Falsification 1:178–190
Word salad (2016). English Oxford Living Dictionaries online. Retrieved from https://en.oxforddictionaries.com/definition/word_salad . Accessed 23 Aug 2016
Download references
Acknowledgments
The authors would like to thank the two anonymous reviewers for their constructive feedback on the original version of this manuscript.
Authors’ contributions
AR 80%. GM 20%. Both authors read and approved the final manuscript.
Competing interests
The authors declare that they have no competing interests.
Author information
Authors and affiliations.
Faculty of Business, University of Wollongong, Building 40, Northfields Avenue, Wollongong, NSW, 2522, Australia
Ann M. Rogerson & Grace McCarthy
You can also search for this author in PubMed Google Scholar
Corresponding author
Correspondence to Ann M. Rogerson .
Rights and permissions
Open Access This article is distributed under the terms of the Creative Commons Attribution 4.0 International License ( http://creativecommons.org/licenses/by/4.0/ ), which permits unrestricted use, distribution, and reproduction in any medium, provided you give appropriate credit to the original author(s) and the source, provide a link to the Creative Commons license, and indicate if changes were made.
Reprints and permissions
About this article
Cite this article.
Rogerson, A.M., McCarthy, G. Using Internet based paraphrasing tools: Original work, patchwriting or facilitated plagiarism?. Int J Educ Integr 13 , 2 (2017). https://doi.org/10.1007/s40979-016-0013-y
Download citation
Received : 09 October 2016
Accepted : 10 December 2016
Published : 26 January 2017
DOI : https://doi.org/10.1007/s40979-016-0013-y
Share this article
Anyone you share the following link with will be able to read this content:
Sorry, a shareable link is not currently available for this article.
Provided by the Springer Nature SharedIt content-sharing initiative
- Paraphrasing
- Internet tools
- Machine translation
- Patchwriting
- Academic integrity
- Paraphrasing tools
International Journal for Educational Integrity
ISSN: 1833-2595
- Submission enquiries: Access here and click Contact Us
- General enquiries: [email protected]
Voice speed
Text translation, source text, translation results, document translation, drag and drop.
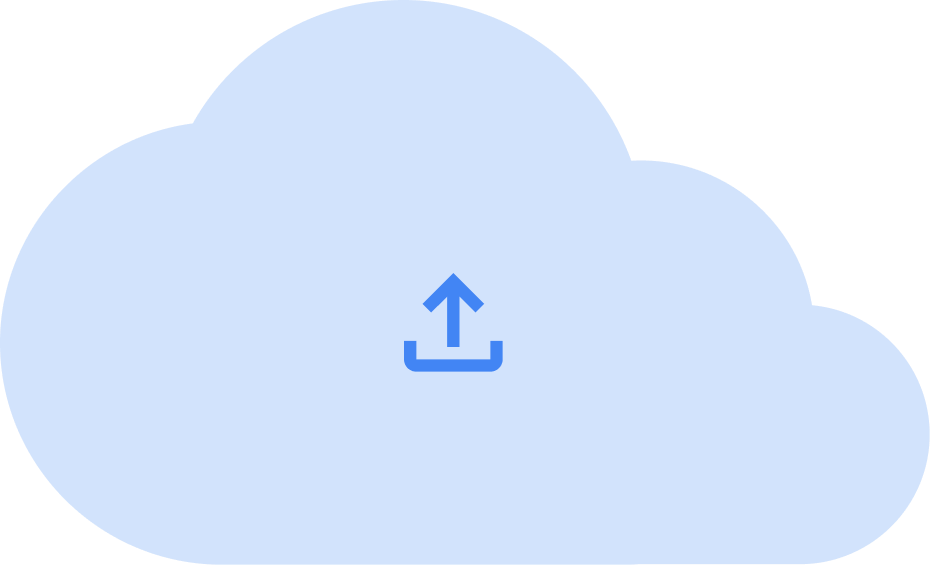
Website translation
Enter a URL
Image translation
The link between translation difficulty and the quality of machine translation: a literature review and empirical investigation
- Original Paper
- Open access
- Published: 10 June 2024
Cite this article
You have full access to this open access article
- Sahar Araghi ORCID: orcid.org/0000-0002-2893-0539 1 &
- Alfons Palangkaraya 1
We survey the relevant literature on translation difficulty and automatic evaluation of machine translation (MT) quality and investigate whether source text’s translation difficulty features contain any information about MT quality. We analyse the 2017–2019 Conferences on Machine Translation (WMT) data of machine translation quality of English news text translated to eleven different languages (Chinese, Czech, Estonian, Finnish, Latvian, Lithuanian, German, Gujarati, Kazakh, Russian, and Turkish). We find (weak) negative correlation between the source text’s length, polysemy and structural complexity and the corresponding human evaluated quality of machine translation. This suggests a potentially important but measureable influence of source text’s translation difficulty on MT quality.
Avoid common mistakes on your manuscript.
1 Introduction
The main objective of this study is to investigate the link between source text’s linguistic features associated with translation difficulty and the quality of machine translation (MT). We first survey the rather separate literature on measures of text-based translation difficulty and automatic evaluation of MT quality. Different methods for automatic evaluation of MT quality have been proposed for a faster, cheaper, and more objective assessment of translation quality compared to manual human evaluation. For example, automatic evaluation can speed up the evaluation of MT systems and the process for identifying problems and weaknesses to improve the MT systems (Comelles & Atserias, 2019 ). However, while most existing automatic evaluation methods use metrics based on similarity measures between the reference and translated text, they ignore the possible variation in the source text in terms of translation difficulty. Unaccounted variation in source text’s translation difficulty could bias automatic evaluation of MT quality.
Specifically, we aim to investigate whether metrics of translation difficulty based on linguistic features can provide additional information to enhance the existing MT algorithms and their automatic quality evaluation methods. There are at least two reasons why MT research and development can benefit from having reliable metrics of source text’s translation difficulty. First, for MT systems development, the information provided by such metrics can be crucial when it comes to comparing the performance of different MT algorithms. If there is a systematic negative correlation between linguistic features of the source text and the quality of MT and if it is not possible to use the same source texts to compare different MT systems, then the MT quality scores from the different text can be adjusted based on the translation difficulty metrics. Second, for users of MT systems, if there is a systematic relationship between source text linguistic features (that is, translation difficulty) and MT quality, the information on “translation difficulty" level would aid in assessing the reliability of the output of the MT systems and deciding on whether a human, professional translator is required.
Surprisingly, while there are many studies which focus on the measurement of either translation difficulty or MT quality, fewer studies exist that consider the potential link between the two. On the one hand, the translation difficulty literature suggests the importance of linguistic features in the process and product of the translation. On the other hand, the literature on automatic quality evaluation of MT has not paid much attention to the importance in the variation of source text translation difficulty level. In fact, in some WMT shared tasks, Footnote 1 the quality of different MT systems may be measured and compared based on different source text with little consideration on how the source text variation would introduce additional variation in the value of the MT performance metric beyond the variation arising from differences in the quality of the MT systems. If a specific source text has a higher translation difficulty level than the other, then we may incorrectly conclude that the machine used to translate it produces more translation errors than the other machine processing a different but easier source text. Adjusting the translation quality metric to take into account the variation in source text’s translation difficulty could help avoid such an incorrect conclusion. In other words, if MT system A is fed with input text set 1 which is more (less) difficult to translate than input text set 2 fed into MT systems B, then the value of the automatic metrics to measure Machine A’s translation quality may need to be adjusted upward (downward) to reflect the fact that it has to process a more difficult set of text.
Furthermore, there is a serious criticism of the existing MT quality evaluation approach because of its reliance on the reference translation text (Lommel, 2016 ). In the real world of translation practice outside of experimental studies, users of MT systems are unlikely to have the reference translation text. Hence, it is often impossible for users to assess the quality of the output of MT systems. To address this criticism, researchers have focused on predicting the quality of MT output under the setting where no reference text is available (Specia et al., 2009 , 2010 ; Almaghout & Specia, 2013 ). Our study is also aimed at contributing to this literature by investigating the potential use of linguistic features of the source text as inputs for MT quality predictive modeling.
Evaluation of the level of translation difficulty of the source text is an important item in translation education, accreditation, research, and language industry. When tasked with translating a relatively easy-to-translate text, human novice and professional translators exhibit fundamentally different cognitive segmentation and speed of translation reflecting the higher capability of the latter. However, such difference disappears when the text to be translated is significantly more difficult, suggesting that even professional translators suffer and are likely to deliver lower quality translation (Dragsted, 2004 , 2005 ). We expect a similar relationship exists between the translation difficulty of the source text and the quality of machine translation.
Without a reliable metric of translation difficulty of the source text, it would be hard to evaluate objectively the quality of different MT systems based on their translation output and the extent of translation errors when the (potential) translation difficulty of the source texts varies. While there are only a few existing studies compared to studies which have attempted at measuring text readability, some researchers in the translation literature have investigated potential metrics for measuring translation difficulty based on the source text. For example, Campbell and Hale ( 1999 , 2003 ) suggested the essential features of the source text such as effective passage length and the time that it takes (a human translator) to complete a translation as potential bases for such metrics since the difficulty in translation can be defined in terms of “the processing effort needed”. Hence, studies such as Jensen ( 2009 ) and Sun and Shreve ( 2014 ) have investigated whether readability metrics can be used to measure translation difficulty given that one of the sources of translation difficulty reflects the reading and reverbalization of the translation process.
