Google Scholar Metrics
Google Scholar Metrics provide an easy way for authors to quickly gauge the visibility and influence of recent articles in scholarly publications. Scholar Metrics summarize recent citations to many publications, to help authors as they consider where to publish their new research.
To get started, you can browse the top 100 publications in several languages , ordered by their five-year h-index and h-median metrics. To see which articles in a publication were cited the most and who cited them, click on its h-index number to view the articles as well as the citations underlying the metrics.
You can also explore publications in research areas of your interest. To browse publications in a broad area of research, select one of the areas in the left column. For example: Engineering & Computer Science or Health & Medical Sciences .
To explore specific research areas, select one of the broad areas, click on the "Subcategories" link and then select one of the options. For example: Databases & Information Systems or Development Economics.
Browsing by research area is, as yet, available only for English publications. You can, of course, search for specific publications in all languages by words in their titles.
Scholar Metrics are currently based on our index as it was in July 2023 .
Available Metrics
The h-index of a publication is the largest number h such that at least h articles in that publication were cited at least h times each. For example, a publication with five articles cited by, respectively, 17, 9, 6, 3, and 2, has the h-index of 3.
The h-core of a publication is a set of top cited h articles from the publication. These are the articles that the h-index is based on. For example, the publication above has the h-core with three articles, those cited by 17, 9, and 6.
The h-median of a publication is the median of the citation counts in its h-core. For example, the h-median of the publication above is 9. The h-median is a measure of the distribution of citations to the articles in the h-core.
Finally, the h5-index , h5-core , and h5-median of a publication are, respectively, the h-index, h-core, and h-median of only those of its articles that were published in the last five complete calendar years.
We display the h5-index and the h5-median for each included publication. We also display an entire h5-core of its articles, along with their citation counts, so that you can see which articles contribute to the h5-index. And there's more! Click on the citation count for any article in the h5-core to see who cited it.
Coverage of Publications
Scholar Metrics currently cover articles published between 2018 and 2022 , both inclusive. The metrics are based on citations from all articles that were indexed in Google Scholar in July 2023 . This also includes citations from articles that are not themselves covered by Scholar Metrics.
Since Google Scholar indexes articles from a large number of websites, we can't always tell in which journal a particular article has been published. To avoid misidentification of publications, we have included only the following items:
- journal articles from websites that follow our inclusion guidelines ;
- selected conference articles in Engineering and Computer Science.
Furthermore, we have specifically excluded the following items:
- court opinions, patents, books, and dissertations;
- publications with fewer than 100 articles published between 2018 and 2022;
- publications that received no citations to articles published between 2018 and 2022.
Overall, Scholar Metrics cover a substantial fraction of scholarly articles published in the last five years. However, they don't currently cover a large number of articles from smaller publications.
Inclusion and Corrections
If you can't find the journal you're looking for, try searching by its abbreviated title or alternate title. There're sometimes several ways to refer to the same publication. (Fun fact: we've seen 959 ways to refer to PNAS.)
If you're wondering why your journal is not included, or why it has fewer citations than it surely deserves, that is often a matter of configuring your website for indexing in Google Scholar. Please refer to the inclusion manual . Also, keep in mind that Scholar Metrics only include publications with at least a hundred articles in the last five years.
- Privacy & Terms
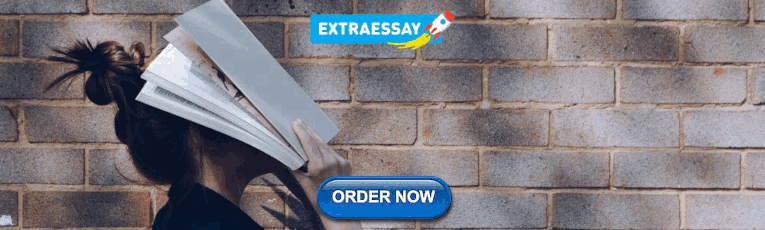
ScienceDirect Support Center
To post social content, you must have a display name. The page will refresh upon submission. Any pending input will be lost.
Where do I find the Impact Factor of a journal?
The Impact Factor is a measure of scientific influence of scholarly journals. It measures the average number of citations received in a particular year by papers published in the journal during the two preceding years and is produced by a publisher called Thomson Reuters. The Impact Factor can be found on the Journal home page of journals that have an Impact Factor.
Please note: Not all journals have an Impact Factor.
Follow these steps to find the Impact Factor of a journal:
- Search for a journal using the ‘Journal/book title’ field on the ScienceDirect homepage or browse journal titles by selecting ' Journals & Books ' in the top right corner.
- Click the journal title to navigate to the journal’s home page.
- The Impact Factor and Journal CiteScore are mentioned in the header on the right side of the page.
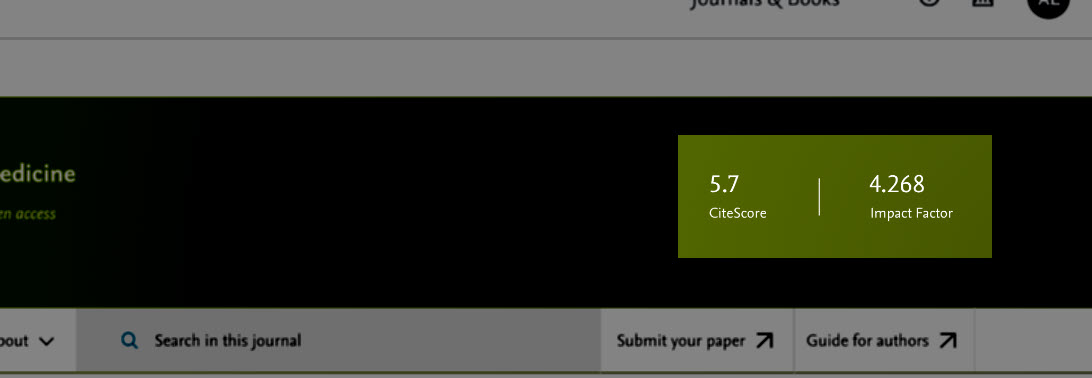
Was this answer helpful?
Thank you for your feedback, it will help us serve you better. If you require assistance, please scroll down and use one of the contact options to get in touch.
Help us to help you:
Thank you for your feedback!
- Why was this answer not helpful?
- It was hard to understand / follow.
- It did not answer my question.
- The solution did not work.
- There was a mistake in the answer.
- Feel free to leave any comments below: Please enter your feedback to submit this form
Related Articles:
- What are Article Metrics?
- Where can I find the DOI?
- What can I do on a journal home page?
- Discover content from other publishers on ScienceDirect
- What are the technical requirements for ScienceDirect?
For further assistance:
- Interlibrary Loan
Ask an Expert
Ask an expert about access to resources, publishing, grants, and more.
MD Anderson faculty and staff can also request a one-on-one consultation with a librarian or scientific editor.
- Library Calendar
Log in to the Library's remote access system using your MyID account.
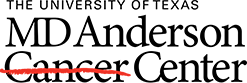
- UT MD Anderson Cancer Center
- Ask the Research Medical Library
Q. What are impact factors and how do I find one?
- Editing Services
- 4 About RML
- 3 altmetrics
- 1 BioRender
- 11 cited references
- 8 collections
- 5 Copyright
- 4 data management
- 15 databases
- 1 Editing Services
- 2 full text
- 4 impact factors
- 4 Interlibrary Loan
- 11 journals
- 7 NIH Public Access Policy
- 4 open access
- 2 Other Libraries
- 2 peer review
- 1 plagiarism
- 18 publishing
- 30 reference
- 13 services
- 13 Systematic Reviews
Answered By: Clara Fowler Last Updated: May 08, 2024 Views: 87683
Impact factors are used to measure the importance of a journal by calculating the number of times selected articles are cited within the last few years. The higher the impact factor, the more highly ranked the journal. It is one tool you can use to compare journals in a subject category.
Impact Factors for scientific journals can be found in the Journal Citation Reports (JCR) database , which is available from the Research Medical Library. You can view all journals at once, search for a specific journal title or choose a group of journals by subject area. Sort journal lists by impact factor by selecting Journal Impact Factor above your search results.
Please note that Journal Impact Factors are released annually around the month of July. The 2023 release of Journal Citation Reports extends the Journal Impact Factor to all 21,522 Web of Science Core Collection™ journals, including those indexed in the Arts and Humanities Citation Index™ (AHCI) and the multidisciplinary Emerging Sources Citation Index™ (ESCI) for the first time. Those journals meet rigorous selection criteria for Web of Science Core Collection inclusion. See JCR 2023 Statistics.
Links & Files
- Getting Started with Journal Citation Reports
- What is considered a good impact factor?
- MD Anderson Library Databases - including JCR (Journal Citation Reports)
- Share on Facebook
Was this helpful? Yes 24 No 24
Comments (0)
Chat or Zoom Live with Staff
Text Us: (281) 369-4872
Call Us: +1 (713) 792-2282
View Research Guides
Request an Online Consultation
Related Topics
- impact factors

Measuring Your Impact: Journal Impact Factor
- What is ORCID
- Citation Analysis
- Journal Impact Factor
- Find Your H-Index
About Journal Impact Factor
The journal impact factor (JIF) is a measure of the frequency with which the average article in a journal has been cited in a particular year. It is used to measure the importance or rank of a journal by calculating the times its articles are cited. Clarivate Analytics is the creator and only provide of the journal impact factor. Other publishers and companies have similar sounding "impact factor" names or terms.
The normalized impact factor (NIF) was introduced by Clarivate Analytics as a method to enable better comparisons between disciplines in each field. It targets 54 disciplines in the biomedical field to assess validity and practical usage.
Find the Journal Impact Factor
Use Journal Citation Reports (JCR) to find the impact factor of a journal.
You can enter a journal title in the Search box under "Go to Journal Profile". Because impact factors mean little on their own, it's best to view the journal you are interested in comparison to the other journals in the same category. To determine the impact factor for a particular journal, select a JCR edition (Science and/ or Social Science), year, and Categories, found on the left of the screen. Click Submit . Scroll the list to find the journal you are interested in.
Contact the library if you have questions for trouble finding a journal in JCR.
Impact Factor Video
More Information
If you have more questions about metrics and assessment contact:
Charlotte Bhasin or Mario Scarcipino
If you have questions about databases or where to publish contact:
Michelle Kraft or Matt Weaver
Where To Publish
The library recommends using these aids to determine the best place to publish your research.
- Contact Matt Weaver for journals with impact factors by article topic
- Find journals indexed in Medline
- Be iNFORMed check list to asses journal & publisher quality
- Beall's List of Predatory Journals & Publishers to avoid potential problem journals
Other Journal Metrics
Eigenfactor.
Eignenfactor scores can be found in the above listed Journal Citation Reports or at eigenfactor.org. Journal Citation Reports or at eigenfactor.org. Eigenfactor scores are intended to give a measure of how likely a journal is to be used, and are thought to reflect how frequently an average researcher would access content from that journal. (Wikipedia) ( Find out more about the Eigenfactor )
SCImago Journal Rank (SJR) (by Elsevier)
“The SCImago Journal & Country Rank is a portal that includes the journals and country scientific indicators developed from the information contained in the Scopus® database (Elsevier B.V.).” Scopus contains more than 15,000 journals from over 4,000 international publishers as well as over 1000 open access journals. SCImago's "evaluation of scholarly journals is to assign weights to bibliographic citations based on the importance of the journals that issued them, so that citations issued by more important journals will be more valuable than those issued by less important ones." ( SJR indicator )
- << Previous: Citation Analysis
- Next: Find Your H-Index >>
- Last Updated: Oct 11, 2022 11:45 AM
- URL: https://my.clevelandclinic.libguides.com/impact
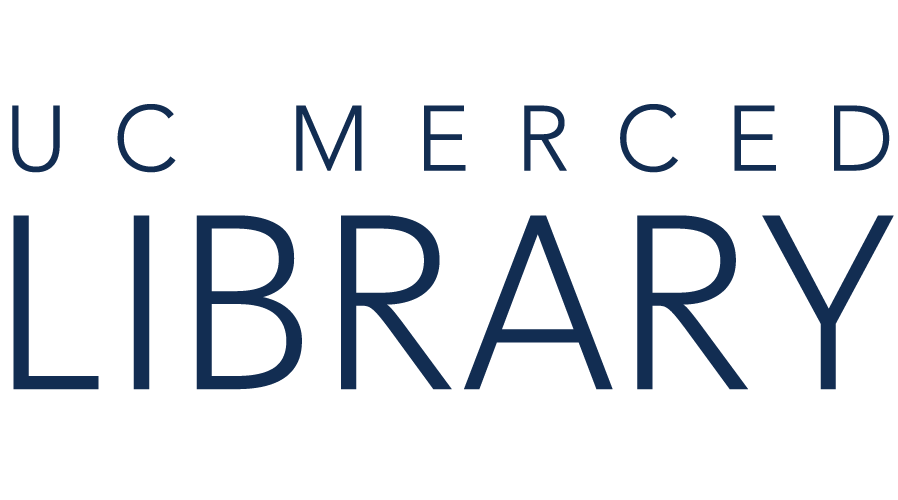
Impact factors: What they are, where to find them, how to use them
- Introduction to impact factors
- Which UC Merced databases include impact factors?
- Video tutorials on impact factors
- Need additional help?
Instruction & Outreach Librarian

More on Citation Metrics
Wikipedia has an excellent collection of articles on various types and aspects of citation metrics, including impact factors, H-indexing, citation analysis and more. Click the image below if you're interested in learning more.

This guide was created in support of the Fall 2022 UC Merced Library workshop, "Impact factors: What they are, where to find them, and how to use them." Here, we'll discuss impact factors with emphasis on their use in writing theses and dissertations for degree credit at UC Merced.
What is an impact factor?
The impact factor measures the number of times a journal article has been cited by researchers in a given year. It's used to measure the importance of a scholarly journal -- that is, its importance to the discipline or field its articles cover, and by extension, the researchers working in that discipline or field -- by measuring the number of times articles in that journal are cited.
- Generally, impact factors are the best way to determine a journal's relative importance in a particular field or discipline. Your own research will be more readily accepted if it's based on the top journals -- meaning, the journals with the highest impact factors -- in your field.
- Impact factors are not perfect, and can be gamed, so to speak. Many journals will attempt to increase their impact factors by requiring that authors whose work is accepted for publication include citations to articles published in those journals.
Creating the impact factor

- Journal impact factors are calculated on the total number of citable articles in the two most recent, previous years. So it's not possible to get a journal impact factor for the present year. In 2022, the most recent journal impact factors will have been calculated on 2020 and 2021.
- Because journal impact factors are calculated on two years of article citations, it's not possible to calculate an impact factor for new journals.
- An impact factor of 10 is an excellent impact factor and indicates that the journal is of major importance in a field or discipline.
- An impact factor of 3 is considered to be good.
- Average impact factors for most journals are less than 1. However, this doesn't indicate that a journal is of poor quality. It may be a journal that publishes research in a field that is not noted for research.
- Next: Which UC Merced databases include impact factors? >>
- Last Updated: Oct 20, 2022 10:28 AM
- URL: https://libguides.ucmerced.edu/impact-factors
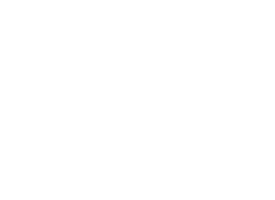
- USC Libraries
Q. How do I find the impact factor of a journal?
- Access Services
- LibAnswers General FAQs
- Special Collections
- 24 About the Libraries
- 22 Access Services
- 12 Business
- 1 ILL, Document Delivery & Course Reserves
- 2 Reproductions, Licensing, and Fees
- 107 Searching & Research Help
- 1 Searching and Research Help
- 82 Subjects & Collections
- 31 Technology & Computing
- 6 USC Digital Library
Answered By: Elizabeth Galoozis (she/her) Last Updated: Jul 14, 2022 Views: 45616
You can look up the impact factor of a journal through the Journal Citation Reports (JCR) database. A journal's impact factor is a measure of how often the average article in a journal has been cited in a particular year. The impact factor will help you evaluate a journal's relative importance, especially when you compare it to others in the same field. Not every journal will have an impact factor, but there are other ways to measure impact to a field.
In Journal Citation Reports, enter the title of the journal or browse journals by rank or category.

[Screenshot of a yellow arrow selecting a "Browse by Journal" search in the Journal Citation Reports database]
Clicking on a journal title will give additional details about the calculation. For example, here is an explanation of how impact factor was calculated for the journal Social Work Research .
[Screenshot of an explanation of a journal impact factor calculation]
Use the "All Years" tab to access key metrics and additional data for the current year and all prior years for this journal.

