17 Longitudinal Study Advantages and Disadvantages
Longitudinal studies are a research design which requires repeated observations of the same variables over specific time periods. These may be shorter examinations or designed to collect long-term data. Under most situations, it is treated as a type of observational study, although there are times when researchers can structure them as more of a randomized experiment.
Most longitudinal studies are used in either clinical psychology or social-personality observations. They are useful when observing the rapid fluctuations of emotion, thoughts, or behaviors between two specific baseline points. Some researchers use them to study life events, compare generational behaviors, or review developmental trends across individual lifetimes.
When they are observational, then longitudinal studies are able to observe the world without manipulating it in any way. That means they may have less power to detect casual relationships that may form in their observed subjects. Because there are repeated observations performed at the individual level with this option, there is also more power than other studies to remove time-invariant differences while review the temporal order of events that occur.
The longest-running longitudinal study in the world today was started in 1921 by psychologist Lewis Terman. He wanted to investigate how highly intelligent children would develop as they turned into adults. The original study had over 1,000 participants, but that figure has dropped to under 200. Researchers plan to continue their work until there are no participants left.
These are the crucial longitudinal studies pros and cons to review before setting up this form of a panel study.
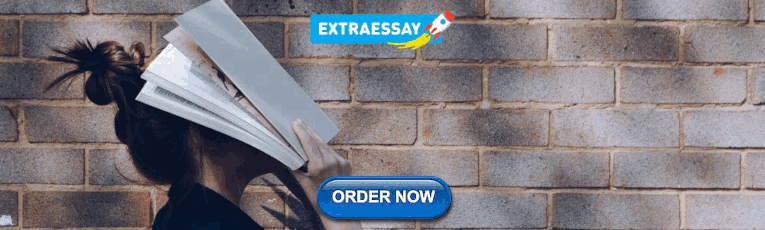
List of the Pros of Longitudinal Studies
1. This form of research is designed to be more flexible than other options. There are times when a longitudinal study will look at one specific data point only when researchers begin observing their subjects. You will also find that this option provides enough data when implemented to provide information on unanticipated relationships or patterns that may be meaningful in specific environments. Since most of these studies are not designed to be lengthy, there are more options to pursue tangents here than in other research formats.
Researchers have an opportunity to pursue additional data points which were collected to determine if a shift in focus is necessary to review a complete set of information. If there is something interesting found in the material, then longitudinal studies allow for an option to pursue them.
2. The accuracy rate of the data collected during longitudinal studies is high. When researchers decide to follow longitudinal studies to collect observational data, then the accurate rate of the information they collect is high because everything occurs in a real-time situation. Although mistakes do happen because no one is perfect, the structure and foundation of this option limits the problems that can occur. This information is also useful in the implementation of changes that may be necessary to achieve the best possible outcome during an observational period.’
3. This research method can identify unique developmental trends. When researchers pursue a short-term longitudinal study, then they are looking for answers to very specific questions. If a long-term model is developed, there is an opportunity to identify specific developmental trends that occur in various fields, including sociology, psychology, and general medicine.
Researchers using longitudinal studies have opportunities to track multiple generations in specific family groups while still collecting real-time data on all of the individuals being tracked to see how current decisions can influence future outcomes for some population demographics.
4. It allows for the consistent use of the observational method. It is a simpler process to collect information when using longitudinal studies for research because it almost always uses the observational method. This structure makes it possible to collect consistent data samples at the individual level instead of relying on extrapolation or other methods of personal identification. It is the consistency offered in this approach which provides for exclusion differences for individuals, making it possible to exclude variations that could adversely impact outcomes as it happens with other research options.
5. Longitudinal studies allow for unique a specific data points to be collected. Most research study options provide a structure where data is available over a short time period for collection, offering a small window where cause-and-effect examples can be observed. Longitudinal studies provide an option to increase the amount of time provided for researchers to collect their data, sometimes on a very dramatic scale. There are some studies which are measured in decades or centuries instead of days, weeks, or months. This process makes it possible to examine the macro- and micro-changes that can occur in the various fields of humanity.
6. This process allows for higher levels of research validity. For any research project to be successful, there are laws, regulations, and rules that must be instituted from the very beginning to ensure all researchers follow the same path of data collection. This structure makes it possible of multiple people to collect similar information from unique individuals because everyone is following the same set of processes. It creates a result that offers higher levels of validity because it is a simpler process to verify the data that is being developed from the direct observations of others.
7. There are three different types of longitudinal studies available for use. Researchers have access to three significant types of longitudinal studies to collect the information that they require. Panel studies are the first option, and they involve a sampling of a cross-section of individuals. Cohort studies are the second type, which involves the selection of a group based on specific events, such as their historical experience, household location, or place of birth.
The final option is called a retrospective study. This option looks at the past by reviewing historical information, such as medical records, to determine if there is a pattern in the data that is useful.
List of the Cons of Longitudinal Studies
1. The structure makes it possible for one person to change everything. Longitudinal studies have a robust reliance on the individual interpretations that researchers develop after making their observations. That makes it possible for personal bias, inexperience, or a mistake to inadvertently alter the data being collected in real-time situations. This issue makes it possible for the information to be invalid without researchers realizing that this disadvantage is present in their work. Even if there are numerous people involved with a project, it is possible for a single person to disrupt potentially decades of work because of their incorrect (and possibly inadvertent) approach.
2. It is more expensive to perform longitudinal studies than other research methods. This disadvantage typically applies to the research studies which are designed to take longer periods of time to collect relevant information. Because observations may last for several years (if not decades), the organizations which are behind the effort of information retention can discover that their costs can be up to 50% higher in some situations when they choose this method over the other options that are available. Although the value of the research remains high, some may find the cost to be a significant barrier to cross.
3. The information collected by researchers may have few controls. The real-time observational data that researchers collect during longitudinal studies is both informative and efficient from a cost perspective when looking at short-term situations. One of the problems that this method encounters is that the information being collected comes from a relatively small number of individuals. Unless it is built into the rules for collection, there may be no controls in place for environmental factors or cultural differences between the individuals involved.
4. It can be challenging for longitudinal research to adapt to changes. There is sometimes no follow up to identify changes in thinking or operations that occur when using longitudinal studies as the primary basis of information collection. Researchers sometimes fail to compare attitudes, behaviors, or perceptions from one point of time to another. Most people change as time passes because they have more information available to them upon which they can draw an opinion. Some people can be very different today than they were 10 years ago. Unless the structures are flexible enough to recognize and adapt to this situation, then the data they gather may not be as useful as it should be.
5. Longitudinal studies often require a larger sample size. Researchers use longitudinal studies to develop a recognition for patterns and relationships. That means there is a large amount of data that must be collected from numerous individual sources to draw meaningful connections to the topic under study. If there is not a significant sample size available to researchers for the project, then there may not be enough information available to find specific conclusions.
Even when there is enough data present for researchers to use, the sheer size of what they collect can require data mining efforts that can take time to sort out.
6. Some people do not authentically participate in longitudinal studies. As with any other form of research that is performed today, you will encounter individuals who behave artificially because they know they are part of a longitudinal study program. When this issue occurs, then it becomes challenging for researchers to sort out what the authentic and inauthentic emotions, thoughts, and behaviors are from each other. Some participants may try to behave in the ways that they believe the researchers want to create specific results.
A study by psychologist Robert S. Feldmen and conducted by the University of Massachusetts found that 60% of people lie at least once during a 10-minute conversation. The average person will lie 2-3 times during that discussion. The content of fibs varies between men and women, trying to make themselves look better or to make the person they are talking to feel good respectively. Researchers must recognize this trait early to remove this potential disadvantage.
7. Longitudinal studies rely on the skill set of the researchers. The data that longitudinal studies collects is presented in real-time to researchers, which means it relies on their individual skills to make it useful. Those who are tasked with this job must follow a specific set of steps to ensure that there is authenticity and value to what they observe. Even if you offer step-by-step guidelines on how to perform the work, two different researchers may interpret the instructions differently, which can then lead to an adverse result. The personal views of the information being collected can also impact the results in ways that are not useful.
8. The data that is collected from longitudinal studies may not be reliable. Although the goal of longitudinal studies is to identify patterns, inaccuracies in the information collected can lead to incorrect interpretations of choices, thoughts, and behaviors. All it takes is one piece of data to be inaccurate for the results to be impacted in negative ways. It is possible that the findings of the research could be invalidated by just one incorrect interpretation of a real-time result. That is why any conclusion made using this method is often taken with a “grain of salt” with regard to its viability.
9. There is a time element to consider with longitudinal studies. Researchers may find that it requires several years of direct observation before any meaningful data becomes available through longitudinal studies. Some relationships or observable behaviors may never occur even though it seems like they should, which means this time investment may never offer dividends. These studies must have the means to maintain continuously open lines of communication with all of the involved parties to ensure that the quality of the data remains high throughout the entire effort.
10. Longitudinal studies always offer a factor of unpredictability. Because the structure of longitudinal studies will follow the same individuals over an extended time period, what happens to each person outside of the scope of the research can have a direct impact on the eventual findings that researchers develop. Some people may choose to stop participating in the study altogether, which may reduce the validity of the final result when published. It is possible for some individuals or households to shift their demographic profile so that they are no longer viable candidates for the research. Unless these factors are included in the initial structure of the project, then the findings that are developed from the work could be invalid.
The pros and cons of longitudinal studies provides us with a valuable foundation of data that makes it possible to recognize long-term relationships, determine their value, and where it may be possible to make healthy changes in numerous fields. There are certain risks to consider with this process that may create unpredictable outcomes, but it is also through this research method that we will likely find new ways to transform numerous scientific and medical fields in the future.
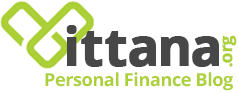
13 Advantages of Disadvantages of Longitudinal Studies
Longitudinal studies are a method of observational research. In this type of study, data is gathered from the same subjects repeatedly over a defined period. Because of this structure, it is possible for a longitudinal study to last for several years or even several decades. This form of research is common in the areas of sociology, psychology, and medicine.
The primary advantage of using this form of research is that it can help find patterns that may occur over long periods, but would not be observed over short periods. Changes can be tracked so that cause and effect relationships can be discovered.
The primary disadvantage of using longitudinal studies for research is that long-term research increases the chances of unpredictable outcomes. If the same people cannot be found for a study update, then the research ceases.
Here are some additional key advantages and disadvantages of longitudinal studies to think about.
What Are the Advantages of Longitudinal Studies?
1. It allows for high levels of validity. For a long-term study to be successful, there must be rules and regulations in place at the beginning that dictate the path that researchers must follow. The end goal of the research must be defined at the beginning of the process as well, with outlined steps in place that verify the authenticity of the data being collected. This means high levels of data validity are often available through longitudinal studies.
2. The data collected is unique. Most research studies will collect short-term data to determine the cause-and-effect of what is being researched. Longitudinal studies follow the same principles, but extend the timeframe for data collection on a dramatic scale. Long-term relationships cannot be discovered in short-term research, but short-term relationships can be tracked in long-term research.
3. Most will use the observational method. Because longitudinal studies will use the observational method for data collection more often than not, it is easier to collect consistent data at a personal level. This consistency allows for differences to be excluded on a personal level, making it easier to exclude variations that could affect data outcomes in other research methods.
4. It makes it possible to identify developmental trends. Whether in medicine, psychology, or sociology, the long-term design of a longitudinal study makes it possible to find trends and relationships within the data collected. It isn’t just the span of a human life that can be tracked with this type of research. Multiple generations can have real-time data collected and analyzed to find trends. Observational changes can also be made from past data so it can be applied to future outcomes.
5. Data collection accuracy is almost always high. Because data is collected in real-time using observational data, the collection process is almost always accurate. Humans are fallible beings, so mistakes are always possible, but the structure of this research format limits those mistakes. That data can also be used to implement necessary changes that a course of action may need to take so the best possible outcome can be identified.
6. Longitudinal studies can be designed for flexibility. Although a longitudinal study may be created to study one specific data point, the collected data may show unanticipated patterns or relationships that may be meaningful. Because this is a long-term study, there is a flexibility available to researchers that is not available in other research formats. Additional data points can be collected to study the unanticipated findings, allowing for shifts in focus to occur whenever something interesting is found.
What Are the Disadvantages of Longitudinal Studies?
1. There is a factor of unpredictability always present. Because longitudinal studies involve the same subjects over a long period, what happens to them outside of the data collection moments can influence future data being collected. Some people may choose to stop participating in the research. Others may no longer find themselves in the correct demographics for the research. If these factors are not included in the initial design of the research, then it could invalidate the findings that are produced.
2. It takes time. Researchers involved with longitudinal studies may never see the full outcome of their work. It may take several years before the data begins producing observable patterns or relationships that can be tracked. That means the ability to maintain open lines of communication with all researchers is vitally important to the eventual success of the study.
3. The data gathered by longitudinal studies is not always accurate or reliable. It only takes one piece of unreliable or inaccurate data to possibly invalidate the findings that the longitudinal studies produce. Because humans have their own personal bias toward certain subjects, the researcher processing the data may unconsciously alter the data to produce intended results.
4. It relies on the skills of the researchers to be complete. Because data collection occurs in real-time and relies heavily on the skills of the researchers who are tasked with this job, the quality of the data is heavily reliant on those skills. Two different researchers with varying skill levels can produce very different data points from the same subject material. Personal views of the data being collected can also impact the results on both ends, from the subject or the collector.
5. Large sample sizes are required to make the research meaningful. To develop relationships or patterns, a large amount of data must be collected and then mined to create results. That means a large sample size is required so the amount of data being collected can meet expectations. When the subjects being studied are people, it can be difficult to find enough people who are willing to honestly participate in the longitudinal studies.
6. There is a direct cost that is higher than other forms of research. Longitudinal research requires a larger sample size, which means there is a larger cost involved in contacting subjects to collect data. It is also a long-term form of research, which means the costs of the study will be extended for years, or decades, when other forms of research may be completed in a fraction of that time.
7. One person can change a long-term outcome. Because there is such a reliance on individual interpretations within longitudinal studies, it is possible for one person to inadvertently alter or invalidate the data being collected. It is entirely possible for decades of research to be invalidated because one subject or researcher was misleading.
The advantages and disadvantages of longitudinal studies show us that there is a tremendous value available in the ability to find long-term patterns and relationships. If the unpredictable factors of this research format can be planned for in advance and steps taken to remove bias, the data collected offers the potential to dramatically change the fields of medicine, psychology, or sociology.
1.6: Longitudinal Research
Chapter 1: research methods, chapter 2: the social self, chapter 3: social judgement and decision-making, chapter 4: understanding and influencing others, chapter 5: attitudes and persuasion, chapter 6: close relationships, chapter 7: stereotypes, prejudice, and discrimination, chapter 8: helping and hurting, chapter 9: group dynamics.
The JoVE video player is compatible with HTML5 and Adobe Flash. Older browsers that do not support HTML5 and the H.264 video codec will still use a Flash-based video player. We recommend downloading the newest version of Flash here, but we support all versions 10 and above.

Sometimes the goal of a psychological study may be to understand how people’s attitudes and behaviors change over time, or to determine what factors may predict future abilities.
These objectives can be accomplished using a longitudinal design —a research study where data are repeatedly collected from the same group of individuals for a period of time, whether it’s as short as a few weeks or months or as long as several decades.
For example, if a researcher wants to know whether college students’ exercise routines change over the course of their first semester of college, she can use a longitudinal approach and ask students to repeatedly report their workout regiments. She may find that as students get more caught up in their studies, they go to the gym less often.
In addition, the same researcher may keep track of a group of people for twenty years, because she wants to explore how their exercise routines shift across their 20s, 30s and 40s. This approach allows her to best measure changes, within individuals, over time.
In this case, she may discover that those who enjoyed running outdoors in their 20s maintain blood pressure levels, display low amounts of stress, and are more likely to do yoga in their 40s.
While longitudinal research can provide informative results, the method also has its drawbacks. For instance, long-running studies can be very expensive and require a significant time-commitment from the research team and their participants.
Because of this commitment, attrition rates tend to be higher—meaning, more participants dropout. For this reason, the researcher would have to recruit more individuals at the start of the study, expecting a certain number to dropout. Attrition may also cause the study’s sample to be less representative of the population.
Despite its disadvantages, longitudinal research has the power to help us understand variation across human development and the lifespan.
One of the longest-running studies—following people over 80 years as opposed to comparing different groups at different ages—provides a robust measure of human growth—even revealing factors, like close relationships, that lead to people living healthy and happy lives.
Sometimes we want to see how people change over time, as in studies of human development and lifespan. When we test the same group of individuals repeatedly over an extended period of time, we are conducting longitudinal research. Longitudinal research is a research design in which data-gathering is administered repeatedly over an extended period of time. For example, we may survey a group of individuals about their dietary habits at age 20, retest them a decade later at age 30, and then again at age 40.
Let's consider another example. In recent years there has been significant growth in the popular support of same-sex marriage. Many studies on this topic break down survey participants into different age groups. In general, younger people are more supportive of same-sex marriage than are those who are older (Jones, 2013). Does this mean that as we age we become less open to the idea of same-sex marriage, or does this mean that older individuals have different perspectives because of the social climates in which they grew up? Longitudinal research is a powerful approach because the same individuals are involved in the research project over time, which means that the researchers need to be less concerned with differences among cohorts affecting the results of their study.
Often longitudinal studies are employed when researching various diseases in an effort to understand particular risk factors. Such studies often involve tens of thousands of individuals who are followed for several decades. Given the enormous number of people involved in these studies, researchers can feel confident that their findings can be generalized to the larger population. The Cancer Prevention Study-3 (CPS-3) is one of a series of longitudinal studies sponsored by the American Cancer Society aimed at determining predictive risk factors associated with cancer. When participants enter the study, they complete a survey about their lives and family histories, providing information on factors that might cause or prevent the development of cancer. Then every few years the participants receive additional surveys to complete. In the end, hundreds of thousands of participants will be tracked over 20 years to determine which of them develop cancer and which do not.
Clearly, this type of research is important and potentially very informative. For instance, earlier longitudinal studies sponsored by the American Cancer Society provided some of the first scientific demonstrations of the now well-established links between increased rates of cancer and smoking (American Cancer Society, n.d.).
As with any research strategy, longitudinal research is not without limitations. For one, these studies require an incredible time investment by the researcher and research participants. Given that some longitudinal studies take years, if not decades, to complete, the results will not be known for a considerable period of time. In addition to the time demands, these studies also require a substantial financial investment. Many researchers are unable to commit the resources necessary to see a longitudinal project through to the end.
Research participants must also be willing to continue their participation for an extended period of time, and this can be problematic. People move, get married and take new names, get ill, and eventually die. Even without significant life changes, some people may simply choose to discontinue their participation in the project. As a result, the attrition rates , or reduction in the number of research participants due to dropouts, in longitudinal studies are quite high and increases over the course of a project. For this reason, researchers using this approach typically recruit many participants fully expecting that a substantial number will drop out before the end. As the study progresses, they continually check whether the sample still represents the larger population, and make adjustments as necessary.
This text is adapted from OpenStax, Psychology. OpenStax CNX.
Get cutting-edge science videos from J o VE sent straight to your inbox every month.
mktb-description
We use cookies to enhance your experience on our website.
By continuing to use our website or clicking “Continue”, you are agreeing to accept our cookies.
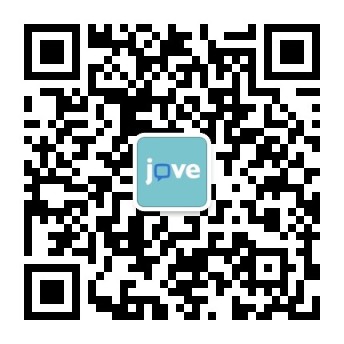
- Bipolar Disorder
- Therapy Center
- When To See a Therapist
- Types of Therapy
- Best Online Therapy
- Best Couples Therapy
- Best Family Therapy
- Managing Stress
- Sleep and Dreaming
- Understanding Emotions
- Self-Improvement
- Healthy Relationships
- Student Resources
- Personality Types
- Guided Meditations
- Verywell Mind Insights
- 2024 Verywell Mind 25
- Mental Health in the Classroom
- Editorial Process
- Meet Our Review Board
- Crisis Support
What Is a Longitudinal Study?
Tracking Variables Over Time
Kendra Cherry, MS, is a psychosocial rehabilitation specialist, psychology educator, and author of the "Everything Psychology Book."
:max_bytes(150000):strip_icc():format(webp)/IMG_9791-89504ab694d54b66bbd72cb84ffb860e.jpg)
Amanda Tust is a fact-checker, researcher, and writer with a Master of Science in Journalism from Northwestern University's Medill School of Journalism.
:max_bytes(150000):strip_icc():format(webp)/Amanda-Tust-1000-ffe096be0137462fbfba1f0759e07eb9.jpg)
Steve McAlister / The Image Bank / Getty Images
The Typical Longitudinal Study
Potential pitfalls, frequently asked questions.
A longitudinal study follows what happens to selected variables over an extended time. Psychologists use the longitudinal study design to explore possible relationships among variables in the same group of individuals over an extended period.
Once researchers have determined the study's scope, participants, and procedures, most longitudinal studies begin with baseline data collection. In the days, months, years, or even decades that follow, they continually gather more information so they can observe how variables change over time relative to the baseline.
For example, imagine that researchers are interested in the mental health benefits of exercise in middle age and how exercise affects cognitive health as people age. The researchers hypothesize that people who are more physically fit in their 40s and 50s will be less likely to experience cognitive declines in their 70s and 80s.
Longitudinal vs. Cross-Sectional Studies
Longitudinal studies, a type of correlational research , are usually observational, in contrast with cross-sectional research . Longitudinal research involves collecting data over an extended time, whereas cross-sectional research involves collecting data at a single point.
To test this hypothesis, the researchers recruit participants who are in their mid-40s to early 50s. They collect data related to current physical fitness, exercise habits, and performance on cognitive function tests. The researchers continue to track activity levels and test results for a certain number of years, look for trends in and relationships among the studied variables, and test the data against their hypothesis to form a conclusion.
Examples of Early Longitudinal Study Design
Examples of longitudinal studies extend back to the 17th century, when King Louis XIV periodically gathered information from his Canadian subjects, including their ages, marital statuses, occupations, and assets such as livestock and land. He used the data to spot trends over the years and understand his colonies' health and economic viability.
In the 18th century, Count Philibert Gueneau de Montbeillard conducted the first recorded longitudinal study when he measured his son every six months and published the information in "Histoire Naturelle."
The Genetic Studies of Genius (also known as the Terman Study of the Gifted), which began in 1921, is one of the first studies to follow participants from childhood into adulthood. Psychologist Lewis Terman's goal was to examine the similarities among gifted children and disprove the common assumption at the time that gifted children were "socially inept."
Types of Longitudinal Studies
Longitudinal studies fall into three main categories.
- Panel study : Sampling of a cross-section of individuals
- Cohort study : Sampling of a group based on a specific event, such as birth, geographic location, or experience
- Retrospective study : Review of historical information such as medical records
Benefits of Longitudinal Research
A longitudinal study can provide valuable insight that other studies can't. They're particularly useful when studying developmental and lifespan issues because they allow glimpses into changes and possible reasons for them.
For example, some longitudinal studies have explored differences and similarities among identical twins, some reared together and some apart. In these types of studies, researchers tracked participants from childhood into adulthood to see how environment influences personality , achievement, and other areas.
Because the participants share the same genetics , researchers chalked up any differences to environmental factors . Researchers can then look at what the participants have in common and where they differ to see which characteristics are more strongly influenced by either genetics or experience. Note that adoption agencies no longer separate twins, so such studies are unlikely today. Longitudinal studies on twins have shifted to those within the same household.
As with other types of psychology research, researchers must take into account some common challenges when considering, designing, and performing a longitudinal study.
Longitudinal studies require time and are often quite expensive. Because of this, these studies often have only a small group of subjects, which makes it difficult to apply the results to a larger population.
Selective Attrition
Participants sometimes drop out of a study for any number of reasons, like moving away from the area, illness, or simply losing motivation . This tendency, known as selective attrition , shrinks the sample size and decreases the amount of data collected.
If the final group no longer reflects the original representative sample , attrition can threaten the validity of the experiment. Validity refers to whether or not a test or experiment accurately measures what it claims to measure. If the final group of participants doesn't represent the larger group accurately, generalizing the study's conclusions is difficult.
The World’s Longest-Running Longitudinal Study
Lewis Terman aimed to investigate how highly intelligent children develop into adulthood with his "Genetic Studies of Genius." Results from this study were still being compiled into the 2000s. However, Terman was a proponent of eugenics and has been accused of letting his own sexism , racism , and economic prejudice influence his study and of drawing major conclusions from weak evidence. However, Terman's study remains influential in longitudinal studies. For example, a recent study found new information on the original Terman sample, which indicated that men who skipped a grade as children went on to have higher incomes than those who didn't.
A Word From Verywell
Longitudinal studies can provide a wealth of valuable information that would be difficult to gather any other way. Despite the typical expense and time involved, longitudinal studies from the past continue to influence and inspire researchers and students today.
A longitudinal study follows up with the same sample (i.e., group of people) over time, whereas a cross-sectional study examines one sample at a single point in time, like a snapshot.
A longitudinal study can occur over any length of time, from a few weeks to a few decades or even longer.
That depends on what researchers are investigating. A researcher can measure data on just one participant or thousands over time. The larger the sample size, of course, the more likely the study is to yield results that can be extrapolated.
Piccinin AM, Knight JE. History of longitudinal studies of psychological aging . Encyclopedia of Geropsychology. 2017:1103-1109. doi:10.1007/978-981-287-082-7_103
Terman L. Study of the gifted . In: The SAGE Encyclopedia of Educational Research, Measurement, and Evaluation. 2018. doi:10.4135/9781506326139.n691
Sahu M, Prasuna JG. Twin studies: A unique epidemiological tool . Indian J Community Med . 2016;41(3):177-182. doi:10.4103/0970-0218.183593
Almqvist C, Lichtenstein P. Pediatric twin studies . In: Twin Research for Everyone . Elsevier; 2022:431-438.
Warne RT. An evaluation (and vindication?) of Lewis Terman: What the father of gifted education can teach the 21st century . Gifted Child Q. 2018;63(1):3-21. doi:10.1177/0016986218799433
Warne RT, Liu JK. Income differences among grade skippers and non-grade skippers across genders in the Terman sample, 1936–1976 . Learning and Instruction. 2017;47:1-12. doi:10.1016/j.learninstruc.2016.10.004
Wang X, Cheng Z. Cross-sectional studies: Strengths, weaknesses, and recommendations . Chest . 2020;158(1S):S65-S71. doi:10.1016/j.chest.2020.03.012
Caruana EJ, Roman M, Hernández-Sánchez J, Solli P. Longitudinal studies . J Thorac Dis . 2015;7(11):E537-E540. doi:10.3978/j.issn.2072-1439.2015.10.63
By Kendra Cherry, MSEd Kendra Cherry, MS, is a psychosocial rehabilitation specialist, psychology educator, and author of the "Everything Psychology Book."
Administrative data
Administrative data is the term used to describe everyday data about individuals collected by government departments and agencies. Examples include exam results, benefit receipt and National Insurance payments.
Age effects
Age effects relates to changes in an outcome as a result of getting older.
Anonymisation
Anonymisation refers to the removal of study participants ’ identifying information (e.g., name, address) in order to preserve their privacy.
Attrition is the discontinued participation of study participants in a longitudinal study. Attrition can reflect a range of factors, from the study participant not being traceable to them choosing not to take part when contacted. Attrition is problematic both because it can lead to bias in the study findings (if the attrition is higher among some groups than others) and because it reduces the size of the sample .
Baseline refers to the start of a study when initial information is collected on participation (however, in longitudinal studies , researchers may adopt an alternative ‘baseline’ for the purposes of analysis).
Biological samples
Biological samples is the term used for specimens collected from human subjects from which biological information, such as genetic markers, can be extracted for analysis. Common examples include blood, saliva or hair.
Body mass index
Body mass index is a measure used to assess if an individual is a healthy weight for their height. It is calculated by dividing the individual’s weight by the square of their height, and it is typically represented in units of kg/m 2 .
Boosted samples
Boosted samples are used to overcome sample bias due to attrition or to supplement the representation of smaller sub-groups within the sample . Inclusion of boosted samples must be accompanied by appropriate survey weights .
Computer-assisted personal interviewing (CAPI) is a technique for collecting data from participants using computers to eliminate common errors such as questionnaire routing and data entry mistakes. The use of computers take place within the context of a face-to-face interview.
Computer-assisted self-interviewing (CASI) is a technique for collecting data from participants using computers to eliminate common errors such as questionnaire routing and data entry mistakes. The use of computers take place within the context of a self-completion questionnaire.
Categorical variable
A categorical variable is a variable that can take one of a limited number of discrete values. They can be either nominal – they contain no inherent order of categories (e.g. sex; marital status) – or ordinal – they can be ranked in some meaningful order (e.g. level of satisfaction with a service).
Computer-assisted telephone interviewing (CATI) is a technique for collecting data from participants using computers to eliminate common errors such as questionnaire routing and data entry mistakes. The use of computers take place within the context of a telephone interview.
For some study participants the exact time of an event will not be known because either: the study ends (or the analysis is carried out) before they have had the event, or the participant drops out of the study before experiencing the event. It is therefore, only known that the event has not occurred up to the time that they were last observed in the study.
Census refers to a universal and systematic collection of data from all individuals within a population . In the UK, the government conducts a census every ten years with the next one due in 2021.
A codebook is a document (online or hard-copy) that contains all the information about how a dataset has been coded, such that it can be deciphered by a researcher not familiar with the original coding frame.
Coding is the process of converting survey responses into numerical codes to facilitate data analysis. All potential responses (as well as possible reasons for non-response) for each variable are assigned numerical values according to a coding frame.
Cognitive assessments
Cognitive assessments are exercises used to measure thinking abilities, such as memory, reasoning and language. Longitudinal studies collecting data in this way can track the extent to which someone’s cognitive abilities change (develop or decline) over time.
Cohort studies
Cohort studies are concerned with charting the lives of groups of individuals who experience the same life events within a given time period. The best known examples are birth cohort studies, which follow a group of people born in a particular period.
Complete case analysis
Complete case analysis is the term used to describe a statistical analysis that only includes participants for which we have no missing data on the variables of interest. Participants with any missing data are excluded.
Conditioning
Conditioning refers to the process whereby participants’ answers to some questions may be influenced by their participation in the study – in other words, their responses are ‘conditioned’ by their being members of a longitudinal study. Examples would include study respondents answering questions differently or even behaving differently as a result of their participation in the study.
Confounding
Confounding occurs where the relationship between independent and dependent variables is distorted by one or more additional, and sometimes unmeasured, variables . A confounding variable must be associated with both the independent and dependent variables but must not be an intermediate step in the relationship between the two (i.e. not on the causal pathway).
For example, we know that physical exercise (an independent variable) can reduce a person’s risk of cardiovascular disease (a dependent variable ). We can say that age is a confounder of that relationship as it is associated with, but not caused by, physical activity and is also associated with coronary health. See also ‘ unobserved heterogeneity ’, below.
Continuous variable
A continuous variable is a variable that has an infinite number of uncountable values e.g. time, temperature. They are also known as quantitative variables or scale variables .
Cohort effects
Cohort effects relates to changes in an outcome associated with being a member of a specific cohort of people (e.g. born in the same year; or starting school at the same time).
In metadata management, coverage refers to the temporal, spatial and topical aspects of the data collection to describe the comprehensiveness of a dataset. For longitudinal studies , this can relate to the topics that are covered across waves, the population to which one can generalise or the geographic extent of the dataset.
Cross-sectional
Cross-sectional surveys involve interviewing a fresh sample of people each time they are carried out. Some cross-sectional studies are repeated regularly and can include a large number of repeat questions (questions asked on each survey round).
Data access agreement
Within the context of data protection , a data access agreement specifies the terms under which users are provided access to specified datasets. This usually forms part of the application process to the data controller to ensure that researchers adhere to a set of terms regarding data confidentiality , sensitivity and dissemination before accessing the data. See also: research ethics
Data cleaning
Data cleaning is an important preliminary step in the data analysis process and involves preparing a dataset so that it can be correctly analysed. ‘Cleaning’ the data usually involves identifying data input errors, assessing the completeness of the dataset and verifying any anomalies (e.g. outliers).
Data confidentiality
Within the context of data protection , data confidentiality is the process of protecting participants’ data from being accessed or disclosed by those unauthorised to do so. Key methods employed in data confidentiality include anonymisation of responses (removal of personal identifying information) and data encryption (protecting the data using codes and/or passwords).
Data harmonisation
Data harmonisation involves retrospectively adjusting data collected by different surveys to make it possible to compare the data that was collected. This enables researchers to make comparisons both within and across studies. Repeating the same longitudinal analysis across a number of studies allows researchers to test whether results are consistent across studies, or differ in response to changing social conditions.
Data imputation
Data imputation is a technique for replacing missing data with an alternative estimate. There are a number of different approaches, including mean substitution and model-based multivariate approaches.
Data linkage
Data linkage simply means connecting two or more sources of administrative, educational, geographic, health or survey data relating to the same individual for research and statistical purposes. For example, linking housing or income data to exam results data could be used to investigate the impact of socioeconomic factors on educational outcomes.
Data protection
Data protection refers to the broad suite of rules governing the handling and access of information about people. Data protection principles include confidentiality of responses, informed consent of participants and security of data access. These principles are legally protected by the Data Protection Act (DPA) and the General Data Protection Regulation (GDPR).
Data structure
Data structure refers to the way in which data are organised and formatting in advance of data analysis.
Dependent variable
In analysis, the dependent variable is the variable you expect to change in response to different values of your independent (or predictor) variables . For example, a students’ test results may be (partially) explained by the number of hours spent on revision. In this case, the dependent variable is students’ test score, which you expect to be different according to the amount of time spent revising.
Derived variable
A derived variable is a variable that is calculated from the values of other variables and not asked directly of the participants. It can involve a mathematical calculation (e.g. deriving monthly income from annual income by dividing by 12) or a recategorisation of one or more existing variables (e.g. categorising monthly income into £500 bands – £0 to £500, £501 to £1,000, etc.)
Diaries are a data collection instrument that is particularly useful in recording information about time use or other regular activity, such as food intake. They have the benefit of collecting data from participants as and when an activity occurs. As such, they can minimise recall bias and provide a more accurate record of activities than a retrospective interview.
Dissemination
Dissemination is the process of sharing information – particularly research findings – to other researchers, stakeholders, policy makers, and practitioners through various avenues and channels, including online, written publications and events. Dissemination is a planned process that involves consideration of target audiences in ways that will facilitate research uptake in decision-making processes and practice.
Dummy variables
Dummy variables , also called indicator variables , are sets of dichotomous (two-category) variables we create to enable subgroup comparisons when we are analysing a categorical variable with three or more categories.
Empirical data
Empirical data refers to data collected through observation or experimentation. Analysis of empirical data can provide evidence for how a theory or assumption works in practice.
In metadata management, fields are the elements of a database which describes the attributes of items of data.
General ability
General ability is a term used to describe cognitive ability, and is sometimes used as a proxy for intelligent quotient (IQ) scores.
Growth curve modelling
Growth curve modelling is used to analyse trajectories of longitudinal change over time allowing us to model the way participants change over time, and then to explore what characteristics or circumstances influence these patterns of longitudinal change.
Hazard rate
Hazard rate refers to the probability that an event of interest occurs at a given time point, given that it has not occurred before.
Health assessments
Health assessments refers to the assessments carried out on research participants in relation to their physical characteristics or function. These can include measurements of height and weight, blood pressure or lung function.
Heterogeneity
Heterogeneity is a term that refers to differences, most commonly differences in characteristics between study participants or samples. It is the opposite of homogeneity, which is the term used when participants share the same characteristics. Where there are differences between study designs, this is sometimes referred to as methodological heterogeneity. Both participant or methodological differences can cause divergences between the findings of individual studies and if these are greater than chance alone, we call this statistical heterogeneity. See also: unobserved heterogeneity .
Household panel surveys
Household panel surveys collect information about the whole household at each wave of data collection, to allow individuals to be viewed in the context of their overall household. To remain representative of the population of households as a whole, studies will typically have rules governing how new entrants to the household are added to the study.
Incentives and rewards
As a way of encouraging participants to take part in research, they may be offered an incentive or a reward. These may be monetary or, more commonly, non-monetary vouchers or tokens. Incentives are advertised beforehand and can act as an aid to recruitment; rewards are a token of gratitude to the participants for giving their time.
Independent variable
In analysis, an independent variable is any factor that may be associated with an outcome or dependent variable . For example, the number of hours a student spends on revision may influence their test result. In this case, the independent variable, revision time (at least partially) ‘explains’ the outcome of the test.
Informed consent
A key principle of research ethics , informed consent refers to the process of providing full details of the research to participants so that they are sufficiently able to choose whether or not to consent to taking part.