In this study, we investigate whether some of the proposed metrics of translation difficulty from the human/professional translation literature can be used to reduce the effect of variation in source text’s translation difficulty on variation in MT systems’ quality. To the best of our knowledge, studying the link between metrics of translation difficulty and MT quality has not been presented in literature before. Specifically, we investigate the relation between certain linguistic features of the source text (which have been identified in the literature on human/professional language translation as related to translation difficulty) and human judgement on the quality of machine translated text. All else equal, as in the case of human translators, we can expect the more difficult the source text for human to translate (as reflected by longer sentence length, greater structural complexity and higher degree of polysemy), the lower the quality of the MT would be. Human judgement based on comparing the MT output with the original text in the source language or with the reference translation in the target language has been used as the main input for evaluating the performance of MT systems in the annual Conference on MT. Footnote 2 In parallel, human judgment has also been used to guide the development of different metrics for automatic evaluation of MT quality. However, to our knowledge, the importance of variation in source text’s translation difficulty has not received much consideration.
Our study is particularly inspired by the work done by Mishra et al. ( 2013 ) on “automatically predicting sentence translation difficulty”. They developed a support vector machine (SVM) to predict the difficulty of a sentence to be translated based on three linguistic features of the sentence: “length”, “structural complexity”, and “average of words polysemy” as inputs. They found a positive correlation between these input features and the time spent for translation. To our knowledge, Mishra et al. ( 2013 ) is the first study which shows an automatic way of linking linguistic features and translation difficulty.
Other related studies on how translation difficulty can be automatically assessed have also paid more attention on the relevant issues on assessment and scoring, such as Williams ( 2004 ), Secară ( 2005 ), and Angelelli ( 2009 ). However, none of these provide as clear and systematic relationships between linguistic features and translation difficulty. For example, Williams ( 2004 ) provides a comprehensive discussion on different aspects of translation quality assessment and why they are important. Secară ( 2005 ) discusses various frameworks used in the process of translation evaluation in the translation industry and teaching institutions, with a special focus on error classification schemes such as wrong term, misspelling, omission, and some other features. Finally, Angelelli ( 2009 ) suggests potential features to look at: 1. Grammar and Mechanics (target language); 2. Style and Cohesion (purpose); 3. Situational Appropriateness (includes audience, purpose, and domain terminology); 4. Source Text Meaning; 5. Translation Skill (evidence of effective use of resource materials). However, the study seeks to measure “translation ability” rather than translation quality, with aims to measure whether the translator has properly understood audience and purpose.
From the machine translation based studies, Costa et al. ( 2015 ) show errors associated with structural complexity and polysemy as the most pervasive types of errors in MT of English-Portuguese texts in their sample. This finding is consistent with Mishra et al. ( 2013 )’s findings. Altogether, these and the studies we discussed earlier serve as another reason why in this study we propose to use the features and metrics of Mishra et al. ( 2013 ). Specifically, we implement the approach of Mishra et al. ( 2013 ) to measure three linguistic properties associated with translation difficulty of the source texts (length (L), degree of polysemy (DP) and structural complexity (SC)) on all English source texts used in the annual Conference on Machine Translation (WMT) over 2017–2019 (WMT 2017, WMT 2018, and WMT 2019). Footnote 3 We then compute the pairwise Pearson correlation coefficients of these measures and human judgement scores of the MT quality of the translation of the English source text to eleven different languages (Chinese, Czech, Estonian, Finnish, Latvian, Lithuanian, German, Gujarati, Kazakh, Russian, and Turkish). Our analysis shows mostly statistically significant (weak) negative correlations between our proxies of translation difficulty and the quality of MT systems.
The rest of the paper is structured as follows. In Sect. 2 we review the literature on translation difficulty measure and automatic evaluation of MT. In Sect. 3 we discuss the data we use for our analysis and the approach for analysing the relationship between linguistic features of the source text associated with translation difficulty and the human evaluated quality of MT systems. In Sect. 4 we present and discuss the results. Finally, in Sect. 5 , we provide some concluding remarks.
2 Related background
2.1 measuring translation difficulty.
In this section we review the literature on translation difficulty. Most studies on the relationship between source text’s translation difficulty and the quality of translation are based analyses of the translation process and product associated with human translators. However, as discussed later, recent studies have begun to explore the relationship between source text’s translation difficulty and MT errors. For our purpose, we will focus on Mishra et al. ( 2013 )’s translation difficulty index (TDI) and how it can be useful in machine translation evaluation. However, it is plausible that some other translation difficulty metrics that have been developed by the literature are also relevant for the evaluation of machine translation quality.
For the case of human translators, to measure translation difficulty, there are four measurement items that can be considered (Akbari & Segers, 2017 ): (1) the identification of sources of translation difficulty, (2) the measurement of text readability, (3) the measurement of translation difficulty by means of translation evaluation products such as holistic, analytic, calibrated dichotomous items, and the preselected items evaluation methods, and (4) the measurement of mental workload. Studies such as Campbell and Hale ( 1999 ) have investigated whether certain essential linguistic features related to the source text reflect “the processing effort needed" or mental workload involved in translating a text. If these features such as the effective passage length and required time for translation serve as potential sources of translation difficulty, they can be used for constructing translation difficulty metrics. Specifically, the study proposed a metric based on cognitive effort required under time constraint to gauge source text difficulty “by identifying those lexical items that require higher amounts of cognitive processing". However, the study admitted that such difficulty criterion may not be necessarily related to the idea of translation correctness, which is the focus of translation quality studies. In other words, it is possible for different translators to mistranslate a segment of text in a similar way that requires little cognitive effort. Hence, the cognitive approach suggested may fail to identify the real difficulty of the text.
In a subsequent study, Hale and Campbell ( 2002 ) investigated the importance of the relationship between source text linguistic features such as official terms, complex noun phrases, passive verbs, and metaphors and the accuracy of the translated text. However, they concluded that there is no clear correlation between these source text features and accuracy of the translation.
Jensen ( 2009 ) investigated whether some of the standard readability indices of relative differences in the complexity of a text (such as word frequency and non-literalness) can be used as predictors of translation difficulty. Unfortunately, while the study explored the potential and weakness of different readability indicators for predicting text difficulty, it is not comprehensive or systematic enough to reach any conclusive finding.
A more recent study, Sun and Shreve ( 2014 ), investigated in a more systematic way how readability is related to translation difficulty using experimental data from a sample of 49 third-year undergraduate students in translation/English and 53 first-year graduate students in translation. All the students in the experiment spoke Mandarin Chinese as their first language and started learning English as a foreign language from the 6th grade. These students were assigned the tasks of translating short English texts (about 121 to 134 words) to Chinese. The Flesch Reading Ease (FRE) formula, one of the most popular and influential readability formulas (DuBay, 2004 ), was used for scoring the readability of the source English texts to be translated and classify them into three categories: easy (FRE scores of 70–80), medium (scores of 46–55), and difficult (FRE scores of 20–30). The study found a weak, negative correlation between readability and translation quality score (obtained by averaging human evaluation scores from three independent graders all of whom had translation teaching experience and had translated at least two books). The study computed an R-square of 0.015, which means the variation in translation quality score could only account for 1.5% of the variation in the translation difficulty scores of the source text. Furthermore, the study found translation quality level as evaluated by better translators (defined as students with higher grades) is not consistently different from the translation quality evaluated by the worse translators (lower grade students). Finally, the study found the time spent on translating the source text as statistically significant positively (but weakly) correlated with the translation difficulty level.
In another related study, Sun ( 2015 ) provided a theoretical and methodological overview of translation difficulty and emphasized that an accurate assessment of the level of translation difficulty of a given source text is a critical factor to consider in translator training, accreditation and related research. Traditionally, people rely on their general impression from reading the source text to gauge its translation difficulty level. However, for a more effective evaluation process, Sun ( 2015 ) argued that a more objective and systematic instrument is required. For that purpose, there are two basic questions to answer: what to measure and how to measure it. The potential sources of translation difficulty can be classified into the translation factors and the translator factors. Accordingly, to measure translation difficulty, we need to be able to measure the source text difficulty, identify the translation-specific difficulty (e.g., non-equivalence, one-to-several equivalence, and one-to-part equivalence situations as mentioned by Baker ( 2011 )), and assess the cognitive factors associated with the translation difficulty (such as the mental workload for the translator). The study mentioned that readability formulas are most often used to measure text difficulty and suggested that, for identifying translation-specific difficulty, grading translations, analysing verbal protocols, and recording and analysing translation behaviour are also required.
Howard ( 2016 ) argued that although short-passage test scores are frequently used for translator certification, we know little about how the text’s features and the test scores are linked to the objective or the purpose of the test. He analysed the text features associated with translation difficulty in Japanese-to-English short-passage translation test scores. The analysis revealed that, first, it is possible to link specific passage features such as implicit or explicit cohesion markers to a desired trait of a good translation such as the creation of a coherent target text. Second, there are elements in the text features that could signal coherence and be objectively scored as acceptable or unacceptable to be used for calculating facility values (percentage of correct responses) in the test population. Finally, these facility values can be used to create a profile of comparative passage difficulty and to quantitatively identify items of difficulty within the passage.