[Screenshot of yellow arrow highlighting the "All Years" tab when searching]
Since impact factors vary widely by discipline, it is helpful to get a baseline for your discipline by searching for subject area (category). Impact factor data is only available for journals indexed by Web of Science.

[Screenshot of search for journal impact factor by subject area]
For more information on citation counts and Impact factors, visit: Evaluating Information Sources: Impact Factors and Citation Counts
- Share on Facebook
Was this helpful? Yes 7 No 28
Comments (0)
Related topics.
- Searching & Research Help
University Library, University of Illinois at Urbana-Champaign

Introduction to Impact Factor and Other Research Metrics
- Types of Metrics
Impact Factor
- Identifying Journals
- More Resources
More Information
- About Journal Impact Factor Visit this article published by Thomson Reuters on journal impact factor to learn more about the bibliometric and how it measures importance.
- Annual Reviews Rankings in JCR Visit this page to see how Annual Review Journals currently rank in Journal Citation Reports.
- SCImago Journal and Country Rank The SCImago Journal & Country Rank is a publicly available portal that includes the journals and country scientific indicators developed from the information contained in the Scopus database.
Ask a Librarian

Impact factor, or Journal Impact Factor, is a measure of the frequency with which the "average article" published in a given scholarly journal has been cited in a particular year or period and is often used to measure or describe the importance of a particular journal to its field. Impact factor was originally developed by Eugene Garfield, the founder of Institute of Scientific Information, which is now a part of Clarivate Analytics. Journal Impact Factor can be found in the Journal Citation Reports or the JCR, as it's commonly known. Over the years various organizations have been created similar journal-level metrics, such as SCImago Journal & Country Rank .
This page describes how to find impact factor in Journal Citation Reports .
Journal Citation Reports
Clarivate Analytics (formerly Institute for Scientific Information (ISI)) ranks, evaluates, and compares journals within subject categories and publishes the results in Journal Citation Reports . Journal Citation Reports provides ranking for journals in science, technology, and the social sciences. For every journal, the database collects and/or calculates information such as:
- citation/article counts
- impact factor
- immediacy index
- cited half-life
- citing half-life
- source data listing
- citing journal listing
- cited journal listing
- publisher information
- subject categories
Find Journal Citation Reports (JCR)
Follow the instructions below to find the Journal Citation Reports using the Library's resources.
- Begin at the Library homepage .
- Click on the orange tab that says "Find Materials," then scroll down until you see a laptop icon with the words "Databases by Subject and A-Z"; click on the icon.
- Type journal citations reports in the search box on the left side of the screen and then click on the magnifying glass to search that title.
- Your result will say "Journal Citation Reports"; click on it. It might ask you to provide your Net ID and password if you are off campus.
Find the Impact Factor
- Once in the database you either search by journal title (if you know which journal you want to see) or browse by category, which will let you view journals by JIF by discipline.
- On the left side you can choose search criteria, like impact factor range, year, and if the journal is open access.
- It is important to choose the right edition based on your subject area, as you won't be able to see specific journals if you choose the wrong one. Once you have finished selecting what to search, click Submit.
- You can't access impact factors from last year because the calculations only happen every two years (i.e. if the current year is 2021 the farthest you can go back is 2020). Most people choose the most current year they can access.
- Journals limited by the subject area, publisher, or geographic region.
- View all journals in order to browse.
- Search for a specific journal if you already know its title
Once you find a journal, the JCR gives you information about the journal, including the journal's abbreviations, how often it is published each year, the publisher, and the ISSN.
Controversy
Many people have questioned the legitimacy of impact factor. Here are a few reasons why:
- Impact factor focuses purely on the numbers. There is no consideration of qualitative elements that have become important in today's world.
- Impact factor fails to incorporate more recent ways of sharing and using research, including Twitter mentions and posts, citation management downloads, and news and community information.
- Because impact factor is based on citations in only indexed journals , it fails to incorporate statistics from journals that might not be indexed and other sources like conference papers (which are important in the social sciences).
- Basic or summary information is usually cited the most in academia. That means that journals that publish articles with basic information are more likely to have higher impact factors. Journals that publish obscure or innovative information might not have as high of an impact factor.
- Some argue that impact factor is encouraging scholars to stick with mainstream topics and research.
- Scholars don't always have to cite something for it to be influential. Sometimes researchers just read something and it influences them, regardless of if they cite it in a future paper or piece of research.
- The journals in the JCR are mostly published in English. This means that many international sources aren't included in the conversation.
- It has been argued that journals have the ability to skew impact factor for their own journal. Before publishing an author, they will ask the author to cite more articles within their journal so that their impact factor goes up. This is NOT a common occurrence but instead something we should be aware of.
- << Previous: Types of Metrics
- Next: Identifying Journals >>
- Last Updated: Feb 28, 2024 12:49 PM
- URL: https://guides.library.illinois.edu/impact
University of Illinois Chicago
University library, search uic library collections.
Find items in UIC Library collections, including books, articles, databases and more.
Advanced Search
Search UIC Library Website
Find items on the UIC Library website, including research guides, help articles, events and website pages.
- Search Collections
- Search Website
Measuring Your Impact: Impact Factor, Citation Analysis, and other Metrics: Journal Impact Factor (IF)
- Measuring Your Impact
- Citation Analysis
- Find Your H-Index
- Other Metrics/ Altmetrics
- Journal Impact Factor (IF)
- Selecting Publication Venues
About Journal Impact
Impact Factor - What is it?; Why use it?
The impact factor (IF) is a measure of the frequency with which the average article in a journal has been cited in a particular year. It is used to measure the importance or rank of a journal by calculating the times its articles are cited.
How Impact Factor is Calculated?
The calculation is based on a two-year period and involves dividing the number of times articles were cited by the number of articles that are citable.
Calculation of 2010 IF of a journal:
Reliability of the Impact Factor
- Seglen, P. O. (1997). Why the impact factor of journals should not be used for evaluating research . British Medical Journal, 314(7079), 498-502.
- Johnstone, M. J. (2007). Journal impact factors: Implications for the nursing profession . International Nursing Review 54(1), 35-40.
- Ironside, P. M. (2007). Advancing the science of nursing education: Rethinking the meaning and significance of journal impact factors . Journal of Nursing Education, 46(3), 99-100.
- Satyanarayana, K. & Sharma, A. (2008). Impact factor: Time to move on . The Indian Journal of Medical Research, 127(1), 4-6.
- Greenwood, D. C. (2007). Reliability of journal impact factor rankings . BMC Medical Research Methodology, 7(48), 48.
- Howard, J. (2009). Humanities journals confront identity crisis. The Chronicle of Higher Education, 55(19), A1.
Tools to Measure Journal Impact (Impact Factor)
Journal Citation Reports ( Learn more )
SJR, CiteScore, SNIP through Scopus ( learn more )
SCImago Journal Rank (SJR) ( Learn more )
SNIP (Learn more)
Journal Citation Reports
Journal Citation Reports provides ranking for journals in the areas of science, technology, and social sciences. For every journal covered, the following information is collected or calculated: Citation and article counts, Impact factor, Immediacy index, Cited half-life, citing half-life, Source data listing, Citing journal listing, Cited journal listing, Subject categories, Publisher information.
- Limited to the citation data of Journals indexed in Web of Science
- Process to determine journals included in the tool
- Indexes over 12,000 journals in arts, humanities, sciences, and social sciences
You can enter a journal title in the Search box under "Go to Journal Profile". Because impact factors mean little on their own, it's best to view the journal you are interested in comparison to the other journals in the same category. To determine the impact factor for a particular journal, select a JCR edition (Science and/ or Social Science), year, and Categories, found on the left of the screen. Click Submit . Scroll the list to find the journal you are interested in. The list can be resorted by Journal time, Cites, Impact Factor, and Eigenfactor.
Scopus (Elsevier)
Scopus provides three journal metrics - CiteScore, SJR (SCImago Journal Rank) and SNIP (Source Normalized Impact per Paper). Once you are in Scopus, click on "Sources" at the top to access the journal impact data. See below for more on SJR and SNIP
Over 22,000 active journals from over 4,000 international publishers
- Process to determine journals included in the tools
SCImago Journal Rank (SJR)
“The SCImago Journal & Country Rank is a portal that includes the journals and country scientific indicators developed from the information contained in the Scopus® database (Elsevier B.V.).” Scopus contains more than 15,000 journals from over 4,000 international publishers as well as over 1000 open access journals. SCImago's "evaluation of scholarly journals is to assign weights to bibliographic citations based on the importance of the journals that issued them, so that citations issued by more important journals will be more valuable than those issued by less important ones." ( SJR indicator )
SNIP (Source Normalized Impact per Paper)
Source Normalized Impact per Paper (SNIP) measures contextual citation impact by weighting citations based on the total number of citations in a subject field. The impact of a single citation is given higher value in subject areas where citations are less likely, and vice versa. Unlike the well-known journal impact factor, SNIP corrects for differences in citation practices between scientific fields, thereby allowing for more accurate between-field comparisons of citation impact. CWTS Journal Indicators also provides stability intervals that indicate the reliability of the SNIP value of a journal. SNIP was created by Professor Henk F. Moed at Centre for Science and Technology Studies (CWTS), University of L
CWTS Journal Indicators currently provides four indicators:
- P. The number of publications of a source in the past three years.
- IPP. The impact per publication, calculated as the number of citations given in the present year to publications in the past three years divided by the total number of publications in the past three years. IPP is fairly similar to the well-known journal impact factor. Like the journal impact factor, IPP does not correct for differences in citation practices between scientific fields. IPP was previously known as RIP (raw impact per publication).
- SNIP. The source normalized impact per publication, calculated as the number of citations given in the present year to publications in the past three years divided by the total number of publications in the past three years. The difference with IPP is that in the case of SNIP citations are normalized in order to correct for differences in citation practices between scientific fields. Essentially, the longer the reference list of a citing publication, the lower the value of a citation originating from that publication. A detailed explanation is offered in our scientific paper.
- % self cit. The percentage of self citations of a source, calculated as the percentage of all citations given in the present year to publications in the past three years that originate from the source itself.
See more at: https://www.journalindicators.com/methodology
- << Previous: Other Metrics/ Altmetrics
- Next: Selecting Publication Venues >>
- Last Updated: Mar 13, 2024 12:51 PM
- URL: https://researchguides.uic.edu/if
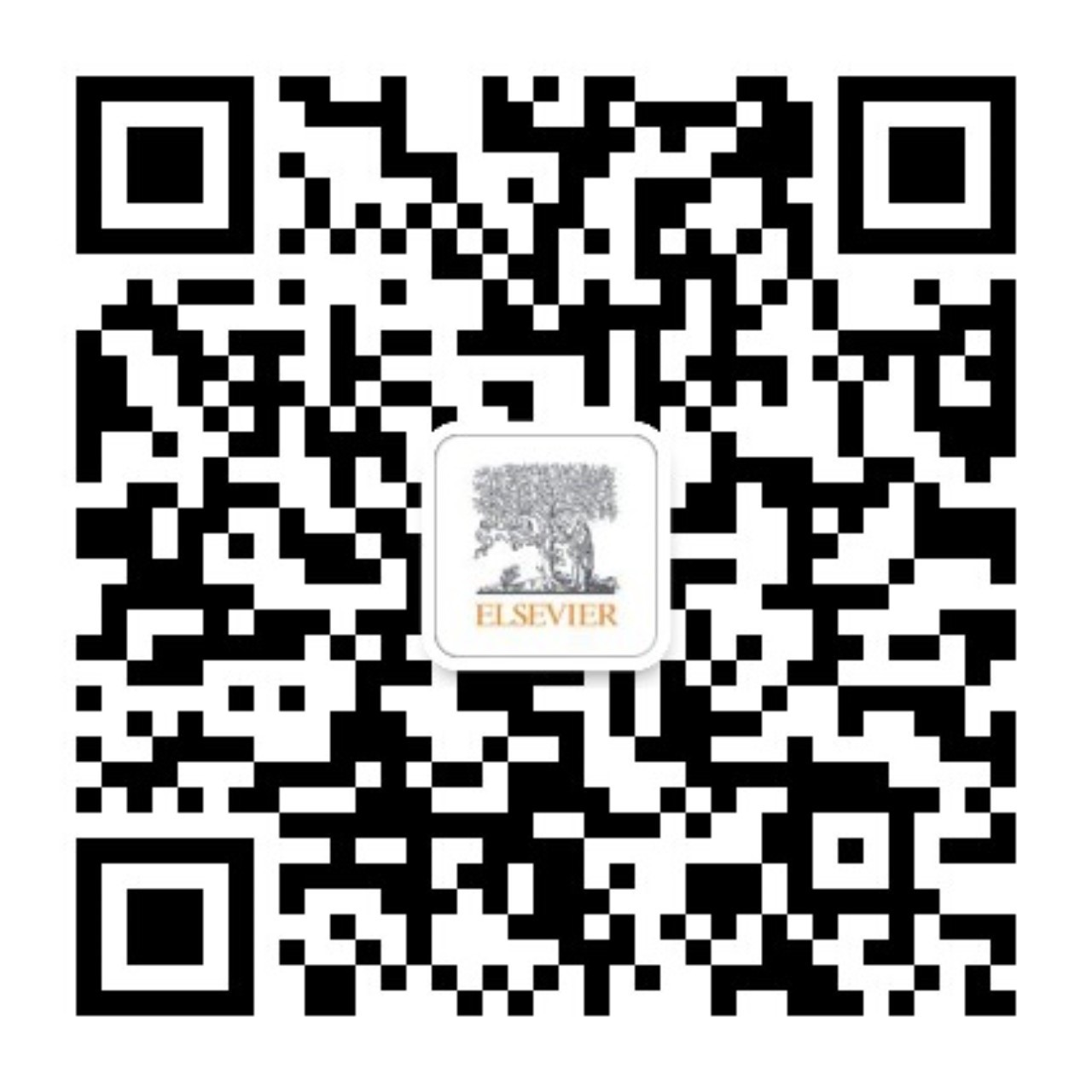
- Research Process
What is Journal Impact Factor?
- 3 minute read
- 240.6K views
Table of Contents
Daunted by the idea of choosing the right journal for your paper? Don’t be. Metrics have become an everyday word in scholarship, in general. Within its many fields of research – if not all of them – they provide important data about a journal’s impact and relevance among its readers. In an era of information proliferation, it has become increasingly important to know where to capture the most attention and interest of your target audience.
So, whenever you are in doubt about which journal suits you better, don’t forget to browse its metrics; they will certainly help you with the decision-making process. Start, for example, with the Journal Impact Factor.
Impact factor (IF) is a measure of the number of times an average paper in a journal is cited, during a year. Clarivate Analytics releases the Journal Impact Factors annually as part of the Web of Science Journal Citation Reports®. Only journals listed in the Science Citation Index Expanded® (SCIE) and Social Sciences Citation Index® (SSCI) receive an Impact Factor.
What is a good impact factor for a scientific journal?
Impact Factors are used to measure the importance of a journal by calculating the number of times selected articles are cited within a particular year. Hence, the higher the number of citations or articles coming from a particular journal, or impact factor, the higher it is ranked. IF is also a powerful tool if you want to compare journals in the subject category.
Measuring a Journal Impact Factor:
- CiteScore metrics – helps to measure journal citation impact. Free, comprehensive, transparent and current metrics calculated using data from Scopus®, the largest abstract and citation database of peer-reviewed literature.
- SJR – or SCImago Journal Rank, is based on the concept of a transfer of prestige between journals via their citation links.
- SNIP – or Source Normalized Impact per Paper, is a sophisticated metric that accounts for field-specific differences in citation practices.
- JIF – or Journal Impact Factor is calculated by Clarivate Analytics as the average of the sum of the citations received in a given year to a journal’s previous two years of publications, divided by the sum of “citable” publications in the previous two years.
- H-index – Although originally conceived as an author-level metric, the H -index has been being applied to higher-order aggregations of research publications, including journals.
Deciding the perfect journal for your paper is an important step. Metrics are excellent tools to guide you through the process. However, we also recommend you not neglect a perfectly written text, not only scientific and grammatically but also fitting the chosen journal’s requirements and scope. At Elsevier, we provide text-editing services that aim to amend and adjust your manuscript, to increase its chances of a successful acceptance by your target journal. Although each journal has its own editorial team, the overall quality, language and whether the article is innovative may also play a role.
Language Editing Services by Elsevier Author Services:
We know that, as an academic researcher, you have many things to do to stay relevant.
Writing relevant manuscripts is a crucial part of your endeavors.
That’s why we, at Elsevier Author Service s, support you throughout your publication journey with a suite of products and services to help improve your manuscript before submission.
Check our video Reach the highest standard with Elsevier Author Services to learn more about Author Services.
Find more about What is Journal Impact Factor? on Pinterest:
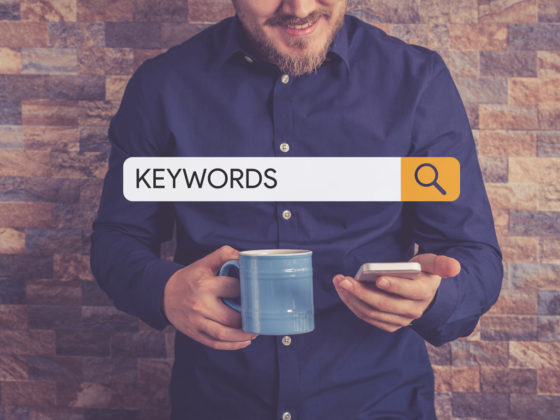
- Manuscript Preparation
How to Choose Keywords for a Manuscript?
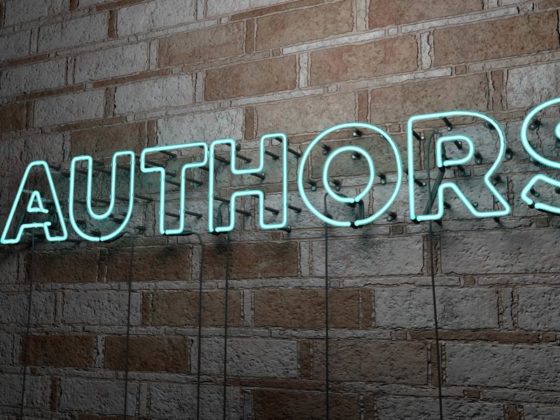
- Publication Recognition
What is a Corresponding Author?
You may also like.
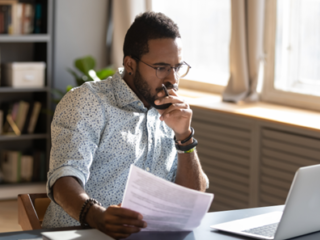
Descriptive Research Design and Its Myriad Uses
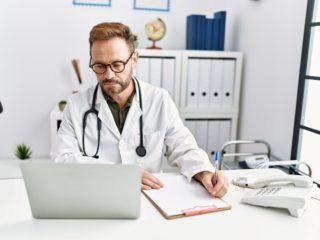
Five Common Mistakes to Avoid When Writing a Biomedical Research Paper
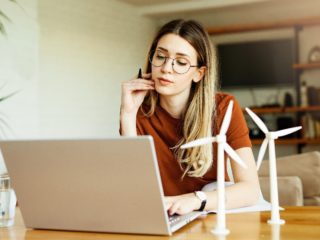
Making Technical Writing in Environmental Engineering Accessible
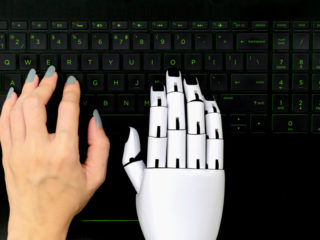
To Err is Not Human: The Dangers of AI-assisted Academic Writing
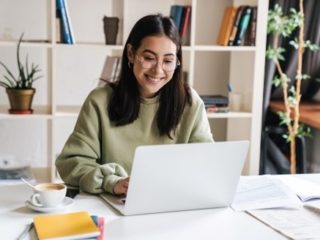
When Data Speak, Listen: Importance of Data Collection and Analysis Methods
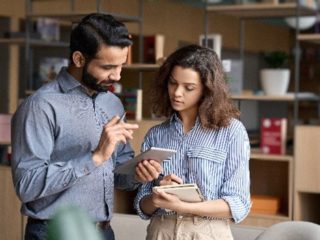
Choosing the Right Research Methodology: A Guide for Researchers
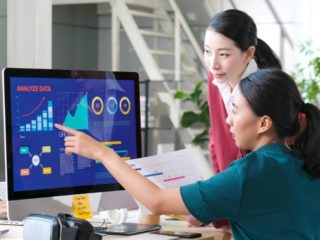
Why is data validation important in research?
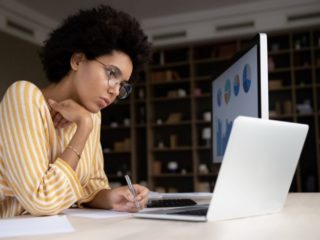
Writing a good review article
Input your search keywords and press Enter.
Research Journal Impact factor: A Complete Guide and Benchmarking
The research journal impact factor is easy to find, but understanding those numbers is quite another matter. Here is a guide for that.
Have you ever had difficulty choosing the most appropriate journal for your paper? The selection of the most relevant journal to target can be overwhelming for any researcher looking to publish an article. It doesn’t have to be.
Research is increasingly grounded on the basis of certain metrics. A journal’s matrices provide important information about its impact and relevance in its many fields of research – if not all of them. As the number of sources of information has increased, finding the most effective ways to reach your target audience is becoming more key.
To be sure that your choice of journal is the best, browse its metrics before selecting one. Metrics will surely help you decide on which journal to use. The Journal Impact Factor , for example, will give you an indication of which journal to submit to.
The number of impact factors a journal has is easy to find, but actually understanding those numbers is quite another matter. The following is a guide that makes journal impact factor simple to understand. Among other things, we will discuss what it is and also list the journals and their impact factors to help your decision.
What is research journal impact factor?
An impact factor (IF) is an indicator of how frequently a particular article in a journal is cited during a given year. Annually, Clarivate Analytics offers Web of Science Journal Citation Reports which include Journal Impact Factors.
Impact Factors are only allocated to journals that are included in the Science Citation Index Expanded (SCIE) and the Social Sciences Citation Index (SSCI).
Research Journal Impact Factor enables users to assess and categorize academic journals for quality and excellence using quantitative and qualitative techniques.
Research journals with high-impact factors tend to be more well-regarded. Eugene Garfield, the founder of the Institute for Scientific Information, first introduced this concept.