Kurtosis is sometimes described as a measure of ‘tailedness’. It is a characteristic of the distribution of observations on a variable and denotes the heaviness of the distribution’s tails. To put it another way, it is a measure of how thin or fat the lower and upper ends of a distribution are.
Life course
A person’s life course refers to the experiences and stages an individual passes through during their life. It centres on the individual and emphasises the changing social and contextual processes that influence their life over time.
Longitudinal studies
Longitudinal studies gather data about the same individuals (‘ study participants ’) repeatedly over a period of time, in some cases from birth until old age. Many longitudinal studies focus upon individuals, but some look at whole households or organisations.
Metadata refers to data about data, which provides the contextual information that allows you to interpret what data mean.
Missing data
Missing data refers to values that are missing and do not appear in a dataset. This may be due to item non-response, participant drop-out (or attrition ) or, in longitudinal studies , some data (e.g. date of birth) may be collected only in some waves. Large amounts of missing data can be a problem and lead researchers to make erroneous inferences from their analysis. There are several ways to deal with the issue of missing data, from casewise deletion to complex multiple imputation models.
Multi-level modelling
Multi-level modelling refers to statistical techniques used to analyse data that is structured in a hierarchical or nested way. For example. study participants who belong to the same household, or students who attend the same school may be expected to be more similar to each other than to participants in other households or schools (such as sharing similar contextual influences). This similarity means that the data from participants within these households/schools are not independent. Multi-level models can account for variability at both the individual level and the group (e.g. household or school) level.
Non-response bias
Non-response bias is a type of bias introduced when those who participate in a study differ to those who do not in a way that is not random (for example, if attrition rates are particularly high among certain sub-groups). Non-random attrition over time can mean that the sample no longer remains representative of the original population being studied. Two approaches are typically adopted to deal with this type of missing data : weighting survey responses to re-balance the sample , and imputing values for the missing information.
Observational studies
Observational studies focus on observing the characteristics of a particular sample without attempting to influence any aspects of the participants’ lives. They can be contrasted with experimental studies, which apply a specific ‘treatment’ to some participants in order to understand its effect.
Panel studies
Panel studies follow the same individuals over time. They vary considerably in scope and scale . Examples include online opinion panels and short-term studies whereby people are followed up once or twice after an initial interview.
Peer review
Peer review is a method of quality control in the process of academic publishing, whereby research is appraised (usually anonymously) by one or more independent academic with expertise in the subject.
Period effects
Period effects relate to changes in an outcome associated with living during a particular time, regardless of age or cohort membership (e.g. living through times of war, economic recession or global pandemic).
Piloting is the process of testing your research instruments and procedures to identify potential problems or issues before implementing them in the full study. A pilot study is usually conducted on a small subset of eligible participants who are encouraged to provide feedback on the length, comprehensibility and format of the process and to highlight any other potential issues.
Population refers to all the people of interest to the study and to whom the findings will be able to be generalized (e.g. a study looking into rates of recidivism may have a [target] population encompassing everyone with a criminal conviction). Owing to the size of the population, a study will usually select a sample from which to make inferences. See also: sample , representiveness.
Percentiles
A percentile is a measure that allows us to explore the distribution of data on a variable. It denotes the percentage of individuals or observations that fall below a specified value on a variable. The value that splits the number of observations evenly, i.e. 50% of the observations on a variable fall below this value and 50% above, is called the 50th percentile or more commonly, the median.
Primary research
Primary research refers to original research undertaken by researchers collecting new data. It has the benefit that researchers can design the study to answer specific questions and hypotheses rather than relying on data collected for similar but not necessarily identical purposes. See also: secondary research
Prospective study
In prospective studies, individuals are followed over time and data about them is collected as their characteristics or circumstances change.
Qualitative data
Qualitative data are non-numeric – typically textual, audio or visual. Qualitative data are collected through interviews, focus groups or participant observation. Qualitative data are often analysed thematically to identify patterns of behaviour and attitudes that may be highly context-specific.
Quantitative data
Quantitative data can be counted, measured and expressed numerically. They are collected through measurement or by administering structured questionnaires . Quantitative data can be analysed using statistical techniques to test hypotheses and make inferences to a population .
Questionnaires
Questionnaires are research instruments used to elicit information from participants in a structured way. They might be administered by an interviewer (either face-to-face or over the phone), or completed by the participants on their own (either online or using a paper questionnaire. Questions can cover a wide range of topics and often include previously-validated instruments and scales (e.g. the Rosenberg Self-Esteem Scale ).
Recall error or bias
Recall error or bias describes the errors that can occur when study participants are asked to recall events or experiences from the past. It can take a number of forms – participants might completely forget something happened, or misremember aspects of it, such as when it happened, how long it lasted, or other details. Certain questions are more susceptible to recall bias than others. For example, it is usually easy for a person to accurately recall the date they got married, but it is much harder to accurately recall how much they earned in a particular job, or how their mood at a particular time.
Record linkage
Record linkage studies involve linking together administrative records (for example, benefit receipts or census records) for the same individuals over time.
Reference group
A reference group is a category on a categorical variable to which we compare other values. It is a term that is commonly used in the context of regression analyses in which categorical variables are being modelled.
Regression analysis
Regression analysis refers to analytical techniques that use a mathematical ‘model’ to predict values of a dependent variable from values of one or many independent variable (s).
Repeated measures
Repeated measures are measurements of the same variable at multiple time points on the same participants, allowing researchers to study change over time.
Representativeness
Representativeness is the extent to which a sample is representative of the population from which it is selected. Representative samples can be achieved through, for example, random sampling, systematic sampling, stratified sampling or cluster sampling.
Research ethics
Research ethics relates to the fundamental codes of practice associated with conducting research. Ethical issues that need to be considered include providing informed consent to participants, non-disclosure of sensitive information, confidentiality and anonymity safeguarding of vulnerable groups , and respect for participants’ well-being. Academic research proposals need be approved by an ethics committee before any actual research (either primary or secondary) can begin.
Research impact
Research impact is the demonstrable contribution that research makes to society and the economy that can be realised through engagement with other researchers and academics, policy makers, stakeholders and members of the general public. It includes influencing policy development, improving practice or service provision, or advancing skills and techniques.
Residuals are the difference between your observed values (the constant and predictors in the model) and expected values (the error), i.e. the distance of the actual value from the estimated value on the regression line.
Respondent burden
Respondent burden is a catch all phrase that describes the perceived burden faced by participants as a result of their being involved in a study. It could include time spent taking part in the interview and inconvenience this may cause, as well as any difficulties faced as a result of the content of the interview.
Response rate
Response rate refers to the proportion of participants in the target sample who completed the survey. Longitudinal surveys are designed with the expectation that response rates will decline over time so will typically seek to recruit a large initial sample in order to compensate for likely attrition of participants.
Retrospective study
In retrospective studies, individuals are sampled and information is collected about their past. This might be through interviews in which participants are asked to recall important events, or by identifying relevant administrative data to fill in information on past events and circumstances.
Sample is a subset of a population that is used to represent the population as a whole. This reflects the fact that it is often not practical or necessary to survey every member of a particular population . In the case of birth cohort studies , the larger ‘ population ’ from which the sample is drawn comprises those born in a particular period. In the case of a household panel study like Understanding Society, the larger population from which the sample was drawn comprised all residential addresses in the UK.
Sample size
Sample size refers to the number of data units contained within a dataset. It most frequently refers to the number of respondents who took part in your study and for whom there is usable data. However, it could also relate to households, countries or other institutions. The size of a sample , relative to the size of the population , will have consequences for analysis: the larger a sample is, the smaller the margin of error of its estimates, the more reliable the results of the analysis and the greater statistical power of the study.
Sampling frame
A sampling frame is a list of the target population from which potential study participants can be selected.
Scales are frequently used as part of a research instrument seeking to measure specific concepts in a uniform and replicable way. Typically, they are composed of multiple items that are aggregated into one or more composite scores. Examples of standardised scales include the British Ability Scale (BAS); the Malaise Inventory; and the Rosenberg Self-Esteem Scale.
Scatterplot
A scatterplot is a way of visualising the relationship between two continuous variables by plotting the value of each associated with a single case on a set of X-Y coordinates. For example, students’ test scores in English and maths can be represented as point on a graph, with each point representing a single student’s English (x-axis) and maths (y-axis) score. Looking at data for many students allows us to build up a visualisation of the relationship between students’ scores in maths and English.
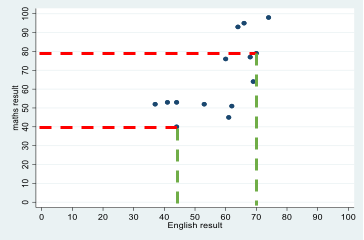
Secondary research
Secondary research refers to new research undertaken using data previously collected by others. It has the benefit of being more cost-effective than primary research whilst still providing important insights into research questions under investigation.
Skewness is the measure of how assymetrical the distribution of observations are on a variable. If the distribution has a more pronounced/longer tail at the upper end of the distribution (right-hand side), we say that the distribution is negatively skewed. If it is more pronounced/longer at the lower end (left-hand side), we say that it is positively skewed.
Statistical model
A statistical model is a mathematical representation of the relationship between variables .
Statistical software
Statistical software packages are specifically designed to carry out statistical analysis; these can either be open-source (e.g. R ) or available through institutional or individual subscription (e.g. SPSS ; Stata ).
Structured metadata
Structured metadata define the relationship between data items/objects to enable computer systems to understand the contextual meaning of the data. It uses standardised content to facilitate the use of metadata for data discovery and sharing, and the relationship between metadata elements.
Study participants
Study participants are the individuals who are interviewed as part of a longitudinal study.
Survey logic
Also called conditional routing (sometimes called ‘filters’), survey logic refers to the flow that takes respondents through a survey. Respondents may be required to answer some questions only if they had provided a relevant response to a previous question. E.g. Only respondents who are currently at university may be asked to answer a question relating to their degree subject. This is important when considering missing data .
Survey weights
Survey weights can be used to adjust a survey sample so it is representative of the survey population as a whole. They may be used to reduce the impact of attrition on the sample , or to correct for certain groups being over-sampled.
Survival analysis
Survival analysis is an analytical technique that uses time-to-event data to statistically model the probability of experiencing an event by a given time point. For example, time to retirement, disease onset or length of periods of unemployment.
The term used to refer to a round of data collection in a particular longitudinal study (for example, the age 7 sweep of the National Child Development Study refers to the data collection that took place in 1965 when the participants were aged 7). Note that the term wave often has the same meaning.
Target population
The population of people that the study team wants to research, and from which a sample will be drawn.
Time to event
Time to event refers to the duration of time (e.g. in hours, days, months, etc.) from a defined baseline to the time of occurrence of an event of interest (e.g. diagnosis of an illness, first re-offence following release from prison). Survival analysis can be used to analyse such data.
Tracing (or tracking)
Tracing (or tracking) describes the process by which study teams attempt to locate participants who have moved from the address at which they were last interviewed.
Unobserved heterogeneity
Unobserved heterogeneity is a term that describes the existence of unmeasured (unobserved) differences between study participants or samples that are associated with the (observed) variables of interest. The existence of unobserved variables means that statistical findings based on the observed data may be incorrect.
Part of the documentation that is usually provided with statistical datasets, user guides are an invaluable resource for researchers. The guides contain information about the study, including the sample , data collection procedures, and data processing. Use guides may also provide information about how to analyse the data, whether there are missing data due to survey logic , and advice on how to analyse the data such the application of survey weights .
Variables is the term that tends to be used to describe data items within a dataset. So, for example, a questionnaire might collect information about a participant’s job (its title, whether it involves any supervision, the type of organisation they work for and so on). This information would then be coded using a code-frame and the results made available in the dataset in the form of a variable about occupation. In data analysis variables can be described as ‘dependent’ and ‘independent’, with the dependent variable being a particular outcome of interest (for example, high attainment at school) and the independent variables being the variables that might have a bearing on this outcome (for example, parental education, gender and so on).
Vulnerable groups
Vulnerable groups refers to research participants who may be particularly susceptible to risk or harm as a result of the research process. Different groups might be considered vulnerable in different settings. The term can encompass children and minors, adults with learning difficulties, refugees, the elderly and infirm, economically disadvantaged people, or those in institutional care. Additional consideration and mitigation of potential risk is usually required before research is carried out with vulnerable groups.
The term used to refer to a round of data collection in a particular longitudinal study (for example, the age 7 wave of the National Child Development Study refers to the data collection that took place in 1965 when the participants were aged 7). Note that the term sweep often has the same meaning.
Learning Hub
Using longitudinal data for research.
- What longitudinal studies show us
- How researchers use longitudinal data
- Strengths of longitudinal data
Weaknesses of longitudinal data
Attrition . Over time, participants may cease to take part in a longitudinal study. This is known as attrition . Attrition can result from a range of factors, some of which are unavoidable, while others can be reduced by careful study design or practice.
Unavoidable attrition might be caused by a participant dying, or leaving the country of interest (emigrating). Sometimes attrition occurs when the study team cannot find a participant to invite them to take part. For example, a participant might move house between sweeps and forget to update their contact information. Longitudinal studies use a range of methods to trace their participants in order to prevent this from happening.
Attrition can also occur because participants simply choose not to take part. Participants can choose not to be interviewed in a particular sweep , or can drop out of the study altogether. Again, longitudinal study teams usually have participant engagement strategies to encourage participants to stay involved.
Attrition is problematic for two main reasons. First, attrition reduces the size of the sample , and if the sample becomes too small, it jeopardises statistical power. Second, if attrition is not random, it can lead to non-response bias (which affects the validity of the study findings).
There are a number of ways in which studies try to avoid attrition happening in the first place. Once it has happened, there are analytical approaches that can help compensate for it.
Conditioning . It is possible that participants’ answers to some study questions may be influenced by their participation in the study – in other words, that their responses are ‘conditioned’ by their being members of a longitudinal study. This is referred to as panel conditioning . Examples would include study participants answering questions differently or even behaving differently as a result of their participation in the study.
Limits of representivity. Limits of representivity. Because cohort studies focus on following a specific group (rather than a representative sample of the population as a whole), they are only representative of that specific group. For instance, the 1970 British Cohort Study yields vital information about people born in that year, but does not tell us anything about the generations that precede or follow it. This attribute of cohort studies can be partly overcome by comparing different cohorts to see how their experiences and circumstances differ. Unless cohort studies seek to refresh their samples, they will also not include people born within the relevant time period who have arrived in the sample area since the study began.
Complexity. The datasets can be more complex to manage and analyse than the data for cross-sectional surveys.
Timeliness. By definition, the value of longitudinal studies builds up gradually over time. However, this means that researchers need to wait until the participants get older before they can answer some key research questions.
Longitudinal studies also face the risk that, by the time participants are old enough to allow researchers to answer certain questions, some of the original measures could appear out of date while the overall social and policy landscape may have changed. Equally, the content and design of a new prospective study will inevitably be shaped by the research context at the point at which it was set up. Questions or measures that, with hindsight, seem obvious ones to have collected at the outset of the study may not have seemed so obvious at that time.
Cost. A longitudinal study with a number of waves of data collection will tend to be more expensive than the equivalent number of fresh cross-sectional studies. This reflects the additional complexity involved in designing, maintaining and carrying out longitudinal data collection.
IOE, UCL's Faculty of Education and Society
The learning hub is a resource for students and educators.

Love longitudinal?
Sign up for our email newsletters to get the latest from CLOSER
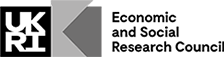
Longitudinal Study Design
Julia Simkus
Editor at Simply Psychology
BA (Hons) Psychology, Princeton University
Julia Simkus is a graduate of Princeton University with a Bachelor of Arts in Psychology. She is currently studying for a Master's Degree in Counseling for Mental Health and Wellness in September 2023. Julia's research has been published in peer reviewed journals.
Learn about our Editorial Process
Saul Mcleod, PhD
Editor-in-Chief for Simply Psychology
BSc (Hons) Psychology, MRes, PhD, University of Manchester
Saul Mcleod, PhD., is a qualified psychology teacher with over 18 years of experience in further and higher education. He has been published in peer-reviewed journals, including the Journal of Clinical Psychology.
Olivia Guy-Evans, MSc
Associate Editor for Simply Psychology
BSc (Hons) Psychology, MSc Psychology of Education
Olivia Guy-Evans is a writer and associate editor for Simply Psychology. She has previously worked in healthcare and educational sectors.
A longitudinal study is a type of observational and correlational study that involves monitoring a population over an extended period of time. It allows researchers to track changes and developments in the subjects over time.
What is a Longitudinal Study?
In longitudinal studies, researchers do not manipulate any variables or interfere with the environment. Instead, they simply conduct observations on the same group of subjects over a period of time.
These research studies can last as short as a week or as long as multiple years or even decades. Unlike cross-sectional studies that measure a moment in time, longitudinal studies last beyond a single moment, enabling researchers to discover cause-and-effect relationships between variables.
They are beneficial for recognizing any changes, developments, or patterns in the characteristics of a target population. Longitudinal studies are often used in clinical and developmental psychology to study shifts in behaviors, thoughts, emotions, and trends throughout a lifetime.
For example, a longitudinal study could be used to examine the progress and well-being of children at critical age periods from birth to adulthood.
The Harvard Study of Adult Development is one of the longest longitudinal studies to date. Researchers in this study have followed the same men group for over 80 years, observing psychosocial variables and biological processes for healthy aging and well-being in late life (see Harvard Second Generation Study).
When designing longitudinal studies, researchers must consider issues like sample selection and generalizability, attrition and selectivity bias, effects of repeated exposure to measures, selection of appropriate statistical models, and coverage of the necessary timespan to capture the phenomena of interest.
Panel Study
- A panel study is a type of longitudinal study design in which the same set of participants are measured repeatedly over time.
- Data is gathered on the same variables of interest at each time point using consistent methods. This allows studying continuity and changes within individuals over time on the key measured constructs.
- Prominent examples include national panel surveys on topics like health, aging, employment, and economics. Panel studies are a type of prospective study .
Cohort Study
- A cohort study is a type of longitudinal study that samples a group of people sharing a common experience or demographic trait within a defined period, such as year of birth.
- Researchers observe a population based on the shared experience of a specific event, such as birth, geographic location, or historical experience. These studies are typically used among medical researchers.
- Cohorts are identified and selected at a starting point (e.g. birth, starting school, entering a job field) and followed forward in time.
- As they age, data is collected on cohort subgroups to determine their differing trajectories. For example, investigating how health outcomes diverge for groups born in 1950s, 1960s, and 1970s.
- Cohort studies do not require the same individuals to be assessed over time; they just require representation from the cohort.
Retrospective Study
- In a retrospective study , researchers either collect data on events that have already occurred or use existing data that already exists in databases, medical records, or interviews to gain insights about a population.
- Appropriate when prospectively following participants from the past starting point is infeasible or unethical. For example, studying early origins of diseases emerging later in life.
- Retrospective studies efficiently provide a “snapshot summary” of the past in relation to present status. However, quality concerns with retrospective data make careful interpretation necessary when inferring causality. Memory biases and selective retention influence quality of retrospective data.
Allows researchers to look at changes over time
Because longitudinal studies observe variables over extended periods of time, researchers can use their data to study developmental shifts and understand how certain things change as we age.
High validation
Since objectives and rules for long-term studies are established before data collection, these studies are authentic and have high levels of validity.
Eliminates recall bias
Recall bias occurs when participants do not remember past events accurately or omit details from previous experiences.
Flexibility
The variables in longitudinal studies can change throughout the study. Even if the study was created to study a specific pattern or characteristic, the data collection could show new data points or relationships that are unique and worth investigating further.
Limitations
Costly and time-consuming.
Longitudinal studies can take months or years to complete, rendering them expensive and time-consuming. Because of this, researchers tend to have difficulty recruiting participants, leading to smaller sample sizes.
Large sample size needed
Longitudinal studies tend to be challenging to conduct because large samples are needed for any relationships or patterns to be meaningful. Researchers are unable to generate results if there is not enough data.
Participants tend to drop out
Not only is it a struggle to recruit participants, but subjects also tend to leave or drop out of the study due to various reasons such as illness, relocation, or a lack of motivation to complete the full study.
This tendency is known as selective attrition and can threaten the validity of an experiment. For this reason, researchers using this approach typically recruit many participants, expecting a substantial number to drop out before the end.
Report bias is possible
Longitudinal studies will sometimes rely on surveys and questionnaires, which could result in inaccurate reporting as there is no way to verify the information presented.
- Data were collected for each child at three-time points: at 11 months after adoption, at 4.5 years of age and at 10.5 years of age. The first two sets of results showed that the adoptees were behind the non-institutionalised group however by 10.5 years old there was no difference between the two groups. The Romanian orphans had caught up with the children raised in normal Canadian families.
- The role of positive psychology constructs in predicting mental health and academic achievement in children and adolescents (Marques Pais-Ribeiro, & Lopez, 2011)
- The correlation between dieting behavior and the development of bulimia nervosa (Stice et al., 1998)
- The stress of educational bottlenecks negatively impacting students’ wellbeing (Cruwys, Greenaway, & Haslam, 2015)
- The effects of job insecurity on psychological health and withdrawal (Sidney & Schaufeli, 1995)
- The relationship between loneliness, health, and mortality in adults aged 50 years and over (Luo et al., 2012)
- The influence of parental attachment and parental control on early onset of alcohol consumption in adolescence (Van der Vorst et al., 2006)
- The relationship between religion and health outcomes in medical rehabilitation patients (Fitchett et al., 1999)
Goals of Longitudinal Data and Longitudinal Research
The objectives of longitudinal data collection and research as outlined by Baltes and Nesselroade (1979):
- Identify intraindividual change : Examine changes at the individual level over time, including long-term trends or short-term fluctuations. Requires multiple measurements and individual-level analysis.
- Identify interindividual differences in intraindividual change : Evaluate whether changes vary across individuals and relate that to other variables. Requires repeated measures for multiple individuals plus relevant covariates.
- Analyze interrelationships in change : Study how two or more processes unfold and influence each other over time. Requires longitudinal data on multiple variables and appropriate statistical models.
- Analyze causes of intraindividual change: This objective refers to identifying factors or mechanisms that explain changes within individuals over time. For example, a researcher might want to understand what drives a person’s mood fluctuations over days or weeks. Or what leads to systematic gains or losses in one’s cognitive abilities across the lifespan.
- Analyze causes of interindividual differences in intraindividual change : Identify mechanisms that explain within-person changes and differences in changes across people. Requires repeated data on outcomes and covariates for multiple individuals plus dynamic statistical models.
How to Perform a Longitudinal Study
When beginning to develop your longitudinal study, you must first decide if you want to collect your own data or use data that has already been gathered.
Using already collected data will save you time, but it will be more restricted and limited than collecting it yourself. When collecting your own data, you can choose to conduct either a retrospective or prospective study .
In a retrospective study, you are collecting data on events that have already occurred. You can examine historical information, such as medical records, in order to understand the past. In a prospective study, on the other hand, you are collecting data in real-time. Prospective studies are more common for psychology research.
Once you determine the type of longitudinal study you will conduct, you then must determine how, when, where, and on whom the data will be collected.
A standardized study design is vital for efficiently measuring a population. Once a study design is created, researchers must maintain the same study procedures over time to uphold the validity of the observation.
A schedule should be maintained, complete results should be recorded with each observation, and observer variability should be minimized.
Researchers must observe each subject under the same conditions to compare them. In this type of study design, each subject is the control.
Methodological Considerations
Important methodological considerations include testing measurement invariance of constructs across time, appropriately handling missing data, and using accelerated longitudinal designs that sample different age cohorts over overlapping time periods.
Testing measurement invariance
Testing measurement invariance involves evaluating whether the same construct is being measured in a consistent, comparable way across multiple time points in longitudinal research.
This includes assessing configural, metric, and scalar invariance through confirmatory factor analytic approaches. Ensuring invariance gives more confidence when drawing inferences about change over time.
Missing data
Missing data can occur during initial sampling if certain groups are underrepresented or fail to respond.
Attrition over time is the main source – participants dropping out for various reasons. The consequences of missing data are reduced statistical power and potential bias if dropout is nonrandom.
Handling missing data appropriately in longitudinal studies is critical to reducing bias and maintaining power.
It is important to minimize attrition by tracking participants, keeping contact info up to date, engaging them, and providing incentives over time.
Techniques like maximum likelihood estimation and multiple imputation are better alternatives to older methods like listwise deletion. Assumptions about missing data mechanisms (e.g., missing at random) shape the analytic approaches taken.
Accelerated longitudinal designs
Accelerated longitudinal designs purposefully create missing data across age groups.
Accelerated longitudinal designs strategically sample different age cohorts at overlapping periods. For example, assessing 6th, 7th, and 8th graders at yearly intervals would cover 6-8th grade development over a 3-year study rather than following a single cohort over that timespan.
This increases the speed and cost-efficiency of longitudinal data collection and enables the examination of age/cohort effects. Appropriate multilevel statistical models are required to analyze the resulting complex data structure.
In addition to those considerations, optimizing the time lags between measurements, maximizing participant retention, and thoughtfully selecting analysis models that align with the research questions and hypotheses are also vital in ensuring robust longitudinal research.
So, careful methodology is key throughout the design and analysis process when working with repeated-measures data.
Cohort effects
A cohort refers to a group born in the same year or time period. Cohort effects occur when different cohorts show differing trajectories over time.
Cohort effects can bias results if not accounted for, especially in accelerated longitudinal designs which assume cohort equivalence.
Detecting cohort effects is important but can be challenging as they are confounded with age and time of measurement effects.
Cohort effects can also interfere with estimating other effects like retest effects. This happens because comparing groups to estimate retest effects relies on cohort equivalence.
Overall, researchers need to test for and control cohort effects which could otherwise lead to invalid conclusions. Careful study design and analysis is required.
Retest effects
Retest effects refer to gains in performance that occur when the same or similar test is administered on multiple occasions.
For example, familiarity with test items and procedures may allow participants to improve their scores over repeated testing above and beyond any true change.
Specific examples include:
- Memory tests – Learning which items tend to be tested can artificially boost performance over time
- Cognitive tests – Becoming familiar with the testing format and particular test demands can inflate scores
- Survey measures – Remembering previous responses can bias future responses over multiple administrations
- Interviews – Comfort with the interviewer and process can lead to increased openness or recall
To estimate retest effects, performance of retested groups is compared to groups taking the test for the first time. Any divergence suggests inflated scores due to retesting rather than true change.
If unchecked in analysis, retest gains can be confused with genuine intraindividual change or interindividual differences.
This undermines the validity of longitudinal findings. Thus, testing and controlling for retest effects are important considerations in longitudinal research.
Data Analysis
Longitudinal data involves repeated assessments of variables over time, allowing researchers to study stability and change. A variety of statistical models can be used to analyze longitudinal data, including latent growth curve models, multilevel models, latent state-trait models, and more.
Latent growth curve models allow researchers to model intraindividual change over time. For example, one could estimate parameters related to individuals’ baseline levels on some measure, linear or nonlinear trajectory of change over time, and variability around those growth parameters. These models require multiple waves of longitudinal data to estimate.
Multilevel models are useful for hierarchically structured longitudinal data, with lower-level observations (e.g., repeated measures) nested within higher-level units (e.g., individuals). They can model variability both within and between individuals over time.
Latent state-trait models decompose the covariance between longitudinal measurements into time-invariant trait factors, time-specific state residuals, and error variance. This allows separating stable between-person differences from within-person fluctuations.
There are many other techniques like latent transition analysis, event history analysis, and time series models that have specialized uses for particular research questions with longitudinal data. The choice of model depends on the hypotheses, timescale of measurements, age range covered, and other factors.
In general, these various statistical models allow investigation of important questions about developmental processes, change and stability over time, causal sequencing, and both between- and within-person sources of variability. However, researchers must carefully consider the assumptions behind the models they choose.
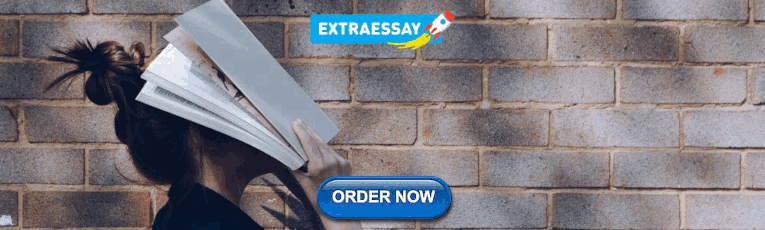
Longitudinal vs. Cross-Sectional Studies
Longitudinal studies and cross-sectional studies are two different observational study designs where researchers analyze a target population without manipulating or altering the natural environment in which the participants exist.
Yet, there are apparent differences between these two forms of study. One key difference is that longitudinal studies follow the same sample of people over an extended period of time, while cross-sectional studies look at the characteristics of different populations at a given moment in time.
Longitudinal studies tend to require more time and resources, but they can be used to detect cause-and-effect relationships and establish patterns among subjects.
On the other hand, cross-sectional studies tend to be cheaper and quicker but can only provide a snapshot of a point in time and thus cannot identify cause-and-effect relationships.
Both studies are valuable for psychologists to observe a given group of subjects. Still, cross-sectional studies are more beneficial for establishing associations between variables, while longitudinal studies are necessary for examining a sequence of events.
1. Are longitudinal studies qualitative or quantitative?
Longitudinal studies are typically quantitative. They collect numerical data from the same subjects to track changes and identify trends or patterns.
However, they can also include qualitative elements, such as interviews or observations, to provide a more in-depth understanding of the studied phenomena.
2. What’s the difference between a longitudinal and case-control study?
Case-control studies compare groups retrospectively and cannot be used to calculate relative risk. Longitudinal studies, though, can compare groups either retrospectively or prospectively.
In case-control studies, researchers study one group of people who have developed a particular condition and compare them to a sample without the disease.
Case-control studies look at a single subject or a single case, whereas longitudinal studies are conducted on a large group of subjects.
3. Does a longitudinal study have a control group?
Yes, a longitudinal study can have a control group . In such a design, one group (the experimental group) would receive treatment or intervention, while the other group (the control group) would not.
Both groups would then be observed over time to see if there are differences in outcomes, which could suggest an effect of the treatment or intervention.
However, not all longitudinal studies have a control group, especially observational ones and not testing a specific intervention.
Baltes, P. B., & Nesselroade, J. R. (1979). History and rationale of longitudinal research. In J. R. Nesselroade & P. B. Baltes (Eds.), (pp. 1–39). Academic Press.
Cook, N. R., & Ware, J. H. (1983). Design and analysis methods for longitudinal research. Annual review of public health , 4, 1–23.
Fitchett, G., Rybarczyk, B., Demarco, G., & Nicholas, J.J. (1999). The role of religion in medical rehabilitation outcomes: A longitudinal study. Rehabilitation Psychology, 44, 333-353.
Harvard Second Generation Study. (n.d.). Harvard Second Generation Grant and Glueck Study. Harvard Study of Adult Development. Retrieved from https://www.adultdevelopmentstudy.org.
Le Mare, L., & Audet, K. (2006). A longitudinal study of the physical growth and health of postinstitutionalized Romanian adoptees. Pediatrics & child health, 11 (2), 85-91.
Luo, Y., Hawkley, L. C., Waite, L. J., & Cacioppo, J. T. (2012). Loneliness, health, and mortality in old age: a national longitudinal study. Social science & medicine (1982), 74 (6), 907–914.
Marques, S. C., Pais-Ribeiro, J. L., & Lopez, S. J. (2011). The role of positive psychology constructs in predicting mental health and academic achievement in children and adolescents: A two-year longitudinal study. Journal of Happiness Studies: An Interdisciplinary Forum on Subjective Well-Being, 12( 6), 1049–1062.
Sidney W.A. Dekker & Wilmar B. Schaufeli (1995) The effects of job insecurity on psychological health and withdrawal: A longitudinal study, Australian Psychologist, 30: 1,57-63.
Stice, E., Mazotti, L., Krebs, M., & Martin, S. (1998). Predictors of adolescent dieting behaviors: A longitudinal study. Psychology of Addictive Behaviors, 12 (3), 195–205.
Tegan Cruwys, Katharine H Greenaway & S Alexander Haslam (2015) The Stress of Passing Through an Educational Bottleneck: A Longitudinal Study of Psychology Honours Students, Australian Psychologist, 50:5, 372-381.
Thomas, L. (2020). What is a longitudinal study? Scribbr. Retrieved from https://www.scribbr.com/methodology/longitudinal-study/
Van der Vorst, H., Engels, R. C. M. E., Meeus, W., & Deković, M. (2006). Parental attachment, parental control, and early development of alcohol use: A longitudinal study. Psychology of Addictive Behaviors, 20 (2), 107–116.
Further Information
- Schaie, K. W. (2005). What can we learn from longitudinal studies of adult development?. Research in human development, 2 (3), 133-158.
- Caruana, E. J., Roman, M., Hernández-Sánchez, J., & Solli, P. (2015). Longitudinal studies. Journal of thoracic disease, 7 (11), E537.

Related Articles
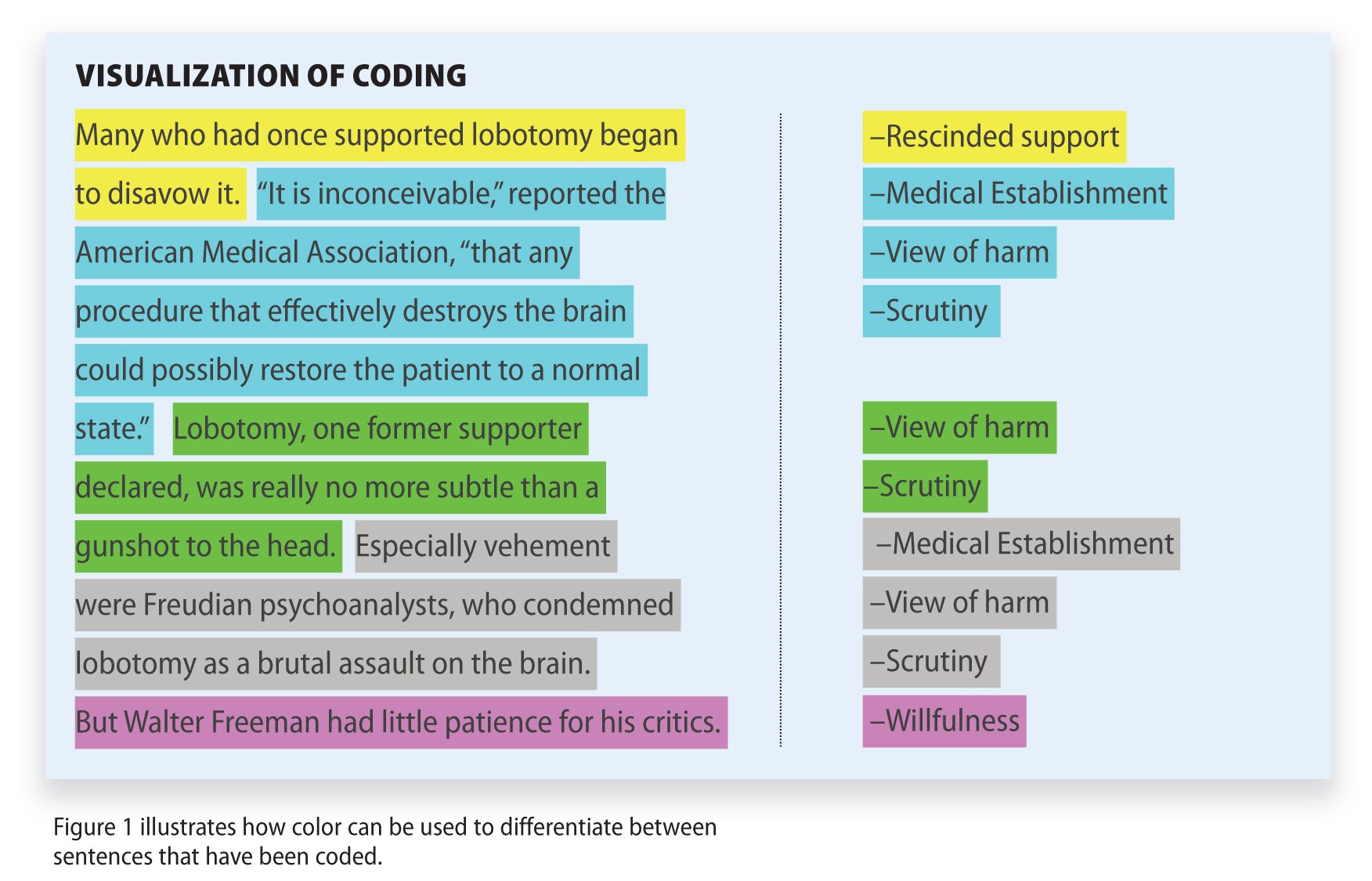
Research Methodology
Qualitative Data Coding
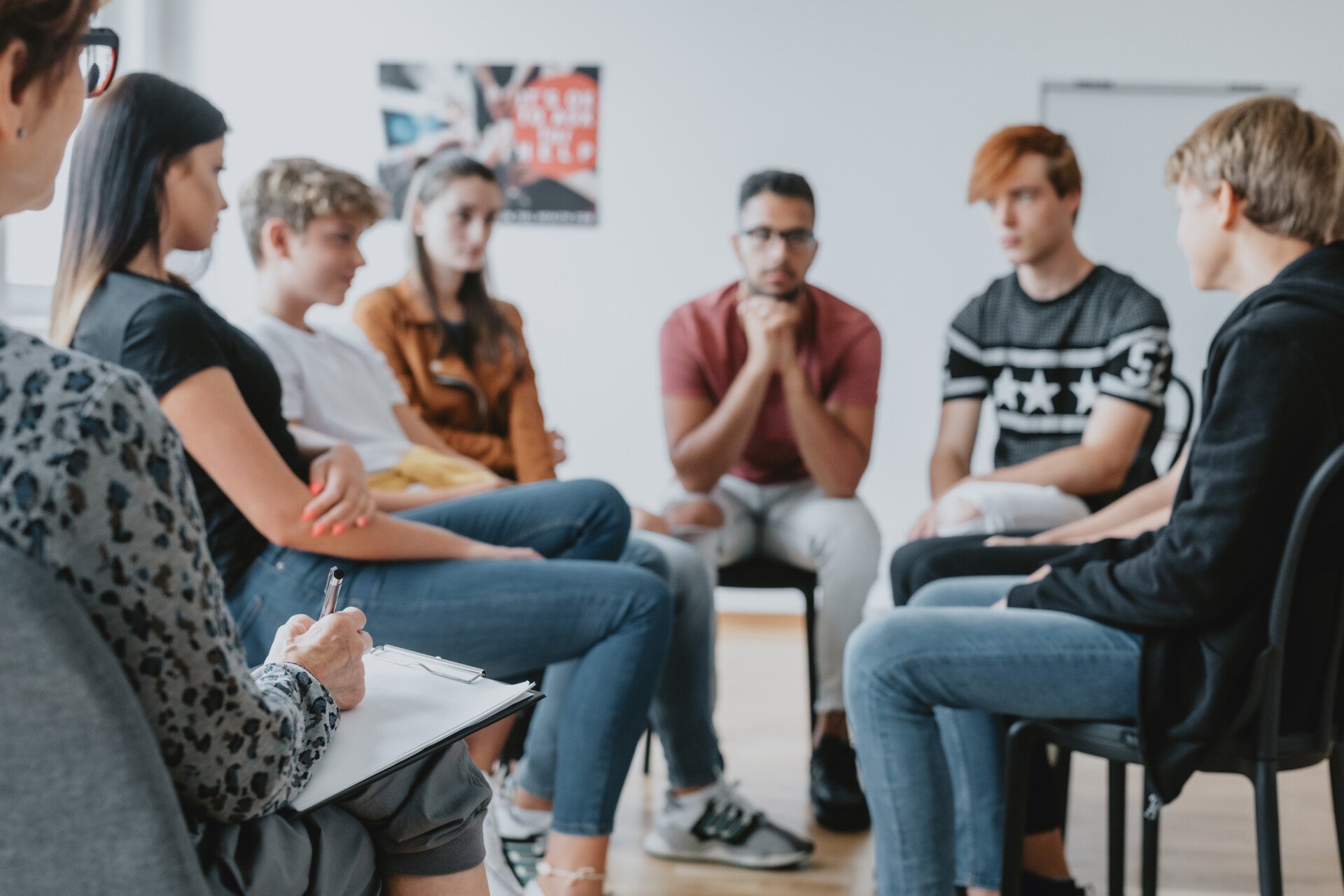
What Is a Focus Group?