Wu ( 2019 ) explored the relationship between text characteristics, perceived difficulty and task performance in sight translation. In the study, twenty-nine undergraduate interpreters were asked to sight-translate six texts with different properties. Correlation analysis showed the students’ fluency and accuracy in performing the tasks as related to sophisticated word type, mean length of T-units, and the lexical and syntactic variables in the source texts.
Eye tracking is a process-based alternative to outcome-based measures such as translation test scores for measuring the effect of translation difficulty. In this case translation difficulty is inferred from an analysis of the translator’s attention (as reflected by their eyes and gaze) on both the source and target texts during actual translation process. The analysis assumes that when “the eye remains fixated on a word as long as the word is being processed" it reflects difficulty (Just & Carpenter, 1980 ). Thus, this approach rests on the theory that “the more complex texts require readers to make more regressions in order to grasp the meaning and produce a translation" (Sharmin et al., 2008 ).
Mishra et al. ( 2013 ) developed a translation difficulty index (TDI) based on a theory that “difficulty in translation stems from the fact that most words are polysemous and sentences can be long and have complex structure". They illustrated this by comparing two simple sentences of eight words: (i) “The camera-man shot the policeman with a gun.” and (ii) “I was returning from my old office yesterday.” According to them, the first sentence is more difficult to process and translate because of the lexical ambiguity of the word “shoot” which may represent taking a picture or firing a shot and the structural ambiguity (policeman with a gun or shot with a gun). They argued that to obtain fluent and adequate translations requires the ability of the translator to analyse both the lexical and syntactic properties of the source text. They then proceeded to construct the TDI measure based on the eye tracking cognitive data, defining the TDI value as the sum of the fixation (gaze) time and saccade (rapid eye movement) time. In addition, they measured three linguistic features of the source text: (i) length (L), defined as the total number of words occurring in a sentence (L), (ii) degree of polysemy (DP), defined as the sum of senses possessed by each word in the Wordnet (Miller, 1995 ) normalized by the sentence length, and (iii) structural complexity (SC), defined as the total length of dependency links in the dependency structure of the sentence (Lin, 1996 ). The idea behind SC is that words, phrases, and clauses are syntactically attached to each other in a sentence, and the sentence has higher structural complexity if these units lie far from each other. For example, as shown in Fig. 1 , the structural complexity of the sentence “The man who the boy attacked escaped." is 1+5+4+3+1+1=15. They showed that the TDI and text linguistic measures (L, DP, and SC) are positively correlated, confirming the hypothesis that linguistic features can serve as indicators of translation difficulty.
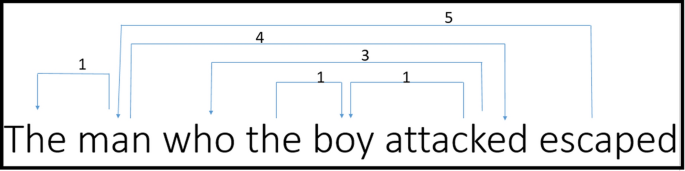
Dependency graph
Interestingly, the finding that the degree of polysemy (DP) positively correlates with translation difficulty (Mishra et al., 2013 ) appears to be consistent with the findings of a study from a separate machine translation study that analysed the different types of MT errors (Costa et al., 2015 ). In that study, as many as seventeen machine translation error types are identified including orthography errors such as those from misspelling, lexical errors such as those from omission, grammatical errors such as incorrect ordering, discourse style errors such as variety, and semantic errors such as confusion of senses. The study found, based on a sample of 750 sentence pairs English-Portuguese translations, lexical, grammatical and semantic groups of errors are the most pervasive. Among each individual error type, the error from confusion of senses is one of the two most pervasive ones. The other most pervasive error type is misselection, from the grammatical group of errors, which is most closely related to source text’s structural complexity.
Furthermore, the findings from the following two studies are particularly relevant for our analysis because they help us understand why a metric based on sense ambiguity in the source text could indicate the degree of translation difficulty. First, Raganato et al. ( 2019 )’s work focuses on word sense ambiguity. They presented MUCOW, a multilingual contrastive test suite that covers 16 language pairs with more than 200,000 contrastive sentence pairs, automatically built from word-aligned parallel corpora and the wide-coverage multilingual sense inventory of BabelNet. They then evaluated the quality of the ambiguity lexicons and of the resulting test suite on all submissions from nine language pairs presented in the WMT19 news shared translation task, plus on other five language pairs using pretrained NMT models. They used the proposed benchmark to assess the word sense disambiguation ability of neural machine translation systems. Their finding shows the state-of-the-art and fine-tuned neural machine translation systems still present some drawbacks on handling ambiguous words, especially when evaluated on out-of-domain data and when the encoder has to deal with a morphologically rich language.
Second, Popović ( 2021 ) carried out an extensive analysis of MT errors observed and highlighted by different human evaluators according to different quality criteria. Her analysis includes three language pairs, two domains and eleven NMT systems. The main findings of the work show that most perceived errors are caused by rephrasing, ambiguous words, noun phrases and mistranslations. Other important sources of errors include untranslated words and omissions.
2.2 Automatic machine translation evaluation
The measurement of translation quality, specifically when it comes to MT, is one of the highly active areas in translation research. The use of automatic evaluation translation metrics has distinctly accelerated the development cycle of MT systems. Currently, one of the most widely used metrics for automated translation evaluation is BLEU, a string-matching metric based on the idea that “the closer a MT is to a professional human translation, the better it is" (Papineni et al., 2002 ). However, several problems have been detected in translation evaluation based on BLEU. Callison-Burch et al. ( 2006 ) and Koehn and Monz ( 2006 ) discussed possible disagreements between automatic system evaluation rankings produced by BLEU and those of human assessors. They argued BLEU may not be reliable when the systems under evaluation are different in nature, such as rule-based systems and statistical systems or human-aided and fully automatic systems. Reiter ( 2018 ) reviewed 34 papers and, based on the reported 284 correlation coefficients between human score of MT quality and BLEU metrics, concluded that overall the evidence supports the use of BLEU as a diagnostic evaluation metrics for MT systems. However, they also concluded that the evidence does not support the use of BLEU for outside of MT evaluation such as individual texts evaluation or scientific hypothesis testing.
Essentially, the automated evaluation metrics for MT such as BLEU and other earlier introduced metrics discussed below are all based on the concept of lexical similarity of the reference translation text and the MT systems’ output. These metrics assign higher translation quality scores to machine translated text having higher lexical similarity to the reference, human translated, text. The most basic lexical similarity metrics include metrics based on edit distances such as PER (Tillmann et al., 1997 ), WER (Nießen et al., 2000 ), and TER (Snover et al., 2006 ). More sophisticated metrics which are based on lexical precision but without consideration of any linguistic information include BLEU and NIST (Doddington, 2002 ). Both metrics and others such as ROUGE (Lin & Och, 2004 ) and CDER (Leusch et al., 2006 ) are based on lexical recall. Metrics that consider a balance between precision and recall include GTM (Melamed et al., 2003 ), METEOR (Banerjee & Lavie, 2005 ), BLANC (Lita et al., 2005 ), SIA (Liu & Gildea, 2006 ), and MAXSIM (Chan & Ng, 2008 ). Lexical information such as synonyms, stemming, and paraphrasing for evaluation are considered by the following metrics: METEOR, M-BLEU and M-TER (Agarwal & Lavie, 2008 ), TERp (Snover et al., 2009 ), SPEDE (Wang & Manning, 2012 ), and MPEDA (Zhang et al., 2016 ). Popović ( 2015 ) proposed the use of character n-gram F-score for automatic evaluation of machine translation output. Wang et al. ( 2016 ) proposed translation edit rate on character level (CharacTER), which calculates the character level edit distance while performing the shift edit on word level.
Although lexical based measures appear to generally perform well over a variety of translation quality evaluation metrics, there is a broad criticism against their use for such purpose (see, for examples, Coughlin ( 2003 ) and Culy and Riehemann ( 2003 )). The main argument against lexical measures is that they are more for document similarity measures rather than translation quality measures. Hence, the suggested improvements include the use of models of language variability by comparing the syntactic and semantic structure of candidate and reference translations. Liu and Gildea ( 2005 ), for example, proposed different syntactic measures based on comparing head-word dependency chains and constituent subtrees. Popović and Ney ( 2007 ) introduced several measures based on edit distance over parts of speech. Owczarzak et al. ( 2007 ) proposed measure based on comparing dependency structures from a probabilistic lexical-functional grammar parser. Mehay and Brew ( 2006 ) developed a measure based on combinatorial categorical grammar parsing without the need to parse the possible ill-formed automatic candidate translations, just parsing the reference translations. Kahn et al. ( 2009 ) used a probabilistic context-free grammar parser and deterministic head-finding rules. Other proposed measures are based on morphological information like suffixes, roots, and prefixes. Among them we can mention AMBER (Chen et al., 2012 ) and INFER ((Popović et al., 2012 ). There are also similar measures based on syntax such as part of speech tags, constituents and dependency relation information about morphology such as HWCM (Liu & Gildea, 2005 ) and UOWREVAL (Gupta et al., 2015 ) or on applying semantic information such as SAGAN-STS (Castillo & Estrella, 2012 ), MEANT (Lo & Yu, 2013 ), MEANT 2.0 (Lo, 2017 ).