Many organizations and clinicians utilize journal impact factors, but they are unaware of the method for calculating them, their importance, and how they are used.
There are a number of journals that publish more reviews or review articles that will result in a higher impact factor. As Eugene Garfield noted, the impact is simply a function of how well journals and editors attract the best papers to their journals.
It then becomes a question of how the impact factor is calculated , and how do you find the right journal with a good impact factor? Let’s take a closer look at these really important factors.
How is a good Research journal’s impact factor determined?
Is there anything you can tell me about a good journal’s impact factor? Is the answer 5 or 99? There is no fixed numerical answer to this question for every journal.
Generally speaking, impact factors are used to determine the relative importance of a journal in its niche and refers to a measure of how frequently an article in a journal has been cited within a specified period of time.
Citations earned by a wide range of scholarly journals are used to calculate impact factors for Clarivate Analytics. Journal Citation Reports (JCR) computes the yearly Journal Impact Factor by aggregating all citations attributed in a single year to articles published by a journal in the two preceding years.
To calculate the 2022 Journal Impact Factor for a journal, all citations to its 2020 and 2021 articles must be counted. After dividing the total by the number of articles cited in the journal in those years, we arrive at the JIF for the current year (2022), which is published in 2023.
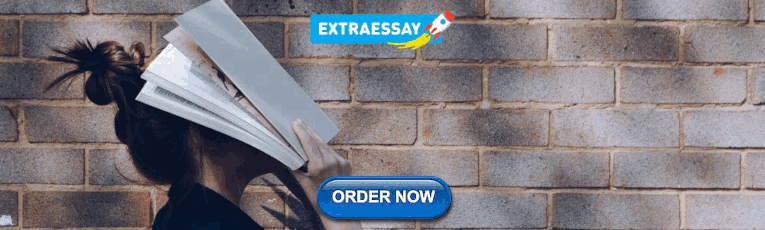
Calculation of 2022 Impact Factor of a journal
A = the frequency of articles published in 2020 and 2021 and were cited by indexed journals during 2022.
B = the total amount of “citable articles” published in 2020 and 2021.
A/B = 2022 impact factor
Therefore, this question arises again, what is a good impact factor for a research journal? There is no simple answer as it varies by journal type and discipline but we can raise some average conclusions.
What is a good impact factor for a research journal
In the sciences, where citations are more rapid and frequent than probably in other fields, a journal with a JIF near 350 will receive an exceptionally high number of citations. In many fields of study, impact factors of 10 or above are considered exceptional, and in some, it is above 3. However, Journal Citation Reports(JCR) impact factors for journals differ significantly from one discipline to another.
The following factors should be considered when evaluating impact factors:
- Date of Publication: Impact factors are calculated by counting the number of citations that an article receives within a short period of publication date. As a result, journals in fast-growing fields like life sciences and technology have higher straight away citation metrics and correspondingly higher Impact Factors. For purposes of calculating the denominator, only research papers or articles and reviews articles that are cited are considered.
- Impact factors are not calculated for articles cited in journals not included in the Web of Science database.
- Review Articles: Review articles, articles of opinion, letter pieces, and news articles are not included in the total number of articles; if cited, they will still count as citations for the journal. In some cases, this could lead to boosted impact factors through alteration of ratios used to calculate impact factors.
- Journals with a low citation count are usually clinical journals. Consequently, such journals suffer from citation points that are lower than those of other journals in their similar subject areas.
- For the first year following the title change of a journal, the Impact Factor for the journal is zero since the number of articles published in the two years prior to the title change is zero. The same applies to new journals added to the database of JCR.
- There is an uneven distribution of coverage since the Journal Citation Reports concentrate on subjects where journal articles are primarily published. As a result, it does not provide much coverage of the Humanities and Social Sciences, which rely more on book formats and other formats of publishing.
An impact factor for a journal in a specific subject category can be determined by comparing it with other journals in the same JCR subject category.
In order to achieve this, you must go to the JCR website on the InCites platform and click Browse by Category. On this page, you’ll find subject classifications ranked by the number of journals in each category.
It is also possible to compare all the journals in a subject category with the Journal Impact Factor, and you can also select particular journals to compare by using the options from the menu. Let’s explore some tools that can be used to calculate journal impact factors.
Journal Impact Factor Measurement Tools
We now know how the calculation is done, so let’s find out where it can be done. The tools listed below are all standard tools and give the most prominent impact factor for journals of various fields.
Journal Citation Reports
Rankings for scientific, technological, and social sciences journals are provided by Journal Citation Reports . Each journal included in the database includes the following information: 1)Citation figures and article numbers, 2) Impact factor, 3) Cited half-life, 4) Immediacy Index, 5) Source record listing, 6) Citing journal listing, 7) Cited journal listing, 8)Research categories, 9)author information.
This database only includes citation data from journals indexed by Web of Science. More than 12,000 journals in arts, literature, sciences and social sciences are indexed.
Using the menu, select a JCR edition (Science or Social Science), year, and Category to determine the impact factor for that journal. Press submit to retrieve the impact factor.
Click on the journal of interest to retrieve the impact factor. There are four ways of classifying the list: by Journal time, by Cites, by Impact Factor, and by Eigenfactor.
Scopus (Elsevier)
Using Scopus Journal Analyzer , you can also evaluate ten journals within one subject area before submitting your manuscript. The Scopus database contains abstracts and citations (bibliography) published by Elsevier.
A Scopus Journal Analyzer does citation analysis for more than 20,500 titles in science, technology, life sciences, and social sciences. These citations date from 1996. Every two months, Scopus updates its database.
As a result of Scopus’s Journal Analyzer, you can determine three metrics about journal performance: CiteScore , SJR ( SCImago Journal Rank ), and SNIP ( Source Normalized Impact per Paper ).
SCImago Journal Rank (SJR)(Elsevier)
The SCImago Journal & Country Rank is an online tool that includes journals and country scientific statistics based on data from the Scopus® database (Elsevier).
More than 10,000 journals are available through Scopus from over 4,000 international publishers, including more than 1,000 open access journals.
This database contains citation details from over 34,100 article titles from more than 5,000 publishers worldwide.
It also includes country-specific impact metrics from 239 countries. You can also integrate significant journal metrics into your page as a clickable image widget through the SJCR.
Eigenfactor
In January 2007, Carl Bergstrom and Jevin West co-founded the Eigenfactor® . University of Washington’s West Lab at the Information School and Bergstrom Lab in the Department of Biology sponsored this project.
A journal’s Eigenfactor score is a measure that is intended to indicate how widely a journal is used. Accordingly, they are supposed to represent how often an ideal researcher would access material from that journal.
Furthermore, the Eigenfactor score changes as a function of journal size, increasing by two-fold with an increase in the number of articles per year published by the journal.
A journal’s Article Influence Score measures the average impact of its articles, which makes it akin to a conventional impact factor.
SNIP (Source Normalized Impact per Paper)
The SNIP project was founded by Professor Henk F. Moed of the Centre for Science and Technology Studies at Leiden University.
In Source Normalized Impact per Paper (SNIP), citation impact is measured by weighting citations according to the volume of citations in a particular subject area. Source Normalized Impact per Paper is calculated annually using Scopus data.
In subject areas with few citations, a single citation holds greater significance, and the reverse is also true. Incorrect citation practices are corrected for by SNIP, making comparisons of citation impact more accurate across fields.
H-index measures a writer’s scientific research output at the level of his or her authorship. This index evaluates the scientific contribution of a scientist while simultaneously evaluating their impact.
This is defined as the largest number for which the author has been cited so many times in so many of his papers. For example, if an author has a h-index of 5, then at least 5 of his papers have been cited at least 5 times.
With a h-index of over 20, an author can be considered an expert in their field. A journal’s h-index may also be calculated. This refers to the maximum number of papers published by the journal that have been cited a number of times in the past. Journals that have been around longer tend to have higher h-index.
The CiteScore of a journal is equal to the number of citations received by it in a particular year to articles published in the previous three years, dividing it by the number of articles indexed by Scopus from the same time period.
The citation impact of a journal is measured with Scopus’ CiteScore. This tool calculates metrics using Scopus data and is free to use. It contains more than 24,000 ranked titles covering more than 300 fields of study.
The CiteScore Tracker provides you with a way of keeping an overview of the performance of particular journals over the course of a year.
The impact factor of major research journals
Impact plays a critical role in understanding how a journal has performed over time and in determining its direction in the coming years.
The true picture of impact can never be achieved by using a single metric, so a range of indicators should be used to support the decision-making process. We’ve listed a few of the major journals with their impact factors below .
Here is how to improve impact factor through Visuals
For a journal to improve its impact factor, its articles must be of a high standard and cited frequently. In order to achieve that, the articles submitted must have elevated factors that make them stand out.
Increasingly, journals are asking for ‘visual abstracts’ along with articles when accepting submissions. The main findings of the article are required to be presented visually and in a comprehensive, concise pictorial summary.
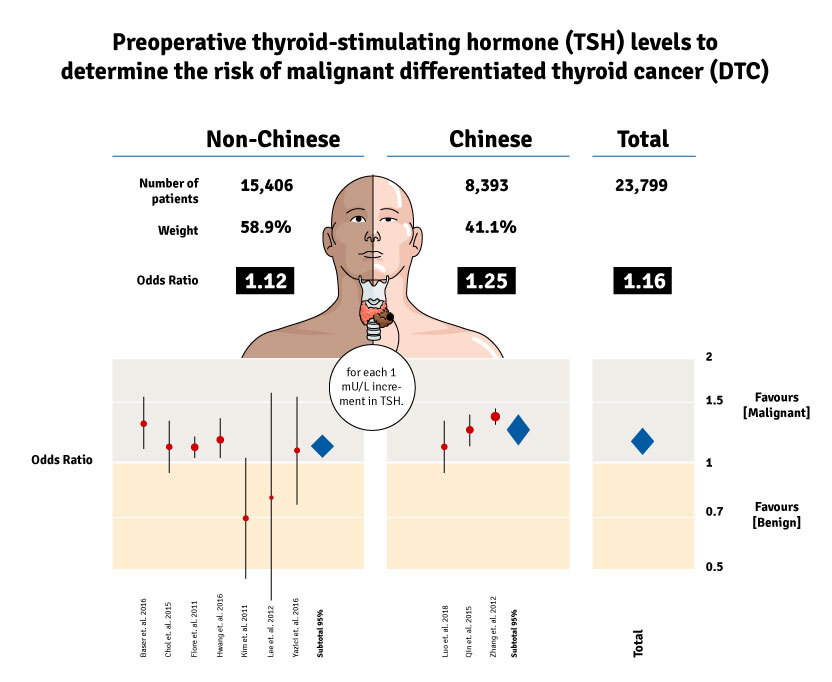
You could choose to use the conclusion of the article or even better yet, a figure that communicates the essence of the article in a quick glance, such as an article-specific figure.
Articles with graphical abstracts have been shown to have a positive effect on both the view count of the article and citations , ultimately improving the impact factor of the journal.
It is significant to note that compared to articles without a visual abstract, it is estimated that the average amount of use per year has doubled for articles with a visual abstract .
In addition to increasing your paper’s citations, you will make it stand out by including an ample number of graphics. It has become increasingly common for researchers to include graphical illustrations in their research.
Using graphic illustrations as a way to communicate your research can be powerful and effective. Read our article on What is Visual Abstract and how to make one in the easiest way .
Nevertheless, you must ensure that illustrations and visual abstracts are not copied and pasted. How and where should you begin? You’ve come to the right place! With Mind the Graph , you can choose from an array of illustrations in multiple fields.
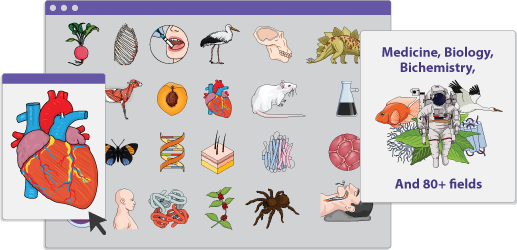
What’s even more fascinating is the fact that you will also be able to get it custom-made by our team of professionals if you so desire. We also post articles relating to science-related topics and other useful guides on our blog. If you’d like to take a look, click here .
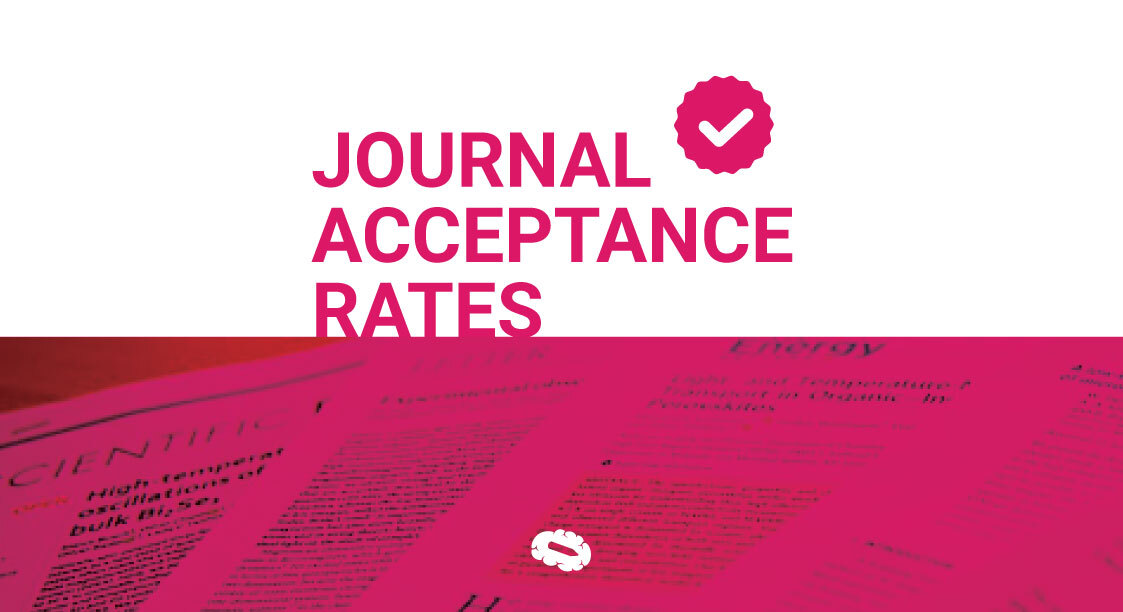
Subscribe to our newsletter
Exclusive high quality content about effective visual communication in science.
Unlock Your Creativity
Create infographics, presentations and other scientifically-accurate designs without hassle — absolutely free for 7 days!
About Fabricio Pamplona
Fabricio Pamplona is the founder of Mind the Graph - a tool used by over 400K users in 60 countries. He has a Ph.D. and solid scientific background in Psychopharmacology and experience as a Guest Researcher at the Max Planck Institute of Psychiatry (Germany) and Researcher in D'Or Institute for Research and Education (IDOR, Brazil). Fabricio holds over 2500 citations in Google Scholar. He has 10 years of experience in small innovative businesses, with relevant experience in product design and innovation management. Connect with him on LinkedIn - Fabricio Pamplona .
Content tags