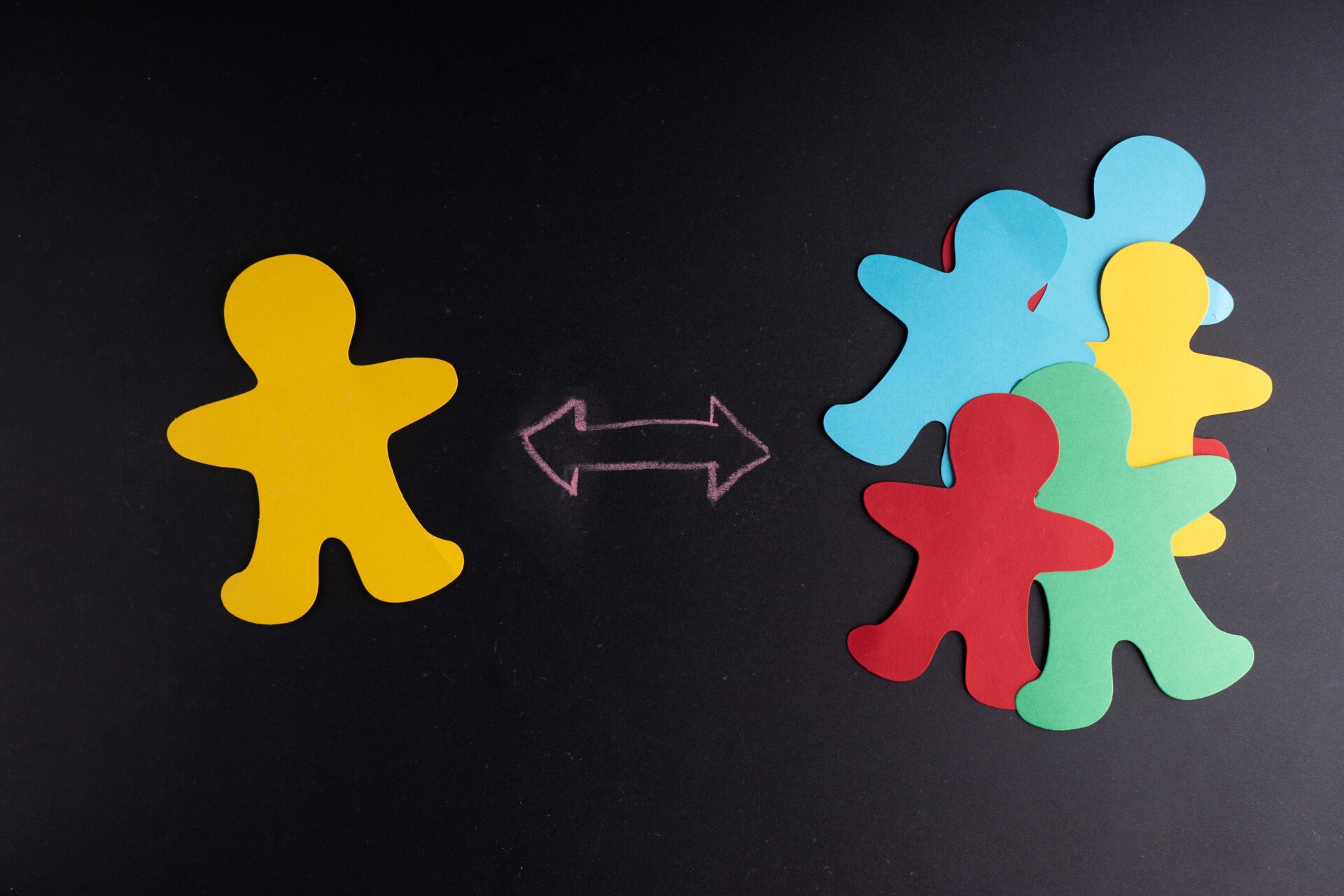
Cross-Cultural Research Methodology In Psychology
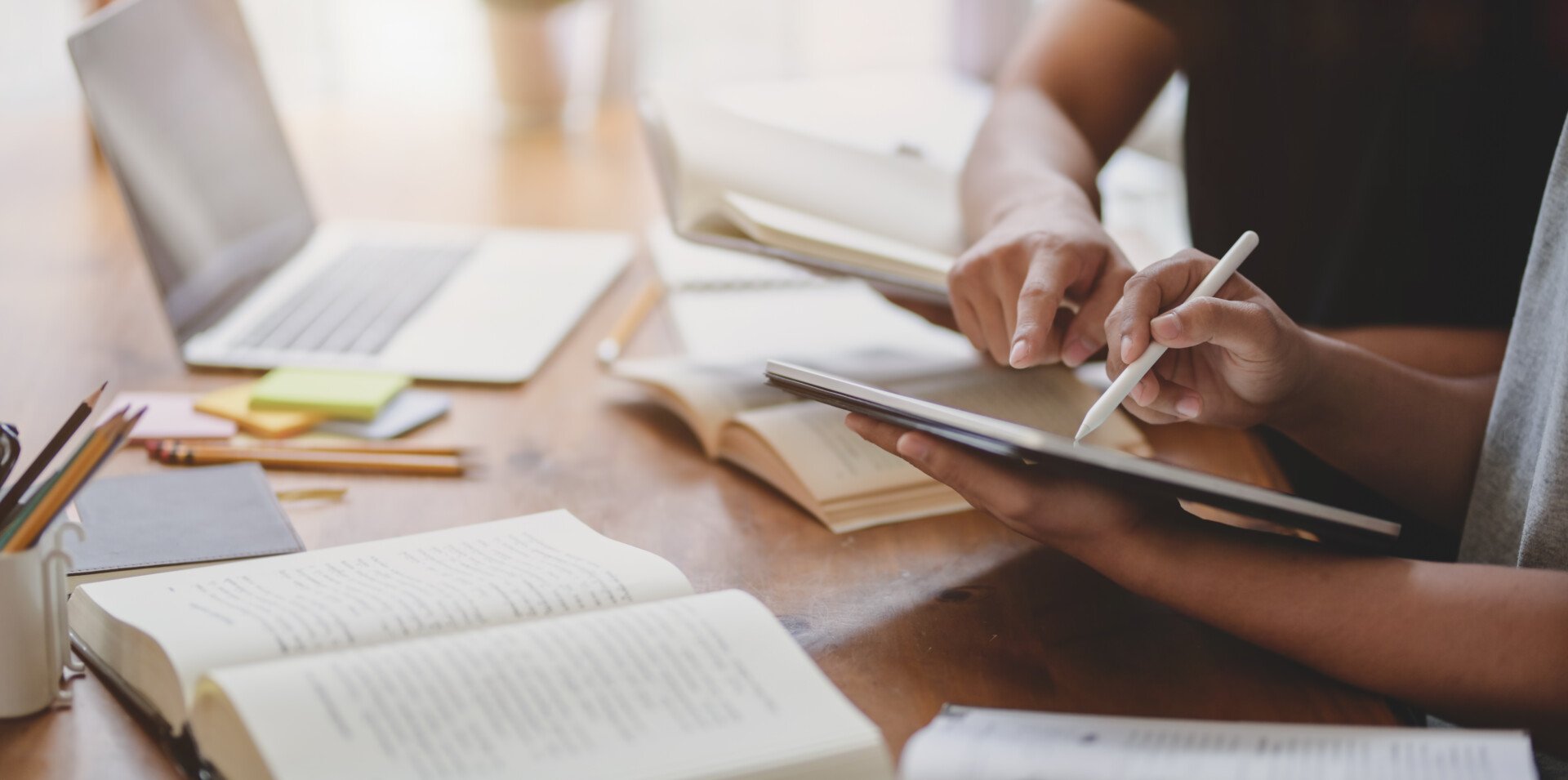
What Is Internal Validity In Research?
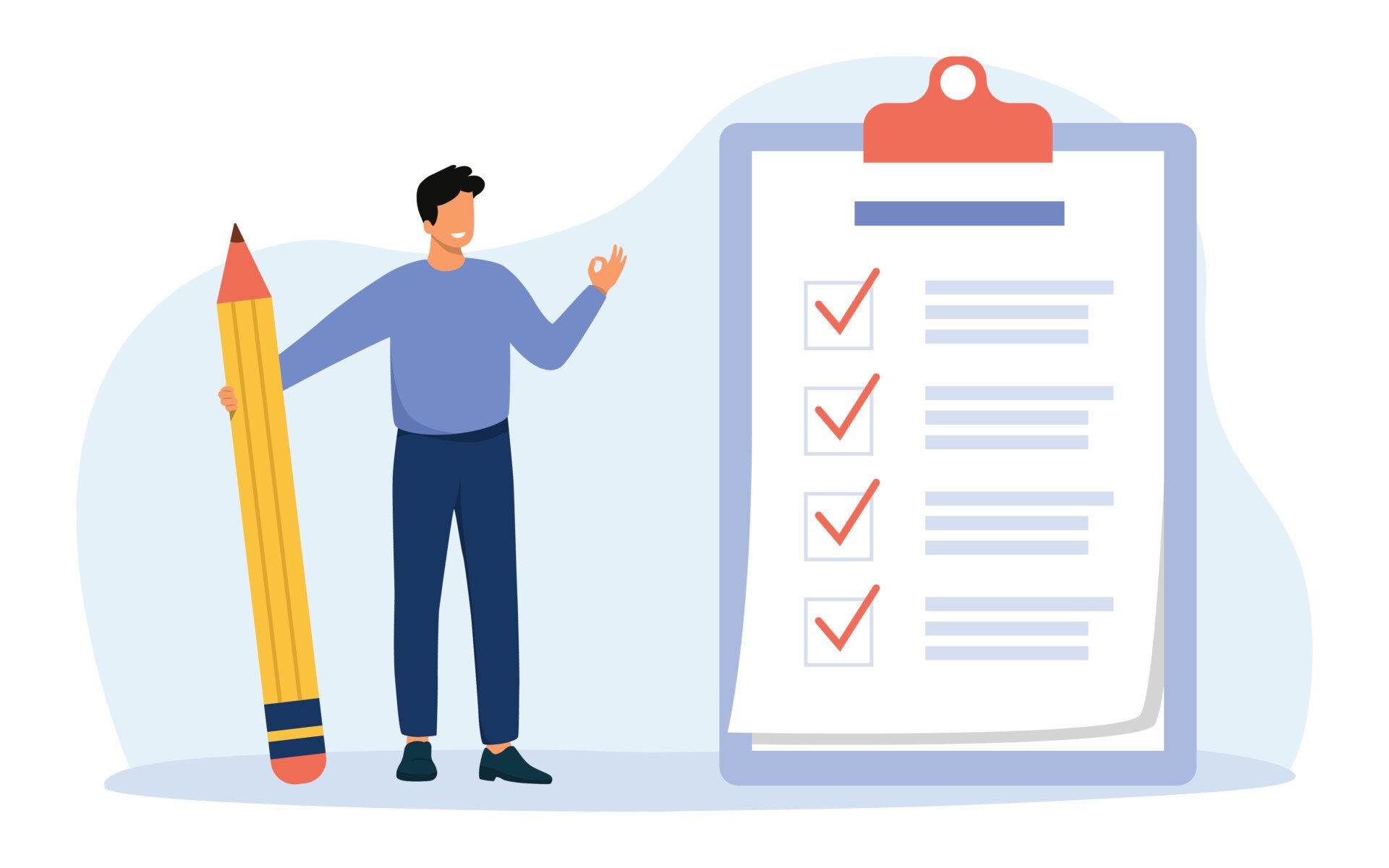
Research Methodology , Statistics
What Is Face Validity In Research? Importance & How To Measure
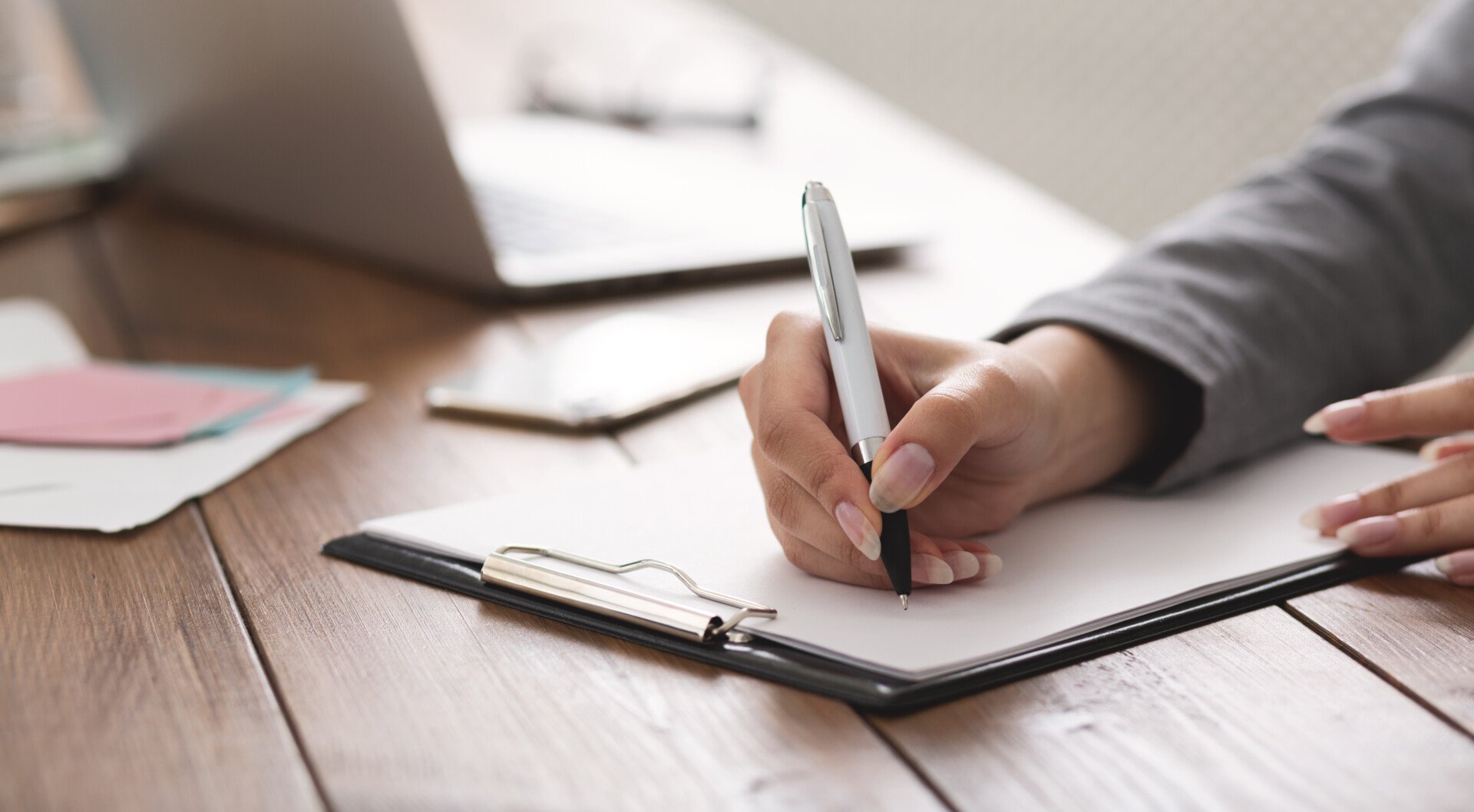
Criterion Validity: Definition & Examples
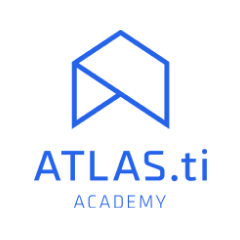
Longitudinal Studies: Methods, Benefits and Challenges
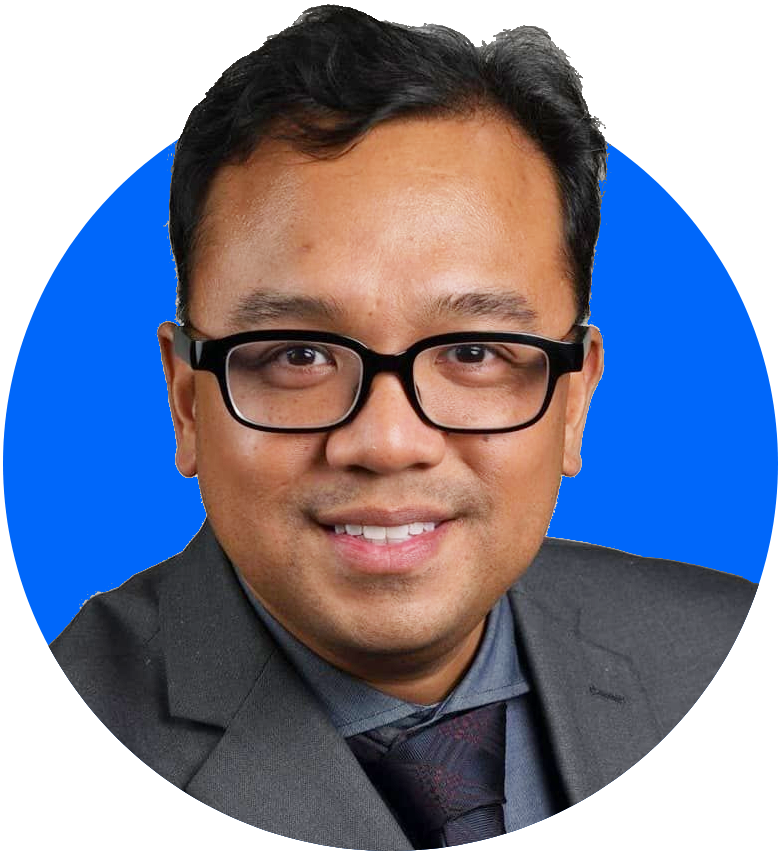
Introduction
What is a longitudinal study, what are examples of longitudinal studies, longitudinal studies vs. cross-sectional studies, benefits of longitudinal studies, types of longitudinal studies, how do you conduct a longitudinal study, challenges of longitudinal research.
Longitudinal research refers to any study that collects the same sample of data from the same group of people at different points in time. While time-consuming and potentially costly in terms of resources and effort, a longitudinal study has enormous utility in understanding complex phenomena that might change as time passes.
In this article, we will explore the nature and importance of longitudinal studies to allow you to decide whether your research inquiry warrants a longitudinal inquiry or if a cross-sectional study is more appropriate.
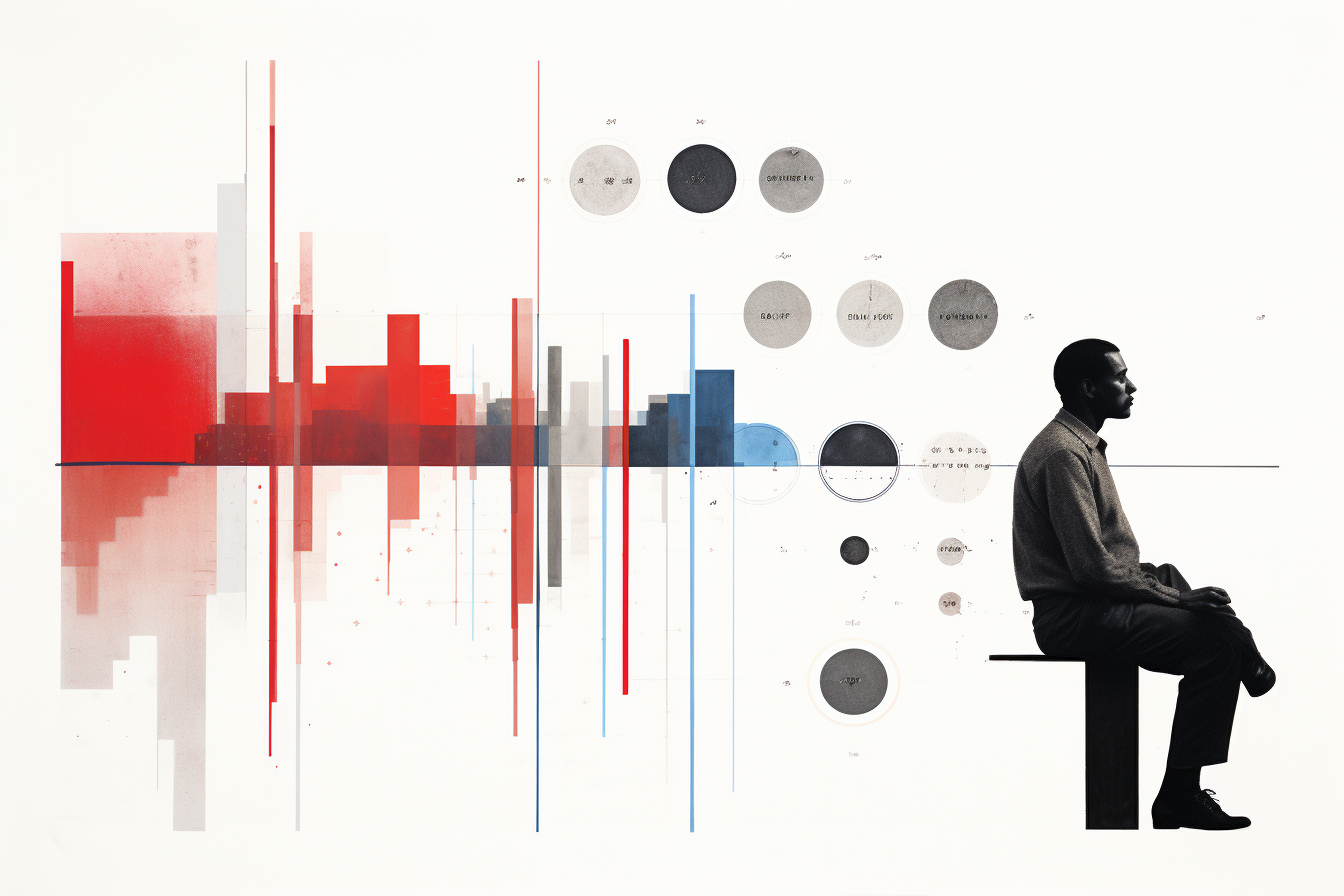
To understand a longitudinal study, let's start with a simple survey as an example. Determining the popularity of a particular product or service at a specific point in time can simply be a matter of collecting and analyzing survey responses from a certain number of people within a population. The qualitative and quantitative data collected from these surveys can tell you what people think at the moment those surveys were conducted. This is what is known as a cross-sectional study .
Now imagine the product that you're trying to assess is seasonal like a brand of ice cream or hot chocolate. What's popular in summer may not be popular in winter, and trends come and go as competing products enter the market. In this context, the one survey that was conducted is merely a snapshot of a moving phenomenon at a single point in time.
In a longitudinal study design, that same survey will be distributed to the same group of people at different time intervals (e.g., twice a year or once a month) to allow researchers to see if there are any changes. Perhaps there is an ice cream that is as popular in the winter as it is in the summer, which may be worth identifying to expand profitability. A longitudinal study would thus be useful to explore this question.
Longitudinal research isn't conducted simply for the sake of being able to say research was conducted over a extended period of time. A longitudinal analysis collects data at different points in time to observe changes in the characteristics of the object of inquiry. Ultimately, collecting data for a longitudinal study can help identify cause-and-effect relationships that cannot otherwise be perceived in discrete or cross-sectional studies.
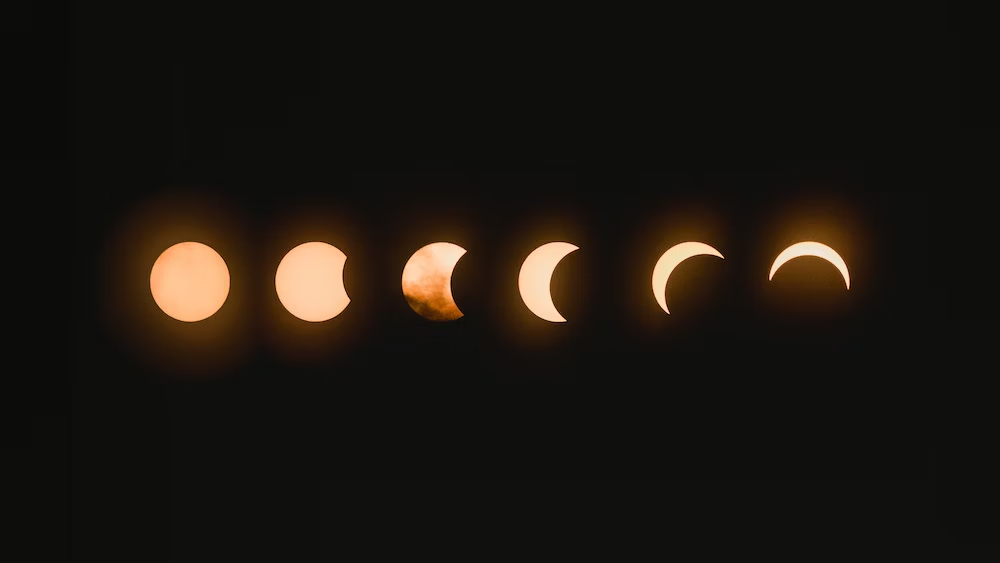
Longitudinal studies are found in many research fields where time is an important factor. Let's look at examples in three different research areas.
Classroom research is often longitudinal because of the acknowledgment that successful learning takes place over time and not merely in a single class session. Such studies take place over several classes, perhaps over a semester or an entire academic year. A researcher might observe the same group of students as they progress academically or, conversely, identify any significant decline in learning outcomes to determine how changes in teaching and learning over time might affect student development.
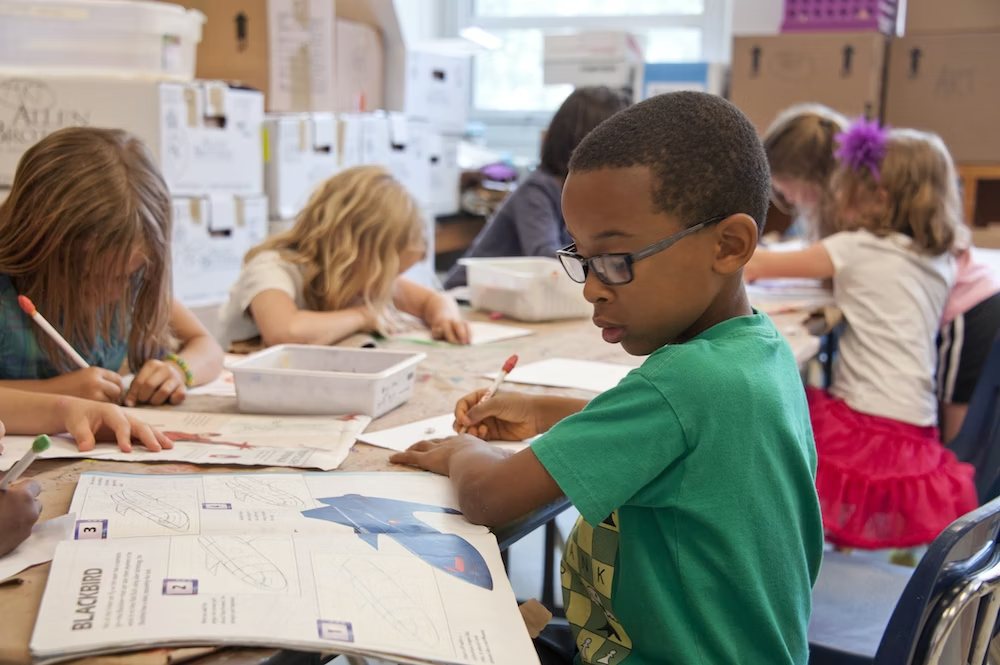
Health sciences
Medical research often relies on longitudinal studies to determine the effectiveness and risk factors involved with drugs, treatments, or other medical remedies. Consider a dietary supplement that is purported to help people lose weight. Perhaps, in the beginning, people who take this supplement actually do lose weight. But what happens later on? Do they keep the weight off, gain it back or, even worse, gain even more weight in the long term? A longitudinal study can help researchers determine if that supplement produces sustainable results or is merely a quick fix that has negative side effects later on.
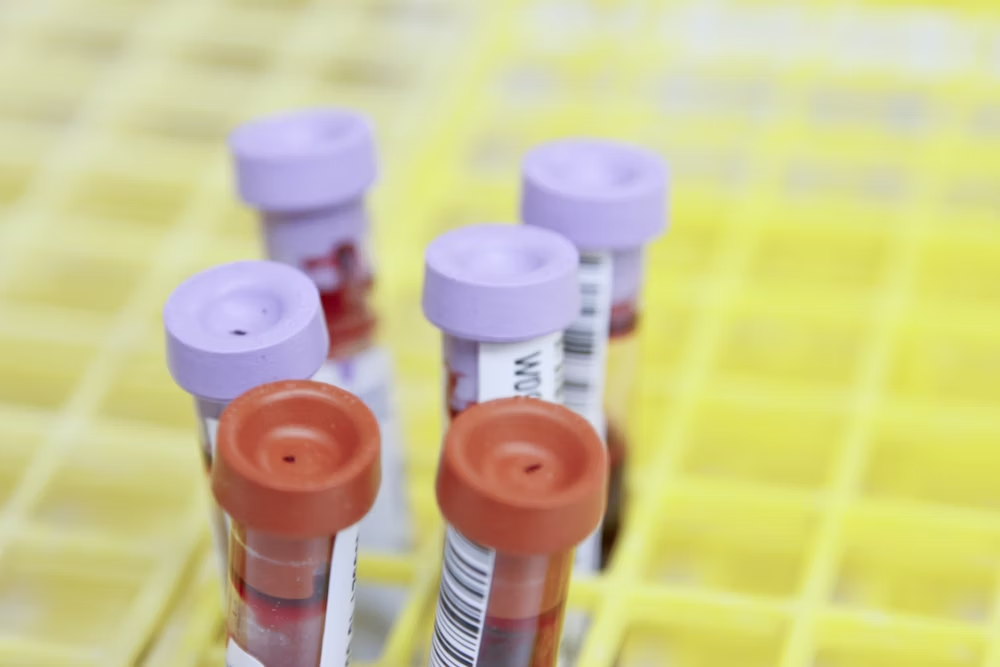
Product life cycles and market trends can take extended periods of time to manifest. In the meantime, competing products might enter the market and consequently affect customer loyalty and product image. If a cross-sectional study captures a snapshot of opinions in the marketplace, then think of a longitudinal study as several snapshots spread out over time to allow researchers to observe changes in market behavior and their underlying causes as time passes.
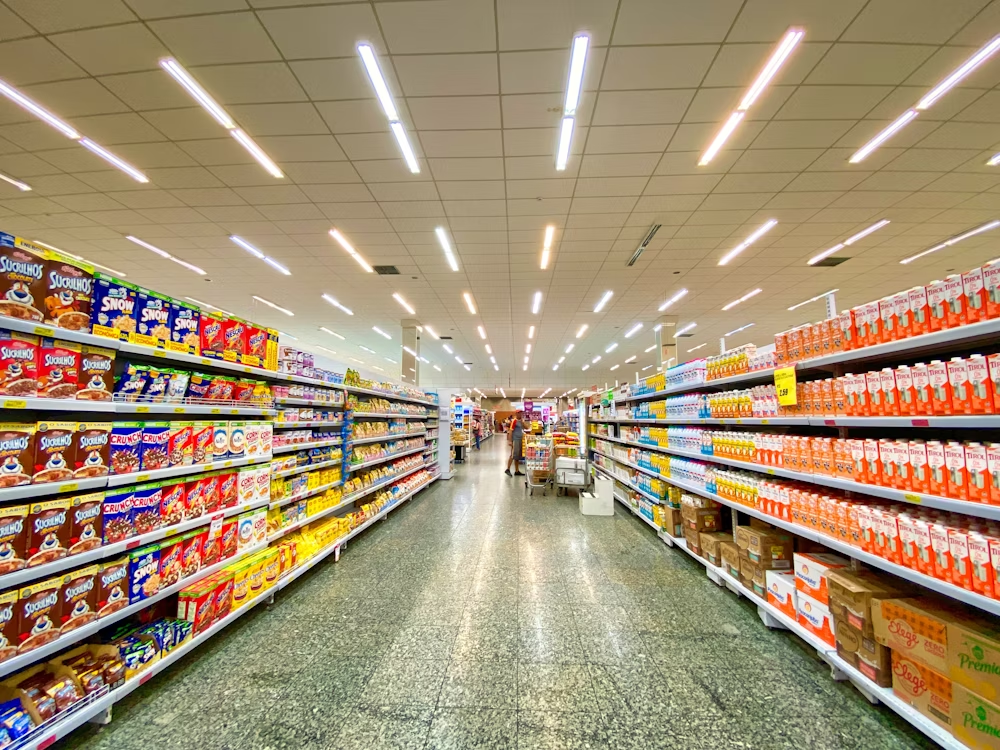
Cross-sectional studies are discrete studies that capture data within a particular context at a particular point in time. These kinds of studies are more appropriate for research inquiries that don't examine some form of development or evolution, such as concepts or phenomena that are generally static or unchanging over extended periods of time.
To determine which type of study would be more appropriate for your research inquiry, it's important to identify the object of inquiry that is being studied. Ask yourself the following questions when planning your study:
- Do you need an extended period of time to sufficiently capture the phenomenon?
- Is the sample of data collected likely to change over time?
- Is it feasible to commit time and resources to an extended study?
If you said yes to all of these questions, a longitudinal study would be suited to addressing your research questions . Otherwise, cross-sectional studies may be more appropriate for your research.
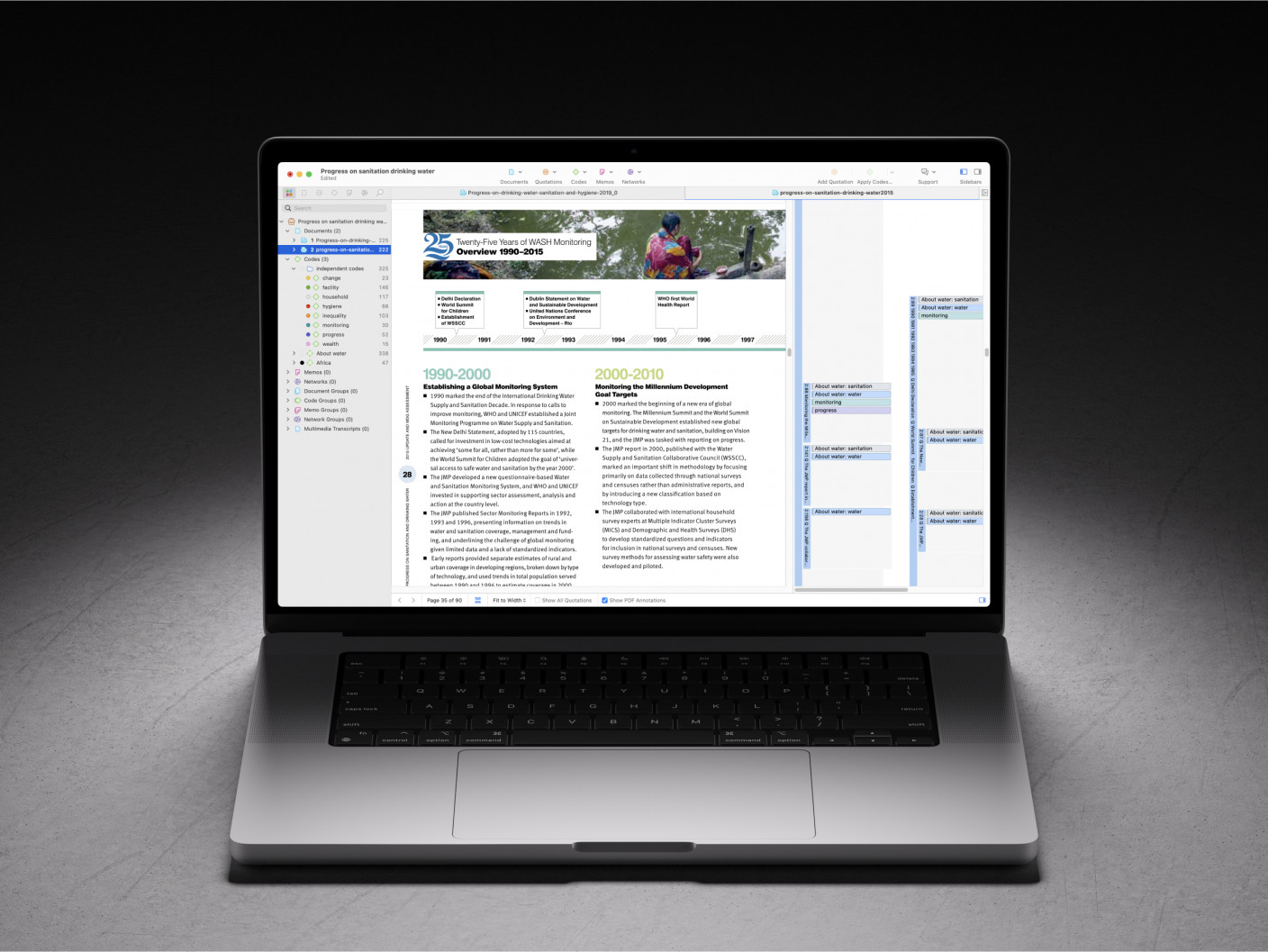
Intuitive tools, powerful analysis with ATLAS.ti
Make the most of your research with our easy-to-use data analysis platform. Download a free trial today.
A longitudinal study can provide many benefits potentially relevant to the research question you are looking to address. Here are three different advantages you might consider.
Abundance of data
In many cases, research rigor is served by collecting abundant data . Research approaches like thematic analysis and content analysis benefit from a large set of data that helps you identify the most frequently occurring phenomena within a research context. Large data sets collected through longitudinal studies can be useful for separating abundance from anecdotes.
Identification of patterns
Analyzing patterns often implies exploring how things interact sequentially or over time, which is best captured with longitudinal data. Think about, for example, how sports competitions and political elections take place over a year or even multiple years. Construction of ships and buildings can be a long and protracted process. Doctoral students can spend four or more years before earning their degree. A simple cross-sectional study in such contexts may not gather sufficient data captured over a period of time long enough to observe sequences of related events.
Observation of relationships
Certain relationships between different phenomena can only be observed longitudinally. The famous marshmallow test that asserted connections between behaviors in childhood and later life outcomes spawned decades of longitudinal study. Even if your research is much simpler, your research question might involve the observation of distant but related phenomena that only a longitudinal study can capture.
There are two types of longitudinal studies to choose from, primarily depending on what you are looking to examine. Keep in mind that longitudinal study design, no matter what type of study you might pursue, is a matter of sustaining a research inquiry over time to capture the necessary data. It's important that your decision-making process is both transparent and intentional for the sake of research rigor.
Cohort studies
A cohort study examines a group of people that share a common trait. This trait could be a similar age group, a common level of education, or a shared experience.
An example of a cohort study is one that looks to identify factors related to successful aging found in lifestyles among people of middle age. Such a study could observe a group of people, all of whom are similar in age, to identify a common range of lifestyles and activities that are applicable for others of the same age group.
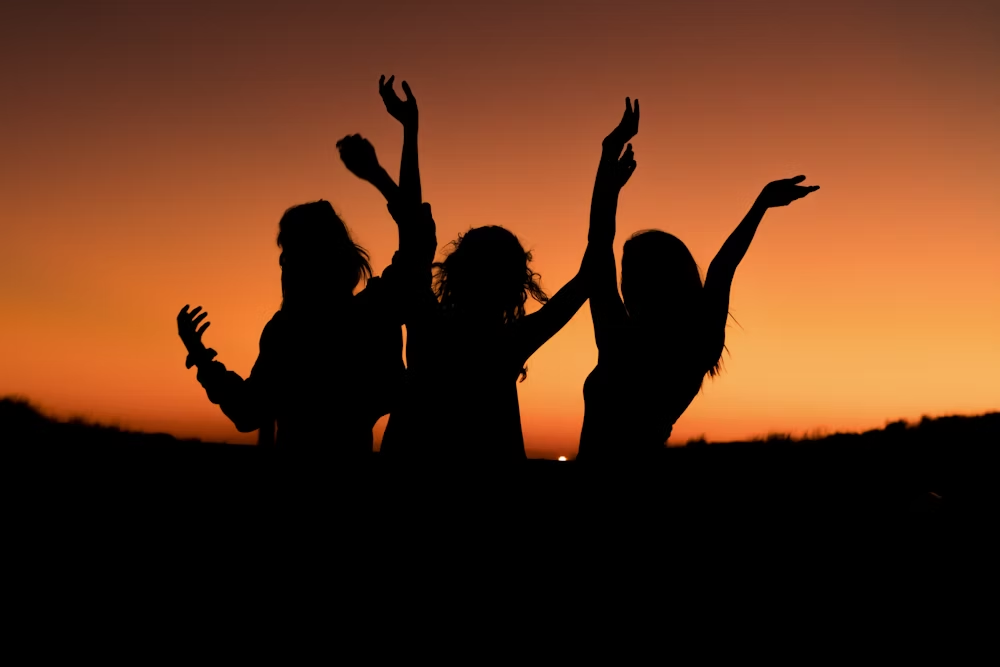
Panel studies
The difference between a cohort study and a panel study is that panel studies collect data from within a general population, rather than a specific set of particular individuals with a common characteristic. The goal of a panel study is to examine a representative sample of a larger population rather than a specific subset of people.
A longitudinal survey that adopts a panel study model, for example, would randomly sample a population and send out questionnaires to the same sample of people over time. Such a survey could look at changes in everyday habits regarding spending or work-life balance and how they might be influenced by environmental or economic shifts from one period of time to the next.
Planning a prospective or future research study that is longitudinal requires careful attention to detail prior to conducting the study. By itself, a longitudinal study can be considered a repeated sequence of the same discrete study across different periods of time.
However, ensuring that multiple iterations of the same study are conducted repeatedly and rigorously is the challenge in longitudinal studies. With that in mind, let's look at some of the different research methods that might be employed in longitudinal research.
Observational research
Action research and ethnographies rely on longitudinal observations to provide sufficient depth to the cultural practices and interactions that are under study. In anthropological and sociological research, some phenomena are so complex or dynamic that they can only be observed longitudinally.
Organizational research, for example, employs longitudinal research to identify how people in the workplace or other similar settings interact with each other. This kind of research is useful for understanding how rapport is established and whether productivity increases as a result.
A longitudinal survey can address research questions that deal with opinions and perspectives that may change over time. Unlike a cross-sectional survey from a particular point in time, longitudinal surveys are administered repeatedly to the same group of people to collect data on changes or developments.
A personal wellness study, for example, might examine how healthy habits (or the lack thereof) affect health by asking respondents questions about their daily routine. By comparing their routines over time with information such as blood pressure, weight, and waist size, survey data on lifestyle routines can allow researchers to identify what habits can cause the greatest improvement in individual health.
Experiments
Various experimental studies, especially in medical research, can be longitudinal in nature. A longitudinal experiment usually collects data from a control group and an experimental group to observe the effects of a certain treatment on the same participants over a period of time.
This type of research is commonly employed to examine the effects of medical treatments on outcomes such as cardiovascular disease or diabetes. The requirements for governmental approval are incredibly stringent and call for rigorous data collection that establishes causality.
Needless to say, longitudinal studies tend to be time-consuming. The most obvious drawback of longitudinal studies is that they take up a significant portion of researchers' time and effort.
However, there are other disadvantages of longitudinal studies, particularly the likelihood of participant attrition. In other words, the more lengthy the study, the more likely it is that participants may drop out of the study. This is especially true when working with vulnerable or marginalized populations such as migrant workers or homeless people, populations that may not always be easy to contact for collecting data.
Over the course of time, the research context that a researcher studies may change with the appearance of new technologies, trends, or other developments that may not have been anticipated. While confounding influences are possible in any study, they are likely to be more abundant in studies on a longitudinal scale. As a result, it's important for the researcher to try to account for these influences when analyzing the data . It could even be worthwhile to examine how the appearance of that phenomenon or concept impacted a relevant outcome of interest in your area.
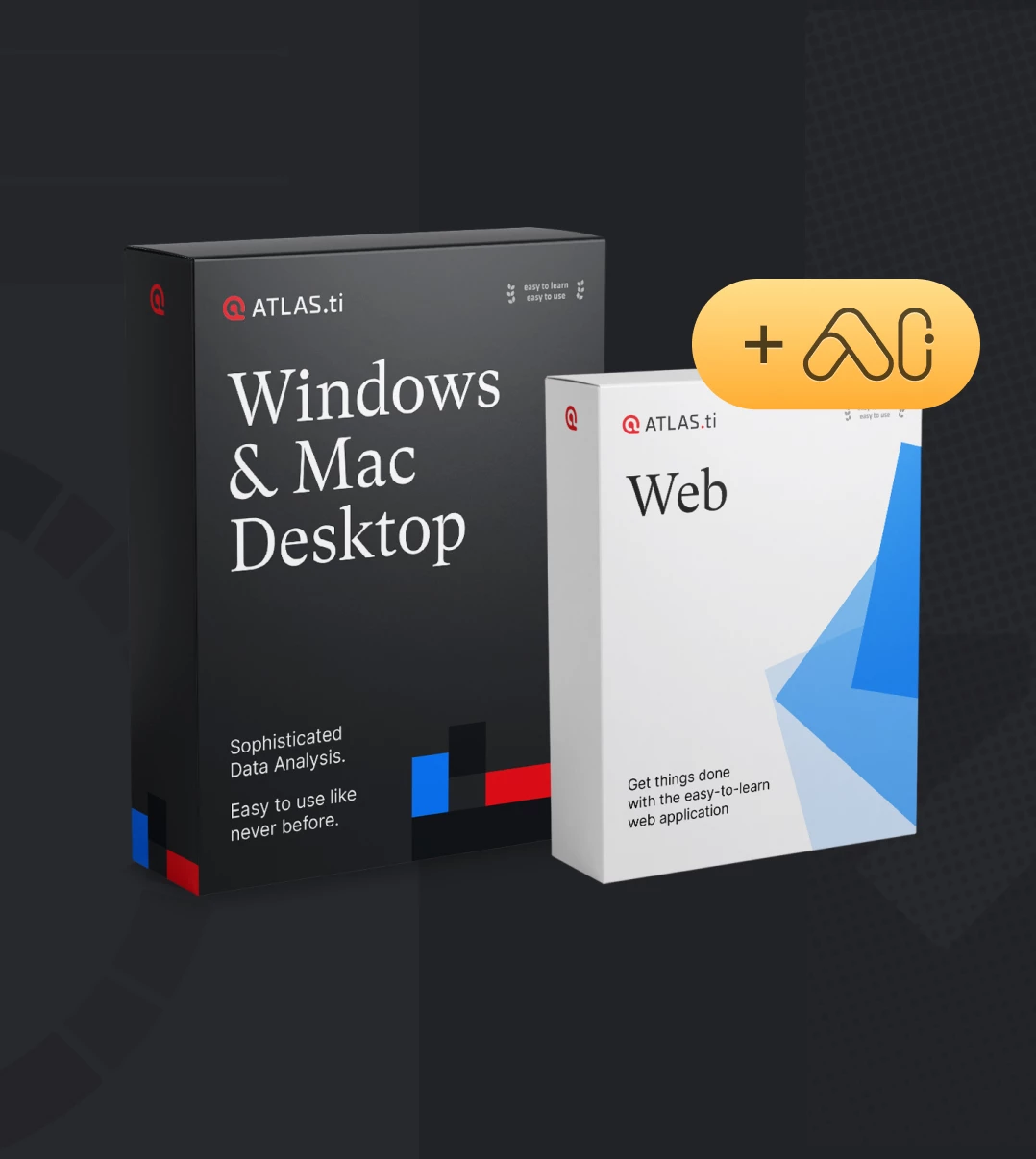
Turn your research into critical insights with ATLAS.ti
Powerful tools to make the most of your data are just a click away. Download a free trial of ATLAS.ti.
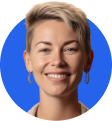
- Skip to main content
- Skip to primary sidebar
- Skip to footer
- QuestionPro

- Solutions Industries Gaming Automotive Sports and events Education Government Travel & Hospitality Financial Services Healthcare Cannabis Technology Use Case NPS+ Communities Audience Contactless surveys Mobile LivePolls Member Experience GDPR Positive People Science 360 Feedback Surveys
- Resources Blog eBooks Survey Templates Case Studies Training Help center

Home Market Research
What is a Longitudinal Study?: Definition and Explanation
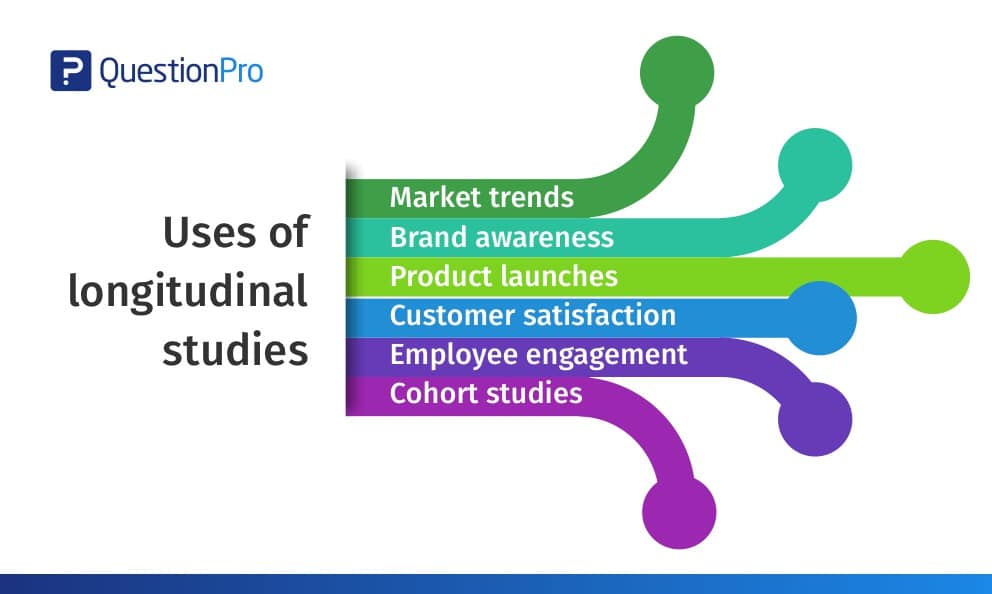
In this article, we’ll cover all you need to know about longitudinal research.
Let’s take a closer look at the defining characteristics of longitudinal studies, review the pros and cons of this type of research, and share some useful longitudinal study examples.
Content Index
What is a longitudinal study?
Types of longitudinal studies, advantages and disadvantages of conducting longitudinal surveys.
- Longitudinal studies vs. cross-sectional studies
Types of surveys that use a longitudinal study
Longitudinal study examples.
A longitudinal study is a research conducted over an extended period of time. It is mostly used in medical research and other areas like psychology or sociology.
When using this method, a longitudinal survey can pay off with actionable insights when you have the time to engage in a long-term research project.
Longitudinal studies often use surveys to collect data that is either qualitative or quantitative. Additionally, in a longitudinal study, a survey creator does not interfere with survey participants. Instead, the survey creator distributes questionnaires over time to observe changes in participants, behaviors, or attitudes.
Many medical studies are longitudinal; researchers note and collect data from the same subjects over what can be many years.
LEARN ABOUT: Action Research
Longitudinal studies are versatile, repeatable, and able to account for quantitative and qualitative data . Consider the three major types of longitudinal studies for future research:
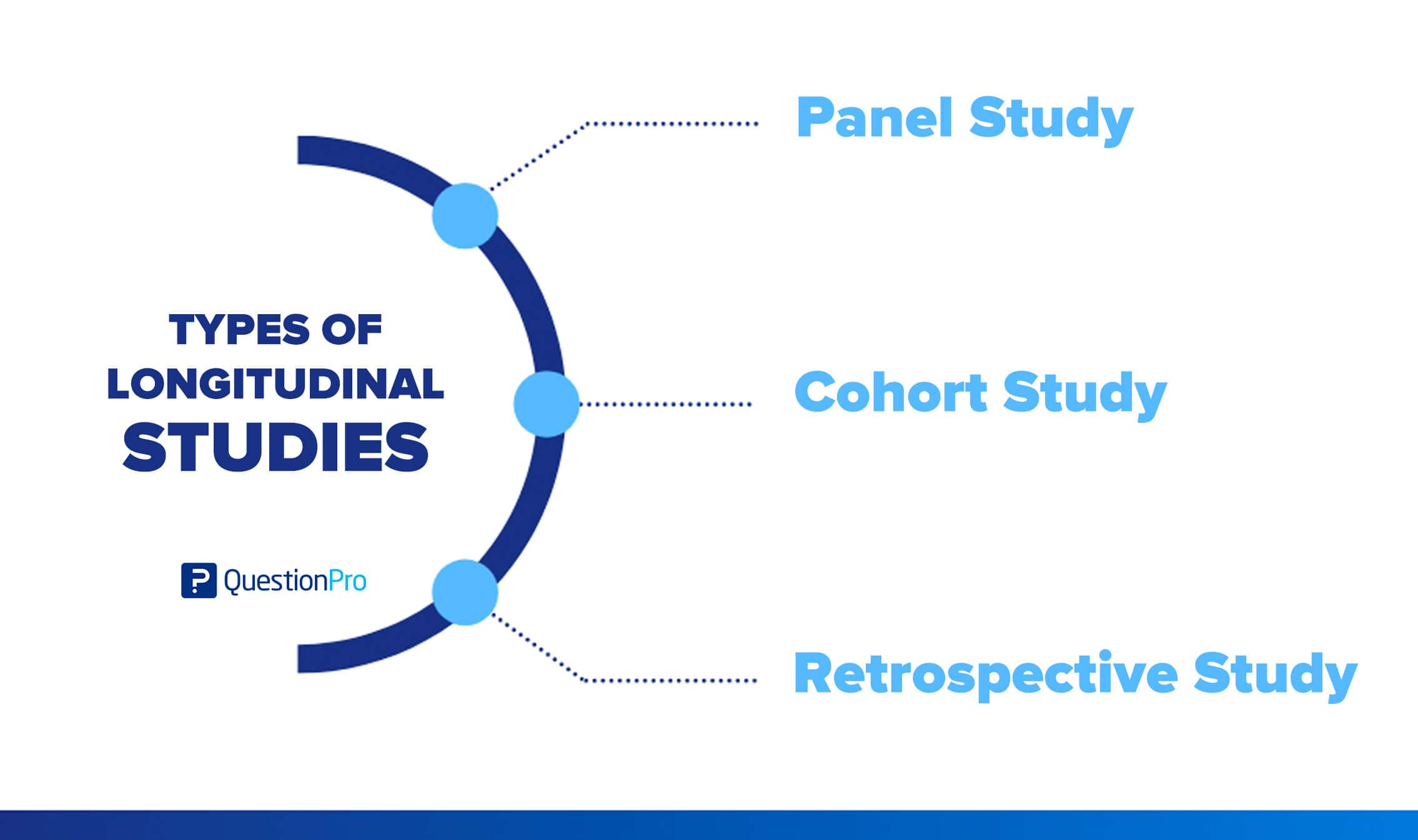
Panel study: A panel survey involves a sample of people from a more significant population and is conducted at specified intervals for a more extended period.
One of the panel study’s essential features is that researchers collect data from the same sample at different points in time. Most panel studies are designed for quantitative analysis , though they may also be used to collect qualitative data and unit of analysis .
LEARN ABOUT: Level of Analysis
Cohort Study: A cohort study samples a cohort (a group of people who typically experience the same event at a given point in time). Medical researchers tend to conduct cohort studies. Some might consider clinical trials similar to cohort studies.
In cohort studies, researchers merely observe participants without intervention, unlike clinical trials in which participants undergo tests.
Retrospective study: A retrospective study uses already existing data, collected during previously conducted research with similar methodology and variables.
While doing a retrospective study, the researcher uses an administrative database, pre-existing medical records, or one-to-one interviews.
As we’ve demonstrated, a longitudinal study is useful in science, medicine, and many other fields. There are many reasons why a researcher might want to conduct a longitudinal study. One of the essential reasons is, longitudinal studies give unique insights that many other types of research fail to provide.
Advantages of longitudinal studies
- Greater validation: For a long-term study to be successful, objectives and rules must be established from the beginning. As it is a long-term study, its authenticity is verified in advance, which makes the results have a high level of validity.
- Unique data: Most research studies collect short-term data to determine the cause and effect of what is being investigated. Longitudinal surveys follow the same principles but the data collection period is different. Long-term relationships cannot be discovered in a short-term investigation, but short-term relationships can be monitored in a long-term investigation.
- Allow identifying trends: Whether in medicine, psychology, or sociology, the long-term design of a longitudinal study enables trends and relationships to be found within the data collected in real time. The previous data can be applied to know future results and have great discoveries.
- Longitudinal surveys are flexible: Although a longitudinal study can be created to study a specific data point, the data collected can show unforeseen patterns or relationships that can be significant. Because this is a long-term study, the researchers have a flexibility that is not possible with other research formats.
Additional data points can be collected to study unexpected findings, allowing changes to be made to the survey based on the approach that is detected.
Disadvantages of longitudinal studies
- Research time The main disadvantage of longitudinal surveys is that long-term research is more likely to give unpredictable results. For example, if the same person is not found to update the study, the research cannot be carried out. It may also take several years before the data begins to produce observable patterns or relationships that can be monitored.
- An unpredictability factor is always present It must be taken into account that the initial sample can be lost over time. Because longitudinal studies involve the same subjects over a long period of time, what happens to them outside of data collection times can influence the data that is collected in the future. Some people may decide to stop participating in the research. Others may not be in the correct demographics for research. If these factors are not included in the initial research design, they could affect the findings that are generated.
- Large samples are needed for the investigation to be meaningful To develop relationships or patterns, a large amount of data must be collected and extracted to generate results.
- Higher costs Without a doubt, the longitudinal survey is more complex and expensive. Being a long-term form of research, the costs of the study will span years or decades, compared to other forms of research that can be completed in a smaller fraction of the time.
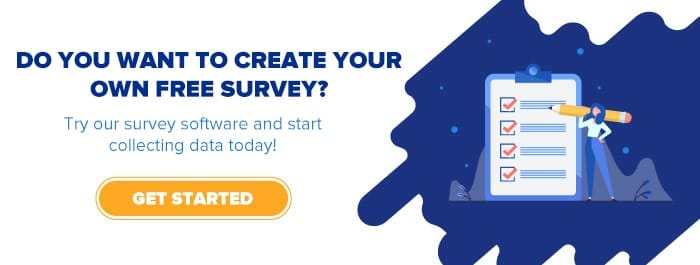
Longitudinal studies vs. Cross-sectional studies
Longitudinal studies are often confused with cross-sectional studies. Unlike longitudinal studies, where the research variables can change during a study, a cross-sectional study observes a single instance with all variables remaining the same throughout the study. A longitudinal study may follow up on a cross-sectional study to investigate the relationship between the variables more thoroughly.
The design of the study is highly dependent on the nature of the research questions . Whenever a researcher decides to collect data by surveying their participants, what matters most are the questions that are asked in the survey.
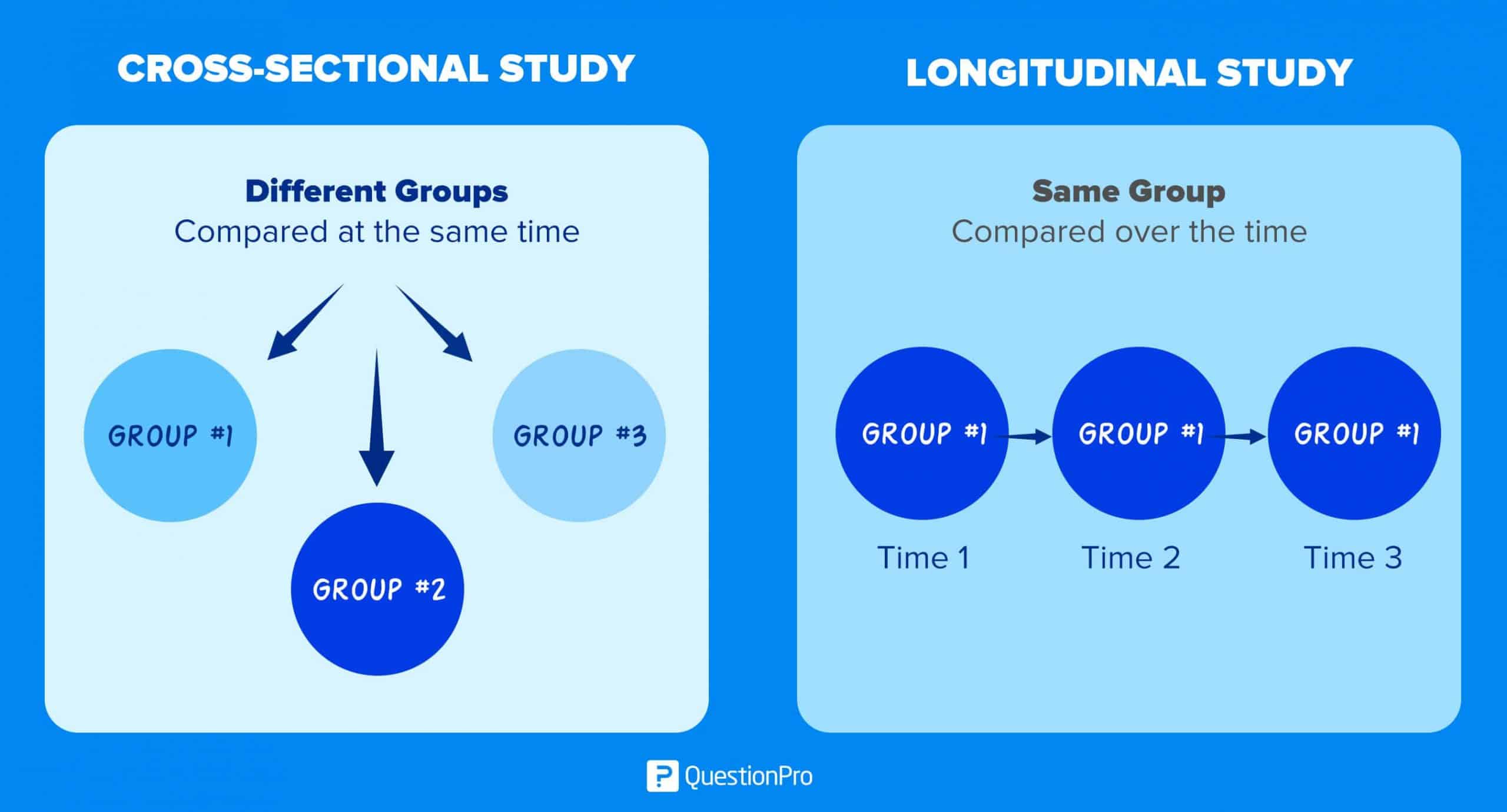
Knowing what information a study should gather is the first step in determining how to conduct the rest of the study.
With a longitudinal study, you can measure and compare various business and branding aspects by deploying surveys. Some of the classic examples of surveys that researchers can use for longitudinal studies are:
Market trends and brand awareness: Use a market research survey and marketing survey to identify market trends and develop brand awareness. Through these surveys, businesses or organizations can learn what customers want and what they will discard. This study can be carried over time to assess market trends repeatedly, as they are volatile and tend to change constantly.
Product feedback: If a business or brand launches a new product and wants to know how it is faring with consumers, product feedback surveys are a great option. Collect feedback from customers about the product over an extended time. Once you’ve collected the data, it’s time to put that feedback into practice and improve your offerings.
Customer satisfaction: Customer satisfaction surveys help an organization get to know the level of satisfaction or dissatisfaction among its customers. A longitudinal survey can gain feedback from new and regular customers for as long as you’d like to collect it, so it’s useful whether you’re starting a business or hoping to make some improvements to an established brand.
Employee engagement: When you check in regularly over time with a longitudinal survey, you’ll get a big-picture perspective of your company culture. Find out whether employees feel comfortable collaborating with colleagues and gauge their level of motivation at work.
Now that you know the basics of how researchers use longitudinal studies across several disciplines let’s review the following examples:
Example 1: Identical twins
Consider a study conducted to understand the similarities or differences between identical twins who are brought up together versus identical twins who were not. The study observes several variables, but the constant is that all the participants have identical twins.
In this case, researchers would want to observe these participants from childhood to adulthood, to understand how growing up in different environments influences traits, habits, and personality.
LEARN MORE ABOUT: Personality Survey
Over many years, researchers can see both sets of twins as they experience life without intervention. Because the participants share the same genes, it is assumed that any differences are due to environmental analysis , but only an attentive study can conclude those assumptions.
Example 2: Violence and video games
A group of researchers is studying whether there is a link between violence and video game usage. They collect a large sample of participants for the study. To reduce the amount of interference with their natural habits, these individuals come from a population that already plays video games. The age group is focused on teenagers (13-19 years old).
The researchers record how prone to violence participants in the sample are at the onset. It creates a baseline for later comparisons. Now the researchers will give a log to each participant to keep track of how much and how frequently they play and how much time they spend playing video games. This study can go on for months or years. During this time, the researcher can compare video game-playing behaviors with violent tendencies. Thus, investigating whether there is a link between violence and video games.
Conducting a longitudinal study with surveys is straightforward and applicable to almost any discipline. With our survey software you can easily start your own survey today.
GET STARTED
MORE LIKE THIS
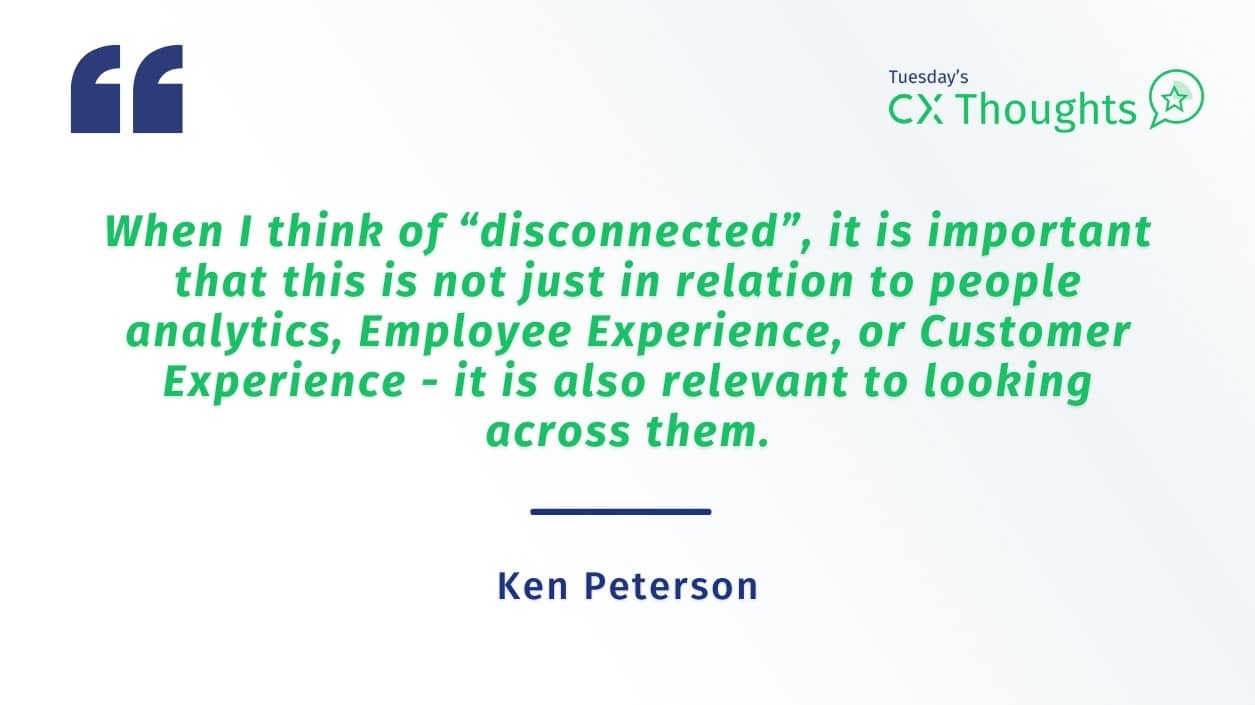
I Am Disconnected – Tuesday CX Thoughts
May 21, 2024
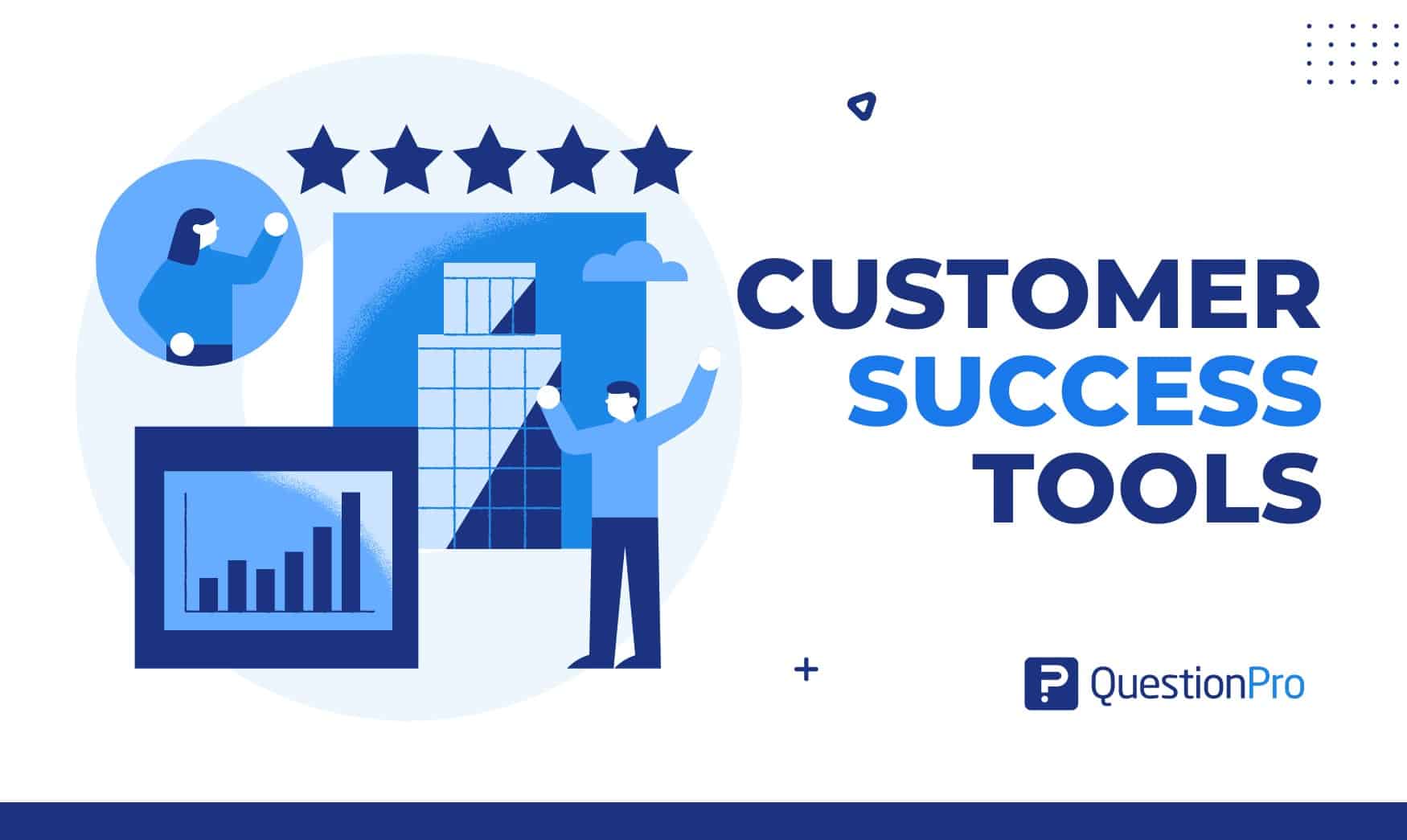
20 Best Customer Success Tools of 2024
May 20, 2024
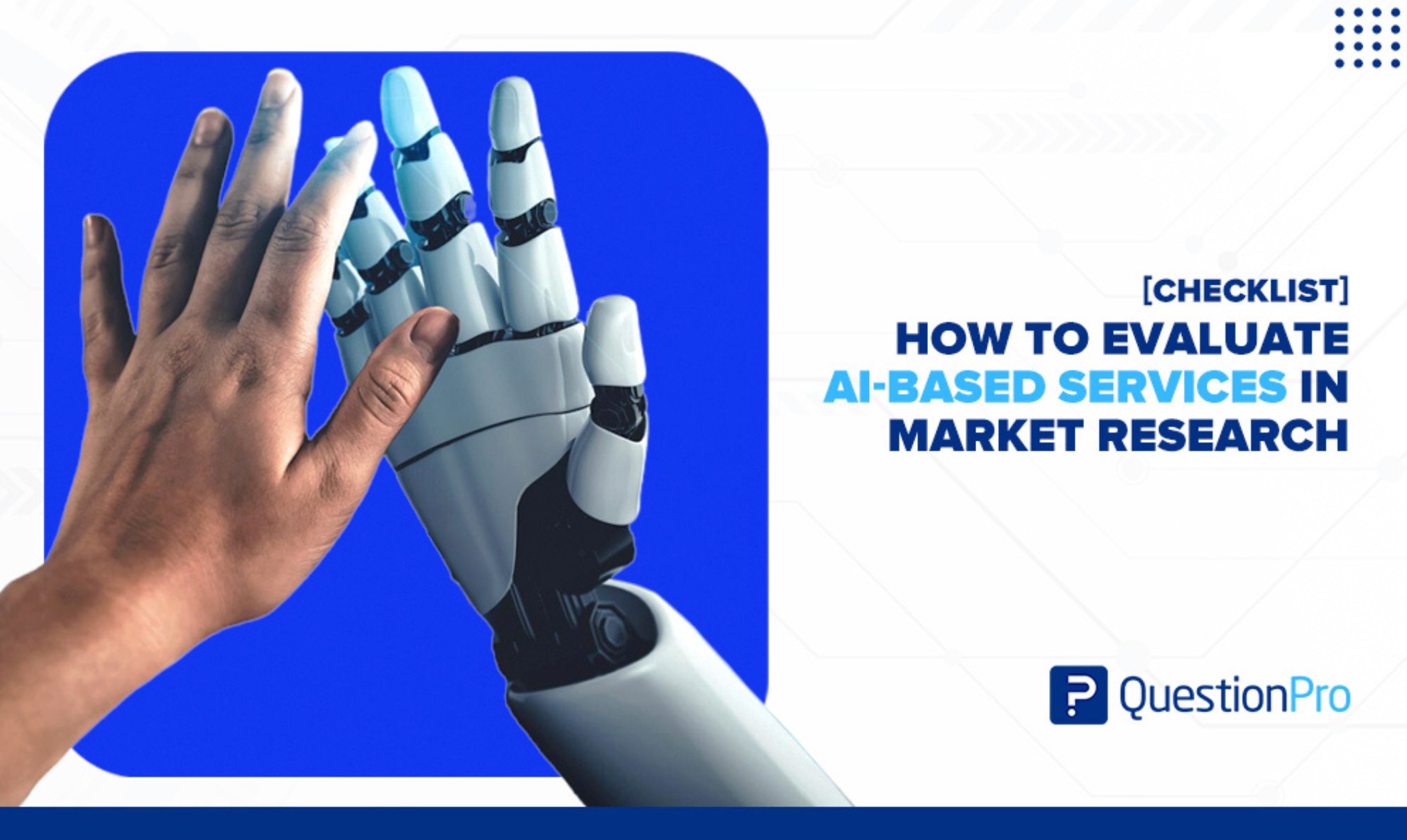
AI-Based Services Buying Guide for Market Research (based on ESOMAR’s 20 Questions)
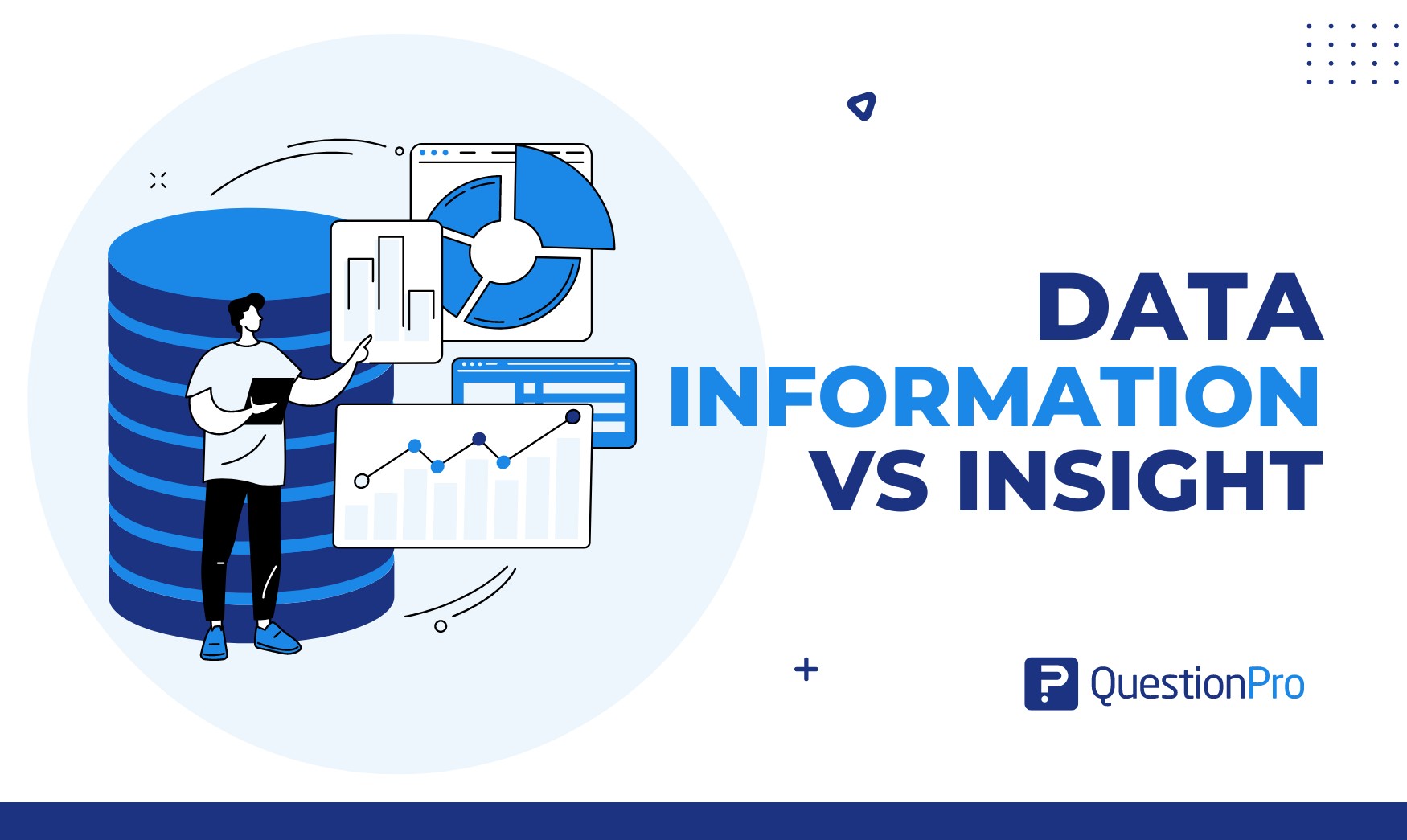
Data Information vs Insight: Essential differences
May 14, 2024
Other categories
- Academic Research
- Artificial Intelligence
- Assessments
- Brand Awareness
- Case Studies
- Communities
- Consumer Insights
- Customer effort score
- Customer Engagement
- Customer Experience
- Customer Loyalty
- Customer Research
- Customer Satisfaction
- Employee Benefits
- Employee Engagement
- Employee Retention
- Friday Five
- General Data Protection Regulation
- Insights Hub
- Life@QuestionPro
- Market Research
- Mobile diaries
- Mobile Surveys
- New Features
- Online Communities
- Question Types
- Questionnaire
- QuestionPro Products
- Release Notes
- Research Tools and Apps
- Revenue at Risk
- Survey Templates
- Training Tips
- Uncategorized
- Video Learning Series
- What’s Coming Up
- Workforce Intelligence

11 Advantages and Disadvantages of Longitudinal Studies
Longitudinal studies are a type of research or survey that primarily uses the method of observation, which entails that they do not involve interfering with the subjects in any means. These studies are also unique in a way that they follow a certain timeline that is entirely dependent on the respondents, which means that data collection could take years depending on the exact timetable put in place. Most of the time, they are used by psychologists who are looking to measure or identify the impact therapy can have over time, involving long time frames and vast amounts of data.
Now, like any other type of method in conducting research, longitudinal studies also come with certain disadvantages, while they offer obvious advantages. Here are important things to take note when planning to use this methodology:
List of Advantages of Longitudinal Studies
1. They are effective in determining variable patterns over time. Because these studies involve the use and collection of data in long periods of time, they can determine patterns efficiently. By using them, it would be possible for researchers to learn more about cause and effect relationships and make connections in a clearer manner. Aside from this, remember that more data over longer periods of time will allow for more concise and better results. These studies are considered highly valid for determining long-term changes and are unique in themselves when it comes to being able to provide useful data about these individual changes.
2. They can ensure clear focus and validity. With a clear focus, longitudinal studies would let us observe how a certain set of circumstances or an end state would come to be. And while it is natural for people not to remember past events, this problem can be addressed by means of actual recording that ensures a high level of validity.
3. They are very effective in doing research on developmental trends. As mentioned above, these studies are often used in psychology to conduct research on developmental trends across life spans. They are used in sociology to study life events throughout lifetimes or generations. This is so because, unlike cross-sectional studies where different individuals with similar characteristics are being compared, longitudinal studies would track the same people, which means that the differences observed in a group will be less likely to be the result of a change or difference in culture across generations.
4. They are more powerful than cross-sectional studies. As they utilize the observation method without manipulating the state of the world, longitudinal studies have been argued to having less power in terms of detecting causal relationships compared with experiments. However, they are known to have more power than cross-sectional studies when it comes to excluding time-invariants and unobserved individual differences and when it comes to observing a certain event’s temporal order, as they use repeated observations at individual levels.
5. They are highly flexible. Longitudinal studies are often observed to allow flexibility to occur. This means that the focus they use can be shifted while researchers are collecting data.
6. They can provide high accuracy when observing changes. With their quality of being the perfect method to conduct research on developmental trends, these studies can make observation of changes more accurate, making them as the usual option in various fields. In medicine, for example, longitudinal studies are used to discover predictors or indicators of certain diseases, while in advertising, they are used to determine changes that a campaign has made in the behavior of consumers who belong to its target audience and have seen the advertisement.
List of Disadvantages of Longitudinal Studies
1. They require huge amounts of time. Time is definitely a huge disadvantage to any longitudinal study, as it typically takes a substantial amount of time to collect all the data that is required. Also, it takes equally long periods to gather results before the patterns can even start to be made.
2. They risk gathering data that is not 100% reliable. While data is collected at multiple points in this method of conducting research, you cannot pre-determine and take into account the observation periods regardless of what happens between these points. Aside from this, respondents would unknowingly change their qualitative responses over time to better suit what they see as the objective of the observer. Generally, the process involved in longitudinal studies will change how respondents and subjects the questions that are being used.
3. They would risk experiencing panel attrition. One of the biggest disadvantages of conducting longitudinal studies is panel attrition. This means that, if researchers are only relying upon the same group of subjects for a research that takes place at certain points in time in years, then there is the possibility that some of the subjects would no longer be able to participate because of various reasons, such as changes in contact details, refusal, incapacity and even death, which cuts down the usable data to be drawn to formulate the conclusion.
4. They require a large sample size. Another disadvantage that makes longitudinal studies not the perfect option to conduct research is that they typically require large sample sizes. So, you must have a large number of cooperating subjects for your research or else it will not realize or be valid.
5. They can be more expensive compared with cross-sectional studies. Cross-sectional studies are known to be more affordable compared with longitudinal studies and are much quicker in reaching an observational conclusion as they use fewer touch points. Considering that they utilize a sample size that is carefully chosen, rather than subsets, the former can also be more of a help in representing entire populations. The former is observed to be very beneficial when it comes to considering a change in policy, unlike the latter.
A lot of researchers encourage and welcome the use of longitudinal data sets, where they can apply and access data via relevant pathways that are set out by the groups that hold such information. However, longitudinal studies also have some limitations. Based on the advantages and disadvantages listed on this article, do you think these method are more helpful to society than not?

An official website of the United States government
The .gov means it’s official. Federal government websites often end in .gov or .mil. Before sharing sensitive information, make sure you’re on a federal government site.
The site is secure. The https:// ensures that you are connecting to the official website and that any information you provide is encrypted and transmitted securely.
- Publications
- Account settings
Preview improvements coming to the PMC website in October 2024. Learn More or Try it out now .
- Advanced Search
- Journal List
- HHS Author Manuscripts

Longitudinal Qualitative Methods in Health Behavior and Nursing Research: Assumptions, Design, Analysis and Lessons Learned
Emily l. tuthill.
1 Department of Community Health Systems, School of Nursing, University of California San Francisco, CA, USA
Ann E. Maltby
Kira diclemente.
2 Department of Behavioral and Social Sciences, Brown University School of Public Health, Providence, RI, USA
Jennifer A. Pellowski
3 International Health Institute, Brown University School of Public Health, Providence, RI, USA
4 Department of Epidemiology and Biostatistics, University of Cape Town, South Africa
Longitudinal qualitative research (LQR) is an emerging methodology in health behavior and nursing research. Researchers are turning to LQR to understand experiences across time as well as identify facilitators and inhibitors of health/illness behaviors and transitions. Currently, a lack of information exists to guide researchers on LQR techniques and considerations. Our objective was to provide a methodological resource for health behavior and nursing researchers conducting LQR. LQR may be applied to understand any human experience, as well as the sequalae of the experience and is well suited for studying transitions and developmental or behavioral changes. Conducting LQR is resource intensive and requires flexibility and complex analyses. We discuss multiple components of LQR such as design considerations, analysis options, and our lessons learned. Despite complexities, LQR provides the opportunity to understand experiences across time within an individual and among a group resulting in holistic, in-depth understandings beyond a cross-sectional time point.
Introduction
Longitudinal qualitative research (LQR) is an emerging methodology in health behavior and nursing research—fields focused on generating evidence to support nursing practices as well as programs, and policies promoting healthy behaviors ( Glanz et al., 2008 ; Polit & Beck, 2017 ). Because human experiences are rarely comprised of concrete, time-limited events, but evolve and change across time, the use of LQR offers an innovative option to capture this natural history. The advantage of LQR over cross-sectional designs in health behavior and nursing research is that LQR provides a unique understanding of experiences across time, turning points, critical time points in transitions as well as the facilitators or challenges that support or undermine behaviors aligned with health/illness and life course transitions ( SmithBattle et al., 2018 ). In pursuit of understanding the natural history or trajectories of human experiences, LQR generates in-depth data on the social and behavioral aspects of transitions that are less evident through cross-sectional or quantitative data alone. However, the broader nursing and health behavior research literature is deficient in resources offering theoretical, methodological, and analytical guidance on conducting LQR. To fill this gap, we developed a methodological resource to guide planning and decision making in LQR for health behavior and nursing researchers by pulling from our experiences and other disciplines such as education where more literature exists on conducting LQR. Many of the examples presented here are based on our research team’s LQR applied to better understanding the transition from pregnancy to postpartum among women living with HIV in South Africa and Kenya (i.e., K23MH116807 ELT; K01MH112443 JAP). Depending on the goals of the research team, this resource may be used in its entirety or by section.
This resource includes the following sections relevant to conducting LQR:
- Philosophical assumptions of LQR
- Methods of LQR, including design strategies and data collection.
- Analysis of LQR data, including an overview of several analysis options.
- Results of LQR, including how to ensure trustworthiness of findings.
- Discussion of challenges in LQR.
Examples of Longitudinal Qualitative Research from Health Sciences Disciplines.
Study Considerations for LQR and Lessons Learned From Conducting Our Own LQR.
Potential Theoretical Framework for LQR and its Application
Transition theory (tt).
( Chick & Meleis, 1986 ; Meleis et al., 2000 ) is a well-suited model to describe health behaviors that occur across time and across a transition, such as diet and physical activity across the transition from pregnancy to postpartum or drinking behaviors across the transition from adolescence to early adulthood. TT allows researchers to characterize and describe transitions, such as those described above, and define the relevant personal, community, and societal characteristics that may facilitate and inhibit health behaviors necessary for a successful transition.
Application:
In our recent study of HIV treatment adherence among pregnant and postpartum women we utilized Transitions Theory (TT) to examine maternal motivations, behaviors, and social contexts from the 8th month of pregnancy up to a year postpartum in Cape Town, South Africa ( Pellowski et al., 2019 ). During the design process, TT was chosen as an overarching theoretical framework for the study and influenced the sample of interest (recruiting women during pregnancy as opposed to only focusing on the postpartum period). TT also guided the main sections of each interview agenda with a focus on the concepts that the theory posits to be the most influential on health behaviors across this transition (e.g. personal meanings, cultural beliefs and attitudes, socioeconomic status, preparation and knowledge, partners, families, and community stigma). Additionally, the four interview time points were carefully selected to align with what TT describes as critical points and events: 1) late pregnancy was chosen to capture preparations for the transition, 2) 6–8 weeks postpartum was chosen to capture reflections on birth and initial impacts of the newborn on daily life, 3) 4–6 months postpartum was chosen to capture early infant HIV testing, the end of exclusive breastfeeding/introduction of other foods, and maternal transfer of HIV care services, and 4) 9–12 months postpartum was chosen to capture possible disengagement from HIV care and the end of the breastfeeding period for many women in this context. Finally, TT was used to guide the analysis, which utilized an inductive approach. It is of note that the application of theory to an LQR project does not restrict the types of analyses that can be utilized (e.g. inductive vs deductive approaches). In this example, TT was used as a guiding framework to ensure that all phenomena relevant to the transition from pregnancy to postpartum for women living with HIV were captured in data collection. During data analysis, the main objective was not to support or refine the theory (deductive approach), but rather to understand and make generalizations about the unique experiences of women with the constructs defined by TT serving as general reference points ( Pellowski et al., 2019 ).
An Application of the Serial Interview Approach from NIH K23MH116807
A sample of 30 perinatal women were interviewed during their 3rd trimester of pregnancy. They were asked about their breastfeeding intentions, expectations and past experiences with breastfeeding. Based on the findings from the initial interview the women were interviewed again at 6 weeks postpartum. The 6-week interview focused on how the reality of breastfeeding compared to the intentions and expectations the women had prior to giving birth, while looking for newly identified inhibitors and facilitators of breastfeeding behavior. Based on these findings, the researchers tailored a third interview guide to be used when the women reached 5–6 months postpartum to inquire how breastfeeding behaviors evolved as infants grew while exploring new contexts, such as mothers returning to the workplace.
What Makes Longitudinal Qualitative Research a Distinct Methodology?
We have already introduced LQR as an emerging methodology. However, depending on one’s understanding of what a research methodology entails, LQR may appear to be something too broad or flexible to be considered a distinct methodology in and of itself ( McCoy, 2017 ). This seems especially true when LQR is held up against long established qualitative methodologies (with more prescriptive methods) such as Grounded Theory ( Glaser & Strauss, 1967 ; Glaser, 1978 ), Ethnography ( Pelto, 2013 ) or Phenomenology ( Colaizzi, 1978 ). Further confusion surrounding LQR’s classification as a methodology may stem from the substantial overlap of qualitative techniques and procedures between methodologies ( Hermanowicz, 2013 ). Indeed, many LQR studies include the use of data collection techniques or analysis procedures commonly used in other qualitative methodologies.
We propose, however, that LQR exhibits all of the defining characteristics of a unique qualitative methodology ( Carter & Little, 2007 ), including distinct research objectives, foundational assumptions, and well-developed explanations of the methodological and analytic principles as outlined in the following sections. Central to qualitative research, while some procedures or techniques may overlap between methodologies, the research objectives, assumptions, and principles of the chosen methodology should justify the procedures/techniques used ( Carter & Little, 2007 ). For example, LQR may not simply apply a Grounded Theory analysis plan because Grounded Theory analysis procedures do not account for change across time (a primary objective of LQR). However, an inductive thematic analysis (as is applied in Grounded Theory) might be used in LQR to cross-sectionally analyze baseline data in order to identify emergent themes from the initial research encounter. Similarly, LQR studies may employ ethnographic data collection techniques such as observing behaviors across time. However, while ethnographic studies aim to understand a cultural phenomenon or behavior from the viewpoint of participants ( De Chesnay & Abrums, 2015 ) LQR aims to establish a shared understanding of how and why the phenomenon or behavior changes across time. Thus, the management and analysis of data in LQR is inherently different from other methodologies.
What are the Unique Objectives of Longitudinal Qualitative Research?
LQR’s distinction is in its aim to understand an experience or behavior(s) across time; explicitly seeking to answer, “how did this change?” “how is this different?” “why did this change?” and/or “what remains the same?” ( Saldaña, 2003 ). LQR designs have been applied in a variety of research areas including, transitions in human development ( Schmidt et al., 2019 ), the experiences of incarceration ( Cooper et al., 2015 ), aging ( Oosterveld-Vlug et al., 2013 ) and the progression of chronic illness ( Namukwaya et al., 2017 ), as well as behavioral research investigating medication adherence ( Salter et al., 2014 ; Weiser et al., 2017 ) and breastfeeding ( Doherty et al., 2006 ; Jardine et al., 2017 ). LQR may be applied to understand any human experience, as well as its sequalae and is particularly well suited for studying transition periods and developmental or behavioral changes across time. LQR may also be applied to inform the development of health behavior theories or interventions and may be used to understand if a policy or program was effective, why or why not and in what contexts might similar results be expected ( Lewis, 2007 ; see Table 1 for selected examples of study objectives).
Philosophical Assumptions of Longitudinal Qualitative Research
Although the origins of LQR are not strictly defined, there are several assumptions that comprise the philosophical underpinnings of the methodology. First, LQR is based on the assumption that two key concepts— time and change are contextual ( Saldaña, 2003 ). While LQR often occurs over months to years, it is not only the chronological passing of time that creates meaning, but rather how the individual experiences that passage of time ( Saldaña, 2003 ). Different points in a person’s life may make the experience of time qualitatively different from another—passing quickly when one is busy or having fun and slowly when one sits quietly. In addition to personal level variation in the experience of time, the cultural context may also influence the interpretation of time. For example, the acceptable age for marriage or first-time parenthood can vary greatly across cultures, which may impose different time-based milestones or experiences in one’s life.
Since time and our human experiences within it are both contextual, the change we experience across time is also contextual ( Saldaña, 2003 ). Change may not be a linear or ordered journey from one state to another with a definitive end point. Thus, the depth of transitions may not be captured when change is viewed in isolation either as a single unit of analysis or as a solitary episode. LQR assumes the need to explore the complex, haphazard and potentially contradictory ways change emerges and to conceptualize the pathways in which these complexities in experiences and behaviors exist across time ( Pettigrew, 1990 ). Overall, LQR assumes change is multi-faceted and holistic where continuity, patterns, idiosyncrasies, and contexts are key components ( Pettigrew, 1990 ).
The second assumption in LQR centers on the human experience being a construct of the participants’ personal reflections and the researchers understanding of them, allowing multiple realities to exist simultaneously ( Balmer & Richards, 2017 ; McCoy, 2017 ). Furthermore, the construction of these experiences relies on the notion that participants are willing and able to articulate their experiences in a way that can be understood by the researcher ( Baillie et al., 2000 ). In qualitative research, and LQR in particular, participants share their experiences and researchers listen, analyze, and interpret these experiences. Researchers may present their findings back to the participant for their evaluation or ask the participants about the same experience again at a later timepoint to evaluate how their experience or their feelings about it may have changed. Through this process, the essence of the experience across time is established for each participant ( Balmer & Richards, 2017 ; McCoy, 2017 ).
Methods of Longitudinal Qualitative Research
There are no gold standards or fixed rules for data collection in LQR. In general, LQR applies either prospective or retrospective designs that include two or more data collection sessions using qualitative techniques (e.g., interviews, observations, multi-qualitative methods) over a specified time frame ( Saldaña, 2003 ). Yet, the defining principles of data collection in LQR go beyond having data collected at multiple time points. The chosen data collection techniques in LQR must also ensure the quality of data collected as well as cater to the researchers’ abilities to systematically manage and thoughtfully analyze these data across time ( Smith, 2003 ). The researcher’s ongoing assessment of data coupled with the flexibility to make adjustments are hallmarks of LQR methods.
General Design Strategies
Designing LQR studies that effectively capture change is not straightforward. Two overarching complexities are, 1) the length of time needed to be considered longitudinal is not definitive and 2) a universally accepted definition of change does not exist, making it challenging to identify change processes or outcomes across time a priori ( Pettigrew, 1990 ). These complexities are key, however, for researchers to work through as they consider the change they are seeking to understand and the corresponding outcomes. Some design strategies to consider include theoretical frameworks, target population and size, setting and personnel (see Table 1 for selected examples of LQR designs, see Table 2 for additional design considerations and personal lessons learned).
Theoretical framework.
A theoretical framework is chosen based on the research objectives (See Box 1 for an example of a theoretical framework and its application in LQR). Theoretical frameworks are particularly helpful in identifying concepts relevant to the phenomenon of interest and how these concepts may change across time to influence behavior ( Chinn & Kramer, 2011 ). A theoretical framework should be chosen at the outset of project planning and inform 1) sample(s) of interest, 2) content of data collection (e.g. questions/probes developed for in-depth interviews), 3) timing of data collection and, 4) plans for data analysis. Researchers can then operationalize and explore concepts from the framework by asking: How could we define and measure these concepts in the context we are interested in? What information would help us describe and understand these concepts across time? In addition, researchers must remain open to new concepts and pathways that emerge from their data.
Identifying the target population and sample size.
Participants in longitudinal studies are selected based on their shared experience of the phenomenon of interest ( Saldaña, 2003 ). Yet, an individual’s experience is distinct and close observers (friends, family, or caregivers of the individual) can also lend valuable insight ( Johansen et al., 2013 ). Moreover, LQR does not limit the unit of analysis to individual participants. Data might also be collected from focus groups, families, or groups of co-workers ( Johansen et al., 2013 ; see Table 1 for other examples). Thus, researchers must carefully consider who to collect their data from and how many units of analysis (individuals, focus groups, families, etc.) are needed to adequately address the research aims ( Kneck & Audulv, 2019 ). In LQR, in particular, researchers must also anticipate a certain level of attrition because over time participants may migrate, die, or simply lose interest in participating in the study ( Calman et al., 2013 ; Kneck & Audulv, 2019 ). One approach researchers may use to determine sample size is estimating the number of cases needed to reach saturation ( Hennink et al., 2017 ), which for a phenomenology design is typically 10–12 participants ( Polit & Beck, 2017 ). Saldaña (2003) recommends LQR studies start with more participants than you anticipate needing to ensure data saturation is reached, especially if a study takes place over two or more years. Because the context, study design, population, and setting are study specific, determining a certain number or percent to overestimate on sample size is best left to the research team’s judgment, which is based on the stability of their target population. In a systematic review of LQR in nursing, attrition was either a major limitation (20% of studies estimated 50% attrition) or a major strength (30% of studies had 0% attrition; SmithBattle et al., 2018 ). Given these extremes, during the planning phases of the research, attention should be given to understanding sample characteristics including potential barriers to long term participation.
A number of considerations are helpful when determining study setting in LQR. First, the venue must be convenient for the participants over the study period such as one close to the participants’ home or a venue the participants frequent such as their health clinic. Second, if the research team is conducting their study within a clinic or hospital where participants are patients, gaining the support of the clinicians and administrators prior to the start of the study and maintaining strong relationships throughout the study period is key to a collaborative, lasting partnership. Support from stakeholders ensures the desired space is reserved, the study does not disrupt the patient flow, and that the research encounters can be coordinated with participants’ regularly scheduled appointments. Third, the study team needs a private, quiet and secure location where participants will be able to focus on the interview questions while feeling relaxed and comfortable enough to fully express thoughts and experiences. This will also mitigate interruptions and background noise which may distract the participant and detract from capturing clear audio recordings. Fourth, supplying refreshments, child care, and easy access to restrooms may lead to a better experience for participants. Finally, if the researcher chooses to collect data in the homes of participants, the added value of observing participants in their own environment must be weighed against the challenges of working in a less controlled setting (more distractions, interference from other people in the home, potential safety concerns for the researcher, etc.) as well as privacy concerns (particularly when discussing stigmatized diseases or behaviors). Whatever venue is chosen, to the extent that it is feasible, maintaining the same venue throughout the duration of the LQR provides important design consistency and familiarity for participants, which may help retention. Some of these items may be relevant for cross-sectional studies as well, however, we have found that accounting for the aforementioned considerations are of paramount importance in LQR as they nurture long-term participation.
LQR is labor intensive as collecting, organizing and analyzing data is time consuming. Researchers should plan ahead, mapping out the time required for each phase, strategically selecting who will carry out each task and which tasks are best executed collaboratively. Many different skills may be required including, interviewing, conducting focus groups, videography, transcribing audio, translating transcribed text, organizing and managing data and finally conducting the analysis. In addition, there are other demands on staff time including, 1) reviewing and quality checking initial data, 2) revising subsequent interview protocols and guides and 3) maintaining contact with participants between study sessions. The research team should consider the different skills each staff member brings to optimize effectiveness of the study procedures. For example, team members with knowledge of the local language and culture who conduct interviews may also provide invaluable insight into the interpretation of data and its meaning beyond the strictly literal translations of the interviews. Additionally, planning for the same study team member(s) to interact with participants at each data collection point optimizes rapport and trust and aids in retention efforts—particularly when the LQR is occurring over long periods of time (months and years; Nevedal et al., 2018 ). Managing the ebb and flow of workloads across data collection time points requires the thoughtful organization and adaptability of project coordinators in collaboration with principal investigators.
Steps for Data Collection
Step one: operationalize concepts, including time and change..
Conceptually, the notion of time may be different between participants or from the research team’s design expectations. To alleviate this potential disharmony, Pettigrew (1990) suggests that the research team clearly operationalize the concepts of time and change at the outset of the study (as discussed in the “ Philosophical Assumptions of Longitudinal Qualitative Research ” section above). In some cases, the “baseline” (starting point) from which the change/transition of interest begins may not fall within the first interview. For example, when looking at the experience of living with HIV, the baseline might be when the person was first diagnosed with HIV (i.e., years prior) or rather the first time they engaged in treatment sometime after their diagnosis. Change may also be absent across time, which may reflect positive or negative behaviors (maintaining medication compliance vs. maintaining unhealthy habits; Lewis, 2007 ; Saldaña, 2003 ).
Step two: Type of data to be collected.
LQR data may originate from interviews with members of the target population, or with key informants such as family, friends, clinicians or other stake-holders. Data may also come from short answer surveys, focus group discussions or direct observations ( Johansen et al., 2013 ). Initially, data may be in the form of audio recordings, videos, pictures, drawings or field notes. In some cases, LQR studies are embedded in randomized control trials or mixed-methods studies where various types of data were collected. For example, a study on depression might use an established screening tool to assess depression scores at each encounter prior to conducting in-depth interviews with participants. There are no restrictions or limitations to type or quantity of data collected, only the a priori considerations of the desired contribution from each data source, data management and data analysis plan.
Step three: Study approach.
There are several approaches to consider in longitudinal qualitative inquiry. The primary approach used in LQR is serial interviews ( Calman et al., 2013 ; Murray et al., 2009 ). This approach utilizes emergent issues or themes from one interview to inform the line of inquiry used in subsequent interviews. The time between data collection points allows the research team an opportunity to review the data and modify interview guides ( Smith, 2003 ). Subsequent interviews can then be designed to build on rather than duplicate the previously collected data. Importantly, process notes/interview summaries and frequent debriefing of interviews is key to ensuring subsequent interviews are on target (See Box 2 for an example of a study on breastfeeding behaviors using the serial interviews approach).
Step four: Triangulation of data.
This step is meant to validate preliminary findings and ensure data completeness and trustworthiness. There are several ways to triangulate data. For example, findings from interviews with key informants, or focus group discussions can be compared to findings from in-depth interviews with individuals to compare completeness and consistency in findings. Another option is to conduct a final exit interview with each participant ( Saldaña, 2003 ). The purpose of a final exit interview is to present the researcher’s findings to the participant for feedback. Questions may be asked to confirm or disconfirm preliminary assertions, themes or trends. Participants may also be asked to reconstruct their experience within the study timeframe. Similarly, questions could be focused on areas of uncertainty or missing details revealed by the analysis and interpretation. These final insights can assist the research team in confirming their description of change across time using a collaborative, reflective and flexible approach ( Pettigrew, 1990 ).
Analysis of Longitudinal Qualitative Research Data
Longitudinal qualitative data analyses attempt to transform data into explanations and insights which address the original research objective—understanding an experience or behavior across time. Analysis in LQR is challenging on many levels given the large amounts of data to analyze ( Lewis, 2007 ; Pope et al., 2000 ; Smith, 2003 ), the multiple types of data such as field notes, interview summaries, surveys, transcripts or even videos ( Miles et al., 2014 ) as well as the challenge of describing how the experience may change across time within participant and among a group.
The research team is tasked with managing data collection, revision/development of subsequent interview guides and possibly even initiating data analysis while data collection is still ongoing ( McLeod & Thoon, 2009 ; Pope et al., 2000 ). This is especially challenging because carefully transcribing (and when necessary translating) data is time consuming and it is not always feasible to allow ample time in between data collection time points for analysis to be completed ( McLeod & Thoon, 2009 ). Some studies are chronologically time sensitive such as those seeking to understand distinct developmental time periods that would not be captured if data collection were postponed to a later date—early parenthood for example. In these cases, detailed process notes or summaries of individual interviews and frequent debriefings with study staff may be crucial for informing subsequent rounds of data collection. Bearing in mind the aforementioned challenges, what follows are the central analytic principles and procedures for LQR analyses (see Table 1 for selected examples of LQR analyses).
Step 1: Consider the Analysis Approach
The analysis of LQR data can be carried out using a variety of different approaches with the precise methods used often evolving alongside the data collection ( Saldaña, 2003 ). Applying a deductive and/or inductive lens is often a good starting place. Using a deductive approach, researchers begin with a theory or framework in mind and analyze their data to identify specific findings that lend support to, clarify, or refine the theory/framework ( Burnard et al., 2008 ). If applying an inductive approach, researchers start from their original observations and seek to find patterns or make generalizations about their data eventually using their findings to create a theory or framework, establish pathways, or to develop themes or categories related to the phenomenon of interest ( Burnard et al., 2008 ). Researchers can also fall somewhere in between relying on predetermined codes or a framework to organize their data while still trying to identify new patterns or generalizations emerging from the data (see Box 1 for an example of this).
Researchers should also consider if their research objectives are best suited to a diachronic or synchronic analysis approach. Synchronic analysis implies analysis is simultaneous (synchronized) with data collection or occurring as a cross-sectional analysis after each wave of data collection ( Nevedal et al., 2018 ). Synchronic analyses are common in LQR because data collection and analysis are often a fluid process where initial and ongoing analyses are imperative to inform subsequent data collection encounters ( Balmer & Richards, 2017 ; Calman et al., 2013 ; Pope et al., 2000 ). Researchers must stop and ask, “what do we know so far?” “what have we missed?” and “what do we need to know more about to fully understand this experience?” The next round of inquiry is then directed accordingly ( Pope et al., 2000 ). As mentioned, in some instances, synchronic analysis may be less feasible due to time constraints or less important for achieving the study objectives. In these cases, researchers may opt for a diachronic approach, meaning they wait to conduct their analysis until after all data has been collected. Of note, researchers may also choose to conduct both synchronic analysis (cross sectional, after each research encounter) and diachronic analysis (longitudinal, using all data once data collection is complete).
Step 2: Setting Up an Analytic Roadmap
Regardless of the chosen approach, an analytic roadmap outlining the specific steps of analysis is critical to providing direction given the complexity of LQR data. As the study progresses, the initial roadmap may change, and when this happens documenting how the path taken differs from the original plan is needed. A clear and auditable “trail of decisions” ( Guba & Lincoln, 1981 , as cited in Sandelowski, 1986 , p. 33) can establish the dependability of results in qualitative research. Thus, recording when and how decisions about conducting the analysis were made is important for the research team’s reference as well as future reporting of results (see Trustworthiness of Longitudinal Qualitative Research below). The roadmap documentation should include: detailed explanations of what was done, when, and why as well as what did and did not lead to meaningful findings.
Step 3: Familiarization and Coding
After converting raw data (audio recordings, field notes, etc.) into coherent text, the next step of most analytic roadmaps is to read and reread transcripts to become familiar with the content, start identifying potential themes, and assess data quality and effectiveness of the interview guide. For some researchers, highlighting excerpts and adding comments or descriptive memos is also useful during this time, whether by hand or with qualitative data analysis software. Discussing initial data and data quality within the research team is also a part of this process. This is especially important in research teams where different members conducted the interviews and others are leading the analysis. Constructive feedback from the team can provide direction and suggestions for the interviewer in the next round of data collection while the interviewer can offer insight about the interactions with the participants (such as their tone or body language) that may not be fully evident to team members reading the transcripts.
After team discussions on data quality, the next step is often applying codes to the text. This could be a predetermined list of codes or one that emerges from the text. There are many different types of coding schemas such as descriptive coding, versus coding, or in vivo codes that one can apply to suit their analysis (for a comprehensive review on types and procedures for coding see Saldaña, 2009 ). In addition, one can apply the long table or manual approach to code data or use a qualitative data analysis software ( Polit & Beck, 2017 ). Regardless of the type(s) of codes or method by which the coding is done, the objective is to inductively and/or deductively apply codes (labels) to segments of data for the purpose of grouping and organizing thematic segments as well as highlighting exemplar excerpts.
In LQR, there may be one or more members of the research team coding data. Having multiple members of the team coding has several advantages. First, this allows for the inter-rater reliability or the degree of agreement between coders to be assessed. Higher inter-rater reliability shows that codes were applied consistently and supports the rigor and trustworthiness of the study (see trustworthiness of LQR below; Tracy, 2010 ). Moreover, when more than one team member is coding there is opportunity to discuss discrepancies in the application of codes. This guides the team in developing codes with more complete and articulate definitions as well as develops a deeper common understanding of the meaning of each code ( Miles et al., 2014 ). In addition, when various team members code transcripts inductively (without a predetermined code list) multiple perspectives may emerge and be useful, both in terms of capturing all of the possible emerging codes and also in terms of distinguishing between an individual coder’s interpretation of the text and the participants intended meaning ( Pope et al., 2000 ). Conversely, some researchers prefer to have one member do all the coding. An advantage of this approach is that one person can be fully immersed in all the data which may optimize consistency in the analysis. It may also be a pragmatic decision; for example, when an ethnographer embedded in their field site conducts all the data collection and proceeds to do the analysis, this may result in a consistent, comprehensive and thoughtful telling of an experience ( Saldaña, 2003 ).
Step 4: Describing Cross-sectional Data
Analysis of coded data in LQR frequently begins as a cross sectional analysis of the first round of data collected and can include repeated cross-sectional analyses as the researchers work to understand the experience at each timepoint of data collection ( Nevedal et al., 2018 ). Cross-sectional analyses are often conducted using techniques borrowed from other methodologies such as thematic analyses, where coded data are grouped into common sub-themes, sub-themes are grouped into themes and themes into broad categories. Importantly, a meaningful analysis must subsequently attempt to develop a longitudinal (across time) description of the themes or experiences ( Nevedal et al., 2018 ). As the analysis moves from cross sectional to longitudinal it evolves from descriptive (i.e., describing the changes observed) to exploratory (i.e., uncovering the causes and consequences of change or lack of change across time) ( Kneck & Audulv, 2019 ; Lewis, 2007 ).
Step 5: Exploring Longitudinal Data
The final analytical leap from descriptive cross-sectional to exploratory longitudinal is often poorly described in LQR ( Calman et al., 2013 ; Nevedal et al., 2018 ). This is likely because, until recently, neither prescribed nor clearly explained analysis plans for longitudinal data have been documented ( Sheard & Marsh, 2019 ). Within the LQR methodology, researchers are developing variant and sometimes discipline specific analysis techniques consistent with the objectives, assumptions, and principles of LQR ( Carter & Little, 2007 ; Sheard & Marsh, 2019 ). Such analysis plans primarily aim to find patterns of change across time and include: Longitudinal Interpretive Phenomenological Analysis (see McCoy, 2017 ), the Pen and Portrait Technique (see Sheard & Marsh, 2019 ), and the Trajectory Approach (see Grossoehme & Lipstein, 2016 ), which are described in detail elsewhere. In addition, there are the following approaches we describe in detail below:
Longitudinal analysis approaches
Framework analysis ( lewis, 2007 )..
Framework analysis organizes data into one table for each participant (or other unit of analysis) which can then be used to find patterns across participants, across time, and across various identified themes. Patterns might be similar behavioral changes, similar feelings about an experience, or related changes in themes across time. For example, a change in a participant’s understanding of their own health condition may be closely linked to the services they are inclined to access ( Lewis, 2007 ). The rows of the table (sometimes referred to as a framework or matrix) are labeled as the participant encounters (one row for each encounter) while the columns of the tables are topics or themes identified from the theoretical framework, the interview guide, or the initial readings, coding and/or thematic analysis of data (see Table 3 ). Additional columns can be left open for emerging themes ( Lewis, 2007 ). The table is filled in with summaries from each participant in each cell as applicable. Kneck and Audulv (2019) suggest using descriptive summaries during this phase so as not to make any “analytic leaps” too early in the analysis. This process helps remedy the challenge that arises should there be a misinterpretation of data early on in the analysis process upon which future analyses are then based—making it challenging to look back and identify where the misinterpretation occurred. The cells of the table may also include salient words or phrases cut and pasted directly from the transcripts. Reading down the columns the researcher can explore the themes across time, while reading along the rows of the tables the researchers can explore the linkages between themes at a given timepoint. Researchers may also “zig-zag” through the tables to identify other patterns or trends ( Lewis, 2007 ). As these fully populated descriptive tables are explored and analyzed, the researchers can create a second “analysis matrix” where each row represents one unit of analysis and the columns continue to represent the topics/issues/themes of interest. The analysis matrix is then populated with the researcher’s interpretations of how each theme changed (if at all) across time, for each unit of analysis (individual, focus group, family, etc.; Grossoehme & Lipstein, 2016 ).
Example Table for Framework Analysis—Phase 1 and Phase 2.
Cross-sectional Profiling ( Smith, 2003 ).
Cross-sectional profiling develops descriptive summaries of each theme, issue, or topic identified for each participant whereby the participant’s thematic profile is developed further with every encounter ( Smith, 2003 ). The summaries might also be arranged as tables with a separate table for each theme, each row representing a participant with a column for each encounter (see Table 4 ). A profile contains a summary of the researcher’s findings related to a specific theme for each participant for each encounter. Within each table, the individual participants (the rows of the table) may be organized in groups according to demographic characteristics, intervention vs. control, or outcomes. Initially, the first column(s) of the profile table (the participants experience of the theme at the first research encounter) guides further inquiry. For example, Smith (2003) identified ineffective lines of questioning related to one of their interview topics in a first wave of profiling and subsequently adjusted their approach. Once the profile is complete (contains summarized data from each participant at each time point), the researcher establishes the overall narrative of change for each theme for the entire group as well as the sub-groups. Then the individual narratives of change can be viewed relative to the narrative of the entire group or subgroup to which the participant belonged ( Smith, 2003 ). In this way the researcher can understand patterns and facilitating or inhibiting factors for individual change as well as develop individual case studies of change within a particular theme. The case studies can then be explored in terms of theme’s findings for the whole group—is it an exemplar or deviant case, or is the change more or less significant than among other participants ( Smith, 2003 )?
Example Tables for Cross Sectional Profiling.
Case Histories ( Thomson, 2007 ).
This type of analysis uses archives of data to construct accounts of change and continuity across time including the researchers understanding of why things happened the way they did ( Thomson, 2007 ). Researchers use multiple data sources (interview transcripts, field notes, diaries, or notes from focus groups) and synthesize large amounts of information to develop a storyline for each case (individual or group) narrating change or continuity across time (see Table 5 ; Thomson, 2007 ). Case histories go beyond the descriptive level as researchers form a more analytic narrative of the case throughout ( Henderson et al., 2012 ). Sheard and Marsh (2019) describe a similar technique which they refer to as the “pen and portrait analytic technique.” They recommend researchers focus the summaries on the information that is pertinent to the research questions—perhaps centering them around an important theme identified by the researchers. In this way the narratives help to focus the analysis rather than simply serving as an all-encompassing summary. Researchers then use the case histories or narratives to analyze trends. They can group individual case histories by demographics, intervention vs. control or outcomes looking for similarities and differences between the groups as well as exceptional cases within groups. Thomson (2007) describes putting individual case histories “in conversation with each other.” She tried to understand the differences and similarities from different perspectives such as the perspective of the individual versus the perspective of society. Using individual case histories, researchers may also seek to explain why two seemingly different cases have similar outcomes or why two similar cases have different outcomes ( Lewis, 2007 ).
Example Table for Case Histories.
Pattern-Oriented Longitudinal Analysis ( Kneck & Audulv, 2019 ).
The Pattern Oriented Longitudinal Analysis (POLA) approach is meant to be applied in nursing research when there is a single phenomenon in focus for the duration of the study and where questions and interview formats are generally consistent at each data collection point ( Kneck & Audulv, 2019 ). POLA focuses initially on describing each individual participant’s change across time and later looks for patterns of change shared among participants. The shared patterns are developed inductively rather than grouping participants into predetermined categories or outcomes ( Kneck & Audulv, 2019 ). Researchers must think critically to define a shared pattern as well as to assess the sufficiency of data which supports the defining aspects of the pattern and its boundaries (the limits outside which cases no longer fit the pattern). The POLA approach also uses matrices to organize data often with a specific analytic question in mind. For example, “how did the participants thoughts about their disease change across time?” The matrices evolve along with the analysis from organizing individual data to organizing group data. Shared patterns may eventually be categorized into types of patterns such as “a consistent pattern,” “an episodic pattern,” “an on-demand pattern” or “a translation pattern” ( Kneck & Audulv, 2019 ).
Collaboration During Analysis
In some cases, a researcher may carry out their LQR analysis independently. However, it is often necessary, and arguably advisable that researchers work collaboratively within a team to design and execute their LQR data analysis ( Calman et al., 2013 ; Pope et al., 2000 ). Working in teams can be useful for establishing reliability in coding as well as in theme development. Team members of various backgrounds will inevitably have conflicting interpretations of data leading to necessary discussions where multiple perspectives are taken into account and researchers attempt to distinguish between what is the researcher’s interpretation and what is an actual finding ( Kinnafick et al., 2014 ; Pope et al., 2000 ).
Results of Longitudinal Qualitative Research
The emergent nature of qualitative inquiry requires flexibility in research design, data collection and analyses. Defining the endpoint for analyses can be difficult and knowing at what point and in what format to disseminate your findings is equally challenging ( Thomson & Holland, 2003 ). Likewise, identifying a “gold standard” or “rules” that must be followed to ensure rigor is also a challenge and potentially less relevant as LQR research may be enriched by diverse strategies tailored to address specific research questions. Indeed, Nevedal et al. (2018) credits flexibility in LQR as a key facilitator that fosters innovation and creativity.
Ultimately, researchers aim to present results that speak to their original research objectives and in LQR, this includes a deeper understanding of the experience of change across time. Common outcomes presented in LQR publications are themes (and how they change across time), intervention development/evaluation, or conceptual pathways. For example, Clermont et al. (2018) were able to identify themes that explained decreased utilization of nutrient supplements in pregnant women despite their stated high level of acceptance. Mean-while, Corepal et al. (2018) used their qualitative study to better understand how and why an intervention designed to promote physical activity was effective among a group of adolescents. Findings from another LQR study among people living with HIV in Kenya provided key information to understand how and why a livelihood intervention impacted health behaviors ( Weiser et al., 2017 ). LQR may also identify changes in needs or levels of stress that can in turn be used to inform the development of supportive interventions ( Murray et al., 2009 ). Findings from LQR may ultimately be used by providers and case managers designing interventions to support an experience or transition that occurs across time such as a person transitioning from aggressive curative therapies to hospice care or an individual managing a progressive chronic illness (see Table 1 for additional examples).
Trustworthiness of Longitudinal Qualitative Research
The outcomes of the LQR must also adhere to a standard of rigor and quality that ensures meaningful qualitative findings. One way to describe this is by using the principle referred to as trustworthiness of data ( Polit & Beck, 2017 ). Trustworthiness of qualitative data is based on four principles, 1) credibility—how confident the researcher is in the truth of the data; 2) transferability—the extent to which the findings can be compared to similar populations in other settings; 3) confirmability—the degree to which research findings are based on participant narratives—the researcher was neutral in their analysis and interpretation and 4) dependability—the study design could be repeated with consistent findings ( Polit & Beck, 2017 ). Strategies to ensure each of these principles are met have been thoroughly discussed in the nursing literature (see Polit & Beck, 2017 ). In LQR, however, other considerations may be necessary to achieve trustworthiness.
In LQR there is often the need to make ongoing decisions about processes and procedures throughout the study including revising study guides or protocols, even if midway into the study. Changes may be essential to effectively achieve meaningful data that can be used to develop new knowledge ( Saldaña, 2003 ). That said, some qualitative researchers note that changing interview guides and formats can make it challenging to compare the responses of participants across time ( Kneck & Audulv, 2019 ). In contrast, Saldaña (2003) , argues that adjusting methods to enhance data richness allows the opportunity to gain greater descriptions that ultimately may serve a larger audience, thus satisfying the transferability principle of data trustworthiness. Transparency in reporting how and why decisions and changes to the study were made, is therefore vital to trustworthiness as it allows others to consider the decisions and changes that were made in conjunction with the researcher’s findings ( Sandelowski, 1986 ).
Discussion of Challenges in Longitudinal Qualitative Research
Despite clear benefits of LQR, there are several noteworthy challenges. First, depending on the objective of the study and the nature of the change being observed, researchers may be balancing a number of different logistical and conceptual challenges. Whether the study is investigating a disease state versus a significant life change will result in different participant experiences that may need varying amounts of time to capture the essence of that change; the amount of time needed may be a feasibility limitation for some researchers in terms of securing long-term funding as well as retaining participants. A second and related challenge is the labor-intensive nature of LQR which requires adequate funding to maintain research staff throughout the study period. Third, ethical considerations may be different for LQR versus cross sectional. By nature of LQR trying to ascertain a change across time, some studies may focus on enrolling youth or adolescents to follow over a certain number of years. This will require the careful consideration of consent processes, including the participants ability to consent and understand the objective of the study. In addition, informed consent should acknowledge the potential (albeit unknown) effects of long-term participation especially among young people whose life changes may be more unpredictable than middle-aged adults. Likewise, in cases where a person’s condition deteriorates, perhaps due to end of life, the ability to reconsent may be lost ( Murray et al., 2009 ). A fourth challenge that we note is LQR analyses are often poorly described in the literature making it difficult to follow the “recipe” (or even the thought processes) of other researchers with regard to how results were generated. This lack of explanation compromises the trustworthiness (more specifically the dependability) of the results.
Finally, a note about causality in LQR. Determining causality often requires longitudinal data to establish pathways where there is no doubt about the role of an independent variable on a dependent outcome. However, in terms of human experiences, causation is neither linear nor singular in many cases. Transitions are often impacted by multiple causes and may be better explained as “loops” versus “lines” ( Pettigrew, 1990 ). Causation is also shown when isolating independent and dependent variables to account for any confounding. However, transitions and behaviors are marked by convergent interactions and interconnected variables across time. Thus, LQR is well suited to establish or verify patterns of interactions and complex pathways but is not meant to show causation.
Summary and Conclusion
In summary, LQR provides a unique and important opportunity to understand human experiences across time within an individual and among a group using a more holistic, in-depth approach than is possible with retrospective or cross-sectional research alone. However, conducting LQR is complex and time consuming given the inherent contextual considerations of time and change and the many challenges and considerations unique to LQR. Ultimately, the task of exploring change is most effective when flexibility and acknowledgment of the process is considered at the outset. The main process elements include, managing large amounts of data; flexibility in data collection techniques to respond to data quality; sensitivity to many possible types of change that may be occurring; determining whether and in what ways these multiple types of change interrelate with each other; analyzing how and/why these changes occur; and pulling everything together in a complete and coherent report.
Ultimately, researchers must consider these complexities and processes alongside their research objectives to determine whether LQR is an appropriate choice. Our aim was to provide guidance on methodological considerations to aid the decision processes and support well informed study implementation.
Acknowledgments
The author(s) disclosed receipt of the following financial support for the research, authorship, and/or publication of this article: This work was supported by UCSF Center for AIDS Prevention Studies and UCSF Center for AIDS Research (ELT) and by the National Institutes of Health Grants K23MH116807 (ELT) and K01MH112443 (JAP).
Declaration of Conflicting Interests
The author(s) declared no potential conflicts of interest with respect to the research, authorship, and/or publication of this article.
- Baillie C, Smith J, Hewison J, & Mason G (2000). Ultrasound screening for chromosomal abnormality: Women’s reactions to false positive results . British Journal of Health Psychology , 5 ( 4 ), 377–394. 10.1348/135910700168991 [ CrossRef ] [ Google Scholar ]
- Balmer D, & Richards B (2017). Longitudinal qualitative research in medical education . Perspectives on Medical Education , 6 ( 5 ), 306–310. 10.1007/s40037-017-0374-9 [ PMC free article ] [ PubMed ] [ CrossRef ] [ Google Scholar ]
- Burnard P, Gill P, Stewart K, Treasure E, & Chadwick B (2008). Analysing and presenting qualitative data . British Dental Journal , ( 204 ), 429–432. 10.1038/sj.bdj.2008.292 [ PubMed ] [ CrossRef ] [ Google Scholar ]
- Calman L, Brunton L, & Molassiotis A (2013). Developing longitudinal qualitative designs: Lessons learned and recommendations for health services research . BMC Medical Research Methodology , 13 ( 14 ), 1–10. 10.1186/1471-2288-13-14 [ PMC free article ] [ PubMed ] [ CrossRef ] [ Google Scholar ]
- Carter S, & Little M (2007). Justifying knowledge, justifying method, taking action: Epistemologies, methodologies, and methods in qualitative research . Qualitative Health Research , 17 ( 10 ), 1316–1328. 10.1177/1049732307306927 [ PubMed ] [ CrossRef ] [ Google Scholar ]
- Chick N, & Meleis AI (1986). Transitions: A nursing concern. In Chinn PL (Ed.) Nursing research methodology (pp. 237–257). Aspen Publication. [ Google Scholar ]
- Chinn P, & Kramer M (Eds.). (2011). Integrated theory and knowledge development in nursing (8th ed.). Elsevier. [ Google Scholar ]
- Clermont A, Kodish SR, Matar Seck A, Salifou A, Rosen J, Grais RF, & Isanaka S (2018). Acceptability and utilization of three nutritional supplements during pregnancy: Findings from a longitudinal, mixed-methods study in Niger . Nutrients , 10 ( 8 ), 1073. 10.3390/nu10081073 [ PMC free article ] [ PubMed ] [ CrossRef ] [ Google Scholar ]
- Colaizzi P (1978). Psychological research as a phenomenologist views it. In Valle RS & King M (Eds.), Existential phenomenological alternatives for psychology . Open University Press. [ Google Scholar ]
- Cooper HL, Caruso B, Barham T, Embry V, Dauria E, Clark C, & Comfort M (2015). Partner incarceration and African-American women’s sexual relationships and risk: A longitudinal qualitative study . Journal of Urban Health , 92 ( 3 ), 527–547. 10.1007/s11524-015-9941-8 [ PMC free article ] [ PubMed ] [ CrossRef ] [ Google Scholar ]
- Corepal R, Best P, O’Neill R, Tully MA, Edwards M, Jago R, Miller SJ, Kee F, & Hunter RF (2018). Exploring the use of a gamified intervention for encouraging physical activity in adolescents: A qualitative longitudinal study in Northern Ireland . BMJ Open , 8 ( 4 ), e019663. 10.1136/bmjopen-2017-019663 [ PMC free article ] [ PubMed ] [ CrossRef ] [ Google Scholar ]
- De Chesnay M, & Abrums ME (2015). Nursing research using ethnography: Qualitative designs and methods . Springer Publishing Company. [ Google Scholar ]
- Doherty T, Chopra M, Nkonki L, Jackson D, & Persson L (2006). A longitudinal qualitative study of infant-feeding decision making and practices among HIV-positive women in South Africa . The Journal of Nutrition , 136 ( 9 ), 2421–2426. 10.1093/jn/136.9.2421 [ PubMed ] [ CrossRef ] [ Google Scholar ]
- Glanz K, Rimer BK, & Viswanath K (2008). Health behavior and health education: Theory, research, and practice (4th ed.). Jossey-Bass. [ Google Scholar ]
- Glaser BG (1978). Theoretical sensitivity: Advances in the methodology of grounded theory . Sociology Press. [ Google Scholar ]
- Glaser BG, & Strauss AL (1967). The discovery of grounded theory: Strategies for qualitative research . Aldine de Gruyter. [ Google Scholar ]
- Gray MA, & Smith LN (2000). The qualities of an effective mentor from the student nurse’s perspective: Findings from a longitudinal qualitative study . Journal of Advanced Nursing , 32 ( 6 ), 1542–1549. 10.1046/j.1365-2648.2000.01606.x [ PubMed ] [ CrossRef ] [ Google Scholar ]
- Grossoehme D, & Lipstein E (2016). Analyzing longitudinal qualitative data: The application of trajectory and recurrent cross-sectional approaches . BMC Research Notes , 9 ( 1 ), 136. 10.1186/s13104-016-1954-1 [ PMC free article ] [ PubMed ] [ CrossRef ] [ Google Scholar ]
- Guba EG, & Lincoln YS (1981). Effective evaluatio ., Jossey-Bass. [ Google Scholar ]
- Hatcher AM, Lemus Hufstedler E, Doria K, Dworkin SL, Weke E, Conroy A, Bukusi A, Cohen C, & Weiser SD (2019). Mechanisms and perceived mental health changes after a livelihood intervention for HIV-positive Kenyans: Longitudinal, qualitative findings . Transcultural Psychiatry , 57 ( 1 ), 124–139. 10.1177/1363461519858446 [ PMC free article ] [ PubMed ] [ CrossRef ] [ Google Scholar ]
- Henderson S, Holland J, McGrellis S, Sharpe S, & Thomson R (2012). Storying qualitative longitudinal research: Sequence, voice and motif . Qualitative Research , 12 ( 1 ), 16–34. 10.1177/1468794111426232 [ CrossRef ] [ Google Scholar ]
- Hennink MM, Kaiser BN, & Marconi VC (2017). Code saturation versus meaning saturation: How many interviews are enough? Qualitative Health Research , 27 ( 4 ), 591–608. 10.1177/1049732316665344 [ PMC free article ] [ PubMed ] [ CrossRef ] [ Google Scholar ]
- Hermanowicz J (2013). The longitudinal qualitative interview . Qualitative Sociology , 36 ( 2 ), 189–208. 10.1007/s11133-013-9247-7 [ CrossRef ] [ Google Scholar ]
- Jardine EE, McLellan J, & Dombrowski SU (2017). Is being resolute better than being pragmatic when it comes to breastfeeding? Longitudinal qualitative study investigating experiences of women intending to breastfeed using the theoretical domains framework . Journal of Public Health (Oxford, England) , 39 ( 3 ), e88–e94. 10.1093/pubmed/fdw073 [ PubMed ] [ CrossRef ] [ Google Scholar ]
- Johansen AB, Brendryen H, Darnell FJ, & Wennesland DK (2013). Practical support aids addiction recovery: The positive identity model of change . BMC Psychiatry , 13 ( 1 ), 201. 10.1186/1471-244X-13-201 [ PMC free article ] [ PubMed ] [ CrossRef ] [ Google Scholar ]
- Kinnafick F, Thøgersen-Ntoumani C, & Duda JL (2014). Physical activity adoption to adherence, lapse, and dropout . Qualitative Health Research , 24 ( 5 ), 706–718. 10.1177/1049732314528811 [ PubMed ] [ CrossRef ] [ Google Scholar ]
- Kneck Å , & Audulv Å . (2019). Analyzing variations in changes over time: Development of the pattern-oriented longitudinal analysis approach . Nursing Inquiry , 26 ( 3 ), e12288–n/a. 10.1111/nin.12288 [ PubMed ] [ CrossRef ] [ Google Scholar ]
- Lewis J (2007). Analysing qualitative longitudinal research in evaluations . Social Policy and Society , 6 ( 4 ), 545–556. 10.1017/S1474746407003880 [ CrossRef ] [ Google Scholar ]
- McCoy LK (2017). Longitudinal qualitative research and interpretative phenomenological analysis: Philosophical connections and practical considerations . Qualitative Research in Psychology , 14 ( 4 ), 442–458. 10.1080/14780887.2017.1340530 [ CrossRef ] [ Google Scholar ]
- McLeod J, & Thoon R (2009). Researching social change . Sage Publications. https://uk.sagepub.com/en-gb/eur/researching-social-change/book229849 [ Google Scholar ]
- Meleis AI, Sawyer LM, Im E, Hilfinger Messias DK, & Schumacher K (2000). Experiencing transitions: An emerging middle-range theory . Advances in Nursing Science , 23 ( 1 ), 12–28. 10.1097/00012272-200009000-00006 [ PubMed ] [ CrossRef ] [ Google Scholar ]
- Miles M, Huberman M, & Saldaña J (Eds.). (2014). Qualitative data analysis: A methods sourcebook (3rd ed.). Sage. [ Google Scholar ]
- Murray SA, Kendall M, Carduff E, Worth A, Harris FM, Lloyd A, Cavers D, Grant L, & Sheikh A (2009). Use of serial qualitative interviews to understand patients’ evolving experiences and needs . The BMJ , 339 ( 7727 ), 958–960. 10.1136/bmj.b3702 [ PubMed ] [ CrossRef ] [ Google Scholar ]
- Namukwaya E, Murray S, Downing J, Leng M, & Grant L (2017). I think my body has become addicted to those tablets’. Chronic heart failure patients’ understanding of and beliefs about their illness and its treatment: A qualitative longitudinal study from Uganda . PLoS One , 12 ( 9 ), e0182876. 10.1371/journal.pone.0182876 [ PMC free article ] [ PubMed ] [ CrossRef ] [ Google Scholar ]
- Nevedal AL, Ayalon L, & Briller SH (2018). A Qualitative evidence synthesis review of longitudinal qualitative research in gerontology . The Gerontologist , 59 ( 6 ), e791–e801. 10.1093/geront/gny134 [ PubMed ] [ CrossRef ] [ Google Scholar ]
- Oosterveld-Vlug MG, Pasman HRW, van Gennip IE, Willems DL, & Onwuteaka-Philipsen BD (2013). Changes in the personal dignity of nursing home residents: A longitudinal qualitative interview study . PLoS One , 8 ( 9 ), e73822. 10.1371/journal.pone.0073822 [ PMC free article ] [ PubMed ] [ CrossRef ] [ Google Scholar ]
- Pellowski JA, Harrison AD, Colvin CJ, Brittain K, Rini Z, Cu-Uvin S, Myer L, Operario D, & Lurie MN (2019, October, 21–22). HIV treatment adherence among pregnant and postpartum women in Cape Town, South Africa: Using transition theory to analyze longitudinal qualitative data [Poster presentation] . Inter-CFAR Women and HIV Symposium, Chicago, IL, United States. [ Google Scholar ]
- Pelto PJ (2013). Applied ethnography: Guidelines for field research . Left Coast Press. [ Google Scholar ]
- Pettigrew AM (1990). Longitudinal field research on change: Theory and practice . Organization Science , 1 ( 3 ), 267–292. 10.1287/orsc.1.3.267 [ CrossRef ] [ Google Scholar ]
- Polit D, & Beck CT (Eds.). (2017). Nursing research: Generating and assessing evidence for nursing practice (10th ed.). Wolters Kluwer Health. [ Google Scholar ]
- Pope C, Ziebland S, & Mays N (2000). Analysing qualitative data . The BMJ , 320 ( 7227 ), 114. 10.1136/bmj.320.7227.114 [ PMC free article ] [ PubMed ] [ CrossRef ] [ Google Scholar ]
- Riessman C (1993). Narrative analysis . Sage Publications. [ Google Scholar ]
- Saldaña J (2003). Longitudinal qualitative research . Rowman & Littlefield Publishers. [ Google Scholar ]
- Saldaña J (2009). Coding manual for qualitative researchers . Sage Publications. [ Google Scholar ]
- Salter C, McDaid L, Bhattacharya D, Holland R, Marshall T, & Howe A (2014). Abandoned acid? Understanding adherence to bisphosphonate medications for the prevention of osteoporosis among older women: A qualitative longitudinal study . PloS One , 9 ( 1 ), e83552. 10.1371/journal.pone.0083552 [ PMC free article ] [ PubMed ] [ CrossRef ] [ Google Scholar ]
- Sandelowski M (1986). The problem of rigor in qualitative research . Advances in Nursing Science , 8 ( 3 ), 27–37. [ PubMed ] [ Google Scholar ]
- Schmidt B, Schoppe-Sullivan S, Frizzo G, & Piccinini C (2019). A qualitative multiple case study of the division of labor across the transition to parenthood in south-Brazilian families . Sex Roles , 81 ( 5 ), 272–289. 10.1007/s11199-018-0999-0 [ CrossRef ] [ Google Scholar ]
- Sheard L, & Marsh C (2019). How to analyse longitudinal data from multiple sources in qualitative health research: The pen portrait analytic technique . BMC Medical Research Methodology , 19 ( 1 ), 169. 10.1186/s12874-019-0810-0 [ PMC free article ] [ PubMed ] [ CrossRef ] [ Google Scholar ]
- Smith N (2003). Cross-sectional profiling and longitudinal analysis: Research notes on analysis in the longitudinal qualitative study, ‘negotiating transitions to citizenship’ . International Journal of Social Research Methodology , 6 ( 3 ), 273–277. 10.1080/1364557032000091888 [ CrossRef ] [ Google Scholar ]
- SmithBattle L, Lorenz R, Reangsing C, Palmer JL, & Pitroff G (2018). A methodological review of qualitative longitudinal research in nursing . Nursing Inquiry , 25 ( 4 ), e12248–n/a. 10.1111/nin.12248 [ PubMed ] [ CrossRef ] [ Google Scholar ]
- Solomon P, O’Brien KK, Nixon S, Letts L, Baxter L, & Gervais N (2018a). Qualitative longitudinal study of episodic disability experiences of older women living with HIV in Ontario, Canada . BMJ Open , 8 ( 4 ), e021507. 10.1136/bmjopen-2018-021507 [ PMC free article ] [ PubMed ] [ CrossRef ] [ Google Scholar ]
- Solomon P, O’Brien KK, Nixon S, Letts L, Baxter L, & Gervais N (2018b). Trajectories of episodic disability in people aging with HIV: A longitudinal qualitative study . Journal of the International Association of Providers of AIDS Care (JIAPAC) , 17 , 1–7. 10.1177/2325958218759210 [ PMC free article ] [ PubMed ] [ CrossRef ] [ Google Scholar ]
- Thomson R (2007). The qualitative longitudinal case history: Practical, methodological and ethical reflections . Social Policy and Society , 6 ( 4 ), 571–582. 10.1017/S1474746407003909 [ CrossRef ] [ Google Scholar ]
- Thomson R, & Holland J (2003). Hindsight, foresight and insight: The challenges of longitudinal qualitative research . International Journal of Social Research Methodology , 6 ( 3 ), 233–244. 10.1080/1364557032000091833 [ CrossRef ] [ Google Scholar ]
- Tracy SJ (2010). Qualitative quality: Eight “big-tent” criteria for excellent qualitative research . Qualitative Inquiry , 16 ( 10 ), 837–851. 10.1177/1077800410383121 [ CrossRef ] [ Google Scholar ]
- Weiser S, Hatcher A, Hufstedler L, Weke E, Shari DL, Bukusi EA, Burger RL, Kodish S, Grede N, Butler LM, & Cohen C (2017). Changes in health and antiretroviral adherence among HIV-infected adults in Kenya: Qualitative longitudinal findings from a livelihood intervention . AIDS and Behavior , 21 ( 2 ), 415–427. 10.1007/s10461-016-1551-2 [ PMC free article ] [ PubMed ] [ CrossRef ] [ Google Scholar ]
- Zeilani R, & Seymour JE (2010). Muslim women’s experiences of suffering in Jordanian intensive care units: A narrative study . Intensive & Critical Care Nursing , 26 ( 3 ), 175–184. 10.1016/j.iccn.2010.02.002 [ PubMed ] [ CrossRef ] [ Google Scholar ]
- Zeilani R, & Seymour JE (2012). Muslim women’s narratives about bodily change and care during critical illness: A qualitative study . Journal of Nursing Scholarship , 44 ( 1 ), 99–107. 10.1111/j.1547-5069.2011.01427 [ PubMed ] [ CrossRef ] [ Google Scholar ]

Home » Pros and Cons » 23 Advantages and Disadvantages of Longitudinal Studies
23 Advantages and Disadvantages of Longitudinal Studies
Longitudinal studies are a form of observational research that is used to collect data. When this type of study is performed, a set of data is collected from each subject over a defined period. The same subjects are used for the research, which means the study can sometimes last for months, if not years.
It is the type of research that is most commonly performed when seeking out information in medical, sociological, or psychological arenas.
Here are the top advantages and disadvantages of longitudinal studies to consider when designing a research study.
Top Advantages of Longitudinal Studies
1. longitudinal studies make it easier to find long-term patterns..
Many research studies focus on short-term data alone. That means long-term data may offer patterns or information that cannot be collected. A longitudinal study would be able to collect that long-term data and locate patterns within it that can benefit the field being researched. This occurs because changes can be tracked over time as the same subjects are being used, allowing cause-and-effect relationships to be found.
2. They offer high validity levels through the collected data.
Because longitudinal studies are a long-term research project, there must be extensive policies and procedures in place from the very beginning of the project. These policies and procedures then dictate the direction of the study, requiring researchers to follow their outline. Because every data collection effort follows this establish protocol, longitudinal studies have high levels of validity because there is a certainty to the authenticity of the research. People can have confidence in the conclusions which are generated.
3. It uses observational methods for data collection.
Most longitudinal studies utilize observational data collection methods because of the long-term nature and design of the research. That makes the data much easier to collect when compared to other study formats. It also provides consistent data that can be applied across all developed metrics. Researches can then use this information to exclude differences or outliers in the data, increasing the overall accuracy of the research while excluding their own personal basis.
4. The format limits the number of mistakes that can be made.
Researchers may wish to draw their own conclusions from the data being collected, but the format of longitudinal studies prevents this from happening. That means from the data collection process to the conclusions that are published, the information being presented has a limited number of mistakes contained within it. That allows the data to be used to create needed changes within the researched field immediately, so that the rest of society can benefit from the conclusions.
5. It offers unique and authentic data.
Because longitudinal studies go directly to the source of information for data, what is collected is often authentic and unique. The principles of data collection allow for long-term relationships to be discovered within the data while short-term benefits can still be collected. It essentially offers the best of both worlds to researchers. Using the results from short-term data, researchers can pursue long-term data that may not have been considered when the study was first implemented.
6. There is more flexibility to be found within longitudinal studies.
Longitudinal studies can be designed to track a single data point. They can also be designed to track multiple metrics simultaneously. Researchers can pivot within the study if they find interesting or unique patterns in the data being collected. Data relationships can be pursued to determine if there is something meaningful within that information. At any time, a shift in focus is permitted if an interesting data point is discovered, which allows for the results and conclusions to be more complete when compared to other study formats and styles.
7. Data collection occurs in real-time.
Longitudinal studies happen in real-time, which means the data being collected is reflective of “now.” That makes it possible for researchers to expand questions or follow tangents based on the responses they receive from each individual. It creates more engagement, which builds a relationship between the subject and the researcher, and that can eventually lead to new insights that might have been held back otherwise.
8. It provides for multiple forms of data collection.
Human memory is a fickle beast. Two people can witness the same event and remember it in very different ways. This occurs because everyone has a unique perspective and their own experiences that affect their memory. For that reason, longitudinal studies offer multiple methods of data collection to ensure the accuracy of what is being collected. Video or audio recordings, diaries, journals, blogs – they can all contribute to the database of information that researchers collect, and then mine, to find conclusions that can be reached.
9. Longitudinal studies make an effort not to manipulate the environment.
This is the advantage of the observational approach that is used with this type of study. Instead of manipulating the environment to produce data, researchers simply collect the data that they can detect with their 5 senses. Asking questions may seem like world manipulation to some. What interviews provide is a way to access personalized information. As long as the researcher is proceeding based on what they observe, the manipulation effect is not present within the researcher.
10. It can be used to pursue developmental trends.
Longitudinal research is often used to determine if developmental trends have any future benefit. It is especially important when considering practical application fields like family medicine to determine better methodologies that can be applied to the general population. Researchers can also pursue trends that they observe on their own to see if there is any correlation between the subjects that would explain the information that they are seeing.
11. They can be used to establish a specific sequence of events.
One person who lives to the age of 100 can be an outlier in any community. Their combination of genetics, healthy living, and optimism, unique to them, can help them live long and happy lives. In a small community, having several people reach the age of 100 is no longer an outlier. There is the potential that there is something present within their environment that is encouraging longer life. Longitudinal studies make it possible to establish a specific sequence of events that would lead to such an outcome, offering the opportunity for that sequence to be duplicated elsewhere.
12. It corrects for the cohort effect.
Whenever there is a large group of people coming together for research purposes, you’re going to have a wide variety of individualized time components. These range from their date of birth, to their current age at the time of the study, to their overall net worth. The long-term nature of this type of study helps to correct any effects that might develop because of these differences, which reduces the individuality of the data that is collected.
Top Disadvantages of Longitudinal Studies
1. the format allows one person to influence the outcome of the study..
Longitudinal studies rely on the expertise, creativity, and honesty of individual researchers for authentic conclusions. That reliance on the individual makes it possible for the data to be corrupted or conclusions to be inaccurate. It does not need to be a purposeful manipulation of the data for the outcomes to be falsified. Misinterpreting data can be just as damaging as purposely misleading the collection of the data and the results would be difficult, at first, to be proven invalid.
2. It offers direct costs that are much higher than other research styles.
Larger sample sizes are required for longitudinal research than in other styles. This requirement is in place because more data is necessary in long-term studies to determine if there are relationships or patterns within the data. That means the direct costs of performing a longitudinal study are typically higher when compared to other research methods. More people must be contacted. More data must be examined. More time must be dedicated to the project. Other research formats do not face these challenges.
3. Long-term studies often see sample sizes change over time.
Larger sample sizes are required for a longitudinal study because of the nature of life. People eventually grow old and die. There may be accidents or natural disasters that occur during the data collection period for the study as well. That means some data may need to be excluded from the research to avoid providing false results and it may be difficult to locate the data that must be removed.
4. It can be difficult to locate willing participants.
Would you want to be tracked over the next 20 years of your life about something? There are three groups of people that researchers encounter when creating a longitudinal study. One group are enthusiastic and willing to help in whatever way they can. One group despises the idea of being contacted by researchers on a regular basis for a long time. The final group doesn’t really care and may not offer accurate data to researchers because of their apathy. Researchers look for enthusiastic participants for authentic data and those folks aren’t always easy to find.
5. Longitudinal research relies heavily on the expertise of each researcher.
Data collection in longitudinal research occurs in real-time, but that means it relies on the skills of the researcher to collect. The average researcher didn’t go to school for journalism, so their ability to recognize follow-up questions or focus on specific data points can be limited. The quality of the data that is collected relies on the quality of the expertise that the researcher brings to the project. That means the quality of the data can also vary if multiple researchers are collecting data.
6. It can have questions of data accuracy.
With a longitudinal study, it only requires one inaccurate data point to throw the entire validity of the study into question. That inaccurate data point can even invalidate years of research and it doesn’t have to be a purposeful collection to cause problems. Researchers are human, just like the rest of us. They have a personal bias toward certain subjects or certain data points that influence how they work, whether they realize it or now. It is not uncommon for researchers to draw conclusions about the data they are collecting before they’ve even collected the information.
7. Time is always an issue with a longitudinal study.
Researchers that begin a longitudinal study may not see the results that their study is able to generate. Some studies take several years to complete. One study, reported on by the Harvard Gazette, discusses a longitudinal study that will be 80 years old in 2018. Scientists began tracking the health of 268 Harvard sophomores in 1938 and as of 2017, 19 of them were still alive. The information collected from this study is important, but it has taken nearly a century for the results to be conclusive. As more data is collected, more time and resources must be utilized to go through the data and look for relationships as well.
8. Study participants may not offer authentic information.
Longitudinal studies involve the same participants over a long period of time. What happens to them can affect the data that is collected. It can also influence how future data is collected. All of this is based on a working assumption that the individual involved is being honest and forthright with the researcher. Some people may stop participating altogether. Unless there are policies and procedures in place that can help to prevent these outcomes, the validity of the study could be questioned before all the data is even collected.
9. Funding is a major challenge for longitudinal studies.
The costs of a longitudinal study don’t need to be prohibitive, but there are factors and influences that can make it difficult to justify the expense. Outside of the actual research cost, many studies of this type must budget for incentives or rewards to encourage subjects to continue their participation. Then, since participants are essentially compensated for providing data, researchers must ensure the information is valid because there will always be critics that question its validity. Some see the compensation of participants as a form of bribery.
10. Inaccuracies are common when analyzing data that is collected by this type of study.
Longitudinal studies often seen inaccuracies during the analysis of the data that is collected. These inaccuracies often arise when hypothesis testing is applied to the collected data, much like it would be for other types of research, such as a cross-sectional study. When this occurs with a longitudinal study, the available data is often under-utilized, which increases the chances of a statistical error occurring.
11. The variable being studied may disappear over time.
Even with the best planning process enacted, policies and procedures cannot stop the fact that a studied variable may disappear from the population group being studied. Should such an event occur, the time and money invested into the research study would be wasted because no valid conclusions could be drawn from the collected data.
The advantages and disadvantages of longitudinal studies direct us toward the unique patterns and relationships of life. Data that is collected is authentic and predictable, which allows researchers to draw conclusions from their findings. For this type of study to work properly, however, there must be procedures in place from the very beginning to remove bias, inaccurate data, and other negative influences.
In return, the data collected has the potential to alter our perspectives in a number of fields.
Related Posts:
- 21 Best SMART Goals Examples for Teachers and Educators
- 10 Proven Psychological Pricing Strategies with Examples
- 50 Best Side Hustles for Accountants
- 30 Best Student Action Plan Examples
- Open access
- Published: 24 February 2024
Physical activity improves stress load, recovery, and academic performance-related parameters among university students: a longitudinal study on daily level
- Monika Teuber 1 ,
- Daniel Leyhr 1 , 2 &
- Gorden Sudeck 1 , 3
BMC Public Health volume 24 , Article number: 598 ( 2024 ) Cite this article
4154 Accesses
30 Altmetric
Metrics details
Physical activity has been proven to be beneficial for physical and psychological health as well as for academic achievement. However, especially university students are insufficiently physically active because of difficulties in time management regarding study, work, and social demands. As they are at a crucial life stage, it is of interest how physical activity affects university students' stress load and recovery as well as their academic performance.
Student´s behavior during home studying in times of COVID-19 was examined longitudinally on a daily basis during a ten-day study period ( N = 57, aged M = 23.5 years, SD = 2.8, studying between the 1st to 13th semester ( M = 5.8, SD = 4.1)). Two-level regression models were conducted to predict daily variations in stress load, recovery and perceived academic performance depending on leisure-time physical activity and short physical activity breaks during studying periods. Parameters of the individual home studying behavior were also taken into account as covariates.
While physical activity breaks only positively affect stress load (functional stress b = 0.032, p < 0.01) and perceived academic performance (b = 0.121, p < 0.001), leisure-time physical activity affects parameters of stress load (functional stress: b = 0.003, p < 0.001, dysfunctional stress: b = -0.002, p < 0.01), recovery experience (b = -0.003, p < 0.001) and perceived academic performance (b = 0.012, p < 0.001). Home study behavior regarding the number of breaks and longest stretch of time also shows associations with recovery experience and perceived academic performance.
Conclusions
Study results confirm the importance of different physical activities for university students` stress load, recovery experience and perceived academic performance in home studying periods. Universities should promote physical activity to keep their students healthy and capable of performing well in academic study: On the one hand, they can offer opportunities to be physically active in leisure time. On the other hand, they can support physical activity breaks during the learning process and in the immediate location of study.
Peer Review reports
Introduction
Physical activity (PA) takes a particularly key position in health promotion and prevention. It reduces risks for several diseases, overweight, and all-cause mortality [ 1 ] and is beneficial for physical, psychological and social health [ 2 , 3 , 4 , 5 ] as well as for academic achievement [ 6 , 7 ]. However, PA levels decrease from childhood through adolescence and into adulthood [ 8 , 9 , 10 ]. Especially university students are insufficiently physically active according to health-oriented PA guidelines [ 11 ] because of academic workloads as well as difficulties in time management regarding study, work, and social demands [ 12 ]. Due to their independence and increasing self-responsibility, university students are at a crucial life stage. In this essential and still educational stage of the students´ development, it is important to study their PA behavior. Furthermore, PA as health behavior represents one influencing factor which is considered in the analytical framework of the impact of health and health behaviors on educational outcomes which was developed by the authors Suhrcke and de Paz Nieves [ 13 , 14 ]. In light of this, the present study examines how PA affects university students' academic situations.
Along with the promotion of PA, the reduction of sedentary behavior has also become a crucial part of modern health promotion and prevention strategies. Spending too much time sitting increases many health risks, including the risk of obesity [ 15 ], diabetes [ 16 ] and other chronic diseases [ 15 ], damage to muscular balances, bone metabolism and musculoskeletal system [ 17 ] and even early death [ 15 ]. University students are a population that has shown the greatest increase in sedentary behavior over the last two decades [ 18 ]. In Germany, they show the highest percentage of sitting time among all working professional groups [ 19 ]. Long times sitting in classes, self-study learning, and through smartphone use, all of which are connected to the university setting and its associated behaviors, might be the cause of this [ 20 , 21 ]. This goes along with technological advances which allow students to study in the comfort of their own homes without changing locations [ 22 ].
To counter a sedentary lifestyle, PA is crucial. In addition to its physical health advantages, PA is essential for coping with the intellectual and stress-related demands of academic life. PA shows positive associations with stress load and academic performance. It is positively associated with learning and educational success [ 6 ] and even shows stress-regulatory potential [ 23 ]. In contrast, sedentary behavior is associated with lower cognitive performance [ 24 ]. Moreover, theoretical derivations show that too much sitting could have a negative impact on brain health and diminish the positive effects of PA [ 16 ]. Given the theoretical background of the stressor detachment model [ 25 ] and the cybernetic approach to stress management in the workplace [ 26 ], PA can promote recovery experience, it can enhance academic performance, and it is a way to reduce the impact of study-related stressors on strain. Load-related stress response can be bilateral: On the one hand, it can be functional if it is beneficial to help cope with the study demands. On the other hand, it can be dysfunctional if it puts a strain on personal resources and can lead to load-related states of strain [ 27 ]. Thus, both, the promotion of PA and reduction of sedentary behavior are important for stress load, recovery, and performance in student life, which can be of particular importance for students in an academic context.
A simple but (presumably) effective way to integrate PA and reduce sedentary behavior in student life are short PA breaks. Due to the exercises' simplicity and short duration, students can perform them wherever they are — together in a lecture or alone at home. Short PA breaks could prevent an accumulation of negative stressors during the day and can help with prolonged sitting as well as inactivity. Especially in the university setting, evidence of the positive effects of PA breaks exists for self-perceived physical and psychological well-being of the university students [ 28 ]. PA breaks buffer university students’ perceived stress [ 29 ] and show positive impacts on recovery need [ 30 ] and better mood ratings [ 31 , 32 ]. In addition, there is evidence for reduction in tension [ 30 ], overall muscular discomfort [ 33 ], daytime sleepiness or fatigue [ 33 , 34 ] and increase in vigor [ 34 ] and experienced energy [ 30 ]. This is in line with cognitive, affective, behavioral, and biological effects of PA, all categorized as palliative-regenerative coping strategies, which addresses the consequences of stress-generating appraisal processes aiming to alleviate these consequences (palliative) or restore the baseline of the relevant reaction parameter (regenerative) [ 35 , 36 ]. This is achieved by, for example, reducing stress-induced cortisol release or tension through physical activity (reaction reduction) [ 35 ]. Such mechanisms are also in accordance with the previously mentioned stressor detachment model [ 25 ]. Lastly, there is a health-strengthening effect that impacts the entire stress-coping-health process, relying on the compensatory effects of PA which is in accordance to the stress-buffering effect of exercise [ 37 ]. Health, in turn, effects educational outcomes [ 13 , 14 ]. Therefore, stress regulating effects are also accompanied with the before mentioned analytical framework of the impact of health and health behaviors on educational outcomes [ 13 , 14 ].
Focusing on the effects of PA, this study is guided by an inquiry into how PA affects university students' stress load and recovery as well as their perceived academic performance. For that reason, the student´s behavior during home studying in times of COVID-19 is examined, a time in which reinforced prolonged sitting, inactivity, and a negative stress load response was at a high [ 38 , 39 , 40 , 41 , 42 ]. Looking separately on the relation of PA with different parameters based on the mentioned evidence, we assume that PA has a positive impact on stress load, recovery, and perceived academic performance-related parameters. Furthermore, a side effect of the home study behavior on the mentioned parameters is assumed regarding the accumulation of negative stressors during home studying. These associations are presented in Fig. 1 and summarized in the following hypotheses:
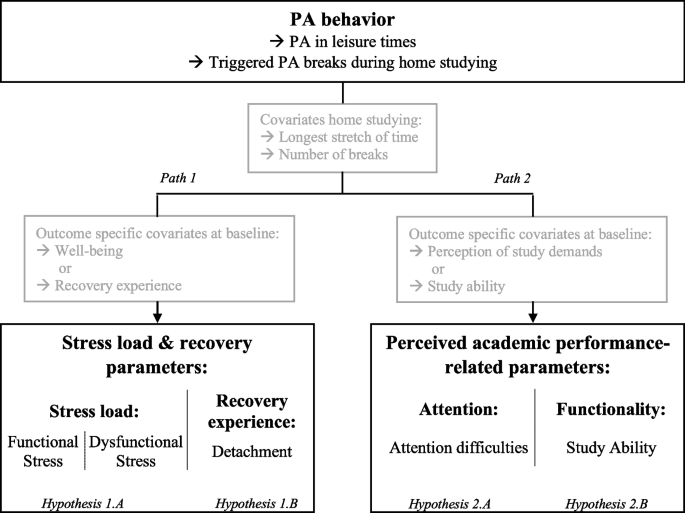
Overview of the assumed effects and investigated hypotheses of physical activity (PA) behavior on variables of stress load and recovery and perceived academic performance-related parameters
Hypothesis 1 (path 1): Given that stress load always occurs as a duality—beneficial if it is functional for coping, or exhausting if it puts a strain on personal resources [ 27 ] – we consider two variables for stress load: functional stress and dysfunctional stress. In order to reduce the length of the daily surveys, we focused the measure of recovery only on the most obvious and accessible component of recovery experience, namely psychological detachment. PA (whether performed in leisure-time or during PA breaks) encourages functional stress and reduce dysfunctional stress (1.A) and has a positive effect on recovery experience through psychological detachment (1.B).
Hypothesis 2 (path 2): The academic performance-related parameters attention difficulties and study ability are positively influenced by PA (whether done in leisure-time or during PA breaks). We have chosen to assess attention difficulties for a cognitive parameter because poor control over the stream of occurring stimuli have been associated with impairment in executive functions or academic failure [ 43 , 44 , 45 , 46 ]. Furthermore, we have assessed the study ability to refer to the self-perceived feeling of functionality regarding the demands of students. PA reduces self-reported attention difficulties (2.A) and improves perceived study ability, indicating that a student feels capable of performing well in academic study (2.B).
Hypothesis 3: We assume that a longer time spent on studying at home (so called home studying) could result in higher accumulation of stressors throughout the day which could elicit immediate stress responses, while breaks in general could reduce the influence of work-related stressors on strain and well-being [ 47 , 48 ]. Therefore, the following covariates are considered for secondary effects:
the daily longest stretch of time without a break spent on home studying
the daily number of breaks during home studying
Study setting
The study was carried out during the COVID-19 pandemic containment phase. It took place in the middle of the lecture period between 25th of November and 4th of December 2020. Student life was characterized by home studying and digital learning. A so called “digital semester” was in effect at the University of Tübingen when the study took place. Hence, courses were mainly taught online (e.g., live or via a recorded lecture). Other events and actions at the university were not permitted. As such, the university sports department closed in-person sports activities. For leisure time in general, there were contact restrictions (social distancing), the performance of sports activities in groups was not permitted, and sports facilities were closed.
Thus, the university sports department of the University of Tübingen launched various online sports courses and the student health management introduced an opportunity for a new digital form of PA breaks. This opportunity provided PA breaks via videos with guided physical exercises and health-promoting explanations for a PA break for everyday home studying: the so called “Bewegungssnack digital” [in English “exercise snack digital” (ESD)] [ 49 ]. The ESD videos took 5–7 min and were categorized into three thematic foci: activation, relaxation, and coordination. Exercises were demonstrated by one or two student exercise leaders, accompanied by textual descriptions of the relevant execution features of each exercise.
Participants
Participants were recruited within the framework of an intervention study, which was conducted to investigate whether a digital nudging intervention has a beneficial effect on taking PA breaks during home study periods [ 49 ]. Students at the University of Tübingen which counts 27,532 enrolled students were approached for participation through a variety of digital means: via an email sent to those who registered for ESD course on the homepage of the university sports department and to all students via the university email distribution list; via advertisement on social media of the university sports department (Facebook, Instagram, YouTube, homepage). Five tablets, two smart watches, and one iPad were raffled off to participants who engaged actively during the full study period in an effort to motivate them to stick with it to the end. In any case, participants knew that the study was voluntary and that they would not suffer any personal disadvantages should they opt out. There was a written informed consent prompt together with a prompt for the approval of the data protection regulations immediately within the first questionnaire (T0) presented in a mandatory selection field. Positive ethical approval for the study was given by the first author´s institution´s ethics committee of the faculty of the University of Tübingen.
Participants ( N = 57) who completed the daily surveys on at least half of the days of the study period, were included in the sample (male = 6, female = 47, diverse = 1, not stated = 3). As not all subjects provided data on all ten study days, the total number of observations was between 468 and 540, depending on the variable under study (see Table 1 ). The average number of observations per subject was around eight. Their age was between 18 and 32 years ( M = 23.52, SD = 2.81) and they were studying between the 1st to 13th semester ( M = 5.76, SD = 4.11) within the following major courses of study: mathematical-scientific majors (34.0%), social science majors (22.6%), philosophical majors (18.9%), medicine (13.2%), theology (5.7%), economics (3.8%), or law (1.9%). 20.4% of the students had on-site classroom teaching on university campus for at least one day a week despite the mandated digital semester, as there were exceptions for special forms of teaching.
Design and procedures
To examine these hypothesized associations, a longitudinal study design with daily surveys was chosen following the suggestion of the day-level study of Feuerhahn et al. (2014) and also of Sonnentag (2001) measuring recovery potential of (exercise) activities during leisure time [ 50 , 51 ]. Considering that there are also differences between people at the beginning of the study period, initial base-line value variables respective to the outcomes measured before the study period were considered as independent covariates. Therefore, the well-being at baseline serves as a control for stress load (2.A), the psychological detachment at baseline serves as a control for daily psychological detachment (2.B), the perception of study demands serves as a control for self-reported attention difficulties (1.A), and the perceived study ability at baseline serves as a control for daily study ability (2.B).
Subjects were asked to continue with their normal home study routine and additionally perform ESD at any time in their daily routine. Data were collected one to two days before (T0) as well as daily during the ten-day study period (Wednesday to Friday). The daily surveys (t 1 -t 10 ) were sent by email at 7 p.m. every evening. Each day, subjects were asked to answer questions about their home studying behavior, study related requirements, recovery experience from study tasks, attention, and PA, including ESD participation. The surveys were conducted online using the UNIPARK software and were recorded and analyzed anonymously.
Measures and covariates
In total, five outcome variables, two independent variables, and seven covariates were included in different analyses: three variables were used for stress load and recovery parameters, two variables for academic performance-related parameters, two variables for PA behavior, two variables for study behavior, four variables for outcome specific baseline values and one variable for age.
Outcome variables
Stress load & recovery parameters (hypothesis 1).
Stress load was included in the analysis with two variables: functional stress and dysfunctional stress. Followingly, a questionnaire containing a word list of adjectives for the recording of emotions and stress during work (called “Erfassung von Emotionen und Beanspruchung “ in German, also known as EEB [ 52 ]) was used. It is an instrument which were developed and validated in the context of occupational health promotion. The items are based on mental-workload research and the assessment of the stress potential of work organization [ 52 ]. Within the questionnaire, four mental and motivational stress items were combined to form a functional stress scale (energetic, willing to perform, attentive, focused) (α = 0.89) and four negative emotional and physical stress items were combined to form dysfunctional stress scale (nervous, physically tensioned, excited, physically unwell) (α = 0.71). Participants rated the items according to how they felt about home studying in general on the following scale (adjustment from “work” to “home studying”): hardly, somewhat, to some extent, fairly, strongly, very strongly, exceptionally.
Recovery experience was measured via psychological detachment. Therefore, the dimension “detachment” of the Recovery Experience Questionnaire (RECQ [ 53 ]) was adjusted to home studying. The introductory question was "How did you experience your free time (including short breaks between learning) during home studying today?". Students responded to four statements based on the extent to which they agreed or disagreed (not at all true, somewhat true, moderately true, mostly true, completely true). The statements covered subjects such as forgetting about studying, not thinking about studying, detachment from studying, and keeping a distance from student tasks. The four items were combined into a score for psychological detachment (α = 0.94).
Academic performance-related parameters (hypothesis 2)
Attention was assessed via the subscale “difficulty maintaining focused attention performance” of the “Attention and Performance Self-Assessment” (ASPA, AP-F2 [ 54 ]). It contains nine items with statements about disturbing situations regarding concentration (e.g. “Even a small noise from the environment could disturb me while reading.”). Participants had to answer how often such situations happened to them on a given day on the following scale: never, rarely, sometimes, often, always. The nine items were combined into the AP-F2 score (α = 0.87).
The perceived study ability was assessed using the study ability index (SAI [ 55 ]). The study ability index captures the current state of perceived functioning in studying. It is based on the Work Ability Index by Hasselhorn and Freude ([ 56 ]) and consists of an adjusted short scale of three adapted items in the context of studying. Firstly, (a) the perceived academic performance was asked after in comparison to the best study-related academic performance ever achieved (from 0 = completely unable to function to 10 = currently best functioning). Secondly, the other two items were aimed at assessing current study-related performance in relation to (b) study tasks that have to be mastered cognitively and (c) the psychological demands of studying. Both items were answered on a five-point Likert scale (1 = very poor, 2 = rather poor, 3 = moderate, 4 = rather good, 5 = very good). A sum index, the SAI, was formed which can indicate values between 2 and 20, with higher values corresponding to higher assessed functioning in studies (α = 0.86). In a previous study it already showed satisfying reliability (α = 0.72) [ 55 ].
Independent variables
Pa behavior.
Two indicators for PA behavior were included via self-reports: the time spent on ESD and the time spent on leisure-time PA (LTPA). Participants were asked the following overarching question daily: “How much time did you spend on physical activity today and in what context”. For the independent variable time spent on PA breaks, participants could answer the option “I participated in the Bewegungssnack digital” with the amount of time they spent on it (in minutes). To assess the time spent on LTPA besides PA breaks, participants could report their time for four different contexts of PA which comprised two forms: Firstly, structured supervised exercise was reported via time spent on (a) university sports courses and (b) other organized sports activities. Secondly, self-organized PA was indicated via (c) independent PA at home, such as a workout or other physically demanding activity such as cleaning or tidying up, as well as via (d) independent PA outside, like walking, cycling, jogging, a workout or something similar. Referring to the different domains of health enhancing PA [ 57 ], the reported minutes of these four types of PA were summed up to a total LTPA value. The total LTPA value was included in the analysis as a metric variable in minutes.
Covariates (hypothesis 3)
Regarding hypothesis 3 and home study behavior, the longest daily stretch of time without a break spent on home studying (in hours) and the daily number of breaks during home studying was assessed. Therein, participants had to answer the overarching question “How much time did you spend on your home studying today?” and give responses to the items: (1) longest stretch of time for home studying (without a break), and (2) number of short and long breaks you took during home studying.
In principle, efforts were made to control for potential confounders at the individual level (level 2) either by including the baseline measure (T0) of the respective variable or by including variables assessing related trait-like characteristics for respective outcomes. The reason why related trait-like characteristics were used for the outcomes was because brief assessments were used for daily surveys that were not concurrently employed in the baseline assessment. To enable the continued use of controlling for person-specific baseline characteristics in the analysis of daily associations, trait-like characteristics available from the baseline assessment were utilized as the best possible approximation.To sum up, four outcome specific baseline value variables were measured before the study period (at T0). The psychological detachment with the RECQ (α = 0.87) [ 53 ] was assessed at the beginning to monitor daily psychological detachment. Further, the SAI [ 55 ] was assessed at the beginning of the study period to monitor daily study ability. To monitor daily stress load, which in part measures mental stress aspects and negative emotional stress aspects, the well-being was assessed at the beginning using the WHO-Five Well-being Index (WHO-5 [ 58 ]). It is a one-dimensional self-report measure with five items. The index value is the sum of all items, with higher values indicating better well-being. As the well-being and stress load tolerance may linked with each other, this variable was assumed to be a good fit with the daily stress load indicating mental and emotional stress aspects. With respect to student life, daily academic performance-related attention was monitored with an instrument for the perception of study demands and resources (termed “Berliner Anforderungen Ressourcen-Inventar – Studierende” in German, the so-called BARI-S [ 59 ]). It contains eight items which capture overwork in studies, time pressure during studies, and the incompatibility of studies and private life. All together they form the BARI-S demand scale (α = 0.85) which was included in the analysis. As overwork and time pressure may result in attention difficulties (e.g. Elfering et al., 2013), this variable was assumed to have a good fit with academic performance-related attention [ 60 ]. Additionally, age in years at T0 was considered as a sociodemographic factor.
Statistical analysis
Since the study design provided ten measurement points for various people, the hierarchical structure of the nested data called for two-level analyses. Pre-analyses of Random-Intercept-Only models for each of the outcome variables (hypothesis 1 to 3) revealed an Intra-Class-Correlation ( ICC ) of at least 0.10 (range 0.26 – 0.64) and confirmed the necessity to perform multilevel analyses [ 61 ]. Specifically, the day-level variables belong to Level 1 (ESD time, LTPA time, longest stretch of time without a break spent on home studying, daily number of breaks during home studying). To analyze day-specific effects within the person, these variables were centered on the person mean (cw = centered within) [ 50 , 62 , 63 , 64 ]. This means that the analyses’ findings are based on a person’s deviations from their average values. The variables assessed at T0 belong to Level 2, which describe the person level (psychological detachment baseline, SAI baseline, well-being, study demands scale, age). These covariates on person level were centered around the grand mean [ 50 ] indicating that the analyses’ findings are based how far an individual deviates from the sample's mean values. As a result, the models’ intercept reflects the outcome value of an average student in the sample at his/her daily average behavior in PA and home study when all parameters are zero. For descriptive statistics SPSS 28.0.1.1 (IBM) and for inferential statistics R (version 4.1.2) were used. The hierarchical models were calculated using the package lme4 with the lmer-function in R in the following steps [ 65 ]. The Null Model was analyzed for all models first, with the corresponding intercept as the only predictor. Afterwards, all variables were entered. The regression coefficient estimates (”b”) were considered for statistical significance for the models and the respective BIC was provided.
In total, five regression models with ‘PA break time’ and ‘LTPA time’ as independent variables were computed due to the five measured outcomes of the present study. Three models belonged to hypothesis 1 and two models to hypothesis 2.
Hypothesis 1: To test hypothesis 1.A two outcome variables were chosen for two separate models: ‘functional stress’ and ‘dysfunctional stress’. Besides the PA behavior variables, the ‘number of breaks’, the ‘longest stretch of time without a break spent on home studying’, ‘age’, and the ‘well-being’ at the beginning of the study as corresponding baseline variable to the output variable were also included as independent variables in both models. The outcome variable ‘psychological detachment’ was utilized in conjunction with the aforementioned independent variables to test hypotheses 1.B, with one exception: psychological detachment at the start of the study was chosen as the corresponding baseline variable.
Hypothesis 2: To investigate hypothesis 2.A the outcome variable ‘attention difficulties’ was selected. Hypothesis 2.B was tested with the outcome variables ‘study ability’. Both models included both PA behavior variables as well as the ‘number of breaks’, the ‘longest stretch of time without a break spent on home studying’, ‘age’ and one corresponding baseline variable each: the ‘study demand scale’ at the start of the study for ‘attention difficulties’ and the ‘SAI’ at the beginning of the study for the daily ‘study ability’.
Hypothesis 3: In addition to both PA behavior variables, age and one baseline variable that matched the outcome variable, the covariates ‘daily longest stretch of time spent on home studying’ and ‘daily number of breaks during home studying’ were included in the models for all five outcome variables.
Handling missing data
The dataset had up to 18% missing values (most exhibit the variables ‘daily longest stretch of time without a break spent on home studying’ with 17.89% followed by ‘daily number of breaks during homes studying’ with 16.67%, and ‘functional / dysfunctional stress’ with 12.45%). Therefore, a sensitivity analysis was performed using the multiple imputation mice-package in the statistical program R [ 66 ], the package howManyImputation based on Von Hippel (2020, [ 67 ]), and the additional broom package [ 68 ]. The results of the models remained the same, with one exception for the Attention Difficulties Model: The daily longest stretch of time without a break spent on home studying showed a significant association (Table 1 in supplement). Due to this almost perfect consistency of results between analyses based on the dataset with missing data and those with imputed data alongside the lack of information provided by the packages for imputed datasets, we decided to stick with the main analysis including the missing data. Thus, in the following the results of the main analysis without imputations are presented.
Table 1 shows the descriptive statistics of the variables used in the analysis. An overview of the analysed models is presented in Table 2 .
Effects on stress load and recovery (hypothesis 1)
Hypothesis 1.A: The Model Functional Stress explained 13% of the variance by fixed factors (marginal R 2 = 0.13), and 52% by both fixed and random factors (conditional R 2 = 0.52). The time spent on ESD as well as the time spent on PA in leisure showed a positive significant influence on functional stress (b = 0.032, p < 0.01). The same applied to LTPA (b = 0.003, p < 0.001). The Model Dysfunctional Stress (marginal R 2 = 0.027, conditional R 2 = 0.647) showed only one significant result. The dysfunctional stress was only significantly negatively influenced by the time spent on LTPA (b = 0.002, p < 0.01).
Hypothesis 1.B: With the Model Detachment, fixed factors contributed 18% of the explained variance and fixed and random factors 46% of the explained variance for psychological detachment. Only the amount of time spent on LTPA revealed a positive impact on psychological detachment (b = 0.003, p < 0.001).
Effects on academic performance-related parameters (hypothesis 2)
Hypothesis 2.A: The Model Attention Difficulties showed 13% of the variance explained by fixed factors, and 51% explained by both fixed and random factors. It showed a significant negative association only for the time spent on LTPA (b = 0.003, p < 0.001).
Hypothesis 2.B: The Model SAI showed 18% of the variance explained by fixed factors, and 39% explained by both fixed and random factors. There were significant positive associations for time spent on ESD (b = 0.121, p < 0.001) and time spent on LTPA (b = 0.012, p < 0.001). The same applied to LTPA (b = 0.012, p < 0.001).
Effects of home study behavior (hypothesis 3)
Regarding the independent covariates for the outcome variables functional and dysfunctional stress, there were no significant results for the number of breaks during homes studying or the longest stretch of time without a break spent on home studying. Considering the outcome variable ‘psychological detachment’, there were significant results with negative impact for both study behavior variables: breaks during home studying (b = 0.058, p < 0.01) and daily longest stretch of time without a break (b = 0.120, p < 0.01). Evaluating the outcome variables ‘attention difficulties’, there were no significant results for the number of breaks during home studying or the longest stretch of time without a break spent on home studying. Testing the independent study behavior variables for the SAI, it increased with increasing number in daily breaks during homes studying relative to the person´s mean (b = 0.183, p < 0.05). No significant effect was found for the longest stretch of time without a break spent on home studying ( p = 0.07).
The baseline covariates of the models showed expected associations and thus confirmed their inclusion. The baseline variables well-being showed a significant impact on functional stress (b = 0.089, p < 0.001), psychological detachment showed a positive effect on the daily output variables psychological detachment (b = 0.471, p < 0.001), study demand scale showed a positive association on difficulties in attention (b = 0.240, p < 0.01), and baseline SAI had a positive effect on the daily SAI (b = 0.335, p < 0.001).
The present study theorized that PA breaks and LTPA positively influence the academic situation of university students. Therefore, impact on stress load (‘functional stress’ and ‘dysfunctional stress’) and ‘psychological detachment’ as well as academic performance-related parameters ‘self-reported attention difficulties’ and ‘perceived study ability’ was taken into account. The first and second hypotheses assumed that both PA breaks and LTPA are positively associated with the aforementioned parameters and were confirmed for LTPA for all parameters and for PA breaks for functional stress and perceived study ability. The third hypothesis assumed that home study behavior regarding the daily number of breaks during home studying and longest stretch of time without a break spent on home studying has side effects. Detected negative effects for both covariates on psychological detachment and positive effects for the daily number of breaks on perceived study ability were partly unexpected in their direction. These results emphasize the key position of PA in the context of modern health promotion especially for students in an academic context.
Regarding hypothesis 1 and the detected positive associations for stress load and recovery parameters with PA, the results are in accordance with the stress-regulatory potential of PA from the state of research [ 23 ]. For hypothesis 1.A, there is a positive influence of PA breaks and LTPA on functional stress and a negative influence of LTPA on dysfunctional stress. Given the bilateral role of stress load, the results indicate that PA breaks and LTPA are beneficial for coping with study demands, and may help to promote feelings of joy, pride, and learning progress [ 27 ]. This is in line with previous evidence that PA breaks in lectures can buffer university students’ perceived stress [ 29 ], lead to better mood ratings [ 29 , 31 ], and increase in motivation [ 28 , 69 ], vigor [ 34 ], energy [ 30 ], and self-perceived physical and psychological well-being [ 28 ]. Looking at dysfunctional stress, the result point that LTPA counteract load-related states of strain such as inner tension, irritability and nervous restlessness or feelings of boredom [ 27 ]. In contrast, short PA breaks during the day could not have enough impact in countering dysfunctional stress at the end of the day regarding the accumulation of negative stressors during home studying which might have occurred after the participant took PA breaks. Other studies have been able to show a reduction in tension [ 30 ] and general muscular discomfort [ 33 ] after PA breaks. However, this was measured as an immediate effect of PA breaks and not with general evening surveys. Blasche and colleagues [ 34 ] measured effects immediately and 20 min after different kind of breaks and found that PA breaks led to an additional short‐ and medium‐term increase in vigor while the relaxation break lead to an additional medium‐term decrease in fatigue compared to an unstructured open break. This is consistent with the results of the present study that an effect of PA breaks is only observed for functional stress and not for dysfunctional stress. Furthermore, there is evidence that long sitting during lectures leads to increased fatigue and lower concentration [ 31 , 70 ], which could be counteracted by PA breaks. For both types of stress loads, functional and dysfunctional stress, there is an influence of students´ well-being in this study. This shows that the stress load is affected by the way students have mentally felt over the last two weeks. The relevance of monitoring this seems important especially in the time of COVID-19 as, for example, 65.3% of the students of a cross-sectional online survey at an Australian university reported low to very low well-being during that time [ 71 ]. However, since PA and well-being can support functional stress load, they should be of the highest priority—not only as regards the pandemic, but also in general.
Looking at hypothesis 1.B; while there is a positive influence of LTPA on experienced psychological detachment, no significant influence for PA breaks was detected. The fact that only LTPA has a positive effect can be explained by the voluntary character of the activity [ 50 ]. The voluntary character ensures that stressors no longer affect the student and, thus, recovery as detachment can take place. Home studying is not present in leisure times, and thus detachment from study is easier. The PA break videos, on the other hand, were shot in a university setting, which would have made it more difficult to detach from study. In order to further understand how PA breaks affect recovery and whether there is a distinction between PA breaks and LTPA, future research should also consider other types of recovery (e.g. relaxation, mastery, and control). Additionally, different types of PA breaks, such as group PA breaks taken on-site versus video-based PA breaks, should be taken into account.
Considering the confirmed positive associations for academic performance-related parameters of hypothesis 2, the results are in accordance with the evidence of positive associations between PA and learning and educational success [ 6 ], as well as between PA breaks and better cognitive functioning [ 28 ]. Looking at the self-reported attention difficulties of hypothesis 2.A, only LTPA can counteract it. PA breaks showed no effects, contrary to the results of a study of Löffler and collegues (2011, [ 31 ]), in which acute effects of PA breaks could be found for higher attention and cognitive performance. Furthermore, the perception of study demands before the study periods has a positive impact on difficulties in attention. That means that overload in studies, time pressure during studies, and incompatibility of studies and private life leads to higher difficulties with attention in home studying. In these conditions, PA breaks might have been seen as interfering, resulting in the expected beneficial effects of exercise on attention and task-related participation behavior [ 72 , 73 ] therefore remaining undetected. With respect to the COVID-19 pandemic, accompanying education changes, and an increase in student´s worries [ 74 , 75 ], the perception of study demands could be affected. This suggests that especially in times of constraint and changes, it is important to promote PA in order to counteract attention difficulties. This also applies to post-pandemic phase.
Regarding the perceived academic performance of hypothesis 2.B, both PA breaks and LTPA have a positive effect on perceived study ability. This result confirms the positive short-term effects on cognition tasks [ 76 ]. It is also in line with the positive function of PA breaks in interrupting sedentary behavior and therefore counteracting the negative association between sitting behavior and lower cognitive performance [ 24 ]. Additionally, this result also fits with the previously mentioned positive relationship between LTPA and functional stress and between PA breaks and functional stress.
According to hypothesis 3, in relation to the mentioned stress load and recovery parameters, there are negative effects of the daily number of breaks during home studying and the longest stretch of time without a break spent on home studying on psychological detachment. As stressors result in negative activation, which impede psychological detachment from study during non-studying time [ 25 ], it was expected and confirmed that the longest stretch of time without a break spent on home studying has a negative effect on detachment. Initially unexpected, the number of breaks has a negative influence on psychological detachment, as breaks could prevent the accumulation of strain reactions. However, if the breaks had no recovery effect through successful detachment, the number might not have any influence on recovery via detachment. This is indicated by the PA breaks, which had no impact on psychological detachment. Since there are other ways to recover from stress besides psychological detachment, such as relaxation, mastery, and control [ 53 ], PA breaks must have had an additional impact in relation to the positive results for functional stress.
In relation to the mentioned academic performance-related parameters, only the number of breaks has a positive influence on the perceived study ability. This indicates that not only PA breaks but also breaks in general lead to better perceived functionality in studying. Paulus and colleagues (2021) found out that an increase in cognitive skills is not only attributed to PA breaks and standing breaks, but also to open breaks with no special instructions [ 28 ]. Either way, they found better improvement in self-perceived physical and psychological well-being of the university students with PA breaks than with open breaks. This is also reflected in the present study with the aforementioned positive effects of PA breaks on functional stress, which does not apply to the number of breaks.
Overall, it must be considered that the there is a more complex network of associations between the examined parameters. The hypothesized separate relation of PA with different parameters do not consider associations between parameters of stress load / recovery and academic performance although there might be a interdependency. Furthermore, moderation aspects were not examined. For example, PA could be a moderator which buffer negative effects of stress on the study ability [ 55 ]. Moreover, perceived study ability might moderate stress levels and academic performance. Further studies should try to approach and understand the different relationships between the parameters in its complexity.
Limitations
Certain limitations must be taken into account. Regarding the imbalanced design toward more female students in the sample (47 female versus 6 male), possible sampling bias cannot be excluded. Gender research on students' emotional states during COVID-19, when this study took place, or students´ acceptance of PA breaks is diverse and only partially supplied with inconsistent findings. For example, during the COVID-19 pandemic, some studies reported that female students were associated with lower well-being [ 71 ] or worse mental health trajectories [ 75 , 77 ]. Another study with a large sample of students from 62 countries reported that male students were more strongly affected by the pandemic because they were significantly less satisfied with their academic life [ 74 ]. However, Keating and colleges (2020) discovered that, despite the COVID-19 pandemic, females rated some aspects of PA breaks during lectures more positively than male students did. However, this was also based on a female slanted sample [ 78 ]. Further studies are needed to get more insights into gender bias.
Furthermore, the small sample size combined with up to 16% missing values comprises a significant short-coming. There were a lot of possibilities which could cause such missing data, like refused, forgotten or missed participation, technical problems, or deviation of the personal code for the questionnaire between survey times. Although the effects could be excluded by sensitive analysis due to missing data, the sample is still small. To generalize the findings, future replication studies are needed.
Additionally, PA breaks were only captured through participation in the ESD, the specially instructed PA break via video. Effects of other short PA breaks were not include in the study. However, participants were called to participate in ESD whenever possible, so the likelihood that they did take part in PA breaks in addition to the ESD could be ignored.
With respect to the baseline variables, it must be considered that two variables (stress load, attention difficulties) were adjusted not with their identical variable in T0, but with other conceptually associated variables (well-being index, BARI-S). Indeed, contrary to the assumption the well-being index does only show an association with functional stress, indicating that it does not control dysfunctional stress. Although the other three assumed associations were confirmed there might be a discrepancy between the daily measured variables and the variables measured in T0. Further studies should either proof the association between these used variables or measure the same variables in T0 for control the daily value of these variables.
Moreover, the measuring instruments comprised the self-assessed perception of the students and thus do not provide an objective information. This must be considered, especially for measuring cognitive and academic-performance-related measures. Here, existing objective tests, such as multiple choice exams after a video-taped lecture [ 72 ] might have also been used. Nevertheless, such methods were mostly used in a lab setting and do not reflect reality. Due to economic reasons and the natural learning environment, such procedures were not applied in this study. However, the circumstances of COVID-19 pandemic allowed a kind of lab setting in real life, as there were a lot of restrictions in daily life which limited the influence of other covariates. The study design provides a real natural home studying environment, producing results that are applicable to the healthy way that students learn in the real world. As this study took place under the conditions of COVID-19, new transformations in studying were also taken into account, as home studying and digital learning are increasingly part of everyday study.
However, the restrictions during the COVID-19 pandemic could result in a greater extent of leisure time per se. As the available leisure time in general was not measured on daily level, it is not possible to distinguish if the examined effects on the outcomes are purely attributable to PA. It is possible that being more physical active is the result of having a greater extent of leisure time and not that PA but the leisure time itself effected the examined outcomes. To address this issue in future studies, it is necessary to measure the proportion of PA in relation to the leisure time available.
Furthermore, due to the retrospective nature of the daily assessments of the variables, there may be overstated associations which must be taken into account. Anyway, the daily level of the study design provides advantages regarding the ability to observe changes in an individual's characteristics over the period of the study. This design made it possible to find out the necessity to analyze the hierarchical structure of the intraindividual data nested within the interindividual data. The performed multilevel analyses made it possible to reflect the outcome of an average student in the sample at his/her daily average behavior in PA and home study.
Conclusion and practical implications
The current findings confirm the importance of PA for university students` stress load, recovery experience, and academic performance-related parameters in home studying. Briefly summarized, it can be concluded that PA breaks positively affect stress load and perceived study ability. LTPA has a positive impact on stress load, recovery experience, and academic performance-related parameters regarding attention difficulties and perceived study ability. Following these results, universities should promote PA in both fashions in order to keep their students healthy and functioning: On the one hand, they should offer opportunities to be physically active in leisure time. This includes time, environment, and structural aspects. The university sport department, which offers sport courses and provides sport facilities on university campuses for students´ leisure time, is one good example. On the other hand, they should support PA breaks during the learning process and in the immediate location of study. This includes, for example, providing instructor videos for PA breaks to use while home studying, and furthermore having instructors to lead in-person PA breaks in on-site learning settings like universities´ libraries or even lectures and seminars. This not only promotes PA, but also reduces sedentary behavior and thereby reduces many other health risks. Further research should focus not only on the effect of PA behavior but also of sedentary behavior as well as the amount of leisure time per se. They should also try to implement objective measures for example on academic performance parameters and investigate different effect directions and possible moderation effects to get a deeper understanding of the complex network of associations in which PA plays a crucial role.
Availability of data and materials
The datasets used and analyzed during the current study are available from the corresponding author on reasonable request.
Abbreviations
Attention and Performance Self-Assessment
"Berliner Anforderungen Ressourcen-Inventar – Studierende" (instrument for the perception of study demands and resources)
Centered within
Grand centered
“Erfassung von Emotionen und Beanspruchung “ (questionnaire containing a word list of adjectives for the recording of emotions and stress during work)
Exercise snack digital (special physical activity break offer)
Intra-Class-Correlation
Leisure time physical activity
- Physical activity
Recovery Experience Questionnaire
Study ability index
World Health Organization-Five Well-being index
Knight JA. Physical inactivity: associated diseases and disorders. Ann Clin Lab Sci. 2012;42(3):320–37.
PubMed Google Scholar
Kemel PN, Porter JE, Coombs N. Improving youth physical, mental and social health through physical activity: a systematic literature review. Health Promot J Austr. 2002;33(3):590–601.
Article Google Scholar
Gothe NP, Ehlers DK, Salerno EA, Fanning J, Kramer AF, McAuley E. Physical activity, sleep and quality of life in older adults: influence of physical, mental and social well-being. Behav Sleep Med. 2020;18(6):797–808.
Article PubMed Google Scholar
Eime RM, Young JA, Harvey JT, Charity MJ, Payne WR. A systematic review of the psychological and social benefits of participation in sport for children and adolescents: informing development of a conceptual model of health through sport. Int J Behav Nutr Phys Act. 2013;10(1):1–21.
Google Scholar
Iannotti RJ, Janssen I, Haug E, Kololo H, Annaheim B, Borraccino A. Interrelationships of adolescent physical activity, screen-based sedentary behaviour, and social and psychological health. Int J Public Health. 2009;54:191–8.
Dadaczynski K, Schiemann S. Welchen Einfluss haben körperliche Aktivität und Fitness im Kindes-und Jugendalter auf Bildungsoutcomes? German J Exerc Sport Res. 2015;4(45):190–9.
Kari JT, Pehkonen J, Hutri-Kähönen N, Raitakari OT, Tammelin TH. Longitudinal associations between physical activity and educational outcomes. Med Sci Sports Exerc. 2017;49(11).
Grim M, Hortz B, Petosa R. Impact evaluation of a pilot web-based intervention to increase physical activity. Am J Health Promot. 2011;25(4):227–30.
Irwin JD. Prevalence of university students’ sufficient physical activity: A systematic review. Percept Mot Skills. 2004;98:927–43.
Kwan MY, Cairney J, Faulkner GE, Pullenayegum EE. Physical activity and other health-risk behaviors during the transition into early adulthood: a longitudinal cohort study. Am J Prev Med. 2012;42(1):14–20.
John JM, Gropper H, Thiel A. The role of critical life events in the talent development pathways of athletes and musicians: A systematic review. Psychol Sport Exerc. 2019;45.
Bopp M, Bopp C, Schuchert M. Active transportation to and on campus is associated with objectively measured fitness outcomes among college students. J Phys Act Health. 2015;12(3):418–23.
Dadaczynski K. Stand der Forschung zum Zusammenhang von Gesundheit und Bildung. Überblick und Implikationen für die schulische Gesundheitsförderung. Zeitschrift für Gesundheitspsychologie. 2012;20(3):141–53
Suhrcke M, de Paz NC. The impact of health and health behaviours on educational outcomes in high-income countries: a review of the evidence. Copenhagen: WHO Regional Offi ce for Europe; 2011.
Lynch BM, Owen N. Too much sitting and chronic disease risk: steps to move the science forward. Ann Intern Med. 2015;16(2):146–7.
Voss MW, Carr LJ, Clark R, Weng T. Revenge of the “sit” II: Does lifestyle impact neuronal and cognitive health through distinct mechanisms associated with sedentary behavior and physical activity? Ment Health Phys Act. 2014;7(1):9–24.
Huber G. Ist Sitzen eine tödliche Aktivität? B&G Bewegungstherapie und Gesundheitssport. 2014;30(01):13–6.
Peterson NE, Sirard JR, Kulbok PA, DeBoer MD, Erickson JM. Sedentary behavior and physical activity of young adult university students. Res Nurs Health. 2018;4(1):30–8.
Rupp R, Dold C, Bucksch J. Sitzzeitreduktion und Bewegungsaktivierung in der Hochschullehre – Entwicklung und Implementierung der Mehrebenen-Intervention Kopf-Stehen. Die Hochschullehre. 2019;5:525–42.
Ickes MJ, McMullen J, Pflug C, Westgate PM. Impact of a University-based Program on Obese College Students’ Physical Activity Behaviors, Attitudes, and Self-efficacy. Am J Health Educ. 2016;47(1):47–55.
Lepp A, Barkley JE, Karpinski AC. The relationship between cell phone use and academic performance in a sample of US college students. Sage Open. 2015;5(1).
Stapp AC, Prior LF. The Impact of Physically Active Brain Breaks on College Students’ Activity Levels and Perceptions. J Physic Activ Res. 2018;3(1):60–7.
Fuchs R, Klaperski S. Sportliche Aktivität und Stressregulation. In: Fuchs R, Schlicht W, editors. Sportaktivität und seelische Gesundheit Göttingen: Hogrefe; 2012. p. 100–21.
Falck RS, Davis JC, Liu-Ambrose T. What is the association between sedentary behaviour and cognitive function? A systematic review. Br J Sports Med. 2017;51(10):800–11.
Sonnentag S, Fritz C. Recovery from job stress: The stressor-detachment model as an integrative framework. J Organ Behav. 2015;36:72–103.
Edwards JR. A cybernetic theory of stress, coping, and well-being in organizations. Acad Manag Rev. 1992;17(2):238–74.
Wieland R. Status-Bericht: Psychische Gesundheit in der betrieblichen Gesundheitsförderung – eine arbeitspsychologische Perspektive. In: Nold H, Wenninger G, editors. Rückengesundheit und psychische Gesundheit. Rückengesundheit und psychische Gesundheit.: Asanger Verlag; 2013.
Paulus M, Kunkel J, Schmidt SCE, Bachert P, Wäsche H, Neumann R, et al. Standing breaks in lectures improve university students’ self-perceived physical, mental, and cognitive condition. Int J Environ Res Public Health. 2021;18.
Marschin V, Herbert C. A Short, Multimodal Activity Break Incorporated Into the Learning Context During the Covid-19 Pandemic: Effects of Physical Activity and Positive Expressive Writing on University Students’ Mental Health — Results and Recommendations From a Pilot Study. Front Psychol. 2021;12.
Gollner E, Savil M, Schnabel F, Braun C, Blasche G. Unterschiede in der Wirksamkeit von Kurzpausenaktivitäten im Vergleich von Bewegungspausen zu psychoregulativen Pausen bei kognitiver Belastung. Bewegungstherapie Gesundheitssport. 2019;35:134–43.
Löffler SN, Dominok E, von Haaren B, Schellhorn R, Gidion G. Aktivierung, Konzentration, Entspannung: Interventionsmöglichkeiten zur Förderung fitnessrelevanter Kompetenzen im Studium: KIT Scientific Publishing; 2011.
Marschin V, Herbert C. A short, multimodal activity break incorporated into the learning context during the Covid-19 pandemic: effects of physical activity and positive expressive writing on university students' mental health—results and recommendations from a pilot study. Front Psychol. 2021.
Kowalsky RJ, Farney TM, Hearon CM. Resistance Exercise Breaks Improve Ratings of Discomfort and Sleepiness in College Students. Res Q Exerc Sport. 2022;94(1):210–5.
Blasche G, Szabo B, Wagner-Menghin M, Ekmekcioglu C, Gollner E. Comparison of rest-break interventions during a mentally demanding task. Stress Health. 2018;34(5):629–38.
Article PubMed PubMed Central Google Scholar
Fuchs R, Klaperski S. Stressregulation durch Sport und Bewegung. In: Fuchs R, Gerber M, editors. Handbuch Stressregulation und Sport. Berlin: Springer; 2018. p. 205–26.
Chapter Google Scholar
Kaluza G, Renneberg B. Stressbewältigung. In: Bengel J, Jerusalem M, editors. HandbuchGesundheitspsychologie und medizinische Psychologie. Göttingen: Hogrefe; 2009. p. 265–72.
Klaperski S. Exercise, Stress and Health: The Stress-Buffering Effect of Exercise. In: Fuchs R, Gerber M, editors. Handbuch Stressregulation und Sport. Berlin: Springer; 2018. p. 227–50.
Lesser IA, Nienhuis CP. The impact of COVID-19 on physical activity behavior and well-being of Canadians. Int J Environ Res Public Health. 2020;17(11):3899.
Article CAS PubMed PubMed Central Google Scholar
Moore SA, Faulkner G, Rhodes RE, Brussoni M, Chulak-Bozzer T, Ferguson LJ, et al. Impact of the COVID-19 virus outbreak on movement and play behaviours of Canadian children and youth: a national survey. Int J Behav Nutr Phys Act. 2020;17(1):1–11.
Rodríguez-Larrad A, Mañas A, Labayen I, González-Gross M, Espin A, Aznar S, et al. Impact of COVID-19 confinement on physical activity and sedentary behaviour in Spanish university students: Role of gender. Int J Environ Res Public Health. 2021;18(2):369.
Stanton R, To QG, Khalesi S, Williams SL, Alley SJ, Thwaite TL, et al. Depression, anxiety and stress during COVID-19: associations with changes in physical activity, sleep, tobacco and alcohol use in Australian adults. Int J Environ Res Public Health. 2020;17(11):4065.
Zheng C, Huang WY, Sheridan S, Sit CHP, Chen XK, Wong SHS. COVID-19 pandemic brings a sedentary lifestyle in young adults: a cross-sectional and longitudinal study. Int J Environ Res Public Health. 2020;17(17):6035.
Commodari E. Attention Skills and Risk of Developing Learning Difficulties. Curr Psychol. 2012;31:17–34.
Commodari E, Guarnera M. Attention and reading skills. Percept Mot Skills. 2005;100:3753–86.
Raaijmakers MAJ, Smidts DP, Sergeant JA, Maassen GH, Posthumus JA, van Engeland H, et al. Executive functions in preschool children with aggressive behavior: impairments in inhibitory control. J Abnorm Child Psychol. 2008;36:1097–107.
Vellutino FR, Scanlon DM, Sipay ER, Small SG, Pratt A, Chen RS, et al. Cognitive profiles of difficulty to remediate and readily remediate poor readers: early intervention as a vehicle for distinguishing between cognitive and experiential deficits as basic of specific reading disability. J Educ Psychol. 1996;88:601–38.
Ilies R, Dimotakis N, De Pater IE. Psychological and physiological reactions to high workloads: Implications for well-being. Pers Psychol. 2010;63(2):407–36.
Rodell JB, Judge TA. Can “good” stressors spark “bad” behaviors? The mediating role of emotions in links of challenge and hindrance stressors with citizenship and counterproductive behaviors. J Appl Psychol. 2009;94(6).
Teuber M, Leyhr D, Moll J, Sudeck G. Nudging digital physical activity breaks for home studying of university students—A randomized controlled trial during the COVID-19 pandemic with daily activity measures. Front Sports Active Living. 2022;4.
Feuerhahn N, Sonnentag S, Woll A. Exercise after work, psychological mediators, and affect: A day-level study. Eur J Work Organ Psy. 2014;23(1):62–79.
Sonnentag S. Work, Recovery Activities, ans Individual Well-Being: A Diary Study. J Occup Health Psychol. 2001;6(3):196–210.
Article CAS PubMed Google Scholar
Wieland R. Gestaltung gesundheitsförderlicher Arbeitsbedingungen. In: Kleinbeck U, Schmidt K-H, editors. Arbeitspsychologie (Enzyklopädie der Psychologie, Serie Wirtschafts-, Organisations- und Arbeitspsychologie). 1. Göttingen: Hogrefe; 2010. p. 869–919.
Sonnentag S, Fritz C. The Recovery Experience Questionnaire: development and validation of a measure for assessing recuperation and unwinding from work. J Occup Health Psychol. 2007;12(3):204–21.
Bankstahl US, Görtelmeyer R. Measuring subjective complaints of attention and performance failures development and psychometric validation in tinnitus of the self-assessment scale APSA. Health and Quality of Life Outcomes. 2013;11(86).
Teuber M, Arzberger I, Sudeck G. Körperliche Aktivität, Gesundheit und Funktionsfähigkeit im Studium: Sportliche Freizeitaktivitäten und aktive Fortbewegung als Ressource im Studium? In: Göring A, Mayer J, Jetzke M, editors. Sport und Studienerfolg - Analysen zur Bedeutung sportlicher Aktivität im Setting Hochschule. Hochschulsport: Bildung und Wissenschaft, 4. Göttingen: Universitätsverlag Göttingen; 2020. p. 27–49.
Hasselhorn H-M, Freude G. Der Work-Ability-Index: ein Leitfaden In: Arbeitsmedizin BfAu, editor. Dortmund/Berlin/Dresden: Wirtschaftsverl. NW, Verlag für Neue Wissenschaft GmbH; 2007.
Rütten A, Pfeifer K. Nationale Empfehlungen für Bewegung und Bewegungsförderung. Köln: Bundeszentrale für Gesundheitliche Aufklärung (BZgA); 2017.
Brähler E, Mühlan H, Albani C, Schmidt S. Teststatistische Prüfung und Normierung der deutschen Versionen des EUROHIS-QOL Lebensqualität-Index und des WHO-5 Wohlbefindens-Index. Diagnostica. 2007;53(2):83–96.
Gusy B, Wörfel F, Lohmann K. Erschöpfung und Engagement im Studium. Zeitschrift für Gesundheitspsychologie. 2016;24(1):41–53.
Elfering A, Grebner S, de Tribolet-Hardy F. The long arm of time pressure at work: Cognitive failure and commuting near-accidents. Eur J Work Organ Psy. 2013;22(6):737–49.
Kreft IG, de Leeuw J. Introducing multilevel modeling. London: Sage; 1998.
Book Google Scholar
Bates D, Mächler M, Bolker BM, Walker SC. Fitting Linear Mixed-Effects Models Using lme4. J Stat Softw. 2015;67(1):1–48.
Hofmann DA, Gavin MB. Centering decisions in hierarchical linear models: Implications for research in organizations. J Manag. 1998;24(5):623–41.
Nezlek J. Diary Studies in Social and Personality Psychology: An Introduction With Some Recommendations and Suggestions. Social Psychological Bulletin. 2020;15(2).
Knapp G. Gemischte Modelle in R. Begleitskriptum zur Weiterbildung. In: Dortmund TU, editor. Braunschweig2019.
Van Buuren S, Groothuis-Oudshoorn K. mice: Multivariate imputation by chained equations in R. J Stat Softw. 2011;45:1–67.
von Hippel PT. How Many Imputations Do You Need? A Twostage Calculation Using a Quadratic Rule. Sociological Methods & Research. 2020;49(3):699–718.
Article MathSciNet Google Scholar
Robinson D. broom: An R package for converting statistical analysis objects into tidy data frames. arXiv preprint arXiv:14123565. 2014.
Young-Jones A, McCain J, Hart B. Let’s Take a Break: The Impact of Physical Activity on Academic Motivation. Int J Teach Learn High Educ. 2022;33(3):110–8.
Barr-Anderson DJ, AuYoung M, Whitt-Glover MC, Glenn BA, Yancey AK. Integration of short bouts of physical activity into organizational routine: A systematic review of the literature. Am J Prev Med. 2011;40(1):76–93.
Dodd RH, Dadaczynski K, Okan O, McCaffery KJ, Pickles K. Psychological Wellbeing and Academic Experience of University Students in Australia during COVID-19. Int J Environ Res Public Health 2021;18.
Fenesi B, Lucibello K, Kim JA, Heisz JJ. Sweat so you don’t forget: exercise breaks during a university lecture increase on-task attention and learning. J Appl Res Mem Cogn. 2018;7(2):261–9.
Ruhland S, Lange KW. Effect of classroom-based physical activity interventions on attention and on-task behavior in schoolchildren: A systematic review. Sports Med Health Sci. 2021;3:125–33.
Aristovnik A, Keržič D, Ravšelj D, Tomaževič N, Umek L. Impacts of the COVID-19 Pandemic on Life of Higher Education Students: A Global Perspective. Sustainability. 2020;12(20).
Browning MHEM, Larson LR, Sharaievska I, Rigolon A, McAnirlin O, Mullenbach L, et al. Psychological impacts from COVID-19 among university students: Risk factors across seven states in the United States. PLoS ONE 2021;16(1).
Chang Y-K, Labban JD, Gapin JI, Etnier JL. The effects of acute exercise on cognitive performance: a meta-analysis. Brain Res. 2012;1453:87–101.
Elmer T, Mepham K, Stadtfeld C. Students under lockdown: Comparisons of students’ social networks and mental health before and during the COVID-19 crisis in Switzerland. PLoS ONE. 2020;15(7):e0236337.
Keating R, Ahern S, Bisgood L, Mernagh K, Nicolson GH, Barrett EM. Stand up, stand out. Feasibility of an active break targeting prolonged sitting in university students. J Am Coll Health. 2020;70(7).
Download references
Acknowledgements
We would like to thank Juliane Moll, research associate of the Student Health Management of University of Tübingen, for the support in the coordination and realization study. We would like to express our thanks also to Ingrid Arzberger, Head of University Sports at the University of Tübingen, for providing the resources and co-applying for the funding. We acknowledge support by Open Access Publishing Fund of University of Tübingen.
Open Access funding enabled and organized by Projekt DEAL. This research regarding the conduction of the study was funded by the Techniker Krankenkasse, health insurance fund.
Author information
Authors and affiliations.
Institute of Sports Science, Faculty of Economics and Social Sciences, University of Tübingen, Tübingen, Germany
Monika Teuber, Daniel Leyhr & Gorden Sudeck
Methods Center, Faculty of Economics and Social Sciences, University of Tübingen, Tübingen, Germany
Daniel Leyhr
Interfaculty Research Institute for Sports and Physical Activity, University of Tübingen, Tübingen, Germany
Gorden Sudeck
You can also search for this author in PubMed Google Scholar
Contributions
M.T. and G.S. designed the study. M.T. coordinated and carried out participant recruitment and data collection. M.T. analyzed the data and M.T. and D.L. interpreted the data. M.T. drafted the initial version of the manuscript and prepared the figure and all tables. All authors contributed to reviewing and editing the manuscript and have read and agreed to the final version of the manuscript.
Corresponding author
Correspondence to Monika Teuber .
Ethics declarations
Ethics approval and consent to participate.
The study involves human participants and was reviewed and approved by the Ethics Committee of the Faculty of Social Sciences and Economics, University of Tübingen (ref. A2.54-127_kr). The participants provided their written informed consent to participate in this study. All methods were carried out in accordance with relevant guidelines and regulations.
Consent for publication
Not applicable.
Competing interests
The authors declare no competing interests.
Additional information
Publisher’s note.
Springer Nature remains neutral with regard to jurisdictional claims in published maps and institutional affiliations.
Supplementary Information
Supplementary file 1., supplementary file 2., rights and permissions.
Open Access This article is licensed under a Creative Commons Attribution 4.0 International License, which permits use, sharing, adaptation, distribution and reproduction in any medium or format, as long as you give appropriate credit to the original author(s) and the source, provide a link to the Creative Commons licence, and indicate if changes were made. The images or other third party material in this article are included in the article's Creative Commons licence, unless indicated otherwise in a credit line to the material. If material is not included in the article's Creative Commons licence and your intended use is not permitted by statutory regulation or exceeds the permitted use, you will need to obtain permission directly from the copyright holder. To view a copy of this licence, visit http://creativecommons.org/licenses/by/4.0/ . The Creative Commons Public Domain Dedication waiver ( http://creativecommons.org/publicdomain/zero/1.0/ ) applies to the data made available in this article, unless otherwise stated in a credit line to the data.
Reprints and permissions
About this article
Cite this article.
Teuber, M., Leyhr, D. & Sudeck, G. Physical activity improves stress load, recovery, and academic performance-related parameters among university students: a longitudinal study on daily level. BMC Public Health 24 , 598 (2024). https://doi.org/10.1186/s12889-024-18082-z
Download citation
Received : 30 June 2023
Accepted : 12 February 2024
Published : 24 February 2024
DOI : https://doi.org/10.1186/s12889-024-18082-z
Share this article
Anyone you share the following link with will be able to read this content:
Sorry, a shareable link is not currently available for this article.
Provided by the Springer Nature SharedIt content-sharing initiative
- Physical activity breaks
- Stress load
- Psychological detachment
- Academic performance
- Study ability
- University students
BMC Public Health
ISSN: 1471-2458
- Submission enquiries: [email protected]
- General enquiries: [email protected]
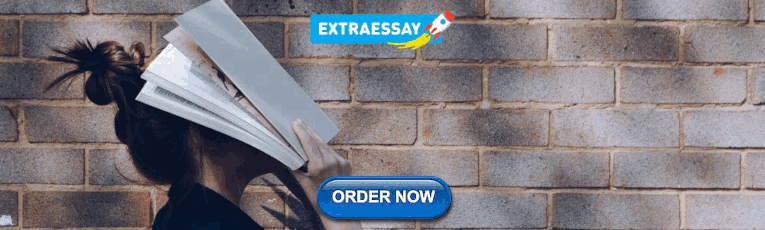
IMAGES
VIDEO
COMMENTS
10. Longitudinal studies always offer a factor of unpredictability. Because the structure of longitudinal studies will follow the same individuals over an extended time period, what happens to each person outside of the scope of the research can have a direct impact on the eventual findings that researchers develop.
Advantages and disadvantages of longitudinal studies. Like any other research design, longitudinal studies have their tradeoffs: they provide a unique set of benefits, but also come with some downsides. Advantages; Longitudinal studies allow researchers to follow their subjects in real time. This means you can better establish the real sequence ...
Disadvantages. Numerous challenges are implicit in the study design; particularly by virtue of this occurring over protracted time periods. ... Embarking on a longitudinal study. Conducting longitudinal research is demanding in that it requires an appropriate infrastructure that is sufficiently robust to withstand the test of time, for the ...
The advantages and disadvantages of longitudinal studies show us that there is a tremendous value available in the ability to find long-term patterns and relationships. If the unpredictable factors of this research format can be planned for in advance and steps taken to remove bias, the data collected offers the potential to dramatically change ...
Disadvantages; Longitudinal studies are time-consuming and often more expensive than other types of studies, so they require significant commitment and resources to be effective. Since longitudinal studies repeatedly observe subjects over a period of time, any potential insights from the study can take a while to be discovered.
Attrition refers to participants leaving a study. It always happens to some extent—for example, in randomized controlled trials for medical research. Differential attrition occurs when attrition or dropout rates differ systematically between the intervention and the control group.As a result, the characteristics of the participants who drop out differ from the characteristics of those who ...
The key is to align the duration with the research goals. Implementing a Longitudinal Study: Your Options. When planning a longitudinal study, you face a crucial decision: gather new data or use existing datasets. Option 1: Utilizing Existing Data. ... Disadvantages of a Longitudinal Study. A longitudinal study can be time-consuming and ...
Despite its disadvantages, longitudinal research has the power to help us understand variation across human development and the lifespan. One of the longest-running studies—following people over 80 years as opposed to comparing different groups at different ages—provides a robust measure of human growth—even revealing factors, like close ...
CRD and LRD. Based on the number of time periods for which the same variable is measured, the research designs in social sciences are broadly classified into two types: CRD and LRD. In CRD, the researcher collects the data on one or more than one variable for a single time period for each case in the study. The researcher measures the variables ...
The researchers should be aware of the advantages and disadvantages of the research design selected and have adequate access to potential participants. ... time was a critical factor in conducting the qualitative longitudinal study. Future research employing a comparative qualitative longitudinal approach should allocate ample time to complete ...
Longitudinal studies, a type of correlational research, are usually observational, in contrast with cross-sectional research. Longitudinal research involves collecting data over an extended time, whereas cross-sectional research involves collecting data at a single point. To test this hypothesis, the researchers recruit participants who are in ...
Weaknesses of longitudinal data. Attrition. Over time, participants may cease to take part in a longitudinal study. This is known as attrition. Attrition can result from a range of factors, some of which are unavoidable, while others can be reduced by careful study design or practice. Unavoidable attrition might be caused by a participant dying ...
Panel Study. A panel study is a type of longitudinal study design in which the same set of participants are measured repeatedly over time. Data is gathered on the same variables of interest at each time point using consistent methods. This allows studying continuity and changes within individuals over time on the key measured constructs.
A longitudinal study is one form of research that observes and analyzes changes in a phenomenon over time. It is a time-consuming form of inquiry, yet it can yield highly impactful findings. Learn about the purpose of and considerations for longitudinal studies in this article. Roehl Sybing.
A longitudinal study (or longitudinal survey, or panel study) is a research design that involves repeated observations of the same variables (e.g., people) over long periods of time (i.e., uses longitudinal data).It is often a type of observational study, although it can also be structured as longitudinal randomized experiment.. Longitudinal studies are often used in social-personality and ...
A longitudinal study is a research conducted over an extended period of time. It is mostly used in medical research and other areas like psychology or sociology. When using this method, a longitudinal survey can pay off with actionable insights when you have the time to engage in a long-term research project.
Learn what a longitudinal study is and check out the different types of longitudinal studies. Also, learn the advantages and disadvantages of longitudinal research designs. Updated: 11/21/2023
1. They require huge amounts of time. Time is definitely a huge disadvantage to any longitudinal study, as it typically takes a substantial amount of time to collect all the data that is required. Also, it takes equally long periods to gather results before the patterns can even start to be made. 2.
Disadvantages of Longitudinal Research Designs The main challenge of using a longitudinal design is the cost in time and resources. These studies are much more expensive and take much longer to conduct than a cross-sectional study with the same number of participants. A second issue is the impact of repeated testing.
Longitudinal Studies are studies in which data is collected at specific intervals over a long period of time in order to measure changes over time. This post provides one example of a longitudinal study and explores some the strengths and limitations of this research method. With a longitudinal study you might start with an original.
Introduction. Longitudinal qualitative research (LQR) is an emerging methodology in health behavior and nursing research—fields focused on generating evidence to support nursing practices as well as programs, and policies promoting healthy behaviors (Glanz et al., 2008; Polit & Beck, 2017).Because human experiences are rarely comprised of concrete, time-limited events, but evolve and change ...
Unlike other research methods, longitudinal studies collect unique, long-term data and highlight relationships that cannot be discovered in a short-term investigation. You can collect additional data to study unexpected findings at any point in your systematic investigation. Disadvantages and Limitations of a Longitudinal Study
Top Advantages of Longitudinal Studies. 1. Longitudinal studies make it easier to find long-term patterns. Many research studies focus on short-term data alone. That means long-term data may offer patterns or information that cannot be collected. A longitudinal study would be able to collect that long-term data and locate patterns within it ...
Design and procedures. To examine these hypothesized associations, a longitudinal study design with daily surveys was chosen following the suggestion of the day-level study of Feuerhahn et al. (2014) and also of Sonnentag (2001) measuring recovery potential of (exercise) activities during leisure time [50, 51].Considering that there are also differences between people at the beginning of the ...
In this study, on the basis of introducing the conventional side-span joint-construction technology, the one-time pouring joint-construction technology was adjusted. ... According to the relevant research, the longitudinal displacement of the cantilever end of the T-structure under the action of insolation temperature can be divided into two ...