Combining different evaluation methods using machine learning has also been proposed to improve automatic MT quality evaluation. The focus of such solution is on evaluating the well-formedness of automatic translations. For example, Corston-Oliver et al. ( 2001 ) applied decision trees to distinguish human-generated translations from machine-generated ones. In their study, from each sentence, they extracted 46 features by performing a syntactic parse using the Microsoft NLPWin natural language processing system (Heidorn, 2000 ) and language modeling tools. Another example, Akiba et al. ( 2001 ) proposed a ranking method by using multiple edit distances to encode machine translated sentences with a human-assigned rank into multi-dimensional vectors from which a classifier of ranks is learned in the form of a decision tree. On the other hand, Kulesza and Shieber ( 2004 ) used support vector machines and features inspired by BLEU, NIST, WER, and PER.
Gamon et al. ( 2005 ) presented a support vector classifier for identifying highly dysfluent and ill-formed sentences. Similar to Akiba et al. ( 2001 ), the classifier uses linguistic features obtained by using French NLPWin analysis system (Heidorn, 2000 ). The machine learning model is trained on the extracted features from machine translated and human translated sentences. Quirk ( 2004 ) and Quirk et al. ( 2005 ) suggested the use of a variety of supervised machine learning algorithms such as perceptron, support vector machines, decision trees, and linear regression on a rich collection of features extracted by their developed system.
Ye et al. ( 2007 ) also considered MT evaluation as a ranking problem. They applied a ranking support vector machine algorithm to sort candidate translations based on several features extracted from three categories: n-gram-based, dependency-based, and translation perplexity according to a reference language model. The approach showed higher correlation with human assessment at the sentence level, even when they use an n-gram match score as a baseline feature. Some other studies using machine learning techniques to combine different types of MT metrics include Yang et al. ( 2011 ), Gautam and Bhattacharyya ( 2014 ), Yu et al. ( 2015 ), and Ma et al. ( 2017 ).
Some researchers also applied neural based machine learning models in their works such as Thompson and Post ( 2020 ) who frame the task of machine translation evaluation as one of scoring machine translation output with a sequence-to-sequence paraphraser, conditioned on a human reference. They propose training the paraphraser as a multilingual NMT system, treating paraphrasing as a zero-shot translation task (e.g., Czech to Czech). This results in the paraphraser’s output mode being centred around a copy of the input sequence, which represents the best case scenario where the MT system output matches a human reference. As another example, Rei et al. ( 2020 ) present COMET, a neural framework for training multilingual machine translation evaluation models. Their framework leverages cross-lingual pretrained language modelling resulting in multilingual and adaptable MT evaluation models that exploit information from both the source input and a target-language reference translation in order to more accurately predict MT quality.
Giménez and Màrquez ( 2010 ) argued that using only a limited number of linguistic features could lead to bias in the development cycle which can cause negative consequences to MT quality. They introduced an automatic MT quality evaluation metric based on a rich set of specialized similarity measures operating at different linguistic dimensions. The approach can both analyse individual and collective behaviour over a wide range of evaluation scenarios. However, instead of using machine learning techniques, their proposed method is based on uniformly averaged linear combinations of measures (ULC). That is, the combined score from various metrics is the normalised arithmetic mean of individual measures:
where M is the measure set, and m ( t , R ) is the normalised similarity between the automatic translation t and the set of references R , for the given test case, according to the measure m . Normalised scores are computed by dividing the maximum score attained over the set of all cases by actual scores.
Based on their implementation results, presented as an online tool called Asiya (Gimenez & Marquez, 2010 ), Giménez and Màrquez ( 2010 ) concluded that measures based on syntactic and semantic information can provide a more reliable metric for MT system ranking than lexical measures, especially when the systems under evaluation are based on different paradigms. They further showed that certain linguistic measures perform better than most lexical measures at the sentence level, some others perform worse when there are parsing problems. However, they argued that combining different measures is still suitable and can yield a substantially improved evaluation quality metric.
Comelles and Atserias ( 2019 ) introduced VERTa, a MT evaluation metric based on linguistic information inspired by Giménez and Màrquez ( 2010 )’s approach, except that they used correlation with human judgements and different datasets to find the best combination of linguistic features. Comelles and Atserias ( 2019 ) argued that VERTa checks for the suitability of the linguistic features selected and how they should interact to better measure adequacy and fluency in English. In essence, VERTa is a modular model which includes lexical, morphological, dependency, n-gram, semantic, language model modules. VERTa uses the Fmean to combine Precision and Recall measures. If there is more than one reference text, the maximum Fmean among all references is returned as the score. When the scores per module are calculated the final score is a weighted average of the different scores (Fmean) of the modules.
Most recently, some researchers have applied neural based machine learning models in their works such as Thompson and Post ( 2020 ) who framed the task of machine translation evaluation as one of scoring machine translation output with a sequence-to-sequence paraphraser, conditioned on a human reference. They proposed training the paraphraser as a multilingual NMT system, treating paraphrasing as a zero-shot translation task (e.g., Czech to Czech). This results in the paraphraser’s output mode being centred around a copy of the input sequence, which represents the best-case scenario where the MT system output matches a human reference. As another example, Rei et al. ( 2020 ) presented COMET, a neural framework for training multilingual machine translation evaluation models. Their framework leverages cross-lingual pretrained language modelling resulting in multilingual and adaptable MT evaluation models that exploit information from both the source input and a target-language reference translation in order to more accurately predict MT quality.
3 Data and approach
The data for our empirical analysis come from the publicly available data used in the series of workshops on machine translation (WMT), an annual international workshop on various topics related to machine translation and the automatic evaluation of MT quality going back to 2006. Specifically, we used data on human judgement scores of MT outputs of a given set of English source text translated into eleven different languages (Chinese, Czech, Estonian, Finnish, German, Gujarati, Kazakh, Latvian, Lithuanian, Russian, and Turkish). We focused our analysis on translation of English as the source language due to the need to identify linguistic features using readily available and most developed tools. We use NLTK Natural Language Toolkit (Loper & Bird, 2002 ) and Stanford natural language processing parser named CoreNLP (Manning et al., 2014 ) to extract the linguistic features from these English source texts. We selected the sample period of 2017, 2018, and 2019 workshop years to ensure that we can use the absolute human quality scores, instead of the relative scores provided in earlier years. Because different workshop years cover different sets of language pairs, our estimating sample is an unbalanced panel of 11 different target languages over three years. For the 2017 workshop, our sample contains target languages of Chinese, Czech, Latvian, Finnish, German, Russian, and Turkish. The 2018 data contain all previous year’s target languages except Latvian, which was replaced with Estonian. The 2019 data add Lithuanian, Gujarati and Kazakh.
3.2 Approach
Our analytical approach is based on correlation analysis between translation difficulty level of the source English text and the quality of MT systems’ output in each of the eleven target languages. We used WMT’s human evaluator scoring data as the metrics for MT quality. For the translation difficulty metrics of the source English text, we considered a similar set of linguistic features in Mishra et al. ( 2013 ):
Length The number of words in the sentence.
Polysemy Sum of senses based on Wordnet for non-stop words in a sentence.
Structural complexity Total length of dependency links in the dependency structure of the sentence.
In the paper, the authors showed the positive correlation between L, DP, SC and the time spent on the translation that is known as an important item in measuring translation difficulties studies. In our implementation, from each sentence in the set of English source texts, we constructed measures of the following linguistic features (using the same measure definition as in Mishra et al. ( 2013 )) and computed their correlation coefficients with MT quality scores. The computation is done separately for each of the ten available target languages.
4 Results and discussion
Table 1 provides summary statistics (sample mean and standard deviation) of the human judgement of MT quality scores and each of the three linguistic features. In WMT2019, the source English texts were the same for all target languages. Hence, the linguistic features are identical. In WMT2017 and 2018, the sets of source English text vary due to the use of both genuine English source text and English source text which were translated text from the target language.
To ensure that we use the evaluation scores that reflect the difficulty of the translation of the source English text, we restrict our sample as follows. First, we only consider source sentences which have been translated by more than one machine. This is to ensure that our analysis does not capture machine variation instead of source text variation. Second, we exclude evaluated translation scores marked as either “REF", “BAD-REF", and “REPEAT" because they are inserted quality control pairs instead of true machine translation output. For example, “BAD-REF" indicates the use of damaged MT outputs to detect if the human judge is as expected in terms of assigning significantly worse scores. The data associated with missing translation scores are also removed. In addition, because of structural complexity checking, we just keep the data that have just one sentence. Because of this restriction, the sample size in Table 1 differs from the original sample size in the WMT Submitted Data. Last, but not least, to take into account the fact that the WMT2017 and 2018 samples used both genuine English pre-translated target sentence into English, we analyse both the full sample and the subsample which only includes genuine English source test.
First, Table 1 shows a significant variation in the translation quality (Q) and, for 2017 and 2018, in the linguistic features (L, DP and SC) across language pairs within the same study year. For example, in 2017 data, the lowest MT quality is observed for EN-TR pair (32.7), whereas the highest is observed for EN-ZH at 65.9 (or around 100% higher). However, because each set of EN-XX language pair in that year may contain different English source text (as confirmed by the variation in each linguistic feature measure), we are not sure whether the variation in MT quality is purely due to variation in the quality of MT algorithm across target language or it is also due to variation in source text translation difficulty. For the case of 2019, however, all language pairs use the same source English text. Hence, there is no variation in linguistic feature across language pairs and the variation in translation quality is likely due to cross-target language variation in MT algorithm and training data.