Reference management. Clean and simple.
What is a good impact factor?
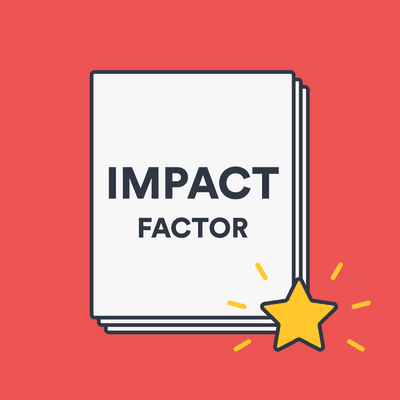
What is an impact factor?
How is an impact factor calculated, how to find the impact factor of a journal, frequently asked questions about impact factors, related articles.
An impact factor measures the average number of a journal's citations in a two-year period. Ultimately, this measure calculates the rank of the journal in question. The more citations a journal has, the higher ranked it is. With higher ranking comes more popularity, and most importantly, credibility.
The calculation of the impact factor of a journal is quite easy. The number of citations of a journal is divided by the number of citable articles (from the same journal) from a two-year period.
X= the number of times articles published in 2018 and 2019 were cited by indexed journals during 2020
Y= the total number of published (citable) articles in 2018 and 2019
X/Y= 2020 impact factor of a journal
Usually, the impact factor of a journal is measured by different entities. You can find a journal's impact factor by referring to the Journal Citations Report (JCR), Scopus , or Resurchify . You only need to type in the title, publisher’s name, ISSN, or search by subject category.
It’s worth highlighting that the impact factor is used to compare journals from the same fields. A history journal cannot be compared to a science journal. Therefore, there is no set impact factor number considered to be ideal since each field has a different measurement. In general, an impact factor of 10 or higher is considered remarkable, while 3 is good, and the average score is less than 1.
The very prestigious journal Nature had an impact factor of 69.504 in the year 2021.
➡️ Learn more: What is a good h-index?
An impact factor measures the average number of a journal's citations, in a two-year period. Ultimately, this measure calculates the rank of the journal in question.
The number of citations of a journal is divided by the number of citable articles (from the same journal) from a two year period.
X= the number of cited articles from 2018 and 2019 in 2020
Y= the number of published articles in 2018 and 2019
You can find a journal's impact factor by referring to the Journal Citations Report (JCR) or Scopus .
In general, an impact factor of 10 or higher is considered remarkable, while 3 is good, and the average score is less than 1.
Eugene Garfield, the founder of the Institute for Scientific Information (ISI), invented the measurement known as impact factor. You can read more about this in Origins of the journal impact factor .
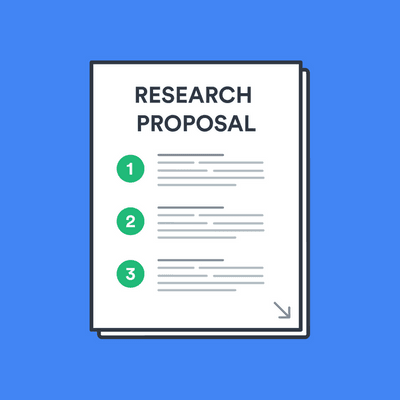
- All subject areas
- Agricultural and Biological Sciences
- Arts and Humanities
- Biochemistry, Genetics and Molecular Biology
- Business, Management and Accounting
- Chemical Engineering
- Computer Science
- Decision Sciences
- Earth and Planetary Sciences
- Economics, Econometrics and Finance
- Engineering
- Environmental Science
- Health Professions
- Immunology and Microbiology
- Materials Science
- Mathematics
- Multidisciplinary
- Neuroscience
- Pharmacology, Toxicology and Pharmaceutics
- Physics and Astronomy
- Social Sciences
- All subject categories
- Acoustics and Ultrasonics
- Advanced and Specialized Nursing
- Aerospace Engineering
- Agricultural and Biological Sciences (miscellaneous)
- Agronomy and Crop Science
- Algebra and Number Theory
- Analytical Chemistry
- Anesthesiology and Pain Medicine
- Animal Science and Zoology
- Anthropology
- Applied Mathematics
- Applied Microbiology and Biotechnology
- Applied Psychology
- Aquatic Science
- Archeology (arts and humanities)
- Architecture
- Artificial Intelligence
- Arts and Humanities (miscellaneous)
- Assessment and Diagnosis
- Astronomy and Astrophysics
- Atmospheric Science
- Atomic and Molecular Physics, and Optics
- Automotive Engineering
- Behavioral Neuroscience
- Biochemistry
- Biochemistry, Genetics and Molecular Biology (miscellaneous)
- Biochemistry (medical)
- Bioengineering
- Biological Psychiatry
- Biomaterials
- Biomedical Engineering
- Biotechnology
- Building and Construction
- Business and International Management
- Business, Management and Accounting (miscellaneous)
- Cancer Research
- Cardiology and Cardiovascular Medicine
- Care Planning
- Cell Biology
- Cellular and Molecular Neuroscience
- Ceramics and Composites
- Chemical Engineering (miscellaneous)
- Chemical Health and Safety
- Chemistry (miscellaneous)
- Chiropractics
- Civil and Structural Engineering
- Clinical Biochemistry
- Clinical Psychology
- Cognitive Neuroscience
- Colloid and Surface Chemistry
- Communication
- Community and Home Care
- Complementary and Alternative Medicine
- Complementary and Manual Therapy
- Computational Mathematics
- Computational Mechanics
- Computational Theory and Mathematics
- Computer Graphics and Computer-Aided Design
- Computer Networks and Communications
- Computer Science Applications
- Computer Science (miscellaneous)
- Computer Vision and Pattern Recognition
- Computers in Earth Sciences
- Condensed Matter Physics
- Conservation
- Control and Optimization
- Control and Systems Engineering
- Critical Care and Intensive Care Medicine
- Critical Care Nursing
- Cultural Studies
- Decision Sciences (miscellaneous)
- Dental Assisting
- Dental Hygiene
- Dentistry (miscellaneous)
- Dermatology
- Development
- Developmental and Educational Psychology
- Developmental Biology
- Developmental Neuroscience
- Discrete Mathematics and Combinatorics
- Drug Discovery
- Drug Guides
- Earth and Planetary Sciences (miscellaneous)
- Earth-Surface Processes
- Ecological Modeling
- Ecology, Evolution, Behavior and Systematics
- Economic Geology
- Economics and Econometrics
- Economics, Econometrics and Finance (miscellaneous)
- Electrical and Electronic Engineering
- Electrochemistry
- Electronic, Optical and Magnetic Materials
- Emergency Medical Services
- Emergency Medicine
- Emergency Nursing
- Endocrine and Autonomic Systems
- Endocrinology
- Endocrinology, Diabetes and Metabolism
- Energy Engineering and Power Technology
- Energy (miscellaneous)
- Engineering (miscellaneous)
- Environmental Chemistry
- Environmental Engineering
- Environmental Science (miscellaneous)
- Epidemiology
- Experimental and Cognitive Psychology
- Family Practice
- Filtration and Separation
- Fluid Flow and Transfer Processes
- Food Animals
- Food Science
- Fuel Technology
- Fundamentals and Skills
- Gastroenterology
- Gender Studies
- Genetics (clinical)
- Geochemistry and Petrology
- Geography, Planning and Development
- Geometry and Topology
- Geotechnical Engineering and Engineering Geology
- Geriatrics and Gerontology
- Gerontology
- Global and Planetary Change
- Hardware and Architecture
- Health Informatics
- Health Information Management
- Health Policy
- Health Professions (miscellaneous)
- Health (social science)
- Health, Toxicology and Mutagenesis
- History and Philosophy of Science
- Horticulture
- Human Factors and Ergonomics
- Human-Computer Interaction
- Immunology and Allergy
- Immunology and Microbiology (miscellaneous)
- Industrial and Manufacturing Engineering
- Industrial Relations
- Infectious Diseases
- Information Systems
- Information Systems and Management
- Inorganic Chemistry
- Insect Science
- Instrumentation
- Internal Medicine
- Issues, Ethics and Legal Aspects
- Leadership and Management
- Library and Information Sciences
- Life-span and Life-course Studies
- Linguistics and Language
- Literature and Literary Theory
- LPN and LVN
- Management Information Systems
- Management, Monitoring, Policy and Law
- Management of Technology and Innovation
- Management Science and Operations Research
- Materials Chemistry
- Materials Science (miscellaneous)
- Maternity and Midwifery
- Mathematical Physics
- Mathematics (miscellaneous)
- Mechanical Engineering
- Mechanics of Materials
- Media Technology
- Medical and Surgical Nursing
- Medical Assisting and Transcription
- Medical Laboratory Technology
- Medical Terminology
- Medicine (miscellaneous)
- Metals and Alloys
- Microbiology
- Microbiology (medical)
- Modeling and Simulation
- Molecular Biology
- Molecular Medicine
- Nanoscience and Nanotechnology
- Nature and Landscape Conservation
- Neurology (clinical)
- Neuropsychology and Physiological Psychology
- Neuroscience (miscellaneous)
- Nuclear and High Energy Physics
- Nuclear Energy and Engineering
- Numerical Analysis
- Nurse Assisting
- Nursing (miscellaneous)
- Nutrition and Dietetics
- Obstetrics and Gynecology
- Occupational Therapy
- Ocean Engineering
- Oceanography
- Oncology (nursing)
- Ophthalmology
- Oral Surgery
- Organic Chemistry
- Organizational Behavior and Human Resource Management
- Orthodontics
- Orthopedics and Sports Medicine
- Otorhinolaryngology
- Paleontology
- Parasitology
- Pathology and Forensic Medicine
- Pathophysiology
- Pediatrics, Perinatology and Child Health
- Periodontics
- Pharmaceutical Science
- Pharmacology
- Pharmacology (medical)
- Pharmacology (nursing)
- Pharmacology, Toxicology and Pharmaceutics (miscellaneous)
- Physical and Theoretical Chemistry
- Physical Therapy, Sports Therapy and Rehabilitation
- Physics and Astronomy (miscellaneous)
- Physiology (medical)
- Plant Science
- Political Science and International Relations
- Polymers and Plastics
- Process Chemistry and Technology
- Psychiatry and Mental Health
- Psychology (miscellaneous)
- Public Administration
- Public Health, Environmental and Occupational Health
- Pulmonary and Respiratory Medicine
- Radiological and Ultrasound Technology
- Radiology, Nuclear Medicine and Imaging
- Rehabilitation
- Religious Studies
- Renewable Energy, Sustainability and the Environment
- Reproductive Medicine
- Research and Theory
- Respiratory Care
- Review and Exam Preparation
- Reviews and References (medical)
- Rheumatology
- Safety Research
- Safety, Risk, Reliability and Quality
- Sensory Systems
- Signal Processing
- Small Animals
- Social Psychology
- Social Sciences (miscellaneous)
- Social Work
- Sociology and Political Science
- Soil Science
- Space and Planetary Science
- Spectroscopy
- Speech and Hearing
- Sports Science
- Statistical and Nonlinear Physics
- Statistics and Probability
- Statistics, Probability and Uncertainty
- Strategy and Management
- Stratigraphy
- Structural Biology
- Surfaces and Interfaces
- Surfaces, Coatings and Films
- Theoretical Computer Science
- Tourism, Leisure and Hospitality Management
- Transplantation
- Transportation
- Urban Studies
- Veterinary (miscellaneous)
- Visual Arts and Performing Arts
- Waste Management and Disposal
- Water Science and Technology
- All regions / countries
- Asiatic Region
- Eastern Europe
- Latin America
- Middle East
- Northern America
- Pacific Region
- Western Europe
- ARAB COUNTRIES
- IBEROAMERICA
- NORDIC COUNTRIES
- Afghanistan
- Bosnia and Herzegovina
- Brunei Darussalam
- Czech Republic
- Dominican Republic
- Netherlands
- New Caledonia
- New Zealand
- Papua New Guinea
- Philippines
- Puerto Rico
- Russian Federation
- Saudi Arabia
- South Africa
- South Korea
- Switzerland
- Syrian Arab Republic
- Trinidad and Tobago
- United Arab Emirates
- United Kingdom
- United States
- Vatican City State
- Book Series
- Conferences and Proceedings
- Trade Journals

- Citable Docs. (3years)
- Total Cites (3years)