We also see from Table 1 a significant variation across the years in terms of translation quality. For example, in 2017 data, the highest level in terms of length, polysemy, and structural complexity is approximately 25%, 28%, and 35% higher than the lowest levels in order. The degree of variation for 2018 is slightly lower, but it is not trivial either. Unconditional comparison of translation quality of the same language pairs across the years may be confounded by cross-year variation in linguistic feature. However, as shown in Table 1 , the higher translation quality (Q) in 2019 compared to the other two years seems to indicate a genuine increase in MT quality since most of the linguistic features that represent translation difficulty in 2019 are at least as high as those in the earlier years.
Table 2 presents the correlation coefficient between average translation quality scores from all MT systems’ output for each sentence in the language pair and each linguistic feature of the source English text in each data year. As expected, with a few exceptions particularly for the EN-ZH pair, linguistic features associated with higher translation difficulty are negatively correlated with translation quality. Most of the correlation coefficients are significantly different from zero with a p-value of less than one per cent. Ignoring the non-statistically significant coefficients and the positive correlation coefficients displayed by EN-ZH data in 2017 and 2018 (separately discussed in a subsequent paragraph), the strength of the negative correlation between translation quality and linguistic feature ranges from \(-\) 0.07 (EN-FI 2017; L) to \(-\) 0.32 (EN-LT 2019; DP).
Comparing the correlation coefficient across different linguistic features within each language pair each year presented in Table 2 , polysemy appeared to be most strongly correlated with translation quality. Excluding all non-statistically significant coefficients and the coefficients for EN-ZH and EN-RU for 2017 and 2018, the average correlation coefficients for L, DP and SC are, respectively, \(-\) 0.186, \(-\) 0.215 and \(-\) 0.156. The evidence that polysemy presents the most important translation difficulty to MT systems appears to be consistent with the works of Costa et al. ( 2015 ) and Popović ( 2021 ) we summarised earlier, who suggested the presence of ambiguous words as a potentially important source of translation errors.
Table 2 also shows a significant variation in the link between translation difficulty linguistic features and MT quality, particularly for 2017 and particularly as shown by the case of the EN-ZH pair. We consider several plausible reasons behind such variation: (1) as shown in Table 1 , variation in the linguistic features of the source text; (2) variation in the quality and sensitivity of MT systems Footnote 4 ; and (3) variation in the quality of human assessment. Without a more extensive data analysis, possibly in a controlled experimental setting, it is difficult to identify which of these reasons is the most important. However, Barrault et al. ( 2019 ) highlighted a significant problem in the quality of Mechanical Turk workers used for the 2017 EN-RU and EN-ZH arising from higher rates of gaming. For example, only eight out of the original 43 workers were retained for providing the “good" human judgement data we analyse. In their accompanying data notes, Bojar et al. ( 2017 ) suggested a minimum of 15 human assessments to obtain an accurate sentence-level score. In 2018, the gaming problem for both language pairs was still the worst among all other pairs, but it was not as bad as in 2017. Only in 2019 data the extent of gaming for EN-RU and EN-ZH pairs appeared to be comparable to the rest of language pairs (Barrault et al., 2019 ). Therefore, we believe the positive correlations are anomalies, indicative of problems of the human judgement data.
Furthermore, the most consistent negative correlation across the three-year data is exhibited by the 2019 data. This is possibly due to the use of genuine English source text in 2019 as opposed to mixed genuine and ‘translationese’ (pre-translated target sentence into English) sentences (Barrault et al., 2019 ; Bojar et al., 2017 ). Graham et al. ( 2019 ), as cited in (Barrault et al., 2019 ), argued that the inclusion of test data (that is, pre-translated target sentence as the source sentence) could introduce inaccuracies in the evaluation of MT quality. We believe the pre-translated text may also affect our computed linguistic features and the human judgement score. To verify this, we redo the correlation analysis for the 2017 and 2018 data by excluding source sentences whose original language is not English. The results, summarised in Table 3 , show that indeed the statistically significant and positive correlation coefficients discussed above were driven by the ‘translationese’. In other words, the use of ‘translationese’ may also affect the accuracy of the relationship between the linguistic measures of translation difficulty and the quality of MT.
5 Conclusions
The quality of translation of a text depends on the capability of the translator, human or machine, and the level of difficulty of the translated source text. One may argue that the link between translation difficulty of the source text and human translation quality may be weak because, provided with enough time a human translator may always be able to deliver a high level of translation quality. However, there is virtually no “translation time" parameter for a MT system since MT output is delivered instantaneously. In other words, variation in source text translation difficulty level is much more likely to be directly reflected by the translation quality produced by a MT system than a human translator.
Surprisingly, when we surveyed the existing studies on linguistic measures of translation difficulty and the quality of MT, we did not find many articles which cover both topics. In the MT literature, most attention has been focused on developing evaluation metrics for translation quality based on test case comparisons of machine translated text and the “reference” translation. This focus is reasonable given the main objective is to improve upon the algorithms used behind the MT systems. However, a more comprehensive understanding on the determinants of translation quality is potentially valuable to introduce refinements to existing algorithms to reduce the most pervasive error types in MT systems related to grammar and, particularly, word confusion.
Hence, in this paper, in addition to providing a survey of the relevant literature, we aimed at contributing to such understanding by empirically investigating the relationship between measurable linguistic features that reflect the translation difficulty of the source and the quality of MT. Specifically, we constructed measures of translation difficulty that have been shown to be correlated with cognitive based measures that capture the extent of efforts required by human translators to complete a given translation task in an experimental setting or, in other words, the extent of translation difficulty. These measures include the length of the sentence, the degree of polysemy and the sentence’s structural complexity. We found mostly negative correlation between each of these translation difficulty measures and MT quality as assessed by human judges/evaluators for the full sample of English to other language pair tests in WMT2017, WMT2018 and WMT2019. This finding is consistent with the existing evidence that MT systems tend to suffer mostly from making the type of errors associated with grammar and sense confusion.
In summary, the results of our analsys suggest that there are measurable linguistic features that can be used to measure translation difficulty and maybe even predict translation quality. Thus, the ability to measure translation difficulty is important for, for example, normalising source text when comparison of translation quality across different translators and different level of source text difficulty is required. Furthermore, we found anomalies in the relationship between translation difficulty and MT quality which upon our closer inspection appeared to be caused by inaccurate human judgement data. In other words, the linguistic features we evaluated might potentially be used also to identify if there is any gaming problem in human judgement of MT quality. Finally, the fact that we found a systematic relationship between translation quality and translation difficulty in terms of word senses suggests a high potential reward from developing better sense disambiguation algorithm in MT systems.
Finally, there are several areas where our analysis could be fruitfully extended, two of which we discuss below. First, while our evidence suggests a negative relationship between translation difficulty features of the source text and the quality of machine translation, the negative correlation is weak. One possible reason for this is because different linguistic features of the source text are likely to be associated with different types of translations errors. However, the human judges’ scores of the MT quality in the WMT data may reflect various types of translation errors. Thus, a further analysis on the type of errors by distinguishing between the errors associated with accuracy (such as mistranslation) and fluency (incorrect word order) as examined by, for example, Carl and Băez ( 2019 ), would likely provide a better understanding of the relationship between source text translation difficulty features and MT quality. Second, the information about translation difficulty could be useful for automatic evaluation metrics, so one direction for future work is to investigate incorporating these linguistic features into existing metrics and/or develop new ones.”. We leave these for future research.
See https://machinetranslate.org/wmt or https://www.statmt.org .
For more details, see the following web sites: https://machinetranslate.org/wmt or https://www.statmt.org .
See Bojar et al. (2017, 2018) and Barrault et al. (2019) and the web pages from where all the data we use can be downloaded: http://www.statmt.org/wmt17/results.html , http://www.statmt.org/wmt18/results.html , and http://www.statmt.org/wmt19/index.html (Accessed: 2019-09-19).
What we meant by the term “sensitivity" is that different MT algorithms may have different sensitivity to each of the three linguistic features. Thus, it is plausible that one machine shows a consistently higher translation quality (compared to other machines) when fed with a “standard" source text, but significantly lower translation quality when fed with text with a very high degree of polysemy.
Agarwal, A. & Lavie, A. (2008). Meteor, m-bleu and m-ter: Flexible matching and parameter tuning for high-correlation with human judgments of machine translation quality. In Proceedings of the ACL2008 workshop on statistical machine translation. Columbus, Ohio, USA .
Akbari, A., & Segers, W. (2017). Translation difficulty: How to measure and what to measure. Lebende Sprachen, 62 (1), 3–29.
Article Google Scholar
Akiba, Y., Imamura, K., & Sumita, E. (2001). Using multiple edit distances to automatically rank machine translation output. In Proceedings of the MT summit VIII , pp. 15–20.
Almaghout, H. & Specia, L. (2013). A ccg-based quality estimation metric for statistical machine translation. Proceedings of MT summit XIV (to appear), Nice, France .
Angelelli, C. V. (2009). Using a rubric to assess translation ability. Testing and assessment in translation and interpreting studies. John Benjamins: Philadelphia , pp. 13–47.
Baker, C. (2011). Foundations of bilingual education and bilingualism . Multilingual matters.
Banerjee, S. & Lavie, A. (2005). Meteor: An automatic metric for mt evaluation with improved correlation with human judgments. In Proceedings of the acl workshop on intrinsic and extrinsic evaluation measures for machine translation and/or summarization , pp. 65–72.