Follow us on @ScimagoJR Scimago Lab , Copyright 2007-2024. Data Source: Scopus®

Cookie settings
Cookie Policy
Legal Notice
Privacy Policy
Thank you for visiting nature.com. You are using a browser version with limited support for CSS. To obtain the best experience, we recommend you use a more up to date browser (or turn off compatibility mode in Internet Explorer). In the meantime, to ensure continued support, we are displaying the site without styles and JavaScript.
- View all journals
- My Account Login
- Explore content
- About the journal
- Publish with us
- Sign up for alerts
- Open access
- Published: 17 October 2023
The impact of founder personalities on startup success
- Paul X. McCarthy 1 , 2 ,
- Xian Gong 3 ,
- Fabian Braesemann 4 , 5 ,
- Fabian Stephany 4 , 5 ,
- Marian-Andrei Rizoiu 3 &
- Margaret L. Kern 6
Scientific Reports volume 13 , Article number: 17200 ( 2023 ) Cite this article
59k Accesses
2 Citations
305 Altmetric
Metrics details
- Human behaviour
- Information technology
An Author Correction to this article was published on 07 May 2024
This article has been updated
Startup companies solve many of today’s most challenging problems, such as the decarbonisation of the economy or the development of novel life-saving vaccines. Startups are a vital source of innovation, yet the most innovative are also the least likely to survive. The probability of success of startups has been shown to relate to several firm-level factors such as industry, location and the economy of the day. Still, attention has increasingly considered internal factors relating to the firm’s founding team, including their previous experiences and failures, their centrality in a global network of other founders and investors, as well as the team’s size. The effects of founders’ personalities on the success of new ventures are, however, mainly unknown. Here, we show that founder personality traits are a significant feature of a firm’s ultimate success. We draw upon detailed data about the success of a large-scale global sample of startups (n = 21,187). We find that the Big Five personality traits of startup founders across 30 dimensions significantly differ from that of the population at large. Key personality facets that distinguish successful entrepreneurs include a preference for variety, novelty and starting new things (openness to adventure), like being the centre of attention (lower levels of modesty) and being exuberant (higher activity levels). We do not find one ’Founder-type’ personality; instead, six different personality types appear. Our results also demonstrate the benefits of larger, personality-diverse teams in startups, which show an increased likelihood of success. The findings emphasise the role of the diversity of personality types as a novel dimension of team diversity that influences performance and success.
Similar content being viewed by others
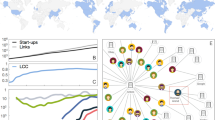
Predicting success in the worldwide start-up network
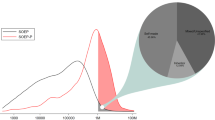
The personality traits of self-made and inherited millionaires
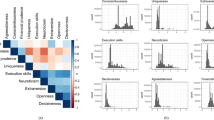
The nexus of top executives’ attributes, firm strategies, and outcomes: Large firms versus SMEs
Introduction.
The success of startups is vital to economic growth and renewal, with a small number of young, high-growth firms creating a disproportionately large share of all new jobs 1 , 2 . Startups create jobs and drive economic growth, and they are also an essential vehicle for solving some of society’s most pressing challenges.
As a poignant example, six centuries ago, the German city of Mainz was abuzz as the birthplace of the world’s first moveable-type press created by Johannes Gutenberg. However, in the early part of this century, it faced several economic challenges, including rising unemployment and a significant and growing municipal debt. Then in 2008, two Turkish immigrants formed the company BioNTech in Mainz with another university research colleague. Together they pioneered new mRNA-based technologies. In 2020, BioNTech partnered with US pharmaceutical giant Pfizer to create one of only a handful of vaccines worldwide for Covid-19, saving an estimated six million lives 3 . The economic benefit to Europe and, in particular, the German city where the vaccine was developed has been significant, with windfall tax receipts to the government clearing Mainz’s €1.3bn debt and enabling tax rates to be reduced, attracting other businesses to the region as well as inspiring a whole new generation of startups 4 .
While stories such as the success of BioNTech are often retold and remembered, their success is the exception rather than the rule. The overwhelming majority of startups ultimately fail. One study of 775 startups in Canada that successfully attracted external investment found only 35% were still operating seven years later 5 .
But what determines the success of these ‘lucky few’? When assessing the success factors of startups, especially in the early-stage unproven phase, venture capitalists and other investors offer valuable insights. Three different schools of thought characterise their perspectives: first, supply-side or product investors : those who prioritise investing in firms they consider to have novel and superior products and services, investing in companies with intellectual property such as patents and trademarks. Secondly, demand-side or market-based investors : those who prioritise investing in areas of highest market interest, such as in hot areas of technology like quantum computing or recurrent or emerging large-scale social and economic challenges such as the decarbonisation of the economy. Thirdly, talent investors : those who prioritise the foundation team above the startup’s initial products or what industry or problem it is looking to address.
Investors who adopt the third perspective and prioritise talent often recognise that a good team can overcome many challenges in the lead-up to product-market fit. And while the initial products of a startup may or may not work a successful and well-functioning team has the potential to pivot to new markets and new products, even if the initial ones prove untenable. Not surprisingly, an industry ‘autopsy’ into 101 tech startup failures found 23% were due to not having the right team—the number three cause of failure ahead of running out of cash or not having a product that meets the market need 6 .
Accordingly, early entrepreneurship research was focused on the personality of founders, but the focus shifted away in the mid-1980s onwards towards more environmental factors such as venture capital financing 7 , 8 , 9 , networks 10 , location 11 and due to a range of issues and challenges identified with the early entrepreneurship personality research 12 , 13 . At the turn of the 21st century, some scholars began exploring ways to combine context and personality and reconcile entrepreneurs’ individual traits with features of their environment. In her influential work ’The Sociology of Entrepreneurship’, Patricia H. Thornton 14 discusses two perspectives on entrepreneurship: the supply-side perspective (personality theory) and the demand-side perspective (environmental approach). The supply-side perspective focuses on the individual traits of entrepreneurs. In contrast, the demand-side perspective focuses on the context in which entrepreneurship occurs, with factors such as finance, industry and geography each playing their part. In the past two decades, there has been a revival of interest and research that explores how entrepreneurs’ personality relates to the success of their ventures. This new and growing body of research includes several reviews and meta-studies, which show that personality traits play an important role in both career success and entrepreneurship 15 , 16 , 17 , 18 , 19 , that there is heterogeneity in definitions and samples used in research on entrepreneurship 16 , 18 , and that founder personality plays an important role in overall startup outcomes 17 , 19 .
Motivated by the pivotal role of the personality of founders on startup success outlined in these recent contributions, we investigate two main research questions:
Which personality features characterise founders?
Do their personalities, particularly the diversity of personality types in founder teams, play a role in startup success?
We aim to understand whether certain founder personalities and their combinations relate to startup success, defined as whether their company has been acquired, acquired another company or listed on a public stock exchange. For the quantitative analysis, we draw on a previously published methodology 20 , which matches people to their ‘ideal’ jobs based on social media-inferred personality traits.
We find that personality traits matter for startup success. In addition to firm-level factors of location, industry and company age, we show that founders’ specific Big Five personality traits, such as adventurousness and openness, are significantly more widespread among successful startups. As we find that companies with multi-founder teams are more likely to succeed, we cluster founders in six different and distinct personality groups to underline the relevance of the complementarity in personality traits among founder teams. Startups with diverse and specific combinations of founder types (e. g., an adventurous ‘Leader’, a conscientious ‘Accomplisher’, and an extroverted ‘Developer’) have significantly higher odds of success.
We organise the rest of this paper as follows. In the Section " Results ", we introduce the data used and the methods applied to relate founders’ psychological traits with their startups’ success. We introduce the natural language processing method to derive individual and team personality characteristics and the clustering technique to identify personality groups. Then, we present the result for multi-variate regression analysis that allows us to relate firm success with external and personality features. Subsequently, the Section " Discussion " mentions limitations and opportunities for future research in this domain. In the Section " Methods ", we describe the data, the variables in use, and the clustering in greater detail. Robustness checks and additional analyses can be found in the Supplementary Information.
Our analysis relies on two datasets. We infer individual personality facets via a previously published methodology 20 from Twitter user profiles. Here, we restrict our analysis to founders with a Crunchbase profile. Crunchbase is the world’s largest directory on startups. It provides information about more than one million companies, primarily focused on funding and investors. A company’s public Crunchbase profile can be considered a digital business card of an early-stage venture. As such, the founding teams tend to provide information about themselves, including their educational background or a link to their Twitter account.
We infer the personality profiles of the founding teams of early-stage ventures from their publicly available Twitter profiles, using the methodology described by Kern et al. 20 . Then, we correlate this information to data from Crunchbase to determine whether particular combinations of personality traits correspond to the success of early-stage ventures. The final dataset used in the success prediction model contains n = 21,187 startup companies (for more details on the data see the Methods section and SI section A.5 ).
Revisions of Crunchbase as a data source for investigations on a firm and industry level confirm the platform to be a useful and valuable source of data for startups research, as comparisons with other sources at micro-level, e.g., VentureXpert or PwC, also suggest that the platform’s coverage is very comprehensive, especially for start-ups located in the United States 21 . Moreover, aggregate statistics on funding rounds by country and year are quite similar to those produced with other established sources, going to validate the use of Crunchbase as a reliable source in terms of coverage of funded ventures. For instance, Crunchbase covers about the same number of investment rounds in the analogous sectors as collected by the National Venture Capital Association 22 . However, we acknowledge that the data source might suffer from registration latency (a certain delay between the foundation of the company and its actual registration on Crunchbase) and success bias in company status (the likeliness that failed companies decide to delete their profile from the database).
The definition of startup success
The success of startups is uncertain, dependent on many factors and can be measured in various ways. Due to the likelihood of failure in startups, some large-scale studies have looked at which features predict startup survival rates 23 , and others focus on fundraising from external investors at various stages 24 . Success for startups can be measured in multiple ways, such as the amount of external investment attracted, the number of new products shipped or the annual growth in revenue. But sometimes external investments are misguided, revenue growth can be short-lived, and new products may fail to find traction.
Success in a startup is typically staged and can appear in different forms and times. For example, a startup may be seen to be successful when it finds a clear solution to a widely recognised problem, such as developing a successful vaccine. On the other hand, it could be achieving some measure of commercial success, such as rapidly accelerating sales or becoming profitable or at least cash positive. Or it could be reaching an exit for foundation investors via a trade sale, acquisition or listing of its shares for sale on a public stock exchange via an Initial Public Offering (IPO).
For our study, we focused on the startup’s extrinsic success rather than the founders’ intrinsic success per se, as its more visible, objective and measurable. A frequently considered measure of success is the attraction of external investment by venture capitalists 25 . However, this is not in and of itself a good measure of clear, incontrovertible success, particularly for early-stage ventures. This is because it reflects investors’ expectations of a startup’s success potential rather than actual business success. Similarly, we considered other measures like revenue growth 26 , liquidity events 27 , 28 , 29 , profitability 30 and social impact 31 , all of which have benefits as they capture incremental success, but each also comes with operational measurement challenges.
Therefore, we apply the success definition initially introduced by Bonaventura et al. 32 , namely that a startup is acquired, acquires another company or has an initial public offering (IPO). We consider any of these major capital liquidation events as a clear threshold signal that the company has matured from an early-stage venture to becoming or is on its way to becoming a mature company with clear and often significant business growth prospects. Together these three major liquidity events capture the primary forms of exit for external investors (an acquisition or trade sale and an IPO). For companies with a longer autonomous growth runway, acquiring another company marks a similar milestone of scale, maturity and capability.
Using multifactor analysis and a binary classification prediction model of startup success, we looked at many variables together and their relative influence on the probability of the success of startups. We looked at seven categories of factors through three lenses of firm-level factors: (1) location, (2) industry, (3) age of the startup; founder-level factors: (4) number of founders, (5) gender of founders, (6) personality characteristics of founders and; lastly team-level factors: (7) founder-team personality combinations. The model performance and relative impacts on the probability of startup success of each of these categories of founders are illustrated in more detail in section A.6 of the Supplementary Information (in particular Extended Data Fig. 19 and Extended Data Fig. 20 ). In total, we considered over three hundred variables (n = 323) and their relative significant associations with success.
The personality of founders
Besides product-market, industry, and firm-level factors (see SI section A.1 ), research suggests that the personalities of founders play a crucial role in startup success 19 . Therefore, we examine the personality characteristics of individual startup founders and teams of founders in relationship to their firm’s success by applying the success definition used by Bonaventura et al. 32 .
Employing established methods 33 , 34 , 35 , we inferred the personality traits across 30 dimensions (Big Five facets) of a large global sample of startup founders. The startup founders cohort was created from a subset of founders from the global startup industry directory Crunchbase, who are also active on the social media platform Twitter.
To measure the personality of the founders, we used the Big Five, a popular model of personality which includes five core traits: Openness to Experience, Conscientiousness, Extraversion, Agreeableness, and Emotional stability. Each of these traits can be further broken down into thirty distinct facets. Studies have found that the Big Five predict meaningful life outcomes, such as physical and mental health, longevity, social relationships, health-related behaviours, antisocial behaviour, and social contribution, at levels on par with intelligence and socioeconomic status 36 Using machine learning to infer personality traits by analysing the use of language and activity on social media has been shown to be more accurate than predictions of coworkers, friends and family and similar in accuracy to the judgement of spouses 37 . Further, as other research has shown, we assume that personality traits remain stable in adulthood even through significant life events 38 , 39 , 40 . Personality traits have been shown to emerge continuously from those already evident in adolescence 41 and are not significantly influenced by external life events such as becoming divorced or unemployed 42 . This suggests that the direction of any measurable effect goes from founder personalities to startup success and not vice versa.
As a first investigation to what extent personality traits might relate to entrepreneurship, we use the personality characteristics of individuals to predict whether they were an entrepreneur or an employee. We trained and tested a machine-learning random forest classifier to distinguish and classify entrepreneurs from employees and vice-versa using inferred personality vectors alone. As a result, we found we could correctly predict entrepreneurs with 77% accuracy and employees with 88% accuracy (Fig. 1 A). Thus, based on personality information alone, we correctly predict all unseen new samples with 82.5% accuracy (See SI section A.2 for more details on this analysis, the classification modelling and prediction accuracy).
We explored in greater detail which personality features are most prominent among entrepreneurs. We found that the subdomain or facet of Adventurousness within the Big Five Domain of Openness was significant and had the largest effect size. The facet of Modesty within the Big Five Domain of Agreeableness and Activity Level within the Big Five Domain of Extraversion was the subsequent most considerable effect (Fig. 1 B). Adventurousness in the Big Five framework is defined as the preference for variety, novelty and starting new things—which are consistent with the role of a startup founder whose role, especially in the early life of the company, is to explore things that do not scale easily 43 and is about developing and testing new products, services and business models with the market.
Once we derived and tested the Big Five personality features for each entrepreneur in our data set, we examined whether there is evidence indicating that startup founders naturally cluster according to their personality features using a Hopkins test (see Extended Data Figure 6 ). We discovered clear clustering tendencies in the data compared with other renowned reference data sets known to have clusters. Then, once we established the founder data clusters, we used agglomerative hierarchical clustering. This ‘bottom-up’ clustering technique initially treats each observation as an individual cluster. Then it merges them to create a hierarchy of possible cluster schemes with differing numbers of groups (See Extended Data Fig. 7 ). And lastly, we identified the optimum number of clusters based on the outcome of four different clustering performance measurements: Davies-Bouldin Index, Silhouette coefficients, Calinski-Harabas Index and Dunn Index (see Extended Data Figure 8 ). We find that the optimum number of clusters of startup founders based on their personality features is six (labelled #0 through to #5), as shown in Fig. 1 C.
To better understand the context of different founder types, we positioned each of the six types of founders within an occupation-personality matrix established from previous research 44 . This research showed that ‘each job has its own personality’ using a substantial sample of employees across various jobs. Utilising the methodology employed in this study, we assigned labels to the cluster names #0 to #5, which correspond to the identified occupation tribes that best describe the personality facets represented by the clusters (see Extended Data Fig. 9 for an overview of these tribes, as identified by McCarthy et al. 44 ).
Utilising this approach, we identify three ’purebred’ clusters: #0, #2 and #5, whose members are dominated by a single tribe (larger than 60% of all individuals in each cluster are characterised by one tribe). Thus, these clusters represent and share personality attributes of these previously identified occupation-personality tribes 44 , which have the following known distinctive personality attributes (see also Table 1 ):
Accomplishers (#0) —Organised & outgoing. confident, down-to-earth, content, accommodating, mild-tempered & self-assured.
Leaders (#2) —Adventurous, persistent, dispassionate, assertive, self-controlled, calm under pressure, philosophical, excitement-seeking & confident.
Fighters (#5) —Spontaneous and impulsive, tough, sceptical, and uncompromising.
We labelled these clusters with the tribe names, acknowledging that labels are somewhat arbitrary, based on our best interpretation of the data (See SI section A.3 for more details).
For the remaining three clusters #1, #3 and #4, we can see they are ‘hybrids’, meaning that the founders within them come from a mix of different tribes, with no one tribe representing more than 50% of the members of that cluster. However, the tribes with the largest share were noted as #1 Experts/Engineers, #3 Fighters, and #4 Operators.
To label these three hybrid clusters, we examined the closest occupations to the median personality features of each cluster. We selected a name that reflected the common themes of these occupations, namely:
Experts/Engineers (#1) as the closest roles included Materials Engineers and Chemical Engineers. This is consistent with this cluster’s personality footprint, which is highest in openness in the facets of imagination and intellect.
Developers (#3) as the closest roles include Application Developers and related technology roles such as Business Systems Analysts and Product Managers.
Operators (#4) as the closest roles include service, maintenance and operations functions, including Bicycle Mechanic, Mechanic and Service Manager. This is also consistent with one of the key personality traits of high conscientiousness in the facet of orderliness and high agreeableness in the facet of humility for founders in this cluster.
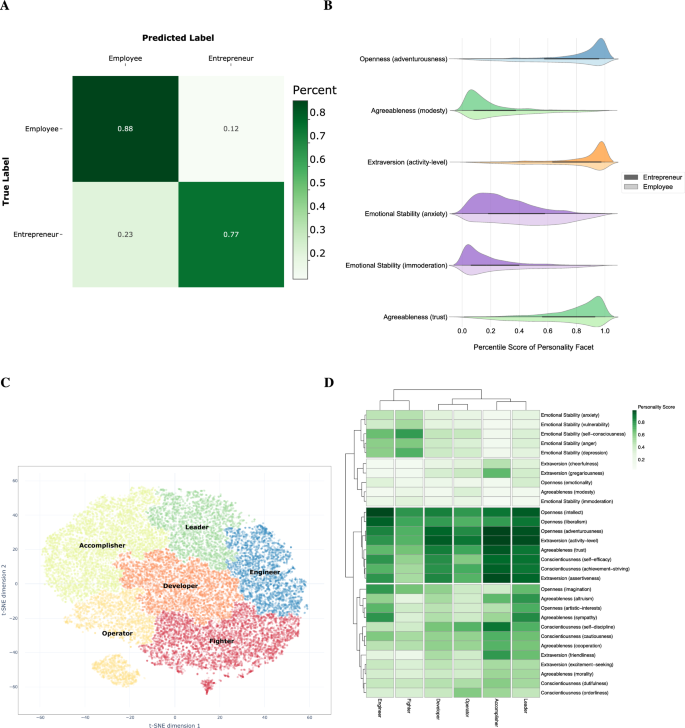
Founder-Level Factors of Startup Success. ( A ), Successful entrepreneurs differ from successful employees. They can be accurately distinguished using a classifier with personality information alone. ( B ), Successful entrepreneurs have different Big Five facet distributions, especially on adventurousness, modesty and activity level. ( C ), Founders come in six different types: Fighters, Operators, Accomplishers, Leaders, Engineers and Developers (FOALED) ( D ), Each founder Personality-Type has its distinct facet.
Together, these six different types of startup founders (Fig. 1 C) represent a framework we call the FOALED model of founder types—an acronym of Fighters, Operators, Accomplishers, Leaders, Engineers and D evelopers.
Each founder’s personality type has its distinct facet footprint (for more details, see Extended Data Figure 10 in SI section A.3 ). Also, we observe a central core of correlated features that are high for all types of entrepreneurs, including intellect, adventurousness and activity level (Fig. 1 D).To test the robustness of the clustering of the personality facets, we compare the mean scores of the individual facets per cluster with a 20-fold resampling of the data and find that the clusters are, overall, largely robust against resampling (see Extended Data Figure 11 in SI section A.3 for more details).
We also find that the clusters accord with the distribution of founders’ roles in their startups. For example, Accomplishers are often Chief Executive Officers, Chief Financial Officers, or Chief Operating Officers, while Fighters tend to be Chief Technical Officers, Chief Product Officers, or Chief Commercial Officers (see Extended Data Fig. 12 in SI section A.4 for more details).
The ensemble theory of success
While founders’ individual personality traits, such as Adventurousness or Openness, show to be related to their firms’ success, we also hypothesise that the combination, or ensemble, of personality characteristics of a founding team impacts the chances of success. The logic behind this reasoning is complementarity, which is proposed by contemporary research on the functional roles of founder teams. Examples of these clear functional roles have evolved in established industries such as film and television, construction, and advertising 45 . When we subsequently explored the combinations of personality types among founders and their relationship to the probability of startup success, adjusted for a range of other factors in a multi-factorial analysis, we found significantly increased chances of success for mixed foundation teams:
Initially, we find that firms with multiple founders are more likely to succeed, as illustrated in Fig. 2 A, which shows firms with three or more founders are more than twice as likely to succeed than solo-founded startups. This finding is consistent with investors’ advice to founders and previous studies 46 . We also noted that some personality types of founders increase the probability of success more than others, as shown in SI section A.6 (Extended Data Figures 16 and 17 ). Also, we note that gender differences play out in the distribution of personality facets: successful female founders and successful male founders show facet scores that are more similar to each other than are non-successful female founders to non-successful male founders (see Extended Data Figure 18 ).
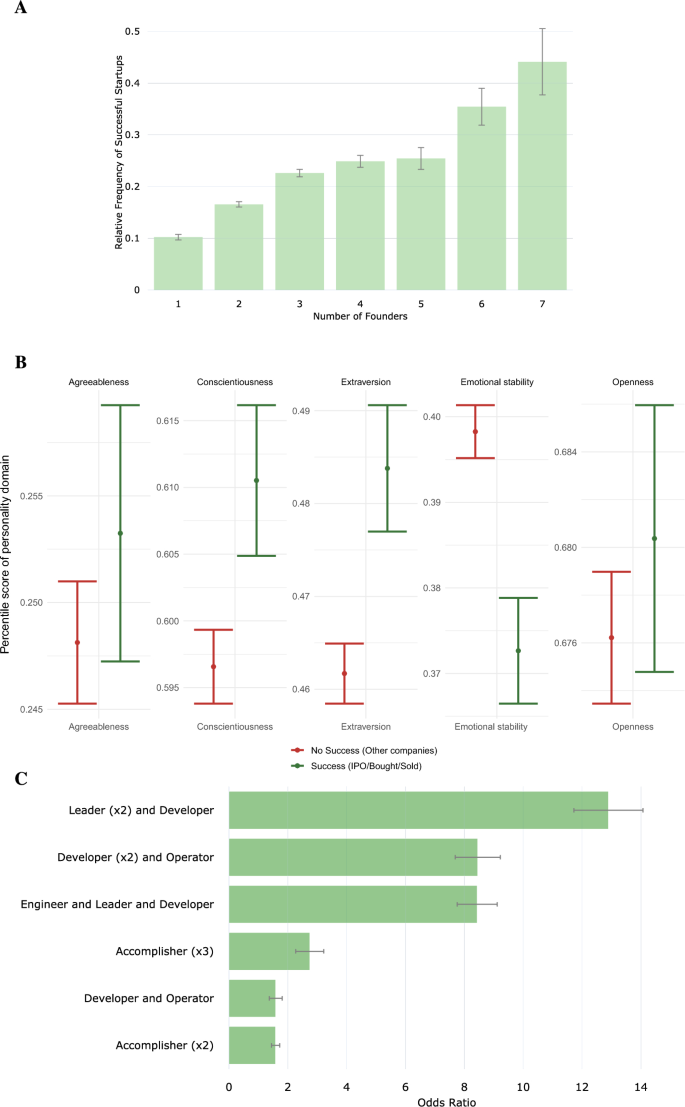
The Ensemble Theory of Team-Level Factors of Startup Success. ( A ) Having a larger founder team elevates the chances of success. This can be due to multiple reasons, e.g., a more extensive network or knowledge base but also personality diversity. ( B ) We show that joint personality combinations of founders are significantly related to higher chances of success. This is because it takes more than one founder to cover all beneficial personality traits that ‘breed’ success. ( C ) In our multifactor model, we show that firms with diverse and specific combinations of types of founders have significantly higher odds of success.
Access to more extensive networks and capital could explain the benefits of having more founders. Still, as we find here, it also offers a greater diversity of combined personalities, naturally providing a broader range of maximum traits. So, for example, one founder may be more open and adventurous, and another could be highly agreeable and trustworthy, thus, potentially complementing each other’s particular strengths associated with startup success.
The benefits of larger and more personality-diverse foundation teams can be seen in the apparent differences between successful and unsuccessful firms based on their combined Big Five personality team footprints, as illustrated in Fig. 2 B. Here, maximum values for each Big Five trait of a startup’s co-founders are mapped; stratified by successful and non-successful companies. Founder teams of successful startups tend to score higher on Openness, Conscientiousness, Extraversion, and Agreeableness.
When examining the combinations of founders with different personality types, we find that some ensembles of personalities were significantly correlated with greater chances of startup success—while controlling for other variables in the model—as shown in Fig. 2 C (for more details on the modelling, the predictive performance and the coefficient estimates of the final model, see Extended Data Figures 19 , 20 , and 21 in SI section A.6 ).
Three combinations of trio-founder companies were more than twice as likely to succeed than other combinations, namely teams with (1) a Leader and two Developers , (2) an Operator and two Developers , and (3) an Expert/Engineer , Leader and Developer . To illustrate the potential mechanisms on how personality traits might influence the success of startups, we provide some examples of well-known, successful startup founders and their characteristic personality traits in Extended Data Figure 22 .
Startups are one of the key mechanisms for brilliant ideas to become solutions to some of the world’s most challenging economic and social problems. Examples include the Google search algorithm, disability technology startup Fingerwork’s touchscreen technology that became the basis of the Apple iPhone, or the Biontech mRNA technology that powered Pfizer’s COVID-19 vaccine.
We have shown that founders’ personalities and the combination of personalities in the founding team of a startup have a material and significant impact on its likelihood of success. We have also shown that successful startup founders’ personality traits are significantly different from those of successful employees—so much so that a simple predictor can be trained to distinguish between employees and entrepreneurs with more than 80% accuracy using personality trait data alone.
Just as occupation-personality maps derived from data can provide career guidance tools, so too can data on successful entrepreneurs’ personality traits help people decide whether becoming a founder may be a good choice for them.
We have learnt through this research that there is not one type of ideal ’entrepreneurial’ personality but six different types. Many successful startups have multiple co-founders with a combination of these different personality types.
To a large extent, founding a startup is a team sport; therefore, diversity and complementarity of personalities matter in the foundation team. It has an outsized impact on the company’s likelihood of success. While all startups are high risk, the risk becomes lower with more founders, particularly if they have distinct personality traits.
Our work demonstrates the benefits of personality diversity among the founding team of startups. Greater awareness of this novel form of diversity may help create more resilient startups capable of more significant innovation and impact.
The data-driven research approach presented here comes with certain methodological limitations. The principal data sources of this study—Crunchbase and Twitter—are extensive and comprehensive, but there are characterised by some known and likely sample biases.
Crunchbase is the principal public chronicle of venture capital funding. So, there is some likely sample bias toward: (1) Startup companies that are funded externally: self-funded or bootstrapped companies are less likely to be represented in Crunchbase; (2) technology companies, as that is Crunchbase’s roots; (3) multi-founder companies; (4) male founders: while the representation of female founders is now double that of the mid-2000s, women still represent less than 25% of the sample; (5) companies that succeed: companies that fail, especially those that fail early, are likely to be less represented in the data.
Samples were also limited to those founders who are active on Twitter, which adds additional selection biases. For example, Twitter users typically are younger, more educated and have a higher median income 47 . Another limitation of our approach is the potentially biased presentation of a person’s digital identity on social media, which is the basis for identifying personality traits. For example, recent research suggests that the language and emotional tone used by entrepreneurs in social media can be affected by events such as business failure 48 , which might complicate the personality trait inference.
In addition to sampling biases within the data, there are also significant historical biases in startup culture. For many aspects of the entrepreneurship ecosystem, women, for example, are at a disadvantage 49 . Male-founded companies have historically dominated most startup ecosystems worldwide, representing the majority of founders and the overwhelming majority of venture capital investors. As a result, startups with women have historically attracted significantly fewer funds 50 , in part due to the male bias among venture investors, although this is now changing, albeit slowly 51 .
The research presented here provides quantitative evidence for the relevance of personality types and the diversity of personalities in startups. At the same time, it brings up other questions on how personality traits are related to other factors associated with success, such as:
Will the recent growing focus on promoting and investing in female founders change the nature, composition and dynamics of startups and their personalities leading to a more diverse personality landscape in startups?
Will the growth of startups outside of the United States change what success looks like to investors and hence the role of different personality traits and their association to diverse success metrics?
Many of today’s most renowned entrepreneurs are either Baby Boomers (such as Gates, Branson, Bloomberg) or Generation Xers (such as Benioff, Cannon-Brookes, Musk). However, as we can see, personality is both a predictor and driver of success in entrepreneurship. Will generation-wide differences in personality and outlook affect startups and their success?
Moreover, the findings shown here have natural extensions and applications beyond startups, such as for new projects within large established companies. While not technically startups, many large enterprises and industries such as construction, engineering and the film industry rely on forming new project-based, cross-functional teams that are often new ventures and share many characteristics of startups.
There is also potential for extending this research in other settings in government, NGOs, and within the research community. In scientific research, for example, team diversity in terms of age, ethnicity and gender has been shown to be predictive of impact, and personality diversity may be another critical dimension 52 .
Another extension of the study could investigate the development of the language used by startup founders on social media over time. Such an extension could investigate whether the language (and inferred psychological characteristics) change as the entrepreneurs’ ventures go through major business events such as foundation, funding, or exit.
Overall, this study demonstrates, first, that startup founders have significantly different personalities than employees. Secondly, besides firm-level factors, which are known to influence firm success, we show that a range of founder-level factors, notably the character traits of its founders, significantly impact a startup’s likelihood of success. Lastly, we looked at team-level factors. We discovered in a multifactor analysis that personality-diverse teams have the most considerable impact on the probability of a startup’s success, underlining the importance of personality diversity as a relevant factor of team performance and success.
Data sources
Entrepreneurs dataset.
Data about the founders of startups were collected from Crunchbase (Table 2 ), an open reference platform for business information about private and public companies, primarily early-stage startups. It is one of the largest and most comprehensive data sets of its kind and has been used in over 100 peer-reviewed research articles about economic and managerial research.
Crunchbase contains data on over two million companies - mainly startup companies and the companies who partner with them, acquire them and invest in them, as well as profiles on well over one million individuals active in the entrepreneurial ecosystem worldwide from over 200 countries and spans. Crunchbase started in the technology startup space, and it now covers all sectors, specifically focusing on entrepreneurship, investment and high-growth companies.
While Crunchbase contains data on over one million individuals in the entrepreneurial ecosystem, some are not entrepreneurs or startup founders but play other roles, such as investors, lawyers or executives at companies that acquire startups. To create a subset of only entrepreneurs, we selected a subset of 32,732 who self-identify as founders and co-founders (by job title) and who are also publicly active on the social media platform Twitter. We also removed those who also are venture capitalists to distinguish between investors and founders.
We selected founders active on Twitter to be able to use natural language processing to infer their Big Five personality features using an open-vocabulary approach shown to be accurate in the previous research by analysing users’ unstructured text, such as Twitter posts in our case. For this project, as with previous research 20 , we employed a commercial service, IBM Watson Personality Insight, to infer personality facets. This service provides raw scores and percentile scores of Big Five Domains (Openness, Conscientiousness, Extraversion, Agreeableness and Emotional Stability) and the corresponding 30 subdomains or facets. In addition, the public content of Twitter posts was collected, and there are 32,732 profiles that each had enough Twitter posts (more than 150 words) to get relatively accurate personality scores (less than 12.7% Average Mean Absolute Error).
The entrepreneurs’ dataset is analysed in combination with other data about the companies they founded to explore questions about the nature and patterns of personality traits of entrepreneurs and the relationships between these patterns and company success.
For the multifactor analysis, we further filtered the data in several preparatory steps for the success prediction modelling (for more details, see SI section A.5 ). In particular, we removed data points with missing values (Extended Data Fig. 13 ) and kept only companies in the data that were founded from 1990 onward to ensure consistency with previous research 32 (see Extended Data Fig. 14 ). After cleaning, filtering and pre-processing the data, we ended up with data from 25,214 founders who founded 21,187 startup companies to be used in the multifactor analysis. Of those, 3442 startups in the data were successful, 2362 in the first seven years after they were founded (see Extended Data Figure 15 for more details).
Entrepreneurs and employees dataset
To investigate whether startup founders show personality traits that are similar or different from the population at large (i. e. the entrepreneurs vs employees sub-analysis shown in Fig. 1 A and B), we filtered the entrepreneurs’ data further: we reduced the sample to those founders of companies, which attracted more than US$100k in investment to create a reference set of successful entrepreneurs (n \(=\) 4400).
To create a control group of employees who are not also entrepreneurs or very unlikely to be of have been entrepreneurs, we leveraged the fact that while some occupational titles like CEO, CTO and Public Speaker are commonly shared by founders and co-founders, some others such as Cashier , Zoologist and Detective very rarely co-occur seem to be founders or co-founders. To illustrate, many company founders also adopt regular occupation titles such as CEO or CTO. Many founders will be Founder and CEO or Co-founder and CTO. While founders are often CEOs or CTOs, the reverse is not necessarily true, as many CEOs are professional executives that were not involved in the establishment or ownership of the firm.
Using data from LinkedIn, we created an Entrepreneurial Occupation Index (EOI) based on the ratio of entrepreneurs for each of the 624 occupations used in a previous study of occupation-personality fit 44 . It was calculated based on the percentage of all people working in the occupation from LinkedIn compared to those who shared the title Founder or Co-founder (See SI section A.2 for more details). A reference set of employees (n=6685) was then selected across the 112 different occupations with the lowest propensity for entrepreneurship (less than 0.5% EOI) from a large corpus of Twitter users with known occupations, which is also drawn from the previous occupational-personality fit study 44 .
These two data sets were used to test whether it may be possible to distinguish successful entrepreneurs from successful employees based on the different patterns of personality traits alone.
Hierarchical clustering
We applied several clustering techniques and tests to the personality vectors of the entrepreneurs’ data set to determine if there are natural clusters and, if so, how many are the optimum number.
Firstly, to determine if there is a natural typology to founder personalities, we applied the Hopkins statistic—a statistical test we used to answer whether the entrepreneurs’ dataset contains inherent clusters. It measures the clustering tendency based on the ratio of the sum of distances of real points within a sample of the entrepreneurs’ dataset to their nearest neighbours and the sum of distances of randomly selected artificial points from a simulated uniform distribution to their nearest neighbours in the real entrepreneurs’ dataset. The ratio measures the difference between the entrepreneurs’ data distribution and the simulated uniform distribution, which tests the randomness of the data. The range of Hopkins statistics is from 0 to 1. The scores are close to 0, 0.5 and 1, respectively, indicating whether the dataset is uniformly distributed, randomly distributed or highly clustered.
To cluster the founders by personality facets, we used Agglomerative Hierarchical Clustering (AHC)—a bottom-up approach that treats an individual data point as a singleton cluster and then iteratively merges pairs of clusters until all data points are included in the single big collection. Ward’s linkage method is used to choose the pair of groups for minimising the increase in the within-cluster variance after combining. AHC was widely applied to clustering analysis since a tree hierarchy output is more informative and interpretable than K-means. Dendrograms were used to visualise the hierarchy to provide the perspective of the optimal number of clusters. The heights of the dendrogram represent the distance between groups, with lower heights representing more similar groups of observations. A horizontal line through the dendrogram was drawn to distinguish the number of significantly different clusters with higher heights. However, as it is not possible to determine the optimum number of clusters from the dendrogram, we applied other clustering performance metrics to analyse the optimal number of groups.
A range of Clustering performance metrics were used to help determine the optimal number of clusters in the dataset after an apparent clustering tendency was confirmed. The following metrics were implemented to evaluate the differences between within-cluster and between-cluster distances comprehensively: Dunn Index, Calinski-Harabasz Index, Davies-Bouldin Index and Silhouette Index. The Dunn Index measures the ratio of the minimum inter-cluster separation and the maximum intra-cluster diameter. At the same time, the Calinski-Harabasz Index improves the measurement of the Dunn Index by calculating the ratio of the average sum of squared dispersion of inter-cluster and intra-cluster. The Davies-Bouldin Index simplifies the process by treating each cluster individually. It compares the sum of the average distance among intra-cluster data points to the cluster centre of two separate groups with the distance between their centre points. Finally, the Silhouette Index is the overall average of the silhouette coefficients for each sample. The coefficient measures the similarity of the data point to its cluster compared with the other groups. Higher scores of the Dunn, Calinski-Harabasz and Silhouette Index and a lower score of the Davies-Bouldin Index indicate better clustering configuration.
Classification modelling
Classification algorithms.
To obtain a comprehensive and robust conclusion in the analysis predicting whether a given set of personality traits corresponds to an entrepreneur or an employee, we explored the following classifiers: Naïve Bayes, Elastic Net regularisation, Support Vector Machine, Random Forest, Gradient Boosting and Stacked Ensemble. The Naïve Bayes classifier is a probabilistic algorithm based on Bayes’ theorem with assumptions of independent features and equiprobable classes. Compared with other more complex classifiers, it saves computing time for large datasets and performs better if the assumptions hold. However, in the real world, those assumptions are generally violated. Elastic Net regularisation combines the penalties of Lasso and Ridge to regularise the Logistic classifier. It eliminates the limitation of multicollinearity in the Lasso method and improves the limitation of feature selection in the Ridge method. Even though Elastic Net is as simple as the Naïve Bayes classifier, it is more time-consuming. The Support Vector Machine (SVM) aims to find the ideal line or hyperplane to separate successful entrepreneurs and employees in this study. The dividing line can be non-linear based on a non-linear kernel, such as the Radial Basis Function Kernel. Therefore, it performs well on high-dimensional data while the ’right’ kernel selection needs to be tuned. Random Forest (RF) and Gradient Boosting Trees (GBT) are ensembles of decision trees. All trees are trained independently and simultaneously in RF, while a new tree is trained each time and corrected by previously trained trees in GBT. RF is a more robust and straightforward model since it does not have many hyperparameters to tune. GBT optimises the objective function and learns a more accurate model since there is a successive learning and correction process. Stacked Ensemble combines all existing classifiers through a Logistic Regression. Better than bagging with only variance reduction and boosting with only bias reduction, the ensemble leverages the benefit of model diversity with both lower variance and bias. All the above classification algorithms distinguish successful entrepreneurs and employees based on the personality matrix.
Evaluation metrics
A range of evaluation metrics comprehensively explains the performance of a classification prediction. The most straightforward metric is accuracy, which measures the overall portion of correct predictions. It will mislead the performance of an imbalanced dataset. The F1 score is better than accuracy by combining precision and recall and considering the False Negatives and False Positives. Specificity measures the proportion of detecting the true negative rate that correctly identifies employees, while Positive Predictive Value (PPV) calculates the probability of accurately predicting successful entrepreneurs. Area Under the Receiver Operating Characteristic Curve (AUROC) determines the capability of the algorithm to distinguish between successful entrepreneurs and employees. A higher value means the classifier performs better on separating the classes.
Feature importance
To further understand and interpret the classifier, it is critical to identify variables with significant predictive power on the target. Feature importance of tree-based models measures Gini importance scores for all predictors, which evaluate the overall impact of the model after cutting off the specific feature. The measurements consider all interactions among features. However, it does not provide insights into the directions of impacts since the importance only indicates the ability to distinguish different classes.
Statistical analysis
T-test, Cohen’s D and two-sample Kolmogorov-Smirnov test are introduced to explore how the mean values and distributions of personality facets between entrepreneurs and employees differ. The T-test is applied to determine whether the mean of personality facets of two group samples are significantly different from one another or not. The facets with significant differences detected by the hypothesis testing are critical to separate the two groups. Cohen’s d is to measure the effect size of the results of the previous t-test, which is the ratio of the mean difference to the pooled standard deviation. A larger Cohen’s d score indicates that the mean difference is greater than the variability of the whole sample. Moreover, it is interesting to check whether the two groups’ personality facets’ probability distributions are from the same distribution through the two-sample Kolmogorov-Smirnov test. There is no assumption about the distributions, but the test is sensitive to deviations near the centre rather than the tail.
Privacy and ethics
The focus of this research is to provide high-level insights about groups of startups, founders and types of founder teams rather than on specific individuals or companies. While we used unit record data from the publicly available data of company profiles from Crunchbase , we removed all identifiers from the underlying data on individual companies and founders and generated aggregate results, which formed the basis for our analysis and conclusions.
Data availability
A dataset which includes only aggregated statistics about the success of startups and the factors that influence is released as part of this research. Underlying data for all figures and the code to reproduce them are available on GitHub: https://github.com/Braesemann/FounderPersonalities . Please contact Fabian Braesemann ( [email protected] ) in case you have any further questions.
Change history
07 may 2024.
A Correction to this paper has been published: https://doi.org/10.1038/s41598-024-61082-7
Henrekson, M. & Johansson, D. Gazelles as job creators: A survey and interpretation of the evidence. Small Bus. Econ. 35 , 227–244 (2010).
Article Google Scholar
Davila, A., Foster, G., He, X. & Shimizu, C. The rise and fall of startups: Creation and destruction of revenue and jobs by young companies. Aust. J. Manag. 40 , 6–35 (2015).
Which vaccine saved the most lives in 2021?: Covid-19. The Economist (Online) (2022). noteName - AstraZeneca; Pfizer Inc; BioNTech SE; Copyright - Copyright The Economist Newspaper NA, Inc. Jul 14, 2022; Last updated - 2022-11-29.
Oltermann, P. Pfizer/biontech tax windfall brings mainz an early christmas present (2021). noteName - Pfizer Inc; BioNTech SE; Copyright - Copyright Guardian News & Media Limited Dec 27, 2021; Last updated - 2021-12-28.
Grant, K. A., Croteau, M. & Aziz, O. The survival rate of startups funded by angel investors. I-INC WHITE PAPER SER.: MAR 2019 , 1–21 (2019).
Google Scholar
Top 20 reasons start-ups fail - cb insights version (2019). noteCopyright - Copyright Newstex Oct 21, 2019; Last updated - 2022-10-25.
Hochberg, Y. V., Ljungqvist, A. & Lu, Y. Whom you know matters: Venture capital networks and investment performance. J. Financ. 62 , 251–301 (2007).
Fracassi, C., Garmaise, M. J., Kogan, S. & Natividad, G. Business microloans for us subprime borrowers. J. Financ. Quantitative Ana. 51 , 55–83 (2016).
Davila, A., Foster, G. & Gupta, M. Venture capital financing and the growth of startup firms. J. Bus. Ventur. 18 , 689–708 (2003).
Nann, S. et al. Comparing the structure of virtual entrepreneur networks with business effectiveness. Proc. Soc. Behav. Sci. 2 , 6483–6496 (2010).
Guzman, J. & Stern, S. Where is silicon valley?. Science 347 , 606–609 (2015).
Article ADS CAS PubMed Google Scholar
Aldrich, H. E. & Wiedenmayer, G. From traits to rates: An ecological perspective on organizational foundings. 61–97 (2019).
Gartner, W. B. Who is an entrepreneur? is the wrong question. Am. J. Small Bus. 12 , 11–32 (1988).
Thornton, P. H. The sociology of entrepreneurship. Ann. Rev. Sociol. 25 , 19–46 (1999).
Eikelboom, M. E., Gelderman, C. & Semeijn, J. Sustainable innovation in public procurement: The decisive role of the individual. J. Public Procure. 18 , 190–201 (2018).
Kerr, S. P. et al. Personality traits of entrepreneurs: A review of recent literature. Found. Trends Entrep. 14 , 279–356 (2018).
Hamilton, B. H., Papageorge, N. W. & Pande, N. The right stuff? Personality and entrepreneurship. Quant. Econ. 10 , 643–691 (2019).
Salmony, F. U. & Kanbach, D. K. Personality trait differences across types of entrepreneurs: A systematic literature review. RMS 16 , 713–749 (2022).
Freiberg, B. & Matz, S. C. Founder personality and entrepreneurial outcomes: A large-scale field study of technology startups. Proc. Natl. Acad. Sci. 120 , e2215829120 (2023).
Article CAS PubMed PubMed Central Google Scholar
Kern, M. L., McCarthy, P. X., Chakrabarty, D. & Rizoiu, M.-A. Social media-predicted personality traits and values can help match people to their ideal jobs. Proc. Natl. Acad. Sci. 116 , 26459–26464 (2019).
Article ADS CAS PubMed PubMed Central Google Scholar
Dalle, J.-M., Den Besten, M. & Menon, C. Using crunchbase for economic and managerial research. (2017).
Block, J. & Sandner, P. What is the effect of the financial crisis on venture capital financing? Empirical evidence from us internet start-ups. Ventur. Cap. 11 , 295–309 (2009).
Antretter, T., Blohm, I. & Grichnik, D. Predicting startup survival from digital traces: Towards a procedure for early stage investors (2018).
Dworak, D. Analysis of founder background as a predictor for start-up success in achieving successive fundraising rounds. (2022).
Hsu, D. H. Venture capitalists and cooperative start-up commercialization strategy. Manage. Sci. 52 , 204–219 (2006).
Blank, S. Why the lean start-up changes everything (2018).
Kaplan, S. N. & Lerner, J. It ain’t broke: The past, present, and future of venture capital. J. Appl. Corp. Financ. 22 , 36–47 (2010).
Hallen, B. L. & Eisenhardt, K. M. Catalyzing strategies and efficient tie formation: How entrepreneurial firms obtain investment ties. Acad. Manag. J. 55 , 35–70 (2012).
Gompers, P. A. & Lerner, J. The Venture Capital Cycle (MIT Press, 2004).
Shane, S. & Venkataraman, S. The promise of entrepreneurship as a field of research. Acad. Manag. Rev. 25 , 217–226 (2000).
Zahra, S. A. & Wright, M. Understanding the social role of entrepreneurship. J. Manage. Stud. 53 , 610–629 (2016).
Bonaventura, M. et al. Predicting success in the worldwide start-up network. Sci. Rep. 10 , 1–6 (2020).
Schwartz, H. A. et al. Personality, gender, and age in the language of social media: The open-vocabulary approach. PLoS ONE 8 , e73791 (2013).
Plank, B. & Hovy, D. Personality traits on twitter-or-how to get 1,500 personality tests in a week. In Proceedings of the 6th workshop on computational approaches to subjectivity, sentiment and social media analysis , pp 92–98 (2015).
Arnoux, P.-H. et al. 25 tweets to know you: A new model to predict personality with social media. In booktitleEleventh international AAAI conference on web and social media (2017).
Roberts, B. W., Kuncel, N. R., Shiner, R., Caspi, A. & Goldberg, L. R. The power of personality: The comparative validity of personality traits, socioeconomic status, and cognitive ability for predicting important life outcomes. Perspect. Psychol. Sci. 2 , 313–345 (2007).
Article PubMed PubMed Central Google Scholar
Youyou, W., Kosinski, M. & Stillwell, D. Computer-based personality judgments are more accurate than those made by humans. Proc. Natl. Acad. Sci. 112 , 1036–1040 (2015).
Soldz, S. & Vaillant, G. E. The big five personality traits and the life course: A 45-year longitudinal study. J. Res. Pers. 33 , 208–232 (1999).
Damian, R. I., Spengler, M., Sutu, A. & Roberts, B. W. Sixteen going on sixty-six: A longitudinal study of personality stability and change across 50 years. J. Pers. Soc. Psychol. 117 , 674 (2019).
Article PubMed Google Scholar
Rantanen, J., Metsäpelto, R.-L., Feldt, T., Pulkkinen, L. & Kokko, K. Long-term stability in the big five personality traits in adulthood. Scand. J. Psychol. 48 , 511–518 (2007).
Roberts, B. W., Caspi, A. & Moffitt, T. E. The kids are alright: Growth and stability in personality development from adolescence to adulthood. J. Pers. Soc. Psychol. 81 , 670 (2001).
Article CAS PubMed Google Scholar
Cobb-Clark, D. A. & Schurer, S. The stability of big-five personality traits. Econ. Lett. 115 , 11–15 (2012).
Graham, P. Do Things that Don’t Scale (Paul Graham, 2013).
McCarthy, P. X., Kern, M. L., Gong, X., Parker, M. & Rizoiu, M.-A. Occupation-personality fit is associated with higher employee engagement and happiness. (2022).
Pratt, A. C. Advertising and creativity, a governance approach: A case study of creative agencies in London. Environ. Plan A 38 , 1883–1899 (2006).
Klotz, A. C., Hmieleski, K. M., Bradley, B. H. & Busenitz, L. W. New venture teams: A review of the literature and roadmap for future research. J. Manag. 40 , 226–255 (2014).
Duggan, M., Ellison, N. B., Lampe, C., Lenhart, A. & Madden, M. Demographics of key social networking platforms. Pew Res. Center 9 (2015).
Fisch, C. & Block, J. H. How does entrepreneurial failure change an entrepreneur’s digital identity? Evidence from twitter data. J. Bus. Ventur. 36 , 106015 (2021).
Brush, C., Edelman, L. F., Manolova, T. & Welter, F. A gendered look at entrepreneurship ecosystems. Small Bus. Econ. 53 , 393–408 (2019).
Kanze, D., Huang, L., Conley, M. A. & Higgins, E. T. We ask men to win and women not to lose: Closing the gender gap in startup funding. Acad. Manag. J. 61 , 586–614 (2018).
Fan, J. S. Startup biases. UC Davis Law Review (2022).
AlShebli, B. K., Rahwan, T. & Woon, W. L. The preeminence of ethnic diversity in scientific collaboration. Nat. Commun. 9 , 1–10 (2018).
Article CAS Google Scholar
Żbikowski, K. & Antosiuk, P. A machine learning, bias-free approach for predicting business success using crunchbase data. Inf. Process. Manag. 58 , 102555 (2021).
Corea, F., Bertinetti, G. & Cervellati, E. M. Hacking the venture industry: An early-stage startups investment framework for data-driven investors. Mach. Learn. Appl. 5 , 100062 (2021).
Chapman, G. & Hottenrott, H. Founder personality and start-up subsidies. Founder Personality and Start-up Subsidies (2021).
Antoncic, B., Bratkovicregar, T., Singh, G. & DeNoble, A. F. The big five personality-entrepreneurship relationship: Evidence from slovenia. J. Small Bus. Manage. 53 , 819–841 (2015).
Download references
Acknowledgements
We thank Gary Brewer from BuiltWith ; Leni Mayo from Influx , Rachel Slattery from TeamSlatts and Daniel Petre from AirTree Ventures for their ongoing generosity and insights about startups, founders and venture investments. We also thank Tim Li from Crunchbase for advice and liaison regarding data on startups and Richard Slatter for advice and referrals in Twitter .
Author information
Authors and affiliations.
The Data Science Institute, University of Technology Sydney, Sydney, NSW, Australia
Paul X. McCarthy
School of Computer Science and Engineering, UNSW Sydney, Sydney, NSW, Australia
Faculty of Engineering and Information Technology, University of Technology Sydney, Sydney, Australia
Xian Gong & Marian-Andrei Rizoiu
Oxford Internet Institute, University of Oxford, Oxford, UK
Fabian Braesemann & Fabian Stephany
DWG Datenwissenschaftliche Gesellschaft Berlin, Berlin, Germany
Melbourne Graduate School of Education, The University of Melbourne, Parkville, VIC, Australia
Margaret L. Kern
You can also search for this author in PubMed Google Scholar
Contributions
All authors designed research; All authors analysed data and undertook investigation; F.B. and F.S. led multi-factor analysis; P.M., X.G. and M.A.R. led the founder/employee prediction; M.L.K. led personality insights; X.G. collected and tabulated the data; X.G., F.B., and F.S. created figures; X.G. created final art, and all authors wrote the paper.
Corresponding author
Correspondence to Fabian Braesemann .
Ethics declarations
Competing interests.
The authors declare no competing interests.
Additional information
Publisher's note.
Springer Nature remains neutral with regard to jurisdictional claims in published maps and institutional affiliations.
The original online version of this Article was revised: The Data Availability section in the original version of this Article was incomplete, the link to the GitHub repository was omitted. Full information regarding the corrections made can be found in the correction for this Article.
Supplementary Information
Supplementary information., rights and permissions.
Open Access This article is licensed under a Creative Commons Attribution 4.0 International License, which permits use, sharing, adaptation, distribution and reproduction in any medium or format, as long as you give appropriate credit to the original author(s) and the source, provide a link to the Creative Commons licence, and indicate if changes were made. The images or other third party material in this article are included in the article's Creative Commons licence, unless indicated otherwise in a credit line to the material. If material is not included in the article's Creative Commons licence and your intended use is not permitted by statutory regulation or exceeds the permitted use, you will need to obtain permission directly from the copyright holder. To view a copy of this licence, visit http://creativecommons.org/licenses/by/4.0/ .
Reprints and permissions
About this article
Cite this article.
McCarthy, P.X., Gong, X., Braesemann, F. et al. The impact of founder personalities on startup success. Sci Rep 13 , 17200 (2023). https://doi.org/10.1038/s41598-023-41980-y
Download citation
Received : 15 February 2023
Accepted : 04 September 2023
Published : 17 October 2023
DOI : https://doi.org/10.1038/s41598-023-41980-y
Share this article
Anyone you share the following link with will be able to read this content:
Sorry, a shareable link is not currently available for this article.
Provided by the Springer Nature SharedIt content-sharing initiative
By submitting a comment you agree to abide by our Terms and Community Guidelines . If you find something abusive or that does not comply with our terms or guidelines please flag it as inappropriate.
Quick links
- Explore articles by subject
- Guide to authors
- Editorial policies
Sign up for the Nature Briefing: AI and Robotics newsletter — what matters in AI and robotics research, free to your inbox weekly.

Climate Change to Have Long-Term Economic Impact: SF Fed Paper
A pedestrian walks near the branch of Federal Reserve Bank of San Francisco, in Salt Lake City, Utah, U.S., April 7, 2023. REUTERS/Ann Saphir/ File Photo
SAN FRANCISCO (Reuters) - An increase in the number of hot days as climate change warms the globe would likely damage the U.S. economy over the long-term, according to research published on Tuesday by the Federal Reserve Bank of San Francisco.
"Our findings suggest that, under a scenario with no large-scale efforts to reduce carbon emissions, future increases in extreme heat would reduce the capital stock by 5.4% and annual consumption by 1.8% by the year 2200," wrote Stephie Fried, a senior economist at the San Francisco Fed, and co-authors Gregory Casey and Matthew Gibson, both professors at Williams College.
The researchers used scientists' best estimates for the number of days per year where working outdoors would cause heat stress, estimated to rise from 22 days in 2020 to 80 in 2100.
They then projected the likely drain on labor productivity in construction, where - unlike most of the services and manufacturing sectors - air-conditioning cannot counter the impact of hot days.
They focused on construction because it makes up a larger share of overall economic output and U.S. investment than other sectors like agriculture or mining where workers are also vulnerable to heat.
"Decreases in construction productivity slow capital accumulation and therefore have long-lasting effects on macroeconomic outcomes," they wrote.
Using a less-likely alternative scenario under which the number of extreme-heat days rises to 125 in 2100, the authors found much larger consequences from a decline in construction productivity, with capital accumulation projected to fall by 18% and consumption by 7% in 2200.
(Reporting by Ann Saphir; Editing by Andrea Ricci)
Copyright 2024 Thomson Reuters .
Tags: environment , United States , pollution
The Best Financial Tools for You
Credit Cards