Barrault, L., Bojar, O., Costa-jussà, M. R., Federmann, C., Fishel, M., Graham, Y., Haddow, B., Huck, M., Koehn, P., Malmasi, S., et al. (2019). Findings of the 2019 conference on machine translation (wmt19). In Proceedings of the fourth conference on machine translation (volume 2: shared task papers, Day 1) , pp. 1–61.
Bojar, O., Rajen, C., Federmann, C., Graham, Y., Haddow, B., Huck, M., Koehn, P., Liu, Q., Logacheva, V., Monz, C., et al. (2017). Findings of the 2017 conference on machine translation (wmt17). In Second conference on machine translation , pp. 169–214. The Association for Computational Linguistics.
Callison-Burch, C., Osborne, M., & Koehn, P. (2006). Re-evaluation the role of bleu in machine translation research. In 11th conference of the European Chapter of the Association for Computational Linguistics .
Campbell, S. & Hale, S. (1999). What makes a text difficult to translate. In Proceedings of the 1998 ALAA Congress , volume 19.
Campbell, S. & Hale, S. (2003). Translation and interpreting assessment in the context of educational measurement. Translation today: Trends and perspectives , pp. 205–224.
Carl, M., & Băez, T. (2019). Machine translation errors and the translation process: A study across different languages. The Journal of Specialised Translation, 31 , 107–132.
Google Scholar
Castillo, J. & Estrella, P. (2012). Semantic textual similarity for mt evaluation. In Proceedings of the seventh workshop on statistical machine translation , pp. 52–58.
Chan, Y. S. & Ng, H. T. (2008). Maxsim: A maximum similarity metric for machine translation evaluation. In Proceedings of ACL-08: HLT , pp. 55–62.
Chen, B., Kuhn, R., & Foster, G. (2012). Improving amber, an mt evaluation metric. In Proceedings of the seventh workshop on statistical machine translation , pp. 59–63. Association for Computational Linguistics.
Comelles, E., & Atserias, J. (2019). Verta: A linguistic approach to automatic machine translation evaluation. Language Resources and Evaluation, 53 (1), 57–86.
Corston-Oliver, S., Gamon, M., & Brockett, C. (2001). A machine learning approach to the automatic evaluation of machine translation. In Proceedings of the 39th annual meeting on Association for Computational Linguistics , pp. 148–155. Association for Computational Linguistics.
Costa, Â., Ling, W., Luís, T., Correia, R., & Coheur, L. (2015). A linguistically motivated taxonomy for machine translation error analysis. Machine Translation, 29 (2), 127–161.
Coughlin, D. (2003). Correlating automated and human assessments of machine translation quality. In Proceedings of MT summit IX , pp. 63–70.
Culy, C. & Riehemann, S. Z. (2003). The limits of n-gram translation evaluation metrics. In MT Summit IX , pp. 71–78.
Doddington, G. (2002). Automatic evaluation of machine translation quality using n-gram co-occurrence statistics. In Proceedings of the second international conference on Human Language Technology Research , pp. 138–145. Morgan Kaufmann Publishers Inc.
Dragsted, B. (2004). Segmentation in translation and translation memory systems: An empirical investigation of cognitive segmentation and effects of integrating a TM system into the translation process . Opstilling: 813 dra Løbe nr.: 051172 Generelle noter: På omslaget: Ph.d.-afhandling af Barbara Dragsted.
Dragsted, B. (2005). Segmentation in translation: Differences across levels of expertise and difficulty. Target. International Journal of Translation Studies, 17 (1), 49–70.
DuBay, W. H. (2004). The principles of readability. Online Submission .
Gamon, M., Aue, A., & Smets, M. (2005). Sentence-level mt evaluation without reference translations: Beyond language modeling. In Proceedings of EAMT , pp. 103–111.
Gautam, S. & Bhattacharyya, P. (2014). Layered: Metric for machine translation evaluation. In Proceedings of the ninth workshop on statistical machine translation , pp. 387–393.
Gimenez, J., & Marquez, L. (2010). Asiya: An open toolkit for automatic machine translation (meta-) evaluation. The Prague Bulletin of Mathematical Linguistics, 94 , 77–86.
Giménez, J., & Màrquez, L. (2010). Linguistic measures for automatic machine translation evaluation. Machine Translation, 24 (3–4), 209–240.
Graham, Y., Haddow, B., & Koehn, P. (2019). Translationese in machine translation evaluation. arXiv preprint arXiv:1906.09833 .
Gupta, R., Orasan, C., & van Genabith, J. (2015). Reval: A simple and effective machine translation evaluation metric based on recurrent neural networks. In Proceedings of the 2015 conference on empirical methods in natural language processing , pp. 1066–1072.
Hale, S., & Campbell, S. (2002). The interaction between text difficulty and translation accuracy. Babel, 48 (1), 14–33.
Heidorn, G. (2000). Intelligent writing assistance. Handbook of natural language processing , pp. 181–207.
Howard, D. L. (2016). A quantitative study of translation difficulty based on an analysis of text features in Japanese-to-English short-passage translation tests . PhD thesis, Universitat Rovira i Virgili.
Jensen, K. T. (2009). Indicators of text complexity. Mees, IM; F. Alves & S. Göpferich (eds.) , pp. 61–80.
Just, M. A., & Carpenter, P. A. (1980). A theory of reading: From eye fixations to comprehension. Psychological Review, 87 (4), 329.
Kahn, J. G., Snover, M., & Ostendorf, M. (2009). Expected dependency pair match: Predicting translation quality with expected syntactic structure. Machine Translation, 23 (2–3), 169–179.
Koehn, P. & Monz, C. (2006). Manual and automatic evaluation of machine translation between european languages. In Proceedings on the workshop on statistical machine translation , pp. 102–121.
Kulesza, A. & Shieber, S. (2004). A learning approach to improving sentence-level mt evaluation. In Proceedings of the 10th international conference on theoretical and methodological issues in machine translation . European Association for Machine Translation.
Leusch, G., Ueffing, N., & Ney, H. (2006). Cder: Efficient mt evaluation using block movements. In 11th conference of the European Chapter of the Association for Computational Linguistics .
Lin, C.-Y. & Och, F. J. (2004). Automatic evaluation of machine translation quality using longest common subsequence and skip-bigram statistics. In Proceedings of the 42nd annual meeting on Association for Computational Linguistics , page 605. Association for Computational Linguistics.
Lin, D. (1996). On the structural complexity of natural language sentences. In COLING 1996 Volume 2: The 16th international conference on computational linguistics .
Lita, L. V., Rogati, M., & Lavie, A. (2005). Blanc: Learning evaluation metrics for mt. In Proceedings of the conference on human language technology and empirical methods in natural language processing , pp. 740–747. Association for Computational Linguistics.
Liu, D., & Gildea, D. (2005). Syntactic features for evaluation of machine translation. In Proceedings of the ACL workshop on intrinsic and extrinsic evaluation measures for machine translation and/or summarization , pp. 25–32.
Liu, D. & Gildea, D. (2006). Stochastic iterative alignment for machine translation evaluation. In Proceedings of the COLING/ACL on main conference poster sessions , pp. 539–546. Association for Computational Linguistics.
Lo, C.-k. (2017). Meant 2.0: Accurate semantic mt evaluation for any output language. In Proceedings of the second conference on machine translation , pp. 589–597.
Lo, C.-k. & Wu, D. (2013). Meant at wmt 2013: A tunable, accurate yet inexpensive semantic frame based mt evaluation metric. In Proceedings of the eighth workshop on statistical machine translation , pp. 422–428.
Lommel, A. (2016). Blues for bleu: Reconsidering the validity of reference-based mt evaluation. In Proceedings of the LREC 2016 workshop “translation evaluation–from fragmented tools and data sets to an integrated ecosystem”, Portoroz, Slovenia , pp. 63–70.
Loper, E., & Bird, S. (2002). Nltk: The natural language toolkit. In Proceedings of the ACL workshop on effective tools and methodologies for teaching natural language processing and computational linguistics. Philadelphia: Association for Computational Linguistics .
Ma, Q., Graham, Y., Wang, S., & Liu, Q. (2017). Blend: a novel combined mt metric based on direct assessment- casict-dcu submission to wmt17 metrics task. In Proceedings of the second conference on machine translation , pp. 598–603.
Manning, C. D., Surdeanu, M., Bauer, J., Finkel, J., Bethard, S. J., & McClosky, D. (2014). The Stanford CoreNLP natural language processing toolkit. In Association for Computational Linguistics (ACL) system demonstrations , pp. 55–60.
Mehay, D. N. & Brew, C. (2006). Bleu atre: Flattening syntactic dependencies for mt evaluation. In Proceedings of MT summit , volume 12, pp. 122–131. Citeseer.
Melamed, I. D., Green, R., & Turian, J. P. (2003). Precision and recall of machine translation. In Companion volume of the proceedings of HLT-NAACL 2003-short papers , pp. 61–63.
Miller, G. A. (1995). Wordnet: A lexical database for English. Communications of the ACM, 38 , 39–41.
Mishra, A., Bhattacharyya, P., & Carl, M. (2013). Automatically predicting sentence translation difficulty. In Proceedings of the 51st annual meeting of the Association for Computational Linguistics (Volume 2: short papers) , pp. 346–351.
Nießen, S., Och, F. J., Leusch, G., Ney, H., et al. (2000). An evaluation tool for machine translation: Fast evaluation for mt research. In LREC .