Personal Loans

Comparative assessments and other editorial opinions are those of U.S. News and have not been previously reviewed, approved or endorsed by any other entities, such as banks, credit card issuers or travel companies. The content on this page is accurate as of the posting date; however, some of our partner offers may have expired.

Subscribe to our daily newsletter to get investing advice, rankings and stock market news.
See a newsletter example .
You May Also Like
7 best high-dividend etfs for income.
Glenn Fydenkevez May 29, 2024

7 Top Women Investors
Coryanne Hicks May 29, 2024

7 Best Mid Cap Stocks to Buy Now
Jeff Reeves May 29, 2024

Best Vanguard Funds for Beginners
Tony Dong May 28, 2024

10 of the Best Stocks to Buy for 2024
John Divine May 28, 2024

9 Best Cheap Stocks to Buy Under $10
Wayne Duggan May 28, 2024

8 Stocks Warren Buffett Bought & Sold
Jeff Reeves May 28, 2024

Top-Rated ETFs to Buy Now
Marc Guberti May 28, 2024

7 Best Long-Term Stocks to Buy
Glenn Fydenkevez May 24, 2024

What Is Par Value for Stocks and Bonds?
Coryanne Hicks May 24, 2024

11 Top Sector ETFs to Buy
Jeff Reeves May 24, 2024

Stock Market Holidays in 2024
Daniel J. Lee May 24, 2024

George Soros' 6 Top Stock Picks
Brian O'Connell May 23, 2024

5 Best Undervalued Blue-Chip Stocks
Glenn Fydenkevez May 23, 2024

15 Best Dividend Stocks to Buy for 2024
Ian Bezek May 23, 2024

7 Best Semiconductor ETFs to Buy in 2024
Tony Dong May 23, 2024

Best Investments for 2024
Kate Stalter May 23, 2024

7 Best Cryptocurrency Exchanges
Jeff Reeves May 22, 2024

6 Best Biotech Stocks to Buy for 2024
Wayne Duggan May 22, 2024

Bill Gates Portfolio: 7 Best Stocks
Brian O'Connell May 22, 2024

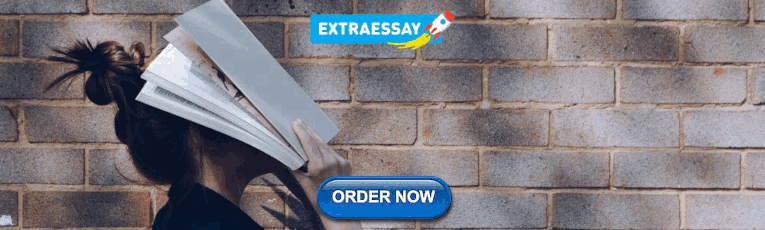
IMAGES
VIDEO
COMMENTS
The impact score (IS), also denoted as Journal impact score (JIS), of an academic journal is a measure of the yearly average number of citations to recent articles published in that journal. It is based on Scopus data. Impact Score is defined as the ratio of the number of citations a journal receives in the latest two years (Including the year ...
How. Follow these steps to find the Impact Factor of a journal: Go to the journal's homepage. When you're on the journal homepage, scroll down the page until you see the 'Journal Metrics' section on the left hand side. The journal Impact Factor is located under the 'Journal Metrics' section. For more information on journal metrics, please visit ...
Journal Impact Factor (JIF) Journal Impact Factor (JIF) is calculated by Clarivate Analytics as the average of the sum of the citations received in a given year to a journal's previous two years of publications (linked to the journal, but not necessarily to specific publications) divided by the sum of "citable" publications in the previous two years.
Find out more about Altmetrics and tools for obtaining altmetrics data, click on the Other Metrics/ Altmetrics tab. Impact Factor: The impact factor is a measure of the frequency in which the average article in a journal is cited in a particular year. Impact factors measure the impact of a journal, not the impact of individual articles.
Google Scholar Metrics provide an easy way for authors to quickly gauge the visibility and influence of recent articles in scholarly publications. Scholar Metrics summarize recent citations to many publications, to help authors as they consider where to publish their new research. To get started, you can browse the top 100 publications in ...
How. Follow these steps to find the Impact Factor of a journal: Search for a journal using the 'Journal/book title' field on the ScienceDirect homepage or browse journal titles by selecting ' Journals & Books ' in the top right corner. Click the journal title to navigate to the journal's home page. The Impact Factor and Journal CiteScore ...
Impact factors are used to measure the importance of a journal by calculating the number of times selected articles are cited within the last few years. The higher the impact factor, the more highly ranked the journal. It is one tool you can use to compare journals in a subject category. Impact Factors for scientific journals can be found in ...
The journal impact factor (JIF) is a measure of the frequency with which the average article in a journal has been cited in a particular year. It is used to measure the importance or rank of a journal by calculating the times its articles are cited. Clarivate Analytics is the creator and only provide of the journal impact factor.
The JCR provides quantitative tools for ranking, evaluating, categorizing, and comparing journals. The impact factor is one of these; it is a measure of the frequency with which the "average article" in a journal has been cited in a particular year or period. The annual JCR impact factor is a ratio between citations and recent citable items ...
They are used to evaluate the quality of a journal, as well as to determine the influence of your research in your field. In this series of free modules, we walk you through some of the key players in metrics. Measure article, author and journal influences using CiteScore metrics, h-index, article-level metrics, SNIP, SJR, impact factor and more.
The impact factor measures the number of times a journal article has been cited by researchers in a given year.It's used to measure the importance of a scholarly journal -- that is, its importance to the discipline or field its articles cover, and by extension, the researchers working in that discipline or field -- by measuring the number of times articles in that journal are cited.
The impact factor (IF) or journal impact factor (JIF) of an academic journal is a scientometric index calculated by Clarivate that reflects the yearly mean number of citations of articles published in the last two years in a given journal, as indexed by Clarivate's Web of Science.. As a journal-level metric, it is frequently used as a proxy for the relative importance of a journal within its ...
Jul 14, 2022 45398. You can look up the impact factor of a journal through the Journal Citation Reports (JCR) database. A journal's impact factor is a measure of how often the average article in a journal has been cited in a particular year. The impact factor will help you evaluate a journal's relative importance, especially when you compare it ...
This online guide will help you identify common research metrics that are used to measure scholarly impact. This guide also outlines methods and tools you can use to identify journals in your field for publishing. This page explains what journal impact factor is, the differences between different impact factors, and the resources you can use to find impact factor.
The impact factor (IF) is a measure of the frequency with which the average article in a journal has been cited in a particular year. It is used to measure the importance or rank of a journal by calculating the times its articles are cited. ... Reliability of journal impact factor rankings. BMC Medical Research Methodology, 7(48), 48. Howard, J ...
Find Journal Bibliometrics and Impact Factors. Journal Impact Factor (Journal Citation Reports, by Clarivate Analytics) If a journal title is not found in Journal Citation Reports, see if it is included in the Web of Science Master List. If not, the journal is unlikely to have a Journal Impact Factor since JCR includes only titles from the ...
SNIP - or Source Normalized Impact per Paper, is a sophisticated metric that accounts for field-specific differences in citation practices. JIF - or Journal Impact Factor is calculated by Clarivate Analytics as the average of the sum of the citations received in a given year to a journal's previous two years of publications, divided by ...
What is a good impact factor for a research journal. In the sciences, where citations are more rapid and frequent than probably in other fields, a journal with a JIF near 350 will receive an exceptionally high number of citations. In many fields of study, impact factors of 10 or above are considered exceptional, and in some, it is above 3.
Browse, search, and explore journals indexed in the Web of Science. The Master Journal List is an invaluable tool to help you to find the right journal for your needs across multiple indices hosted on the Web of Science platform. Spanning all disciplines and regions, Web of Science Core Collection is at the heart of the Web of Science platform. Curated with care by an expert team of in-house ...
<link rel="stylesheet" href="/public/styles.29276a7c2f2290b7.css">
Therefore, there is no set impact factor number considered to be ideal since each field has a different measurement. In general, an impact factor of 10 or higher is considered remarkable, while 3 is good, and the average score is less than 1. Example. The very prestigious journal Nature had an impact factor of 69.504 in the year 2021.
Find the right journal for your research. Looking for the best journal match for your paper? Search the world's leading source of academic journals using your abstract or your keywords and other details. More on how it works. Match my abstract Search by keywords, aims & scope, ...
International Scientific Journal & Country Ranking. SCImago Institutions Rankings SCImago Media Rankings SCImago Iber SCImago Research Centers Ranking SCImago Graphica Ediciones Profesionales de la Información
Abstract. The integration of Environmental, Social, and Governance (ESG) factors into investment decision-making has gained significant traction in recent years, reflecting a growing recognition ...
Here, we show that founder personality traits are a significant feature of a firm's ultimate success. We draw upon detailed data about the success of a large-scale global sample of startups (n ...
Abstract: This research investigates the relationship between entrepreneurship and work-life balance, focusing on challenges, coping strategies, and their impact on well-being. A quantitative approach was adopted, utilizing a structured questionnaire administered to entrepreneurs and self-employed individuals across diverse industries. Convenience sampling was employed to recruit participants ...
This paper presents an association mining approach to identify the influential factors which result in a high volume of air pollution concentration, in particular, the particulate matter with aerodynamic diameter ≤ 2.5 μm (PM2.5). ... Feature papers represent the most advanced research with significant potential for high impact in the field ...
Download the 2024 Gen Z and Millennial Report. 5 MB PDF. To learn more about the mental health findings, read the Mental Health Deep Dive. The 13th edition of Deloitte's Gen Z and Millennial Survey connected with nearly 23,000 respondents across 44 countries to track their experiences and expectations at work and in the world more broadly.
The objective of this study was to examine the potential moderating effects of the relationship between macroeconomic variables and the financial liquidity of enterprises. Given the significance of liquidity for companies and the profound impact of the macroeconomic environment, a research gap was identified in relation to the limited number of studies investigating the influence of ...
REUTERS/Ann Saphir/ File Photo. SAN FRANCISCO (Reuters) - An increase in the number of hot days as climate change warms the globe would likely damage the U.S. economy over the long-term, according ...