Owczarzak, K., Van Genabith, J., & Way, A. (2007). Dependency-based automatic evaluation for machine translation. In Proceedings of the NAACL-HLT 2007/AMTA workshop on syntax and structure in statistical translation , pp. 80–87. Association for Computational Linguistics.
Papineni, K., Roukos, S., Ward, T., & Zhu, W.-J. (2002). Bleu: a method for automatic evaluation of machine translation. In Proceedings of the 40th annual meeting on association for computational linguistics , pp. 311–318. Association for Computational Linguistics.
Popović, M. (2012). Class error rates for evaluation of machine translation output. In Proceedings of the seventh workshop on statistical machine translation , pp. 71–75. Association for Computational Linguistics.
Popović, M. (2015). chrF: character n-gram F-score for automatic MT evaluation. In Proceedings of the Tenth Workshop on Statistical Machine Translation , pp. 392–395, Lisbon, Portugal. Association for Computational Linguistics.
Popović, M. (2021). On nature and causes of observed MT errors. In Proceedings of machine translation summit XVIII: Research track , pp. 163–175. Association for Machine Translation in the Americas.
Popović, M., & Ney, H. (2007). Word error rates: Decomposition over pos classes and applications for error analysis. In Proceedings of the second workshop on statistical machine translation , pp. 48–55. Association for Computational Linguistics.
Quirk, C. (2004). Training a sentence-level machine translation confidence measure . Citeseer: In LREC.
Quirk, C., Menezes, A., & Cherry, C. (2005). Dependency treelet translation: Syntactically informed phrasal smt. In Proceedings of the 43rd annual meeting on Association for Computational Linguistics , pp. 271–279. Association for Computational Linguistics.
Raganato, A., Scherrer, Y., Tiedemann, J., et al. (2019). The mucow test suite at wmt 2019: Automatically harvested multilingual contrastive word sense disambiguation test sets for machine translation. In Fourth conference on machine translation proceedings of the conference (volume 2: shared task papers, day 1) . The Association for Computational Linguistics.
Rei, R., Stewart, C., Farinha, A. C., & Lavie, A. (2020). COMET: A neural framework for MT evaluation. In Proceedings of the 2020 conference on empirical methods in natural language processing (EMNLP) , pp. 2685–2702, Online. Association for Computational Linguistics.
Reiter, E. (2018). A structured review of the validity of bleu. Computational Linguistics, 44 (3), 393–401.
Secară, A. (2005). Translation evaluation: A state of the art survey. In Proceedings of the eCoLoRe/MeLLANGE workshop, Leeds , pp. 39–44.
Sharmin, S., Spakov, O., Räihä, K.-J., & Jakobsen, A. L. (2008). Where on the screen do translation students look while translating, and for how long? Copenhagen Studies in Language, 36 , 31–51.
Snover, M., Dorr, B., Schwartz, R., Micciulla, L., & Makhoul, J. (2006). A study of translation edit rate with targeted human annotation. In Proceedings of association for machine translation in the Americas , volume 200.
Snover, M., Madnani, N., Dorr, B. J., & Schwartz, R. (2009). Fluency, adequacy, or hter? Exploring different human judgments with a tunable mt metric. In Proceedings of the fourth workshop on statistical machine translation , pp. 259–268. Association for Computational Linguistics.
Specia, L., Raj, D., & Turchi, M. (2010). Machine translation evaluation versus quality estimation. Machine Translation, 24 (1), 39–50.
Specia, L., Turchi, M., Cancedda, N., Dymetman, M., & Cristianini, N. (2009). Estimating the sentence-level quality of machine translation systems. In 13th conference of the European Association for Machine Translation , pp. 28–37.
Sun, S. (2015). Measuring translation difficulty: Theoretical and methodological considerations. Across Languages and Cultures, 16 (1), 29–54.
Sun, S., & Shreve, G. M. (2014). Measuring translation difficulty: An empirical study. Target. International Journal of Translation Studies, 26 (1), 98–127.
Thompson, B. & Post, M. (2020). Automatic machine translation evaluation in many languages via zero-shot paraphrasing. In Proceedings of the 2020 conference on Empirical Methods in Natural Language Processing (EMNLP) , pp. 90–121, Online. Association for Computational Linguistics.
Tillmann, C., Vogel, S., Ney, H., Zubiaga, A., & Sawaf, H. (1997). Accelerated dp based search for statistical translation. In Fifth European conference on speech communication and technology .
Wang, M. & Manning, C. D. (2012). Spede: Probabilistic edit distance metrics for mt evaluation. In Proceedings of the seventh workshop on statistical machine translation , pp. 76–83. Association for Computational Linguistics.
Wang, W., Peter, J.-T., Rosendahl, H., & Ney, H. (2016). CharacTer: Translation edit rate on character level. In Proceedings of the first conference on machine translation: Volume 2, shared task papers , pp. 505–510, Berlin, Germany. Association for Computational Linguistics.
Williams, M. (2004). Translation quality assessment: An argumentation-centred approach . University of Ottawa Press.
Wu, Z. (2019). Text characteristics, perceived difficulty and task performance in sight translation: An exploratory study of university-level students. Interpreting, 21 (2), 196–219.
Yang, M.-Y., Sun, S.-Q., Zhu, J.-G., Li, S., Zhao, T.-J., & Zhu, X.-N. (2011). Improvement of machine translation evaluation by simple linguistically motivated features. Journal of Computer Science and Technology, 26 (1), 57–67.
Ye, Y., Zhou, M., & Lin, C.-Y. (2007). Sentence level machine translation evaluation as a ranking problem: one step aside from bleu. In Proceedings of the second workshop on statistical machine translation , pap. 240–247. Association for Computational Linguistics.
Yu, H., Ma, Q., Wu, X., & Liu, Q. (2015). Casict-dcu participation in wmt2015 metrics task. In Proceedings of the tenth workshop on statistical machine translation , pp. 417–421.
Zhang, L., Weng, Z., Xiao, W., Wan, J., Chen, Z., Tan, Y., Li, M., & Wang, M. (2016). Extract domain-specific paraphrase from monolingual corpus for automatic evaluation of machine translation. In Proceedings of the first conference on machine translation: Volume 2, shared task papers , pp. 511–517.
Download references
Open Access funding enabled and organized by CAUL and its Member Institutions.
Author information
Authors and affiliations.
Centre for Transformative Innovation, Swinburne University of Technology, Melbourne, Australia
Sahar Araghi & Alfons Palangkaraya
You can also search for this author in PubMed Google Scholar
Corresponding author
Correspondence to Sahar Araghi .
Ethics declarations
Conflict of interest.
Not applicable.
Additional information
Publisher's note.
Springer Nature remains neutral with regard to jurisdictional claims in published maps and institutional affiliations.
Rights and permissions
Open Access This article is licensed under a Creative Commons Attribution 4.0 International License, which permits use, sharing, adaptation, distribution and reproduction in any medium or format, as long as you give appropriate credit to the original author(s) and the source, provide a link to the Creative Commons licence, and indicate if changes were made. The images or other third party material in this article are included in the article's Creative Commons licence, unless indicated otherwise in a credit line to the material. If material is not included in the article's Creative Commons licence and your intended use is not permitted by statutory regulation or exceeds the permitted use, you will need to obtain permission directly from the copyright holder. To view a copy of this licence, visit http://creativecommons.org/licenses/by/4.0/ .
Reprints and permissions
About this article
Araghi, S., Palangkaraya, A. The link between translation difficulty and the quality of machine translation: a literature review and empirical investigation. Lang Resources & Evaluation (2024). https://doi.org/10.1007/s10579-024-09735-x
Download citation
Accepted : 15 March 2024
Published : 10 June 2024
DOI : https://doi.org/10.1007/s10579-024-09735-x
Share this article
Anyone you share the following link with will be able to read this content:
Sorry, a shareable link is not currently available for this article.
Provided by the Springer Nature SharedIt content-sharing initiative
- Machine translation
- Human translation
- Translation difficulty
- Automatic machine translation evaluation
- Find a journal
- Publish with us
- Track your research
Help | Advanced Search
Computer Science > Computation and Language
Title: lcs: a language converter strategy for zero-shot neural machine translation.
Abstract: Multilingual neural machine translation models generally distinguish translation directions by the language tag (LT) in front of the source or target sentences. However, current LT strategies cannot indicate the desired target language as expected on zero-shot translation, i.e., the off-target issue. Our analysis reveals that the indication of the target language is sensitive to the placement of the target LT. For example, when placing the target LT on the decoder side, the indication would rapidly degrade along with decoding steps, while placing the target LT on the encoder side would lead to copying or paraphrasing the source input. To address the above issues, we propose a simple yet effective strategy named Language Converter Strategy (LCS). By introducing the target language embedding into the top encoder layers, LCS mitigates confusion in the encoder and ensures stable language indication for the decoder. Experimental results on MultiUN, TED, and OPUS-100 datasets demonstrate that LCS could significantly mitigate the off-target issue, with language accuracy up to 95.28%, 96.21%, and 85.35% meanwhile outperforming the vanilla LT strategy by 3.07, 3,3, and 7.93 BLEU scores on zero-shot translation, respectively.
Comments: | ACL2024 Findings, Codes are at |
Subjects: | Computation and Language (cs.CL); Artificial Intelligence (cs.AI) |
Cite as: | [cs.CL] |
(or [cs.CL] for this version) | |
Focus to learn more arXiv-issued DOI via DataCite |
Submission history
Access paper:.
- HTML (experimental)
- Other Formats
References & Citations
- Google Scholar
- Semantic Scholar
BibTeX formatted citation

Bibliographic and Citation Tools
Code, data and media associated with this article, recommenders and search tools.
- Institution
arXivLabs: experimental projects with community collaborators
arXivLabs is a framework that allows collaborators to develop and share new arXiv features directly on our website.
Both individuals and organizations that work with arXivLabs have embraced and accepted our values of openness, community, excellence, and user data privacy. arXiv is committed to these values and only works with partners that adhere to them.
Have an idea for a project that will add value for arXiv's community? Learn more about arXivLabs .
P ara Z h-22 M : A Large-Scale C hinese Parabank via Machine Translation
Wenjie Hao , Hongfei Xu , Deyi Xiong , Hongying Zan , Lingling Mu
Export citation
- Preformatted
Markdown (Informal)
[ParaZh-22M: A Large-Scale Chinese Parabank via Machine Translation](https://aclanthology.org/2022.coling-1.341) (Hao et al., COLING 2022)
- ParaZh-22M: A Large-Scale Chinese Parabank via Machine Translation (Hao et al., COLING 2022)
- Wenjie Hao, Hongfei Xu, Deyi Xiong, Hongying Zan, and Lingling Mu. 2022. ParaZh-22M: A Large-Scale Chinese Parabank via Machine Translation . In Proceedings of the 29th International Conference on Computational Linguistics , pages 3885–3897, Gyeongju, Republic of Korea. International Committee on Computational Linguistics.
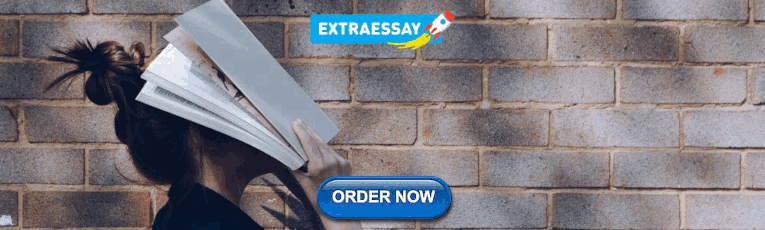
COMMENTS
QuillBot's Paraphraser is fast, free, and easy to use, making it the best paraphrasing tool on the market. You can compare results from 9 predefined modes and use the remarkable Custom mode to define and create an unlimited number of Custom modes. The built-in thesaurus helps you customize your paraphrases, and the rephrase option means you can ...
DeepL uses advanced AI to provide high-quality, context-aware paraphrasing in English and German. Our tool intelligently restructures and rephrases text, preserving the original meaning and enhancing your writing. 2. How do you use DeepL's paraphrasing tool? To accomplish writing tasks, you can: - Paste your existing text into the tool.
Paraphrasing involves expressing someone else's ideas or thoughts in your own words while maintaining the original meaning. Paraphrasing tools can help you quickly reword text by replacing certain words with synonyms or restructuring sentences. They can also make your text more concise, clear, and suitable for a specific audience.
Ahrefs' Paraphrasing Tool uses a language model that learns patterns, grammar, and vocabulary from large amounts of text data - then uses that knowledge to generate human-like text based on a given prompt or input. The generated text combines both the model's learned information and its understanding of the input.
What you can do with QuillBot's online translator. Translate longer texts. Use a translator without ads. Translate text in 45 languages. Edit text and cite sources at the same time with integrated writing tools. Enjoy completely free translation. Use the power of AI to translate text quickly and accurately. Translate online—without ...
Cite (ACL): Jonathan Mallinson, Rico Sennrich, and Mirella Lapata. 2017. Paraphrasing Revisited with Neural Machine Translation. In Proceedings of the 15th Conference of the European Chapter of the Association for Computational Linguistics: Volume 1, Long Papers, pages 881-893, Valencia, Spain. Association for Computational Linguistics.
of paraphrase pairs in 23 different languages. In this paper we revisit the bilingual pivoting approach from the perspective of neural machine translation, a new approach to machine transla-tion based purely on neural networks (Kalchbren-ner and Blunsom, 2013; Bahdanau et al., 2014; Sutskever et al., 2014; Luong et al., 2015). At
The proposed method offers merits over machine-translation-based paraphrase generation methods, as it avoids reliance on bilingual sentence pairs. It also allows human intervene with the model so that more diverse paraphrases can be generated using different filtering criteria. Extensive experiments on existing paraphrase dataset for both the ...
We apply statistical machine translation (SMT) tools to generate novel paraphrases of input sentences in the same language. ... Human evaluation shows that this system outperforms baseline paraphrase generation techniques and, in a departure from previous work, offers better coverage and scalability than the current best-of-breed paraphrasing ...
Naturally existing paraphrase corpora are hard to come by, in contrast to machine translation, where naturally found parallel data in the form of translated articles, books, and presentations are widely available online . The majority of methods for paraphrasing, such as bilingual pivoting, are translation-based.
ParaBank: Monolingual Bitext Generation and Sentential Paraphrasing via Lexically-constrained Neural Machine Translation. Abstract: We present ParaBank, a large-scale English paraphrase dataset that surpasses prior work in both quantity and quality. Following the approach of ParaNMT, we train a Czech-English neural machine translation (NMT ...
The paraphrasing and rescoring improve the translation produced by neural machine translation from 12.03% to 12.92%. Idioms are rich multi-word expressions that can be found in many works of literature. The meaning of most idioms cannot be deduced literally. This makes translating idioms challenging. Moreover, the parallel text that contains idioms is limited. As a result, machine translation ...
Human evaluation shows that this SMT system outperforms baseline paraphrase generation techniques and, in a departure from previous work, offers better coverage and scalability than the current best-of-breed paraphrasing approaches. We apply statistical machine translation (SMT) tools to generate novel paraphrases of input sentences in the same language. The system is trained on large volumes ...
The proposed method offers merits over machine-translation-based paraphrase generation methods, as it avoids reliance on bilingual sentence pairs. It also allows human intervene with the model so that more diverse paraphrases can be generated using different filtering criteria. Extensive experiments on existing paraphrase dataset for both the ...
Inspired by unsupervised machine translation (UMT) models, which align semantic spaces of two languages us-ing monolingual data, we propose a pipeline system to generate paraphrases following two stages: (1) splitting a large-scale monolingual corpus into mul-tiple clusters/sub-datasets, on which UMT models are trained based on pairs of these ...
Internet-based paraphrasing tools are text processing applications and associated with the same approaches used for machine translation (MT). While MT usually focusses on the translation of one language to another, the broader consideration of text processing can operate between or within language corpuses (Ambati et al. 2010 ).
Google's service, offered free of charge, instantly translates words, phrases, and web pages between English and over 100 other languages.
Abstract Recent work has shown that a multilingual neural machine translation (NMT) model can be used to judge how well a sentence paraphrases another sentence in the same language (Thompson and Post, 2020); however, attempting to generate paraphrases from such a model using standard beam search produces trivial copies or near copies.
We present ParaBank, a large-scale English paraphrase dataset that surpasses prior work in both quantity and quality. Following the approach of ParaNMT, we train a Czech-English neural machine translation (NMT) system to generate novel paraphrases of English reference sentences. By adding lexical constraints to the NMT decoding procedure, however, we are able to produce multiple high-quality ...
We analyse the 2017-2019 Conferences on Machine Translation (WMT) data of machine translation quality of English news text translated to eleven different languages (Chinese, Czech, Estonian, Finnish, Latvian, Lithuanian, German, Gujarati, Kazakh, Russian, and Turkish). ... treating paraphrasing as a zero-shot translation task (e.g., Czech to ...
With QuillBot's Tagalog to English translator, you are able to translate text with the click of a button. Our translator works instantly, providing quick and accurate outputs. User-friendly interface. Our translator is easy to use. Just type or paste text into the left box, click "Translate," and let QuillBot do the rest. Text-to-speech feature.
%0 Conference Proceedings %T Automatic Machine Translation Evaluation in Many Languages via Zero-Shot Paraphrasing %A Thompson, Brian %A Post, Matt %Y Webber, Bonnie %Y Cohn, Trevor %Y He, Yulan %Y Liu, Yang %S Proceedings of the 2020 Conference on Empirical Methods in Natural Language Processing (EMNLP) %D 2020 %8 November %I Association for Computational Linguistics %C Online %F thompson ...
We frame the task of machine translation evaluation as one of scoring machine translation output with a sequence-to-sequence paraphraser, conditioned on a human reference. We propose training the paraphraser as a multilingual NMT system, treating paraphrasing as a zero-shot translation task (e.g., Czech to Czech). This results in the paraphraser's output mode being centered around a copy of ...
Multilingual neural machine translation models generally distinguish translation directions by the language tag (LT) in front of the source or target sentences. However, current LT strategies cannot indicate the desired target language as expected on zero-shot translation, i.e., the off-target issue. Our analysis reveals that the indication of the target language is sensitive to the placement ...
In our data augmentation experiments, we show that paraphrasing based on ParaZh-22M can bring about consistent and significant improvements over several strong baselines on a wide range of Chinese NLP tasks, including a number of Chinese natural language understanding benchmarks (CLUE) and low-resource machine translation. Anthology ID: