Have a language expert improve your writing
Run a free plagiarism check in 10 minutes, automatically generate references for free.
- Knowledge Base
- Methodology
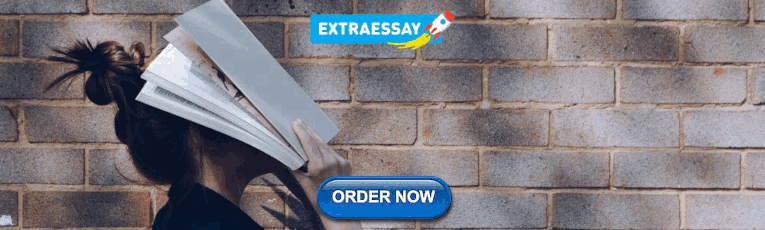
Research Design | Step-by-Step Guide with Examples
Published on 5 May 2022 by Shona McCombes . Revised on 20 March 2023.
A research design is a strategy for answering your research question using empirical data. Creating a research design means making decisions about:
- Your overall aims and approach
- The type of research design you’ll use
- Your sampling methods or criteria for selecting subjects
- Your data collection methods
- The procedures you’ll follow to collect data
- Your data analysis methods
A well-planned research design helps ensure that your methods match your research aims and that you use the right kind of analysis for your data.
Table of contents
Step 1: consider your aims and approach, step 2: choose a type of research design, step 3: identify your population and sampling method, step 4: choose your data collection methods, step 5: plan your data collection procedures, step 6: decide on your data analysis strategies, frequently asked questions.
- Introduction
Before you can start designing your research, you should already have a clear idea of the research question you want to investigate.
There are many different ways you could go about answering this question. Your research design choices should be driven by your aims and priorities – start by thinking carefully about what you want to achieve.
The first choice you need to make is whether you’ll take a qualitative or quantitative approach.
Qualitative research designs tend to be more flexible and inductive , allowing you to adjust your approach based on what you find throughout the research process.
Quantitative research designs tend to be more fixed and deductive , with variables and hypotheses clearly defined in advance of data collection.
It’s also possible to use a mixed methods design that integrates aspects of both approaches. By combining qualitative and quantitative insights, you can gain a more complete picture of the problem you’re studying and strengthen the credibility of your conclusions.
Practical and ethical considerations when designing research
As well as scientific considerations, you need to think practically when designing your research. If your research involves people or animals, you also need to consider research ethics .
- How much time do you have to collect data and write up the research?
- Will you be able to gain access to the data you need (e.g., by travelling to a specific location or contacting specific people)?
- Do you have the necessary research skills (e.g., statistical analysis or interview techniques)?
- Will you need ethical approval ?
At each stage of the research design process, make sure that your choices are practically feasible.
Prevent plagiarism, run a free check.
Within both qualitative and quantitative approaches, there are several types of research design to choose from. Each type provides a framework for the overall shape of your research.
Types of quantitative research designs
Quantitative designs can be split into four main types. Experimental and quasi-experimental designs allow you to test cause-and-effect relationships, while descriptive and correlational designs allow you to measure variables and describe relationships between them.
With descriptive and correlational designs, you can get a clear picture of characteristics, trends, and relationships as they exist in the real world. However, you can’t draw conclusions about cause and effect (because correlation doesn’t imply causation ).
Experiments are the strongest way to test cause-and-effect relationships without the risk of other variables influencing the results. However, their controlled conditions may not always reflect how things work in the real world. They’re often also more difficult and expensive to implement.
Types of qualitative research designs
Qualitative designs are less strictly defined. This approach is about gaining a rich, detailed understanding of a specific context or phenomenon, and you can often be more creative and flexible in designing your research.
The table below shows some common types of qualitative design. They often have similar approaches in terms of data collection, but focus on different aspects when analysing the data.
Your research design should clearly define who or what your research will focus on, and how you’ll go about choosing your participants or subjects.
In research, a population is the entire group that you want to draw conclusions about, while a sample is the smaller group of individuals you’ll actually collect data from.
Defining the population
A population can be made up of anything you want to study – plants, animals, organisations, texts, countries, etc. In the social sciences, it most often refers to a group of people.
For example, will you focus on people from a specific demographic, region, or background? Are you interested in people with a certain job or medical condition, or users of a particular product?
The more precisely you define your population, the easier it will be to gather a representative sample.
Sampling methods
Even with a narrowly defined population, it’s rarely possible to collect data from every individual. Instead, you’ll collect data from a sample.
To select a sample, there are two main approaches: probability sampling and non-probability sampling . The sampling method you use affects how confidently you can generalise your results to the population as a whole.
Probability sampling is the most statistically valid option, but it’s often difficult to achieve unless you’re dealing with a very small and accessible population.
For practical reasons, many studies use non-probability sampling, but it’s important to be aware of the limitations and carefully consider potential biases. You should always make an effort to gather a sample that’s as representative as possible of the population.
Case selection in qualitative research
In some types of qualitative designs, sampling may not be relevant.
For example, in an ethnography or a case study, your aim is to deeply understand a specific context, not to generalise to a population. Instead of sampling, you may simply aim to collect as much data as possible about the context you are studying.
In these types of design, you still have to carefully consider your choice of case or community. You should have a clear rationale for why this particular case is suitable for answering your research question.
For example, you might choose a case study that reveals an unusual or neglected aspect of your research problem, or you might choose several very similar or very different cases in order to compare them.
Data collection methods are ways of directly measuring variables and gathering information. They allow you to gain first-hand knowledge and original insights into your research problem.
You can choose just one data collection method, or use several methods in the same study.
Survey methods
Surveys allow you to collect data about opinions, behaviours, experiences, and characteristics by asking people directly. There are two main survey methods to choose from: questionnaires and interviews.
Observation methods
Observations allow you to collect data unobtrusively, observing characteristics, behaviours, or social interactions without relying on self-reporting.
Observations may be conducted in real time, taking notes as you observe, or you might make audiovisual recordings for later analysis. They can be qualitative or quantitative.
Other methods of data collection
There are many other ways you might collect data depending on your field and topic.
If you’re not sure which methods will work best for your research design, try reading some papers in your field to see what data collection methods they used.
Secondary data
If you don’t have the time or resources to collect data from the population you’re interested in, you can also choose to use secondary data that other researchers already collected – for example, datasets from government surveys or previous studies on your topic.
With this raw data, you can do your own analysis to answer new research questions that weren’t addressed by the original study.
Using secondary data can expand the scope of your research, as you may be able to access much larger and more varied samples than you could collect yourself.
However, it also means you don’t have any control over which variables to measure or how to measure them, so the conclusions you can draw may be limited.
As well as deciding on your methods, you need to plan exactly how you’ll use these methods to collect data that’s consistent, accurate, and unbiased.
Planning systematic procedures is especially important in quantitative research, where you need to precisely define your variables and ensure your measurements are reliable and valid.
Operationalisation
Some variables, like height or age, are easily measured. But often you’ll be dealing with more abstract concepts, like satisfaction, anxiety, or competence. Operationalisation means turning these fuzzy ideas into measurable indicators.
If you’re using observations , which events or actions will you count?
If you’re using surveys , which questions will you ask and what range of responses will be offered?
You may also choose to use or adapt existing materials designed to measure the concept you’re interested in – for example, questionnaires or inventories whose reliability and validity has already been established.
Reliability and validity
Reliability means your results can be consistently reproduced , while validity means that you’re actually measuring the concept you’re interested in.
For valid and reliable results, your measurement materials should be thoroughly researched and carefully designed. Plan your procedures to make sure you carry out the same steps in the same way for each participant.
If you’re developing a new questionnaire or other instrument to measure a specific concept, running a pilot study allows you to check its validity and reliability in advance.
Sampling procedures
As well as choosing an appropriate sampling method, you need a concrete plan for how you’ll actually contact and recruit your selected sample.
That means making decisions about things like:
- How many participants do you need for an adequate sample size?
- What inclusion and exclusion criteria will you use to identify eligible participants?
- How will you contact your sample – by mail, online, by phone, or in person?
If you’re using a probability sampling method, it’s important that everyone who is randomly selected actually participates in the study. How will you ensure a high response rate?
If you’re using a non-probability method, how will you avoid bias and ensure a representative sample?
Data management
It’s also important to create a data management plan for organising and storing your data.
Will you need to transcribe interviews or perform data entry for observations? You should anonymise and safeguard any sensitive data, and make sure it’s backed up regularly.
Keeping your data well organised will save time when it comes to analysing them. It can also help other researchers validate and add to your findings.
On their own, raw data can’t answer your research question. The last step of designing your research is planning how you’ll analyse the data.
Quantitative data analysis
In quantitative research, you’ll most likely use some form of statistical analysis . With statistics, you can summarise your sample data, make estimates, and test hypotheses.
Using descriptive statistics , you can summarise your sample data in terms of:
- The distribution of the data (e.g., the frequency of each score on a test)
- The central tendency of the data (e.g., the mean to describe the average score)
- The variability of the data (e.g., the standard deviation to describe how spread out the scores are)
The specific calculations you can do depend on the level of measurement of your variables.
Using inferential statistics , you can:
- Make estimates about the population based on your sample data.
- Test hypotheses about a relationship between variables.
Regression and correlation tests look for associations between two or more variables, while comparison tests (such as t tests and ANOVAs ) look for differences in the outcomes of different groups.
Your choice of statistical test depends on various aspects of your research design, including the types of variables you’re dealing with and the distribution of your data.
Qualitative data analysis
In qualitative research, your data will usually be very dense with information and ideas. Instead of summing it up in numbers, you’ll need to comb through the data in detail, interpret its meanings, identify patterns, and extract the parts that are most relevant to your research question.
Two of the most common approaches to doing this are thematic analysis and discourse analysis .
There are many other ways of analysing qualitative data depending on the aims of your research. To get a sense of potential approaches, try reading some qualitative research papers in your field.
A sample is a subset of individuals from a larger population. Sampling means selecting the group that you will actually collect data from in your research.
For example, if you are researching the opinions of students in your university, you could survey a sample of 100 students.
Statistical sampling allows you to test a hypothesis about the characteristics of a population. There are various sampling methods you can use to ensure that your sample is representative of the population as a whole.
Operationalisation means turning abstract conceptual ideas into measurable observations.
For example, the concept of social anxiety isn’t directly observable, but it can be operationally defined in terms of self-rating scores, behavioural avoidance of crowded places, or physical anxiety symptoms in social situations.
Before collecting data , it’s important to consider how you will operationalise the variables that you want to measure.
The research methods you use depend on the type of data you need to answer your research question .
- If you want to measure something or test a hypothesis , use quantitative methods . If you want to explore ideas, thoughts, and meanings, use qualitative methods .
- If you want to analyse a large amount of readily available data, use secondary data. If you want data specific to your purposes with control over how they are generated, collect primary data.
- If you want to establish cause-and-effect relationships between variables , use experimental methods. If you want to understand the characteristics of a research subject, use descriptive methods.
Cite this Scribbr article
If you want to cite this source, you can copy and paste the citation or click the ‘Cite this Scribbr article’ button to automatically add the citation to our free Reference Generator.
McCombes, S. (2023, March 20). Research Design | Step-by-Step Guide with Examples. Scribbr. Retrieved 27 May 2024, from https://www.scribbr.co.uk/research-methods/research-design/
Is this article helpful?
Shona McCombes

An official website of the United States government
The .gov means it’s official. Federal government websites often end in .gov or .mil. Before sharing sensitive information, make sure you’re on a federal government site.
The site is secure. The https:// ensures that you are connecting to the official website and that any information you provide is encrypted and transmitted securely.
- Publications
- Account settings
Preview improvements coming to the PMC website in October 2024. Learn More or Try it out now .
- Advanced Search
- Journal List
- Pediatr Investig
- v.3(4); 2019 Dec

Clinical research study designs: The essentials
Ambika g. chidambaram.
1 Children's Hospital of Philadelphia, Philadelphia Pennsylvania, USA
Maureen Josephson
In clinical research, our aim is to design a study which would be able to derive a valid and meaningful scientific conclusion using appropriate statistical methods. The conclusions derived from a research study can either improve health care or result in inadvertent harm to patients. Hence, this requires a well‐designed clinical research study that rests on a strong foundation of a detailed methodology and governed by ethical clinical principles. The purpose of this review is to provide the readers an overview of the basic study designs and its applicability in clinical research.
Introduction
In clinical research, our aim is to design a study, which would be able to derive a valid and meaningful scientific conclusion using appropriate statistical methods that can be translated to the “real world” setting. 1 Before choosing a study design, one must establish aims and objectives of the study, and choose an appropriate target population that is most representative of the population being studied. The conclusions derived from a research study can either improve health care or result in inadvertent harm to patients. Hence, this requires a well‐designed clinical research study that rests on a strong foundation of a detailed methodology and is governed by ethical principles. 2
From an epidemiological standpoint, there are two major types of clinical study designs, observational and experimental. 3 Observational studies are hypothesis‐generating studies, and they can be further divided into descriptive and analytic. Descriptive observational studies provide a description of the exposure and/or the outcome, and analytic observational studies provide a measurement of the association between the exposure and the outcome. Experimental studies, on the other hand, are hypothesis testing studies. It involves an intervention that tests the association between the exposure and outcome. Each study design is different, and so it would be important to choose a design that would most appropriately answer the question in mind and provide the most valuable information. We will be reviewing each study design in detail (Figure 1 ).

Overview of clinical research study designs
Observational study designs
Observational studies ask the following questions: what, who, where and when. There are many study designs that fall under the umbrella of descriptive study designs, and they include, case reports, case series, ecologic study, cross‐sectional study, cohort study and case‐control study (Figure 2 ).

Classification of observational study designs
Case reports and case series
Every now and then during clinical practice, we come across a case that is atypical or ‘out of the norm’ type of clinical presentation. This atypical presentation is usually described as case reports which provides a detailed and comprehensive description of the case. 4 It is one of the earliest forms of research and provides an opportunity for the investigator to describe the observations that make a case unique. There are no inferences obtained and therefore cannot be generalized to the population which is a limitation. Most often than not, a series of case reports make a case series which is an atypical presentation found in a group of patients. This in turn poses the question for a new disease entity and further queries the investigator to look into mechanistic investigative opportunities to further explore. However, in a case series, the cases are not compared to subjects without the manifestations and therefore it cannot determine which factors in the description are unique to the new disease entity.
Ecologic study
Ecological studies are observational studies that provide a description of population group characteristics. That is, it describes characteristics to all individuals within a group. For example, Prentice et al 5 measured incidence of breast cancer and per capita intake of dietary fat, and found a correlation that higher per capita intake of dietary fat was associated with an increased incidence of breast cancer. But the study does not conclude specifically which subjects with breast cancer had a higher dietary intake of fat. Thus, one of the limitations with ecologic study designs is that the characteristics are attributed to the whole group and so the individual characteristics are unknown.
Cross‐sectional study
Cross‐sectional studies are study designs used to evaluate an association between an exposure and outcome at the same time. It can be classified under either descriptive or analytic, and therefore depends on the question being answered by the investigator. Since, cross‐sectional studies are designed to collect information at the same point of time, this provides an opportunity to measure prevalence of the exposure or the outcome. For example, a cross‐sectional study design was adopted to estimate the global need for palliative care for children based on representative sample of countries from all regions of the world and all World Bank income groups. 6 The limitation of cross‐sectional study design is that temporal association cannot be established as the information is collected at the same point of time. If a study involves a questionnaire, then the investigator can ask questions to onset of symptoms or risk factors in relation to onset of disease. This would help in obtaining a temporal sequence between the exposure and outcome. 7
Case‐control study
Case‐control studies are study designs that compare two groups, such as the subjects with disease (cases) to the subjects without disease (controls), and to look for differences in risk factors. 8 This study is used to study risk factors or etiologies for a disease, especially if the disease is rare. Thus, case‐control studies can also be hypothesis testing studies and therefore can suggest a causal relationship but cannot prove. It is less expensive and less time‐consuming than cohort studies (described in section “Cohort study”). An example of a case‐control study was performed in Pakistan evaluating the risk factors for neonatal tetanus. They retrospectively reviewed a defined cohort for cases with and without neonatal tetanus. 9 They found a strong association of the application of ghee (clarified butter) as a risk factor for neonatal tetanus. Although this suggests a causal relationship, cause cannot be proven by this methodology (Figure 3 ).

Case‐control study design
One of the limitations of case‐control studies is that they cannot estimate prevalence of a disease accurately as a proportion of cases and controls are studied at a time. Case‐control studies are also prone to biases such as recall bias, as the subjects are providing information based on their memory. Hence, the subjects with disease are likely to remember the presence of risk factors compared to the subjects without disease.
One of the aspects that is often overlooked is the selection of cases and controls. It is important to select the cases and controls appropriately to obtain a meaningful and scientifically sound conclusion and this can be achieved by implementing matching. Matching is defined by Gordis et al as ‘the process of selecting the controls so that they are similar to the cases in certain characteristics such as age, race, sex, socioeconomic status and occupation’ 7 This would help identify risk factors or probable etiologies that are not due to differences between the cases and controls.
Cohort study
Cohort studies are study designs that compare two groups, such as the subjects with exposure/risk factor to the subjects without exposure/risk factor, for differences in incidence of outcome/disease. Most often, cohort study designs are used to study outcome(s) from a single exposure/risk factor. Thus, cohort studies can also be hypothesis testing studies and can infer and interpret a causal relationship between an exposure and a proposed outcome, but cannot establish it (Figure 4 ).

Cohort study design
Cohort studies can be classified as prospective and retrospective. 7 Prospective cohort studies follow subjects from presence of risk factors/exposure to development of disease/outcome. This could take up to years before development of disease/outcome, and therefore is time consuming and expensive. On the other hand, retrospective cohort studies identify a population with and without the risk factor/exposure based on past records and then assess if they had developed the disease/outcome at the time of study. Thus, the study design for prospective and retrospective cohort studies are similar as we are comparing populations with and without exposure/risk factor to development of outcome/disease.
Cohort studies are typically chosen as a study design when the suspected exposure is known and rare, and the incidence of disease/outcome in the exposure group is suspected to be high. The choice between prospective and retrospective cohort study design would depend on the accuracy and reliability of the past records regarding the exposure/risk factor.
Some of the biases observed with cohort studies include selection bias and information bias. Some individuals who have the exposure may refuse to participate in the study or would be lost to follow‐up, and in those instances, it becomes difficult to interpret the association between an exposure and outcome. Also, if the information is inaccurate when past records are used to evaluate for exposure status, then again, the association between the exposure and outcome becomes difficult to interpret.
Case‐control studies based within a defined cohort
Case‐control studies based within a defined cohort is a form of study design that combines some of the features of a cohort study design and a case‐control study design. When a defined cohort is embedded in a case‐control study design, all the baseline information collected before the onset of disease like interviews, surveys, blood or urine specimens, then the cohort is followed onset of disease. One of the advantages of following the above design is that it eliminates recall bias as the information regarding risk factors is collected before onset of disease. Case‐control studies based within a defined cohort can be further classified into two types: Nested case‐control study and Case‐cohort study.
Nested case‐control study
A nested case‐control study consists of defining a cohort with suspected risk factors and assigning a control within a cohort to the subject who develops the disease. 10 Over a period, cases and controls are identified and followed as per the investigator's protocol. Hence, the case and control are matched on calendar time and length of follow‐up. When this study design is implemented, it is possible for the control that was selected early in the study to develop the disease and become a case in the latter part of the study.
Case‐cohort Study
A case‐cohort study is similar to a nested case‐control study except that there is a defined sub‐cohort which forms the groups of individuals without the disease (control), and the cases are not matched on calendar time or length of follow‐up with the control. 11 With these modifications, it is possible to compare different disease groups with the same sub‐cohort group of controls and eliminates matching between the case and control. However, these differences will need to be accounted during analysis of results.
Experimental study design
The basic concept of experimental study design is to study the effect of an intervention. In this study design, the risk factor/exposure of interest/treatment is controlled by the investigator. Therefore, these are hypothesis testing studies and can provide the most convincing demonstration of evidence for causality. As a result, the design of the study requires meticulous planning and resources to provide an accurate result.
The experimental study design can be classified into 2 groups, that is, controlled (with comparison) and uncontrolled (without comparison). 1 In the group without controls, the outcome is directly attributed to the treatment received in one group. This fails to prove if the outcome was truly due to the intervention implemented or due to chance. This can be avoided if a controlled study design is chosen which includes a group that does not receive the intervention (control group) and a group that receives the intervention (intervention/experiment group), and therefore provide a more accurate and valid conclusion.
Experimental study designs can be divided into 3 broad categories: clinical trial, community trial, field trial. The specifics of each study design are explained below (Figure 5 ).

Experimental study designs
Clinical trial
Clinical trials are also known as therapeutic trials, which involve subjects with disease and are placed in different treatment groups. It is considered a gold standard approach for epidemiological research. One of the earliest clinical trial studies was performed by James Lind et al in 1747 on sailors with scurvy. 12 Lind divided twelve scorbutic sailors into six groups of two. Each group received the same diet, in addition to a quart of cider (group 1), twenty‐five drops of elixir of vitriol which is sulfuric acid (group 2), two spoonfuls of vinegar (group 3), half a pint of seawater (group 4), two oranges and one lemon (group 5), and a spicy paste plus a drink of barley water (group 6). The group who ate two oranges and one lemon had shown the most sudden and visible clinical effects and were taken back at the end of 6 days as being fit for duty. During Lind's time, this was not accepted but was shown to have similar results when repeated 47 years later in an entire fleet of ships. Based on the above results, in 1795 lemon juice was made a required part of the diet of sailors. Thus, clinical trials can be used to evaluate new therapies, such as new drug or new indication, new drug combination, new surgical procedure or device, new dosing schedule or mode of administration, or a new prevention therapy.
While designing a clinical trial, it is important to select the population that is best representative of the general population. Therefore, the results obtained from the study can be generalized to the population from which the sample population was selected. It is also as important to select appropriate endpoints while designing a trial. Endpoints need to be well‐defined, reproducible, clinically relevant and achievable. The types of endpoints include continuous, ordinal, rates and time‐to‐event, and it is typically classified as primary, secondary or tertiary. 2 An ideal endpoint is a purely clinical outcome, for example, cure/survival, and thus, the clinical trials will become very long and expensive trials. Therefore, surrogate endpoints are used that are biologically related to the ideal endpoint. Surrogate endpoints need to be reproducible, easily measured, related to the clinical outcome, affected by treatment and occurring earlier than clinical outcome. 2
Clinical trials are further divided into randomized clinical trial, non‐randomized clinical trial, cross‐over clinical trial and factorial clinical trial.
Randomized clinical trial
A randomized clinical trial is also known as parallel group randomized trials or randomized controlled trials. Randomized clinical trials involve randomizing subjects with similar characteristics to two groups (or multiple groups): the group that receives the intervention/experimental therapy and the other group that received the placebo (or standard of care). 13 This is typically performed by using a computer software, manually or by other methods. Hence, we can measure the outcomes and efficacy of the intervention/experimental therapy being studied without bias as subjects have been randomized to their respective groups with similar baseline characteristics. This type of study design is considered gold standard for epidemiological research. However, this study design is generally not applicable to rare and serious disease process as it would unethical to treat that group with a placebo. Please see section “Randomization” for detailed explanation regarding randomization and placebo.
Non‐randomized clinical trial
A non‐randomized clinical trial involves an approach to selecting controls without randomization. With this type of study design a pattern is usually adopted, such as, selection of subjects and controls on certain days of the week. Depending on the approach adopted, the selection of subjects becomes predictable and therefore, there is bias with regards to selection of subjects and controls that would question the validity of the results obtained.
Historically controlled studies can be considered as a subtype of non‐randomized clinical trial. In this study design subtype, the source of controls is usually adopted from the past, such as from medical records and published literature. 1 The advantages of this study design include being cost‐effective, time saving and easily accessible. However, since this design depends on already collected data from different sources, the information obtained may not be accurate, reliable, lack uniformity and/or completeness as well. Though historically controlled studies maybe easier to conduct, the disadvantages will need to be taken into account while designing a study.
Cross‐over clinical trial
In cross‐over clinical trial study design, there are two groups who undergoes the same intervention/experiment at different time periods of the study. That is, each group serves as a control while the other group is undergoing the intervention/experiment. 14 Depending on the intervention/experiment, a ‘washout’ period is recommended. This would help eliminate residuals effects of the intervention/experiment when the experiment group transitions to be the control group. Hence, the outcomes of the intervention/experiment will need to be reversible as this type of study design would not be possible if the subject is undergoing a surgical procedure.
Factorial trial
A factorial trial study design is adopted when the researcher wishes to test two different drugs with independent effects on the same population. Typically, the population is divided into 4 groups, the first with drug A, the second with drug B, the third with drug A and B, and the fourth with neither drug A nor drug B. The outcomes for drug A are compared to those on drug A, drug A and B and to those who were on drug B and neither drug A nor drug B. 15 The advantages of this study design that it saves time and helps to study two different drugs on the same study population at the same time. However, this study design would not be applicable if either of the drugs or interventions overlaps with each other on modes of action or effects, as the results obtained would not attribute to a particular drug or intervention.
Community trial
Community trials are also known as cluster‐randomized trials, involve groups of individuals with and without disease who are assigned to different intervention/experiment groups. Hence, groups of individuals from a certain area, such as a town or city, or a certain group such as school or college, will undergo the same intervention/experiment. 16 Hence, the results will be obtained at a larger scale; however, will not be able to account for inter‐individual and intra‐individual variability.
Field trial
Field trials are also known as preventive or prophylactic trials, and the subjects without the disease are placed in different preventive intervention groups. 16 One of the hypothetical examples for a field trial would be to randomly assign to groups of a healthy population and to provide an intervention to a group such as a vitamin and following through to measure certain outcomes. Hence, the subjects are monitored over a period of time for occurrence of a particular disease process.
Overview of methodologies used within a study design
Randomization.
Randomization is a well‐established methodology adopted in research to prevent bias due to subject selection, which may impact the result of the intervention/experiment being studied. It is one of the fundamental principles of an experimental study designs and ensures scientific validity. It provides a way to avoid predicting which subjects are assigned to a certain group and therefore, prevent bias on the final results due to subject selection. This also ensures comparability between groups as most baseline characteristics are similar prior to randomization and therefore helps to interpret the results regarding the intervention/experiment group without bias.
There are various ways to randomize and it can be as simple as a ‘flip of a coin’ to use computer software and statistical methods. To better describe randomization, there are three types of randomization: simple randomization, block randomization and stratified randomization.
Simple randomization
In simple randomization, the subjects are randomly allocated to experiment/intervention groups based on a constant probability. That is, if there are two groups A and B, the subject has a 0.5 probability of being allocated to either group. This can be performed in multiple ways, and one of which being as simple as a ‘flip of a coin’ to using random tables or numbers. 17 The advantage of using this methodology is that it eliminates selection bias. However, the disadvantage with this methodology is that an imbalance in the number allocated to each group as well as the prognostic factors between groups. Hence, it is more challenging in studies with a small sample size.
Block randomization
In block randomization, the subjects of similar characteristics are classified into blocks. The aim of block randomization is to balance the number of subjects allocated to each experiment/intervention group. For example, let's assume that there are four subjects in each block, and two of the four subjects in each block will be randomly allotted to each group. Therefore, there will be two subjects in one group and two subjects in the other group. 17 The disadvantage with this methodology is that there is still a component of predictability in the selection of subjects and the randomization of prognostic factors is not performed. However, it helps to control the balance between the experiment/intervention groups.
Stratified randomization
In stratified randomization, the subjects are defined based on certain strata, which are covariates. 18 For example, prognostic factors like age can be considered as a covariate, and then the specified population can be randomized within each age group related to an experiment/intervention group. The advantage with this methodology is that it enables comparability between experiment/intervention groups and thus makes result analysis more efficient. But, with this methodology the covariates will need to be measured and determined before the randomization process. The sample size will help determine the number of strata that would need to be chosen for a study.
Blinding is a methodology adopted in a study design to intentionally not provide information related to the allocation of the groups to the subject participants, investigators and/or data analysts. 19 The purpose of blinding is to decrease influence associated with the knowledge of being in a particular group on the study result. There are 3 forms of blinding: single‐blinded, double‐blinded and triple‐blinded. 1 In single‐blinded studies, otherwise called as open‐label studies, the subject participants are not revealed which group that they have been allocated to. However, the investigator and data analyst will be aware of the allocation of the groups. In double‐blinded studies, both the study participants and the investigator will be unaware of the group to which they were allocated to. Double‐blinded studies are typically used in clinical trials to test the safety and efficacy of the drugs. In triple‐blinded studies, the subject participants, investigators and data analysts will not be aware of the group allocation. Thus, triple‐blinded studies are more difficult and expensive to design but the results obtained will exclude confounding effects from knowledge of group allocation.
Blinding is especially important in studies where subjective response are considered as outcomes. This is because certain responses can be modified based on the knowledge of the experiment group that they are in. For example, a group allocated in the non‐intervention group may not feel better as they are not getting the treatment, or an investigator may pay more attention to the group receiving treatment, and thereby potentially affecting the final results. However, certain treatments cannot be blinded such as surgeries or if the treatment group requires an assessment of the effect of intervention such as quitting smoking.
Placebo is defined in the Merriam‐Webster dictionary as ‘an inert or innocuous substance used especially in controlled experiments testing the efficacy of another substance (such as drug)’. 20 A placebo is typically used in a clinical research study to evaluate the safety and efficacy of a drug/intervention. This is especially useful if the outcome measured is subjective. In clinical drug trials, a placebo is typically a drug that resembles the drug to be tested in certain characteristics such as color, size, shape and taste, but without the active substance. This helps to measure effects of just taking the drug, such as pain relief, compared to the drug with the active substance. If the effect is positive, for example, improvement in mood/pain, then it is called placebo effect. If the effect is negative, for example, worsening of mood/pain, then it is called nocebo effect. 21
The ethics of placebo‐controlled studies is complex and remains a debate in the medical research community. According to the Declaration of Helsinki on the use of placebo released in October 2013, “The benefits, risks, burdens and effectiveness of a new intervention must be tested against those of the best proven intervention(s), except in the following circumstances:
Where no proven intervention exists, the use of placebo, or no intervention, is acceptable; or
Where for compelling and scientifically sound methodological reasons the use of any intervention less effective than the best proven one, the use of placebo, or no intervention is necessary to determine the efficacy or safety of an intervention and the patients who receive any intervention less effective than the best proven one, placebo, or no intervention will not be subject to additional risks of serious or irreversible harm as a result of not receiving the best proven intervention.
Extreme care must be taken to avoid abuse of this option”. 22
Hence, while designing a research study, both the scientific validity and ethical aspects of the study will need to be thoroughly evaluated.
Bias has been defined as “any systematic error in the design, conduct or analysis of a study that results in a mistaken estimate of an exposure's effect on the risk of disease”. 23 There are multiple types of biases and so, in this review we will focus on the following types: selection bias, information bias and observer bias. Selection bias is when a systematic error is committed while selecting subjects for the study. Selection bias will affect the external validity of the study if the study subjects are not representative of the population being studied and therefore, the results of the study will not be generalizable. Selection bias will affect the internal validity of the study if the selection of study subjects in each group is influenced by certain factors, such as, based on the treatment of the group assigned. One of the ways to decrease selection bias is to select the study population that would representative of the population being studied, or to randomize (discussed in section “Randomization”).
Information bias is when a systematic error is committed while obtaining data from the study subjects. This can be in the form of recall bias when subject is required to remember certain events from the past. Typically, subjects with the disease tend to remember certain events compared to subjects without the disease. Observer bias is a systematic error when the study investigator is influenced by the certain characteristics of the group, that is, an investigator may pay closer attention to the group receiving the treatment versus the group not receiving the treatment. This may influence the results of the study. One of the ways to decrease observer bias is to use blinding (discussed in section “Blinding”).
Thus, while designing a study it is important to take measure to limit bias as much as possible so that the scientific validity of the study results is preserved to its maximum.
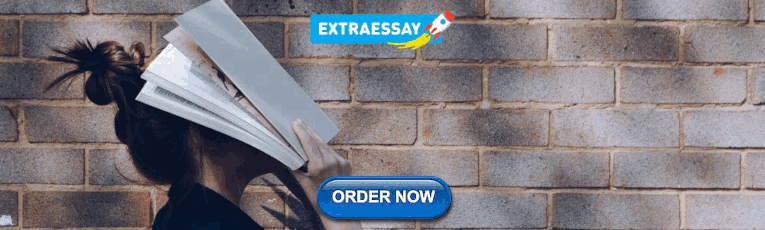
Overview of drug development in the United States of America
Now that we have reviewed the various clinical designs, clinical trials form a major part in development of a drug. In the United States, the Food and Drug Administration (FDA) plays an important role in getting a drug approved for clinical use. It includes a robust process that involves four different phases before a drug can be made available to the public. Phase I is conducted to determine a safe dose. The study subjects consist of normal volunteers and/or subjects with disease of interest, and the sample size is typically small and not more than 30 subjects. The primary endpoint consists of toxicity and adverse events. Phase II is conducted to evaluate of safety of dose selected in Phase I, to collect preliminary information on efficacy and to determine factors to plan a randomized controlled trial. The study subjects consist of subjects with disease of interest and the sample size is also small but more that Phase I (40–100 subjects). The primary endpoint is the measure of response. Phase III is conducted as a definitive trial to prove efficacy and establish safety of a drug. Phase III studies are randomized controlled trials and depending on the drug being studied, it can be placebo‐controlled, equivalence, superiority or non‐inferiority trials. The study subjects consist of subjects with disease of interest, and the sample size is typically large but no larger than 300 to 3000. Phase IV is performed after a drug is approved by the FDA and it is also called the post‐marketing clinical trial. This phase is conducted to evaluate new indications, to determine safety and efficacy in long‐term follow‐up and new dosing regimens. This phase helps to detect rare adverse events that would not be picked up during phase III studies and decrease in the delay in the release of the drug in the market. Hence, this phase depends heavily on voluntary reporting of side effects and/or adverse events by physicians, non‐physicians or drug companies. 2
We have discussed various clinical research study designs in this comprehensive review. Though there are various designs available, one must consider various ethical aspects of the study. Hence, each study will require thorough review of the protocol by the institutional review board before approval and implementation.
CONFLICT OF INTEREST
Chidambaram AG, Josephson M. Clinical research study designs: The essentials . Pediatr Invest . 2019; 3 :245‐252. 10.1002/ped4.12166 [ PMC free article ] [ PubMed ] [ CrossRef ] [ Google Scholar ]

Want to create or adapt books like this? Learn more about how Pressbooks supports open publishing practices.
2 Considerations in Designing Your Research Approach
Once you’ve identified your area of interest, sorted through and analyzed the literature to identify the problem you’d like to address, and developed both a purpose and a question; the next step is to design your study. This chapter will provide a basic overview of the considerations any researcher must think about as they design a research study.
Chapter 2: Learning Objectives
As you work to identify the best approach to identify an answer to your research question, you will be able to:
- Compare the conceptualization and operational activities of the process
- Discuss the difference between an independent and dependent variable
- Discuss the importance of sampling
- Contrast research approaches
- Demonstrate a systematic approach to selecting a research design
Understanding the Language of Research
As you work to determine which approach you will consider in order to best answer your question, you’ll need to consider how to address both the conceptual and operational components of your inquiry. As we discussed in Chapter 1; theory often informs practice (deductive approaches). Theory is often discussed in terms of abstract, or immeasurable, constructs. Because of the ambiguous nature of theory, it is important to conceptualize the parameters of your investigation. Conceptualizing is the process of defining what is or is not included in your description of a specific construct.
Understanding Theoretical and Contextual Framework
You may consider the theoretical or contextual framework for your study as the ‘lens’ through which you want your reader to view the work from. That is, this is your opportunity frame their experience with this information through your educated perspective on the material.
How Will You Determine the Subjective Aspects of Your Work?
Consider exploring one’s motivation to advance their education:
- That is if you’re determining whether clinicians who have advanced credentials are more motivated at work; you’ll need to create a clear delineation between motivation and effort and work out how to measure each of these independently
Operationalization is the process of defining concepts or constructs in a measurable way. As you dive into the ‘HOW’ you will go about your research, you will need to understand the terminology related to study design
As we discussed in Chapter 1, there are several kinds of Variables. As a reminder, a variable is an objective and measurable representation of a theoretical construct. An independent variable is a variable which causes an effect on the dependent, or outcome variable. Note that there may be more than one independent variable in your study. Therefore, the dependent variable is the variable which you are measuring as an effect of an intervention or influence; you can think of this as the outcome variable. Identifying at least these two variables is an essential first step in designing your study. This is because how you explore the relationship between your effect (independent variable) and outcome (dependent variable) with help guide your methodology. Other variables to consider include mediating variables , which are variables that are explained by both the independent and dependent variables. Moderating variables influence the relationship between the independent and dependent variables and control variables which may have an impact on the dependent variable but does not help to explain the dependent variable.
Assigning Dependent and Independent Variables
You would like to determine the relationship between weight and tidal volume:
- Dependent Variable : Which variable DEPENDS on the other? Or, which variable will define the OUTCOME? ( Tidal volume)
- Independent Variable : Does the variable INFLUENCE, HELP EXPLAIN, or have an IMPACT on the dependent variable? (Weight)
You would like to determine whether the number of hours spent in clinical training influences post training test scores :
- Dependent Variable : Score on post training test
- Independent Variable : Number of hours in clinical training
Identifying and assigning the dependent and independent variable(s) is one of the most important research activities as this will help guide you toward the type of information you’ll be collecting and what you will do with that information. However, as you consider both the outcome (dependent) variable and the impact (independent) variable, it is also important to consider what other variables may influence the relationship between these two primary variables.
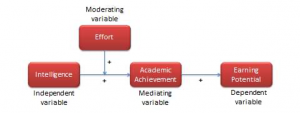
There are very few instances wherein you can control EVERY variable. However, it is your job as a researcher to plan for, acknowledge, and attempt to address anything that may influence the results you present.
levels of measurement can be thought of as values within each variable. For example, traditionally, the variable ‘Gender’ had two values: male or female. The modern variable of ‘Gender’ may have several values which are used to delineate each potential designation within the variable. Each value represents a specific designation of measure.
Values of measures may be considered quantitative (numeric); in our example of traditional gender you may assign a numeric (quantitative) value to male and female as either ‘1’ and ‘2’, respectively. Values may also be assigned non-numerically; meaning they are qualitative. It is important to note that if you want to analyze non-numeric data, it must be coded first.
Understanding and Assigning Value
You may create a question asking respondents to rank their agreement with a statement on a scale ranging from strongly disagree to strongly agree. Although qualitative in nature, we can assign a numeric value to each level of measurement as a ‘code’.
- 1= Strongly Disagree
- 2= Somewhat Disagree
- 3= Neither Disagree nor Agree
- 4= Somewhat Agree
- 5= Strongly Agree
By doing this, we can explore relationships between the attributes and variables using quantitative statistical methods.
Levels of measurement
One of the most important aspects of operationalizing a theoretical construct is to determine the level(s) of measurement. This is done by assessing the types of variables and values:
- Nominal : also called categorical. This level of measurement is used to describe a variable with two or more values BUT there is no intrinsic ordering to the categories
Example of a Nominal Variable
You would like to collect information about the gender (variable) of individuals participating in your study. Your level of measures may be:
You may then assign these measures a numeric value:
- Non-Binary=3
- Ordinal : This level of measurement is used to describe variable values that have a specific rank order. BUT that order does not indicate a specificity between ranks.
Example of an Ordinal Variable
You provide a scale of agreement for respondents to indicate their level of agreement with the use of a current policy within the hospital:
- Strongly Agree
- Strongly Disagree
Note: Those who strongly disagree with the use of this policy disapprove MORE than do those who disagree; however, there is no quantifiable value for how much more.
- Interval : You’ll use this level of measurement for variable values which are rank ordered AND have specified intervals between ranks and can tell you ‘how much more’.
Example of an Interval Variable
You classify the ages of the participants in your study:
- 18-24 years old
- 25-30 years old
- 31-35 years old
- >35 years old
NOTE: 35 is 5 more than 30. The quantifiable ‘how much more’ is what distinguishes age as an interval variable.
- Ratio : Ratio values have all of the qualities of a nominal, ordinal, and/or interval scale BUT ALSO have a ‘true zero’. In this case true zero indicates a lack of the underlying construct (i.e. it does not exist). Additionally, there is a ratio between points on this particular scale. That is, in this case, 10 IS twice that of 5.
Example of a Ratio Variable
You are doing a pre and post bronchodilator treatment trial for a new drug. You must establish baseline heart rate in your treatment group:
- Pulse rate is a ratio variable because the scale has an absolute zero (asystole) and there is a ratio between the number of times the heart beats (i.e. a change in heart rate of 10 beats per minute)
Identification of variable and values is essential to a successful project. Not only will doing this early in the process allow you to predict factors that may affect your research question, but it will also guide you toward the type of data you will collect and determine what kind of statistical analyses you will likely be performing in order to understand and present the results of your work.
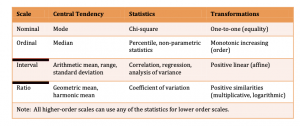
Scales are used to glean insight into a situation or phenomenon and can be used to help quantify information that would otherwise be difficult to understand or convey. Although there are several types of scales used by researchers, we’ll focus on the two of the most common:
- Binary scale : Nominal scale that offers two possible outcomes, or values. Questions that force a respondent to answer either ‘yes’ or ‘no’ utilize a binary scale. IF you offer more than two options, your scale is no longer binary, but is still a nominal scaled item

- Likert scales : Likert scales are popular for measuring ordinal data and include indications from respondents. Data can be quantified using codes assigned to responses and an overall summation for each attribute can be associated with each respondent
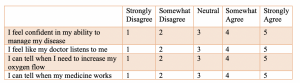
Sampling is the statistical process of selecting a subset (called a “sample”) of a population of interest for purposes of making observations and statistical inferences about that population. We cannot study entire populations because of feasibility and cost constraints, and hence, we must select a representative sample from the population of interest for observation and analysis. It is extremely important to choose a sample that is truly representative of the population so that the inferences derived from the sample can be generalized back to the population of interest. Probability sampling is a technique in which every unit in the population has a chance (non-zero probability) of being selected in the sample, and this chance can be accurately determined. An example of probability sampling is simple random sampling wherein you include ALL possible participants in a population and utilize a method to randomly select a sample that is representative of that population. Nonprobability Sampling is a sampling technique in which some units of the population have zero chance of selection or where the probability of selection cannot be accurately determined. Typically, units are selected based on certain non-random criteria, such as quota or convenience. Because selection is non-random, nonprobability sampling does not allow the estimation of sampling errors, and may be subjected to a sampling bias. Therefore, information from a sample cannot be generalized back to the population. An example of nonprobability sampling is utilizing a convenience sample of participants due to your close proximity or access to them.
Why does sampling matter?
When you measure a certain observation from a given unit, such as a person’s response to a Likert-scaled item, that observation is called a response. In other words, a response is a measurement value provided by a sampled unit. Each respondent will give you different responses to different items in an instrument. Responses from different respondents to the same item or observation can be graphed into a frequency distribution based on their frequency of occurrences. For a large number of responses in a sample, this frequency distribution tends to resemble a bell-shaped curve called a normal distribution, which can be used to estimate overall characteristics of the entire sample, such as sample mean (average of all observations in a sample) or standard deviation (variability or spread of observations in a sample). These sample estimates are called sample statistics (a “statistic” is a value that is estimated from observed data). Populations also have means and standard deviations that could be obtained if we could sample the entire population. However, since the entire population can never be sampled, population characteristics are always unknown, and are called population parameters (and not “statistic” because they are not statistically estimated from data). Sample statistics may differ from population parameters if the sample is not perfectly representative of the population; the difference between the two is called sampling error. Theoretically, if we could gradually increase the sample size so that the sample approaches closer and closer to the population, then sampling error will decrease and a sample statistic will increasingly approximate the corresponding population parameter.
If a sample is truly representative of the population, then the estimated sample statistics should be identical to corresponding theoretical population parameters. How do we know if the sample statistics are at least reasonably close to the population parameters? Here, we need to understand the concept of a sampling distribution . Imagine that you took three different random samples from a given population, as shown below, and for each sample, you derived sample statistics such as sample mean and standard deviation. If each random sample was truly representative of the population, then your three sample means from the three random samples will be identical (and equal to the population parameter), and the variability in sample means will be zero. But this is extremely unlikely, given that each random sample will likely constitute a different subset of the population, and hence, their means may be slightly different from each other. However, you can take these three sample means and plot a frequency histogram of sample means. If the number of such samples increases from three to 10 to 100, the frequency histogram becomes a sampling distribution. Hence, a sampling distribution is a frequency distribution of a sample statistic (like sample mean) from a set of samples, while the commonly referenced frequency distribution is the distribution of a response (observation) from a single sample. Just like a frequency distribution, the sampling distribution will also tend to have more sample statistics clustered around the mean (which presumably is an estimate of a population parameter), with fewer values scattered around the mean. With an infinitely large number of samples, this distribution will approach a normal distribution. The variability or spread of a sample statistic in a sampling distribution (i.e., the standard deviation of a sampling statistic) is called its standard error. In contrast, the term standard deviation is reserved for variability of an observed response from a single sample.
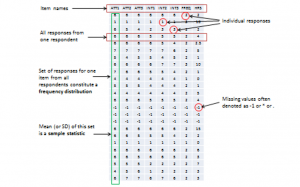
The mean value of a sample statistic in a sampling distribution is presumed to be an estimate of the unknown population parameter. Based on the spread of this sampling distribution (i.e., based on standard error), it is also possible to estimate confidence intervals for that prediction population parameter. Confidence interval is the estimated probability that a population parameter lies within a specific interval of sample statistic values. All normal distributions tend to follow a 68-95-99 percent rule (see below), which says that over 68% of the cases in the distribution lie within one standard deviation of the mean value (μ 1σ), over 95% of the cases in the distribution lie within two standard deviations of the mean (μ 2σ), and over 99% of the cases in the distribution lie within three standard deviations of the mean value (μ 3σ). Since a sampling distribution with an infinite number of samples will approach a normal distribution, the same 68-95-99 rule applies, and it can be said that:
- (Sample statistic one standard error) represents a 68% confidence interval for the population parameter.
- (Sample statistic two standard errors) represents a 95% confidence interval for the population parameter.
- (Sample statistic three standard errors) represents a 99% confidence interval for the population parameter.
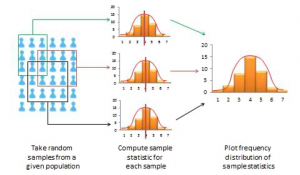
A sample is “biased” (i.e., not representative of the population) if its sampling distribution cannot be estimated or if the sampling distribution violates the 68-95-99 percent rule. As an aside, note that in most regression analysis where we examine the significance of regression coefficients with p<0.05, we are attempting to see if the sampling statistic (regression coefficient) predicts the corresponding population parameter (true effect size) with a 95% confidence interval. Interestingly, the “six sigma” standard attempts to identify manufacturing defects outside the 99% confidence interval or six standard deviations (standard deviation is represented using the Greek letter sigma), representing significance testing at p<0.01.
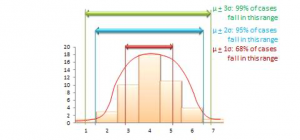
Types of Research Designs
There are many different approaches to research. The list provided here is not exhaustive by any means; rather, this is a brief list of the most common approaches you may identify as you review the literature related to your interest.
Experimental
Experimental research is typically performed in a controlled environment so that the researcher can manipulate an independent variable and measure the outcome (dependent variable) between a group of subjects who received the manipulated variable (intervention) and a group of subjects who did not receive the intervention. This type of design typically adheres to the scientific method in order to test a hypothesis. A hypothesis is a proposed explanation for a phenomenon and serves as the starting point for the investigation. You may see a hypothesis indicated as (H O ), also called the null hypothesis. This is to differentiate it from an alternative hypothesis (H 1 or H A ), which is any hypothesis other than the null.
Development of the Hypothesis
There are two types of hypotheses, the null (HO) and an alternative (H 1 or H A )
- H O = There is no significant difference between length of stay for patients diagnosed with COPD and those diagnosed with CHF.
- H 1 or H A = There is a significant difference between length of stay for patients diagnosed with COPD and those diagnosed with CHF
NOTE: Accepting the null hypothesis would mean that your data confirm that there is no difference. Rejecting the null would mean that your data indicated that there is a significant difference in patient outcomes for these groups; therefore, rejecting the null means accepting an alternative hypothesis.
Randomized Experimental : Participants are randomly assigned to either a treatment (intervention) or a control group. Typically, the treatment group receives an intervention (independent variable) and the outcome of each group is considered dependent variables and compared for effect. Independent variables in this case are considered active in that this variable can be manipulated.
Example of Randomized Experimental Approach
You would like to assess outcomes as they relate to the post delivery resuscitation of very low birthweight infants in the delivery room. You have decided that one group will receive direct intubation and surfactant (intervention group) in the delivery room and the other will receive the standard care of CPAP (control group). Participants will be randomly assigned to groups and as a bonus, the assignment to groups will be blinded. You will then compare the difference between participants in each group regarding need for oxygen at 36 weeks adjusted gestational age.
- Dependent Variable: Need for oxygen at 36 weeks adjusted gestational age
- Independent Variable (Active) : Administration of surfactant
Quasi Experimental : Similar to the randomized experimental approach aside from the random assignment. In quasi-experimental approaches, participants are NOT randomly assigned; however, one group does receive an intervention while the control group does not and outcomes are still compared. The independent variable is also active.
Example of Quasi Experimental Approach
You would like to assess outcomes as they relate to the post delivery resuscitation of very low birthweight infants in the delivery room. You have decided that one group will receive direct intubation and surfactant (intervention group) in the delivery room and the other will receive the standard care of CPAP (control group). Participants will be assigned to groups based on administration of maternal steroids. You will then compare the difference between participants in each group regarding need for oxygen at 36 weeks adjusted gestational age.
Non Experimental
Non-experimental approaches include a wide variety of approaches; therefore, it is difficult to list them all in a succinct way here. However, it is safe to say that a study approach is considered non-experimental when there lacks intentional manipulation of the independent variable.
Comparative approach : Groups are compared to reveal differences in outcome (dependent variable). Groups are typically formed based on independent variables that cannot be manipulated but are important to the study. This type of independent variable is known as an attribute independent variable. In this approach there are a few categories (2-4 levels) of attribute independent variables that are then compared.
Example of Comparative Approach
You would like to investigate the perceptions of first and second year student-instructor engagement and student learning and instructor motivation in the clinical environment.
- Dependent Variable : Student perception of experience (2 levels: First and second year)
- Independent Variable : Student-instructor engagement in learning and motivation
Associational or Correlational approach : Two or more variables for the same group of participants are explored for relationships. Independent variables are also attributive in this approach; meaning, they can be manipulated to impact the dependent variable. Variables included in this approach are typically continuous or have at least five ordered categories.
Example of Associational or Correlational Approach
You would like to conduct a study to better understand practitioner attitudes about the future of the profession.
- Dependent Variable: Attitude about the future of the profession
- Independent Variable(s): Age, gender, autonomy
Descriptive research : Projects which only gather data which can be described, not inferred. That is, results and data collected cannot be inferred back to the population nor can comparisons or associations be made. Many qualitative studies are considered descriptive. This is done by considering only one variable at a time and there is no independent variable.
Example of Descriptive Research
You would like to describe the development of a protocol to implement high flow nasal cannula as an intermediate therapy for acute respiratory failure to be used in the Emergency Department at your institution. You plan to compare rates of intubation before and after implementation of the protocol.
- You are DESCRIBING a process
- You may collect and compare data using descriptive statistics
It is important to note that it is possible to have more than one approach in one research project. This is because the approach selected is specific to the question that has been asked. If there is more than one question asked, it is reasonable to assume that more than one approach may be used.
There are a few areas of research that although fit under the category of non-experimental; do not quite fit within the classifications presented here. Two of these areas are quality improvement (QI) projects and protocol development.
Quality improvement (QI) projects: The purpose of a QI project is to evaluate the performance of systems, processes, or practices to determine whether either function or operational improvements are needed. Using tools such as the SQUIRE explanation and elaboration guidelines , is extremely helpful in developing, conducting, and analyzing a thorough and impactful QI project.
The SQUIRE guidelines focus on the following four questions:
- Why did you start?
- What did you do?
- What did you find?
- What does it mean?
These four questions are then expanded upon to help develop the systematic approach to your inquiry and presentation of your findings. An extended investigation of this method is covered in Chapter 6.
Protocol Development
Before we dig too deep into the development of protocols, a clarification needs to be made regarding vocabulary relating to projects of this nature. Although frequently used interchangeably, the terms protocol and guideline are not synonymous. A protocol is described as an official procedure or system of rules governing a process. A guideline is a suggested course of action, policy, or conduct. In healthcare, this is an important distinction; a protocol is a course of action to which treatment must follow without deviation whereas a guideline, although firmly rooted in evidence, allows for deviation based on best judgment of a clinician or presentation of a specific case. Through a research lens, this distinction is important because the process by which these two objectives are realized are very different. The complete process for the development of guidelines which are generalizable beyond a specific situation is best outlined by the World Health Organization Handbook
The development of both guidelines often involves a team of people who are charged with first evaluating the existing evidence and then contributing an interpretation of that evidence toward the consensus of best practice. This is why guidelines are typically issued by federal or state agencies or professional organization. Protocols are generally less generalizable due to contextual constraints. However, even organizational protocols are not developed by a single individual. This does not mean, however, that you cannot begin the process of developing a guideline or protocol for your organization on you own; rather, it is important to frame the work you contribute as the foundation upon which a group can work toward the consensus of best practice. Typically, this initial work is referred to as a narrative review. A narrative review can be described as a broad perspective on a topic which may or may not be impacted by bias. This type of review differs from a systematic review in that it is understood that a narrative review may not encompass all relevant literature on a relevant topic as might a systematic review. Another note; the development of both guidelines and protocols is often an iterative process requiring several cycles of evaluation and revision. A systematic review is described as exhaustive review of the literature relevant to a specific topic. In addition to being exhaustive, a systematic review includes methodology which is both explicit and reproducible to select, evaluate, and synthesize ALL available evidence. A meta-analysis is a systematic approach to evaluating the data from independent studies of the same subject to evaluate overall trends. Often, a meta-analysis is part of a systematic review.
Selecting your approach
As we’ve discussed, there are several factors which will guide your approach selection. Emphasis should be placed on the development of your purpose and problem statements as well as your research question. Ambiguity in these areas may cause some confusion as you begin to consider what approach you will take to answer your question. Here we will work to narrow the scope of your approach using a systematic process and answering a few specific questions:
Step 1: Outlining your general purpose
Understanding the overarching goal of your study will help direct the rest of your approach. Here, you will ask yourself “What am I trying to do?”.

Step 2: Identifying your general approach
Earlier we discussed the difference between experimental and non-experimental approaches. As we mentioned, these are two broad categories of approaches. Your general purpose will determine which of these two general approaches you take. The determination here will point you toward a more focused, or specific, approach.
- Experimental: Experimental research is typically performed in a controlled environment so that the researcher can manipulate an independent variable and measure the outcome (dependent variable) between a group of subjects who received the manipulated variable (intervention) and a group of subjects who did not receive the intervention. A true experimental approach means that you have random selection or assignment of participants. All other elements aside, if you do NOT have randomization incorporated into your approach, your approach becomes quasi-experimental.
- Non-experimental: Nonexperimental research is an extremely broad category of approaches. Therefore, the simplest way to explain non-experimental research is to simply state that this approach lacks the manipulation of an independent variable. That is, you are not imposing an intervention on one group and comparing the outcome with a control group. Rather, you may have attribute independent variables which influence, or impact, the dependent variable, but the purpose of the research is not the direct manipulation of that variable. There are several different types of non-experimental research approaches, as we will soon see; however, it is important to understand that descriptive research is always classified as nonexperimental.
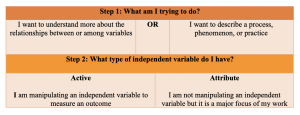
Step 3: Narrowing down your specific purpose
Now that you’ve decided what the general purpose and approach, you can begin to really narrow down the ‘how’ of your research. I find that this is best done by again asking yourself what you are really trying to do. Now that you understand the boundaries of your purpose and approach, you can work to understand the fine points about what types of interactions between variables you’re looking to explore and determine.
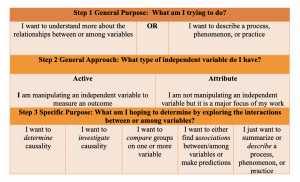
Step 4 : Selecting your specific approach
As you can see, there are specific words you should pay attention to when you’re describing your purpose. Given these key words, like ‘determine causality’, or ‘compare groups’, you’ll have a bit more direction as to what approach is most appropriate to identify the best answer to your question. Once we know what it is we really want to do with the information we’re planning to gather (variables), we can select an approach. Selecting your specific approach
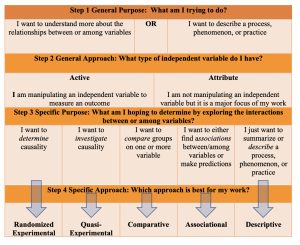
Key Takeaways
There are several important concepts presented in this chapter:
- The theoretical/conceptual framework is the frame, or lens, that YOU build for your reader. It is the perspective through which you would like them to view your work.
- Constructs represent abstract theory
- Variables are the concrete measures of constructs
- There are several different types of variables; however, understanding the relationship between the independent variable (impact variable) and the dependent variable (outcome variable) is extremely important
- Attributes are levels within variables
- Attributes and variables must be classified in terms of measurement: Nominal, ordinal, interval, and ratio variables each represent different information and must be assessed correctly to have meaning
- Sampling is very important because whether your sample represents the larger population is an important factor in how your research is presented and interpreted
- There are A LOT of different approaches to research. Systematically approaching the selection of your approach by first defining your problem and purpose statements and your research question will be helpful as you narrow your focus on the which approach best captures the interaction between or among variables
Crawford, L.M., Burkholder, G.J., Cox, K.A. (2020). Writing the Research Proposal. In G.J. Burkholder, K.A Cox, L.M. Crawford, and J.H. Hitchcock (Eds.), Research design and methods: An applied guide for the scholar-practitioner (pp. 309-334). Sage Publications
Gliner, J.A., Morgan, G.A., & Leech, N.L. (2017). Research methods in applied settings: An integrated approach to design and analysis. Routledge
- This section can be attributed to Bhattacherjee, A. (2012) published under Creative Commons Attribution-NonCommercial-ShareAlike 3.0 Unported License ↵
Defining a construct through your lens as a researcher. How you are choosing to describe the boundaries associated with your work
A measurable representation of an abstract construct
A variable that can explain another variable. A variable which may be manipulated (active) or describes (attribute) to affect an outcome
The variable which is measured as an outcome and is affected by the independent variable(s)
Variables that are explained by both the independent and dependent variables
Influence the relationship between the independent and dependent variable
A variable which has an impact on the dependent variable, but does not explain the outcome (dependent variable)
values within each variable.
The assignment of a number to an attribute to describe a variable
Variable with two or more layers but without a specific order
A variable which has a specific rank order but no specificity between the ranks
Rank ordered variable with specified intervals between ranks
Has a true zero within the scale against which it is measured
A tool, or measure, used to quantify material that may be difficult to do so otherwise
Nominal scale with two potential outcomes
Used to measure ordinal data with a ranking system
Method of selecting a subset of the population to study.
Method of sampling wherein potential for sampling is equally likely for the entire population
Method of sampling where in the likelihood of being selected into a sample is not equal across the population
Visual representation of how a sample falls around a mean
A proposed explanation for the observed phenomenon
A form of experimental study design were participants are randomly assigned to either an intervention or control group
A form of experimental design involving both intervention and control groups but lacks randomization
Groups of participants are compared to identify differences in outcome
Two or more variables for the SAME group of participants are explored for relationships
Research projects wherein data gathered and described, but no relationships are inferred
A subset of nonexperimental research wherein the performance of systems, processes, or practices are evaluated for either efficiency or effectiveness
An official procedure or system
A suggested course of action
A broad perspective on a topic, typically from the perspective of a single author
An exhaustive review of literature relevant to a specific topic; typically performed by a group of people
Systematic approach to evaluating data from independent studies on a topic to evaluate or identify trends
Research performed in a controlled environment in which a researcher can manipulate an independent variable and measure a dependent variable (outcome)
Broad category of research approaches which lack the manipulation of an independent variable
Practical Research: A Basic Guide to Planning, Doing, and Writing Copyright © by megankoster. All Rights Reserved.
Share This Book
User Preferences
Content preview.
Arcu felis bibendum ut tristique et egestas quis:
- Ut enim ad minim veniam, quis nostrud exercitation ullamco laboris
- Duis aute irure dolor in reprehenderit in voluptate
- Excepteur sint occaecat cupidatat non proident
Keyboard Shortcuts
1.3 - steps for planning, conducting and analyzing an experiment.
The practical steps needed for planning and conducting an experiment include: recognizing the goal of the experiment, choice of factors, choice of response, choice of the design, analysis and then drawing conclusions. This pretty much covers the steps involved in the scientific method.
- Recognition and statement of the problem
- Choice of factors, levels, and ranges
- Selection of the response variable(s)
- Choice of design
- Conducting the experiment
- Statistical analysis
- Drawing conclusions, and making recommendations
What this course will deal with primarily is the choice of the design. This focus includes all the related issues about how we handle these factors in conducting our experiments.
Factors Section
We usually talk about "treatment" factors, which are the factors of primary interest to you. In addition to treatment factors, there are nuisance factors which are not your primary focus, but you have to deal with them. Sometimes these are called blocking factors, mainly because we will try to block on these factors to prevent them from influencing the results.
There are other ways that we can categorize factors:
Experimental vs. Classification Factors
Quantitative vs. qualitative factors, try it section .
Think about your own field of study and jot down several of the factors that are pertinent in your own research area? Into what categories do these fall?
Get statistical thinking involved early when you are preparing to design an experiment! Getting well into an experiment before you have considered these implications can be disastrous. Think and experiment sequentially. Experimentation is a process where what you know informs the design of the next experiment, and what you learn from it becomes the knowledge base to design the next.
Designing a research study
Affiliation.
- 1 The Yale School of Nursing, New Haven, Connecticut
- PMID: 18375773
- DOI: 10.1177/0145721708315678
Diabetes educators and advanced-practice nurses in diabetes care often participate in clinical research. The purpose of this article is to provide a broad overview of important decisions in planning a research study. The research process is reviewed, including the conceptual phase, the design and planning phase, the empirical phase, the analytic phase, and the dissemination phase. Issues unique to diabetes education research are highlighted.
- Diabetes Mellitus / nursing
- Diabetes Mellitus / prevention & control*
- Diabetes Mellitus / rehabilitation
- Nursing Research
- Reproducibility of Results
- Research Design*
- Search Search Please fill out this field.
Market Research
Business planning, website development, product or service selection, marketing and promotion, is it a good idea to start an online business, can i start an online business with $100, what are different types of online marketing strategies, the bottom line.
- Small Business
- How to Start a Business
Starting an Online Business: A Step-by-Step Guide
Crafting a Winning Business Plan: Setting Goals and Strategies
:max_bytes(150000):strip_icc():format(webp)/picture-53823-1434118722-5bfc2a8c46e0fb005119858e.jpg)
Katie Miller is a consumer financial services expert. She worked for almost two decades as an executive, leading multi-billion dollar mortgage, credit card, and savings portfolios with operations worldwide and a unique focus on the consumer. Her mortgage expertise was honed post-2008 crisis as she implemented the significant changes resulting from Dodd-Frank required regulations.
- How to Start a Business: A Comprehensive Guide and Essential Steps
- How to Do Market Research, Types, and Example
- Marketing Strategy: What It Is, How It Works, How To Create One
- Marketing in Business: Strategies and Types Explained
- What Is a Marketing Plan? Types and How to Write One
- Business Development: Definition, Strategies, Steps & Skills
- Business Plan: What It Is, What's Included, and How to Write One
- Small Business Development Center (SBDC): Meaning, Types, Impact
- How to Write a Business Plan for a Loan
- Business Startup Costs: It’s in the Details
- Startup Capital Definition, Types, and Risks
- Bootstrapping Definition, Strategies, and Pros/Cons
- Crowdfunding: What It Is, How It Works, and Popular Websites
- Starting a Business with No Money: How to Begin
- A Comprehensive Guide to Establishing Business Credit
- Equity Financing: What It Is, How It Works, Pros and Cons
- Best Startup Business Loans
- Sole Proprietorship: What It Is, Pros & Cons, and Differences From an LLC
- Partnership: Definition, How It Works, Taxation, and Types
- What is an LLC? Limited Liability Company Structure and Benefits Defined
- Corporation: What It Is and How to Form One
- Starting a Small Business: Your Complete How-to Guide
- Starting an Online Business: A Step-by-Step Guide CURRENT ARTICLE
- How to Start Your Own Bookkeeping Business: Essential Tips
- How to Start a Successful Dropshipping Business: A Comprehensive Guide
Oscar Wong / Getty Images
If you want to get into the online business game, it’s a good time to start. The COVID-19 pandemic reshaped online consumer spending, including how people shop online and how they research products.
Today, 76% of Americans buy products online. Furthermore, roughly a third of people purchase items online weekly. From setting up an ecommerce business to offering web design services, there are countless avenues to explore as an entrepreneur.
Below, we’ll walk through each step to building an online business.
Key Takeaways
- When starting an online business, comprehensive market research is critical for identifying your target audience and learning how to resonate with your customers and understand their needs.
- Creating a business plan is an important step for outlining your business goals. It also includes your product description, target market, and financial projections, among other core components.
- Building your website involves setting up a domain name, finding a hosting company, and designing a strong website with consistent branding that allows your customers to navigate it intuitively.
- Choosing the right product or service to sell is essential. It’s important to think about how you’re addressing an unmet need.
- Several digital marketing strategies can be utilized, from content marketing to paid advertising, to help your business grow.
Successful online entrepreneurs study hard in order to have a thorough understanding of their market. This is important for knowing exactly how to reach your target market , because these are the people who will buy your products and drive your business growth.
At its core, market research is about understanding your customers’ needs, pain points, and solutions. It is designed to help your business better meet these needs.
Steps to Conduct Market Research
Market research involves understanding key aspects of your current and future customers. To get a clear sense of your target market, outline the characteristics of your audience—for example, age, location, gender, income, job title, and key pain points.
Once you have identified your target audience, conduct research on the following topics, which will tell you about how they make decisions and how you can better position your business:
- What are the challenges that your target market faces?
- Where do they research a given product or service?
- What are their views on pricing for this product or service?
- What factors influence their decision to make a purchase?
- Who are your competitors?
To put this market research into action, there are a number of different avenues you can take:
- Focus groups
- Competitive analysis
- Brand awareness research
- Market segmentation research
Consider the following questions that may be asked in an interview or focus group to learn more about your audience:
- “How do you search for that product?”
- “How useful was it?”
- “What words do you use when you search on Google?”
When you have completed your market research, identify what you have learned as well as your next steps based on these insights.
Creating a business plan is a key first step for all business owners . It is important for companies looking to secure funding resources. It also serves as a blueprint to summarize your key business objectives and goals.
To write a business plan , incorporate these eight main sections, which are often found in traditional templates:
- Executive summary : This is typically a one-page section that explains your objectives and includes your mission statement, core team, and why your company is positioned for success.
- Company description : This describes what you offer, your competitive advantages, and your business goals.
- Market analysis : This is where you explain your target market, market size, market trends, and competitive landscape.
- Organization and management : Explain who is working on your team and their professional background and experience.
- Service or product line : Describe the product or service you are offering, including any copyright or plans for patenting.
- Marketing and sales : Discuss your marketing and sales strategy. Discuss your pricing, key metrics, and sales plan.
- Funding request : If you are a company looking for funding, here is where you outline the capital you are requesting and where it will be allocated.
- Financial projections : Include projections for your company’s revenue and expenses. Consider including an income statement, balance sheet, and cash flow statement in this section.
A business plan is important because it helps clarify your action points, who you are, and what you offer, all in a coherent template.
Getting your business online is the next key step. In an ever-changing environment, it is important to know the tools, trends, and strategies for building a strong online presence to allow your business to grow.
Registering Your Domain
The first step is registering your name, or your website address. This can be in the form of your business name “.com.” To purchase your domain name, you can go to sites like GoDaddy or Namecheap . If you decide to build your website using WordPress, you will need to use a site such as these to host your website.
Web Hosting Companies
Alternatively, you can buy your domain name at a hosting company. These are companies like Shopify , Wix , or Amazon Web Services , that may also offer tools to build your website and release content on them.
Website Design
A well-designed website is important for many reasons. Using a website builder, such as Mailchimp or Squarespace , can allow you to choose a theme, customize your pages, create relevant content, and set up a payment page.
Other key aspects of your website design include its functionality, simplicity, and ease of use. Allowing your potential customers to navigate the site intuitively will be key to their experience. Brand consistency—in your logo, colors, and typeface, for example—is also key to creating a unified brand.
Another essential part of website design is its mobile application. You’ll want to ensure that your website runs smoothly on mobile, that images load properly, that the text is legible, and that buttons are intuitive to click.
This step focuses on how to choose the right product or service to sell. At the heart of this choice is the goal of solving a customer’s problem. But there are a number of strategies you can use to identify your product idea.
For example, you might consider analyzing companies with high-profit margins, products that align with your passion, burgeoning trends, items trending on online marketplaces, and/or customer reviews.
With this in mind, analyze how this product will get to your customers. Additionally, you may consider products that are not available in stores in your local market but are offered in communities such as Europe or Japan, for example.
Marketing strategy and promotion is an essential driver of business growth. As the digital landscape evolves, it’s important to have an effective marketing plan that resonates with changing consumer preferences and needs.
Here are questions that companies can consider as they create their marketing strategy, navigating today’s environment:
- Impact, value, and growth : What are the goals and key performance indicators (KPIs) that will measure success for your business? How will you explain the value that the business provides to its customers and/or society? Create an “elevator speech”—a 30-second description of what you offer and why it’s special.
- Customer need and brand promise : How does the brand meet a customer’s need through its products and services?
- Customer experience : How will the business deliver the best experiences at each stage of the customer journey?
- Organizational model : How will the business operate to serve the customer with the most impact?
These will help you understand what types of strategies can have real impact.
Types of Marketing Strategies
Consider the following digital marketing strategies that can be used for your online business:
- Email marketing
- Social media marketing
- Paid advertising
- Search engine optimization (SEO)
- Content marketing
- Influencer marketing
Each of these presents a different way to reach your target audience, drive conversions, or build brand awareness, depending on your marketing goals.
You need to determine that for yourself. But before starting an online business, it’s important to assess the time, investment, and resources you’ll need to get it off the ground. While the barrier to entry can be quite low, it’s worth considering your goals and strategies for making it a reality.
However, compared with starting up a traditional brick-and-mortar business, the risks of launching an online business may be reduced due to lower upfront costs such as rent, staff, and materials, among others.
The short answer: yes. While it depends on the type of business you hope to pursue, there are many ways to set up an online business at very little cost. For example, you could offer your services doing freelance work, photography, bookkeeping, or personal training. The primary costs involved include setting up your business website, which can cost as little as $2 to $20 each year with companies such as GoDaddy.
There are a number of digital marketing strategies that online businesses can use, such as content marketing, email marketing, paid advertising, SEO, and influencer marketing. Each of these strategies can be useful, depending on your product and goals.
Starting an online business can be a powerful way to launch a new product or service while reaching a wider audience. With market research, a solid business plan, a strong website, and a digital marketing strategy, you can get started in growing your company effectively. As customers increasingly make decisions virtually, building an online business is vital to any business owner’s success.
Pew Research Center. “ For Shopping, Phones Are Common and Influencers Have Become a Factor—Especially for Young Adults .”
U.S. Small Business Administration. “ Market Research and Competitive Analysis .”
U.S. Small Business Administration. “ Write Your Business Plan .”
Ogilvy. “ Getting Future Ready with Marketing Transformation ,” Page 15.
GoDaddy. “ How Much Does a Domain Name Cost? Find Out! ”
:max_bytes(150000):strip_icc():format(webp)/shutterstock_222400081-5bfc47c7c9e77c00587d6bbf.jpg)
- Terms of Service
- Editorial Policy
- Privacy Policy
- Your Privacy Choices
Developing a Senior Leadership Succession Plan for a Global Manufacturer
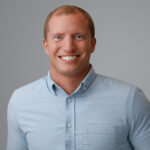
Clarkston Consulting recently partnered with a client on a senior leadership succession plan. Read a synopsis of the project below or download the full case study.
Download the Senior Leadership Succession Plan Case Study Here
A global leader in design and manufacturing asked Clarkston Consulting to evaluate their strategic business needs and make recommendations for executive succession in conjunction with an enterprise strategy development project.
The company required several aspects for its succession planning efforts that included identifying a successor for the senior executive role who leads the global enterprise, assessing internal talent pools to identify high-potential successors, and ensuring a smooth leadership transition without disrupting business operations once identified. Additionally, there was a need to foster communication and engagement among stakeholders to garner support for the succession plan and ensure an effective transition .
To address these challenges, the company adopted Clarkston’s strategic approach to succession planning. The first step involved an exhaustive analysis of the company’s strategic objectives and priorities that had been developed through Clarkston’s Enterprise Destination Mapping process to analyze leadership traits, qualities, and capabilities essential to leading the business into the future. Clarkston determined the leadership criteria needed to successfully execute the company’s strategic plan and priorities as well as developed five critical senior executive leader success metrics, with clear definitions and criteria for the client’s next top global leader.
Clarkston also partnered with the CEO and HR leader to assess the internal talent to determine if there were any qualified candidates with the required capabilities who are ready now for the role or have the potential to be ready within one year. Clarkston conducted a detailed assessment of top qualified candidates and made a recommendation for the top executive role with an accompanying accelerated development plan, transition plan with the current leader, and detailed timeline including key milestones and communication events.
The strategic implementation of succession planning facilitated the identification and development of a high-potential employee, ensuring readiness for future leadership transitions, and enhancing company performance for a successful successor transition to drive growth. The Board of Directors approved the succession plan, and it was successfully implemented.
Download the Senior Leadership Succession Plan case study, and learn more about our Strategic Advisory Consulting Services by contacting us below.
Contact Us to Learn More
Related Insights
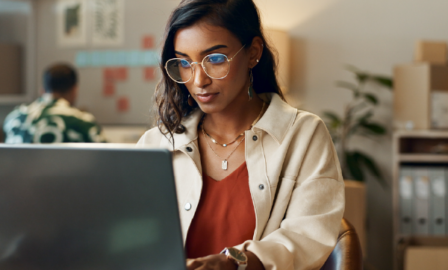
Navigating a Leadership Transition: 5 Key Steps for Succession Planning
Changes in the top leadership ranks of companies are necessary, inevitable, and can be very healthy for the organization. Even ...
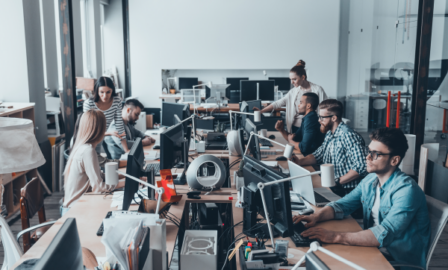
Ensuring Organizational Alignment: A CEO’s Roadmap for Navigating Change
In this eBook, we outline a CEO’s roadmap for navigating change, sharing how they can ensure organizational alignment, and ultimately, ...
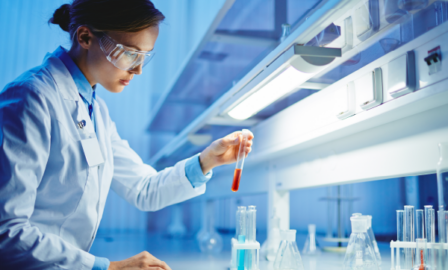
Aligning Organizational Structure with Strategy to Support Future Growth
Clarkston Consulting recently partnered with a global biopharmaceutical company and helped the client in aligning their organizational structure with strategy. ...
Subscribe to All Posts
- Life Sciences
- Consumer Products
You may unsubscribe from these communications at any time.
- Comments This field is for validation purposes and should be left unchanged.
Multimodality treatment planning using the Markov decision process: a comprehensive study of applications and challenges
- Published: 28 May 2024
Cite this article
- Pushpendra Singh ORCID: orcid.org/0000-0001-8608-5188 1 ,
- Seema Singh nAff2 ,
- Alka Mishra nAff1 &
- Santosh Kumar Mishra nAff1
According to the oncologist, a single medication is insufficient to completely cure the disease; as a result, most patients undergo treatment from two or more types of therapy, sometimes in succession, in order to control the progression of their cancer.
This comprehensive review explores the applications and challenges associated with multimodality treatment planning utilizing the Markov decision process (MDP) framework. The benefits of employing MDP in treatment planning include its ability to incorporate multiple treatment modalities, optimize treatment sequences, and account for uncertainties in patient response. However, several limitations exist, such as the complexity of modeling interactions between different treatment modalities, the need for accurate input data, and the computational burden associated with solving large-scale MDP problems.
This review highlights the importance of considering both the benefits and limitations of using MDP in multimodality treatment planning to enhance patient outcomes and optimize resource allocation in healthcare settings. Finally, by taking a case analysis into consideration, we analyze how altering the ultimate cost function affects the efficacy of MDP optimal plans.
We summarize the findings of several studies that have employed MDP in treatment planning and discuss the benefits, limitations, and potential future directions of this approach. It also provides a comprehensive review of the benefits of MDP-based multimodality treatment planning, including improved treatment outcomes, reduced toxicities, personalized treatment decisions, and optimized sequencing and timing of treatment modalities.
This is a preview of subscription content, log in via an institution to check access.
Access this article
Price includes VAT (Russian Federation)
Instant access to the full article PDF.
Rent this article via DeepDyve
Institutional subscriptions
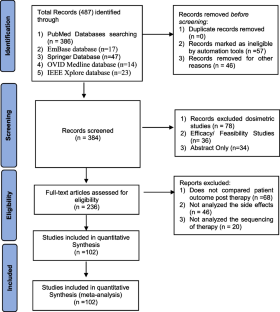
Similar content being viewed by others
Phase I Cancer Clinical Trial Design: Single and Combination Agents
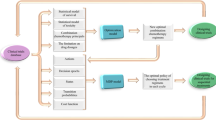
A finite-horizon Markov decision process model for cancer chemotherapy treatment planning: an application to sequential treatment decision making in clinical trials
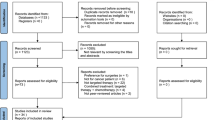
Patient Preferences in Targeted Pharmacotherapy for Cancers: A Systematic Review of Discrete Choice Experiments
Abdellatif AA, Mhaisen N, Mohamed A, Erbad A, Guizani M. Reinforcement learning for intelligent healthcare systems: a review of challenges, applications, and open research issues. IEEE Internet of Things Journal (IEEE). 2023;10(24):21982–2007. https://doi.org/10.1109/JIOT.2023.3288050 .
Article Google Scholar
Adeyiola AO, Rabia SI, Elsaid A, Fadel S, Zakaria A. A Markov decision process framework for optimal cancer chemotherapy dose selection. In J Phys: Conf Ser. 2022;2287(1):012002.
Google Scholar
Alagoz O, Maillart LM, Schaefer AJ, Roberts MS. The optimal timing of living-donor liver transplantation. Manage Sci. 2004;50(10):1420–30.
Alagoz O, Hsu H, Schaefer AJ, Roberts MS. Markov decision processes: a tool for sequential decision making under uncertainty. Med Decis Making. 2010;30(4):474–83. https://doi.org/10.1177/0272989X09353194 .
Bäuerle N, Rieder U. Markov decision processes with applications to finance. Germany: Springer Science & Business Media; 2011. https://doi.org/10.1007/978-3-642-18324-9 .
Book Google Scholar
Bazrafshan N, Lotfi MM. A finite-horizon Markov decision process model for cancer chemotherapy treatment planning: an application to sequential treatment decision making in clinical trials. Ann Oper Res. 2020;295:483–502.
Article MathSciNet Google Scholar
Bennett CC, Hauser K. Artificial intelligence framework for simulating clinical decision-making: a Markov decision process approach. Artif Intell Med. 2013a;57(1):9–19.
Bennett CC, Hauser K. Artificial intelligence framework for simulating clinical decision-making: a Markov decision process approach. Artif Intell Med. 2013b;57(1):9–19. https://doi.org/10.1016/j.artmed.2012.12.003 .
Bertuzzi A, Conte F, Papa F, Sinisgalli C. Applications of nonlinear programming to the optimization of fractionated protocols in cancer radiotherapy. Information. 2020;11(6):313.
Bird TG, Ngan SY, Chu J, Kroon R, Lynch AC, Heriot AG. Outcomes and prognostic factors of multimodality treatment for locally recurrent rectal cancer with curative intent. Int J Colorectal Dis. 2018;33:393–401.
Borera EC, Moore BL, Pyeatt LD. Partially observable markov decision process for closed-loop anesthesia control. IOS Press; 2012.
Bramer WM, Giustini D, de Jonge GB, Holland L, Bekhuis T. De-duplication of database search results for systematic reviews in EndNote. J Med Libr Assoc: JMLA. 2016;104(3):240.
Briggs A, Sculpher M. An introduction to Markov modelling for economic evaluation. Pharmacoeconomics. 1998;13:397–409.
Calderone D, Shankar Sastry S. Markov decision process routing games. In: 2017 ACM/IEEE 8th international conference on cyber-physical systems (ICCPS). Pittsburgh: Association for Computing Machinery; 2017. pp. 273–9. https://doi.org/10.1145/3055004.3055026 .
Chapter Google Scholar
Chanchaichujit J, Tan A, Meng F, Eaimkhong S, Chanchaichujit J, Tan A, Meng F, Eaimkhong S. Optimization, simulation and predictive analytics in healthcare. In: Healthcare 4.0: next generation processes with the latest technologies. Singapore: Palgrave Pivot; 2019. pp. 95–121. https://doi.org/10.1007/978-981-13-8114-0_5 .
Choi SE, Brandeau ML, Basu S. Dynamic treatment selection and modification for personalised blood pressure therapy using a Markov decision process model: a cost-effectiveness analysis. BMJ Open. 2017;7(11):e018374.
Choi J, Tocco B, Smith A, Ahmad S, Josephides E, Bille A. Multimodality treatment and salvage surgery for the treatment of lung cancer. Cancers. 2023;15(14):3586.
Debela DT, Muzazu SGY, Heraro KD, Ndalama MT, Mesele BW, Haile DC, Kitui SK, Manyazewal T. New approaches and procedures for cancer treatment: current perspectives. SAGE Open Medicine. 2021;9:20503121211034370.
Deli T, Orosz M, Jakab A. Hormone replacement therapy in cancer survivors–review of the literature. Pathol Oncol Res. 2020;26:63–78.
Deng J, Wang C, Xiang M, Liu F, Liu Y, Zhao K. Meta-analysis of postoperative efficacy in patients receiving chemoradiotherapy followed by surgery for resectable esophageal carcinoma. Diagn Pathol. 2014;9(1):1–8. https://doi.org/10.1186/1746-1596-9-151 .
Dhas N, Kudarha R, Pandey A, Nikam AN, Sharma S, Singh A, Garkal A, Hariharan K, Singh A, Bangar P, Yadhav D. Stimuli responsive and receptor targeted iron oxide based nanoplatforms for multimodal therapy and imaging of cancer: conjugation chemistry and alternative therapeutic strategies. J Control Release. 2021;333:188–245.
Du F, Plaisant C, Spring N, Crowley K, Shneiderman B. Eventaction: a visual analytics approach to explainable recommendation for event sequences. ACM Trans Interact Intell Syst (TiiS). 2019;9(4):1–31.
Fiorica F, Di Bona D, Filippo Schepis A, Licata L, Shahied A, Venturi AM, Falchi A, Craxì CC. Preoperative chemoradiotherapy for oesophageal cancer: a systematic review and meta-analysis. Gut. 2004;53(7):925–30. https://doi.org/10.1136/gut.2003.025080 .
Forastiere AA, Zhang Q, Weber RS, Maor MH, Goepfert H, Pajak TF, Morrison W. Long-term results of RTOG 91–11: a comparison of three nonsurgical treatment strategies to preserve the larynx in patients with locally advanced larynx cancer. J Clin Oncol. 2013;31(7):845. https://doi.org/10.1200/JCO.2012.43.6097 .
Franklin M, Hunter RM. A modelling-based economic evaluation of primary-care-based fall-risk screening followed by fall-prevention intervention: a cohort-based Markov model stratified by older age groups. Age Ageing. 2020;49(1):57–66. https://doi.org/10.1093/ageing/afz125 .
Garcia G-GP, Steimle LN, Marrero WJ, Sussman JB. Interpretable policies and the price of interpretability in hypertension treatment planning. Manuf Serv Oper Manag. 2024;26(1):80–94. https://doi.org/10.1287/msom.2021.0373 .
Gazdar AF, Girard L, Lockwood WW, Lam WL, Minna JD. Lung cancer cell lines as tools for biomedical discovery and research. J Natl Cancer Inst. 2010;102(17):1310–21. https://doi.org/10.1093/jnci/djq279 .
Ge L, Mourits MCM, Kristensen AR, Huirne RBM. A modelling approach to support dynamic decision-making in the control of FMD epidemics. Prev Vet Med. 2010;95(3–4):167–74. https://doi.org/10.1016/j.prevetmed.2010.04.003 .
Gebski V, Burmeister B, Smithers BM, Foo K, Zalcberg J, Simes J, Australasian Gastro-Intestinal Trials Group. Survival benefits from neoadjuvant chemoradiotherapy or chemotherapy in oesophageal carcinoma: a meta-analysis. Lancet Oncol. 2007;8(3):226–34. https://doi.org/10.1016/S1470-2045(07)70039-6 .
Gondi S, Wright AA, Landrum MB, Zubizarreta J, Chernew ME, Keating NL. Multimodality cancer care and implications for episode-based payments in cancer. Am J Manag Care. 2019;25(11):537–8.
Greer SE, Goodney PP, Sutton JE, Birkmeyer JD. Neoadjuvant chemoradiotherapy for esophageal carcinoma: a meta-analysis. Surgery. 2005;137(2):172–7. https://doi.org/10.1016/j.surg.2004.06.033 .
Harrold JM, Parker RS. Clinically relevant cancer chemotherapy dose scheduling via mixed integer optimization. Comput Chem Eng. 2009;33(12):2042–54.
Hauskrecht M, Fraser H. Planning treatment of ischemic heart disease with partially observable Markov decision processes. Artif Intell Med. 2000;18(3):221–44.
Imani F, Qiu Z, Yang H. Markov decision process modeling for multi-stage optimization of intervention and treatment strategies in breast Cancer. In: 2020 42nd annual international conference of the IEEE engineering in Medicine & Biology Society (EMBC). Montreal: IEEE; 2020. pp. 5394–7. https://doi.org/10.1109/EMBC44109.2020.9175905 .
Ishizawa S. Medical decision making on cancer treatment with Markov decision process. PhD Thesis, Applied Mathematics and Statistics, Stony Brook University. Stony Brook: State University of New York; 2023. https://www.proquest.com/openview/7f7ab3420ffec0cfc7038ad47c5d9edc/1?pqorigsite=gscholar&cbl=18750&diss=y .
Kaplan B. Evaluating informatics applications—clinical decision support systems literature review. Int J Med Informatics. 2001;64(1):15–37.
Katakami N, Tada H, Mitsudomi T, Kudoh S, Senba H, Matsui K, Saka H, Kurata T, Nishimura Y, Fukuoka M. A phase 3 study of induction treatment with concurrent chemoradiotherapy versus chemotherapy before surgery in patients with pathologically confirmed N2 stage IIIA nonsmall cell lung cancer. Cancer. 2012;118(24):6126–35. https://doi.org/10.1002/cncr.26689 .
Kinjo K, Uchibe E, Doya K. Evaluation of linearly solvable Markov decision process with dynamic model learning in a mobile robot navigation task. Front Neurorobot. 2013;7:7.
Kürzinger M-L, Douarin L, Uzun I, El-Haddad C, Hurst W, Juhaeri J, Tcherny-Lessenot S. Structured benefit–risk evaluation for medicinal products: review of quantitative benefit–risk assessment findings in the literature. Ther Adv Drug Saf. 2020;11:2042098620976951.
Larizadeh MH, Mohammadi F, Shabani M, Damghani MA. Induction chemotherapy followed by either chemoradiotherapy or bioradiotherapy in laryngeal cancer. Asian Pac J Cancer Prev. 2021;22(5):1633–7. https://doi.org/10.31557/APJCP.2021.22.5.1633 .
Lazzerini M, Gray D. Oral zinc for treating diarrhoea in children. Commentary. Int J Epidemiol. 2008;37(5):938–40.
Lefebvre JL, Rolland F, Tesselaar M, Bardet E, Leemans CR, Geoffrois L, Hupperets P. Phase 3 randomized trial on larynx preservation comparing sequential vs alternating chemotherapy and radiotherapy. J Natl Cancer Inst. 2009;101(3):142–52. https://doi.org/10.1093/jnci/djn460 .
Lefebvre J-L, Andry G, Dominique Chevalier B, Luboinski LC, Traissac L, De Raucourt D, Langendijk JA. Laryngeal preservation with induction chemotherapy for hypopharyngeal squamous cell carcinoma: 10-year results of EORTC trial 24891. Ann Oncol. 2012;23(10):2708–14. https://doi.org/10.1093/annonc/mds065 .
Lefebvre JL, Pointreau Y, Rolland F, Alfonsi M, Baudoux A, Sire C, de Raucourt D. Induction chemotherapy followed by either chemoradiotherapy or bioradiotherapy for larynx preservation: the TREMPLIN randomized phase II study. J Clin Oncol. 2013;31(7):853–9. https://doi.org/10.1200/JCO.2012.42.3988 .
Li F, Jörg F, Li X, Feenstra T. A promising approach to optimizing sequential treatment decisions for depression: Markov decision process. Pharmacoeconomics. 2022;40(11):1015–32.
Maass K, Kim M. A Markov decision process approach to optimizing cancer therapy using multiple modalities. Math Med Biol: A J IMA. 2020;37(1):22–39.
Magni P, Quaglini S, Marchetti M, Barosi G. Deciding when to intervene: a Markov decision process approach. Int J Med Informatics. 2000a;60(3):237–53.
Magni P, Quaglini S, Marchetti M, Barosi G. Deciding when to intervene: a Markov decision process approach. Int J Med Informatics. 2000b;60(3):237–53. https://doi.org/10.1016/S1386-5056(00)00099-X .
Maitre P, Krishnatry R, Chopra S, Gondhowiardjo S, Likonda BM, Hussain QM, Zubizarreta EH, Agarwal JP. Modern radiotherapy technology: obstacles and opportunities to access in low-and middle-income countries. JCO Global Oncol. 2022;8:e2100376.
Mathur P, Sathishkumar K, Chaturvedi M, Das P, Sudarshan KL, Santhappan S, Nallasamy V, et al. Cancer statistics, 2020: report from national cancer registry programme, India. JCO Global Oncol. 2020;6:1063–75.
Moher D, Liberati A, Tetzlaff J, Altman DG, PRISMA Group. Preferred reporting items for systematic reviews and meta-analyses: the PRISMA statement. Ann Intern Med. 2009;151(4):264–9.
Murray J, Tree AC. Prostate cancer–advantages and disadvantages of MR-guided RT. Clin Transl Radiat Oncol. 2019;18:68–73.
Ni Y, Wang Ke, Zhao L. A Markov decision process model of allocating emergency medical resource among multi-priority injuries. Int J Math Oper Res. 2017;10(1):1–17.
Oh S-H, Lee SJ, Noh J, Mo J. Optimal treatment recommendations for diabetes patients using the Markov decision process along with the South Korean electronic health records. Sci Rep. 2021;11(1):6920.
Ortega-Calvo AS, Morcillo-Jimenez R, Fernandez-Basso C, Gutiérrez-Batista K, Vila M-A, Martin-Bautista MJ. AIMDP: an artificial intelligence modern data platform. Use case for Spanish national health service data silo. Futur Gener Comput Syst. 2023;143:248–64.
Osheroff JA, Teich JM, Middleton B, Steen EB, Wright A, Detmer DE. A roadmap for national action on clinical decision support. J Am Med Inform Assoc. 2007;14(2):141–5.
Pointreau Y, Garaud P, Chapet S, Sire C, Tuchais C, Tortochaux J, Faivre S, Guerrif S, Alfonsi M, Calais G. Randomized trial of induction chemotherapy with cisplatin and 5-fluorouracil with or without docetaxel for larynx preservation. J Natl Cancer Inst. 2009;101(7):498–506. https://doi.org/10.1093/jnci/djp007 .
Pushpendra S, Tripathi S, Gupta S. A unified approach for optimal dose delivery and trajectory optimization for the treatment of prostate cancer. Biomed Signal Process Control. 2021;69:102884.
Pushpendra S, Mishra A, Mishra SK. A comprehensive analysis of the challenges and potential side effects of radiation therapy for palliative cancer treatment. Méd Palliat. 2024. https://doi.org/10.1016/j.medpal.2023.12.002 .
Puterman ML. Markov decision processes: discrete stochastic dynamic programming. John Wiley Sons. 1994. https://doi.org/10.1002/9780470316887 .
Saketkoo LA, Russell AM, Jensen K, Mandizha J, Tavee J, Newton J, Rivera F, Howie M, Reese R, Goodman M, Hart P. Health-related quality of life (HRQoL) in sarcoidosis: diagnosis, management, and health outcomes. Diagnostics. 2021;11(6):1089.
Saure A, Patrick J, Tyldesley S, Puterman ML. Dynamic multi-appointment patient scheduling for radiation therapy. Eur J Oper Res. 2012;223(2):573–84. https://doi.org/10.1016/j.ejor.2012.06.046 .
Schaefer AJ, Bailey MD, Shechter SM, Roberts MS. Modeling medical treatment using Markov decision processes. In: Operations research and health care. Boston, MA: Springer; 2004. pp. 593–612. https://doi.org/10.1007/1-4020-8066-2_23 .
Schell GJ, Marrero WJ, Lavieri MS, Sussman JB, Hayward RA. Data-driven Markov decision process approximations for personalized hypertension treatment planning. MDM Policy Pract. 2016;1(1):2381468316674214.
Sher DJ, Fidler MJ, Liptay MJ, Koshy M. Comparative effectiveness of neoadjuvant chemoradiotherapy versus chemotherapy alone followed by surgery for patients with stage IIIA non-small cell lung cancer. Lung Cancer. 2015;88(3):267–74. https://doi.org/10.1016/j.lungcan.2015.03.015 .
Shi J, Alagoz O, Erenay FS, Qiang Su. A survey of optimization models on cancer chemotherapy treatment planning. Ann Oper Res. 2014;221:331–56.
Shields GE, Elvidge J. Challenges in synthesising cost-effectiveness estimates. Syst Rev. 2020;9(1):1–7.
Singh P, Tripathi S, Tamrakar RK. Dose-volume constraints based inverse treatment planning for optimizing the delivery of radiation therapy. Gedrag Organisatie Rev. 2020a;33(3):1049–58.
Singh P, Tripathi S, Tamrakar RK. Fluence map optimisation for prostate cancer intensity modulated radiotherapy planning using iterative solution method. Polish J Med Phys Eng. 2020b;26(4):201–9.
Sjoquist KM, Burmeister BH, Mark Smithers B, Zalcberg JR, John Simes R, Barbour A, Gebski V. Survival after neoadjuvant chemotherapy or chemoradiotherapy for resectable oesophageal carcinoma: an updated meta-analysis. Lancet Oncol. 2011;12(7):681–92. https://doi.org/10.1016/S1470-2045(11)70142-5 .
Tan AC, Ashley DM, López GY, Malinzak M, Friedman HS, Khasraw M. Management of glioblastoma: state of the art and future directions. CA: A Cancer J Clin. 2020;70(4):299–312.
Tcheng JE, Bakken S, Bates DW. Optimizing strategies for clinical decision support: summary of a meeting series. In: National Academy of medicine; the learning health system series. Washington (DC): National Academies Press (US); 2017. https://doi.org/10.17226/27122 .
Thomas M, Rübe C, Hoffknecht P, Macha HN, Freitag L, Linder A, Willich N. Effect of preoperative chemoradiation in addition to preoperative chemotherapy: a randomised trial in stage III non-small-cell lung cancer. Lancet Oncol. 2008;9(7):636–48. https://doi.org/10.1016/S1470-2045(08)70156-6 .
Tsoukalas A, Albertson T, Tagkopoulos I. From data to optimal decision making: a data-driven, probabilistic machine learning approach to decision support for patients with sepsis. JMIR Med Inform. 2015;3(1):e3445.
Tunc S, Alagoz O, Burnside E. Opportunities for operations research in medical decision making. IEEE Intell Syst. 2014;29(3):59.
Urschel JD, Vasan H. A meta-analysis of randomized controlled trials that compared neoadjuvant chemoradiation and surgery to surgery alone for resectable esophageal cancer. Am J Surg. 2003;185(6):538–43. https://doi.org/10.1016/S0002-9610(03)00066-7 .
Valenca LB, Sweeney CJ, Pomerantz MM. Sequencing current therapies in the treatment of metastatic prostate cancer. Cancer Treat Rev. 2015;41(4):332–40. https://doi.org/10.1016/j.ctrv.2015.02.010 .
Van A, Kyle J, Chow EKH, James NT, Orandi BJ, Ellison TA, Smith JM, Colombani PM, Segev DL. Choosing the order of deceased donor and living donor kidney transplantation in pediatric recipients: a Markov decision process model. Transplantation. 2015;99(2):360. https://doi.org/10.1097/TP.0000000000000588 .
Varni JW, Limbers CA, Burwinkle TM. How young can children reliably and validly self-report their health-related quality of life?: an analysis of 8,591 children across age subgroups with the PedsQL™ 4.0 Generic Core Scales. Health Qual Life Outcomes. 2007;5(1):1–13.
Vozikis A, Goulionis JE, Benos VK. The partially observable Markov decision processes in healthcare: an application to patients with ischemic heart disease (IHD). Oper Res Int Journal. 2012;12(1):3–14. https://doi.org/10.1007/s12351-010-0095-x .
Wang Z, Li H, Guan Y. Machine learning for cancer drug combination. Clin Pharmacol Ther. 2020;107(4):749.
Weaver DT. Novel approaches for optimal therapy design in drug-resistant populations. PhD thesis, Department of Nutrition Systems Biology and Bioinformatics. Cleveland, OH: Case Western Reserve University; 2023.
Wu C, Jarrett AM, Zhou Z, Elshafeey N, Adrada BE, Candelaria RP, Mohamed RM, Boge M, Huo L, White JB, Tripathy D. MRI-based digital models forecast patient-specific treatment responses to neoadjuvant chemotherapy in triple-negative breast cancer. Can Res. 2022;82(18):3394–404.
Yan L, Shen J, Wang J, Yang X, Dong S, Saijun Lu. Nanoparticle-based drug delivery system: a patient-friendly chemotherapy for oncology. Dose-Response. 2020;18(3):1559325820936161.
Youn S, Neil Geismar H, Pinedo M. Planning and scheduling in healthcare for better care coordination: current understanding, trending topics, and future opportunities. Prod Oper Manag. 2022;31(12):4407–23.
Yu C, Liu J, Nemati S, Yin G. Reinforcement learning in healthcare: a survey. ACM Comput Surv (CSUR). 2021;55(1):1–36.
Zhang S, Suen S-C, Gong CL, Pham J, Trebicka J, Duvoux C, Klein AS, Tiffany Wu, Jalan R, Sundaram V. Early transplantation maximizes survival in severe acute-on-chronic liver failure: results of a Markov decision process model. JHEP Rep (HEP Reports). 2021;3(6):100367. https://doi.org/10.1016/j.jhepr.2021.100367 .
Zhao L, Li J, Bai C, Nie Y, Lin G. Multi-modality treatment for patients with metastatic gastric cancer: a real-world study in China. Front Oncol. 2019a;9:1155.
Zhao Z, Zheng L, Chen W, Weng W, Song J, Ji J. Delivery strategies of cancer immunotherapy: recent advances and future perspectives. J Hematol Oncol. 2019b;12(1):126.
Download references
Author information
Alka Mishra & Santosh Kumar Mishra
Present address: Bhilai Institute of Technology, Durg, Chhattisgarh, India
Seema Singh
Present address: Shri Shankaracharya Group of Institutions: Shri Shankaracharya Technical Campus Bhilai, Chhattisgarh, India
Authors and Affiliations
Bhilai Institute of Technology, Durg, Chhattisgarh, India
Pushpendra Singh
You can also search for this author in PubMed Google Scholar
Corresponding author
Correspondence to Seema Singh .
Ethics declarations
Conflict of interest.
The authors declare no competing interests.
Additional information
Publisher's note.
Springer Nature remains neutral with regard to jurisdictional claims in published maps and institutional affiliations.
Rights and permissions
Springer Nature or its licensor (e.g. a society or other partner) holds exclusive rights to this article under a publishing agreement with the author(s) or other rightsholder(s); author self-archiving of the accepted manuscript version of this article is solely governed by the terms of such publishing agreement and applicable law.
Reprints and permissions
About this article
Singh, P., Singh, S., Mishra, A. et al. Multimodality treatment planning using the Markov decision process: a comprehensive study of applications and challenges. Res. Biomed. Eng. (2024). https://doi.org/10.1007/s42600-024-00349-4
Download citation
Received : 19 July 2023
Accepted : 02 April 2024
Published : 28 May 2024
DOI : https://doi.org/10.1007/s42600-024-00349-4
Share this article
Anyone you share the following link with will be able to read this content:
Sorry, a shareable link is not currently available for this article.
Provided by the Springer Nature SharedIt content-sharing initiative
- Multimodality treatment planning
- Markov decision process
- Cancer therapy
- Find a journal
- Publish with us
- Track your research
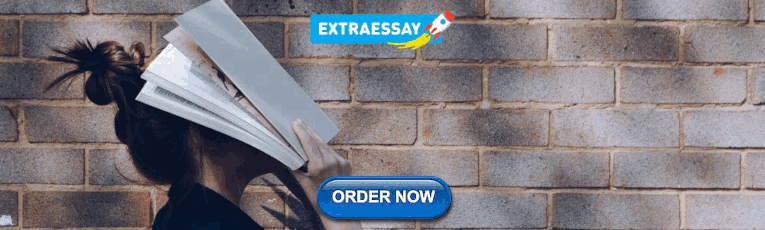
IMAGES
VIDEO
COMMENTS
Table of contents. Step 1: Consider your aims and approach. Step 2: Choose a type of research design. Step 3: Identify your population and sampling method. Step 4: Choose your data collection methods. Step 5: Plan your data collection procedures. Step 6: Decide on your data analysis strategies.
Step 1: Consider your aims and approach. Step 2: Choose a type of research design. Step 3: Identify your population and sampling method. Step 4: Choose your data collection methods. Step 5: Plan your data collection procedures. Step 6: Decide on your data analysis strategies. Other interesting articles.
Clinical research can be completed in two major steps: study designing and study reporting. Three study designs should be planned in sequence and iterated until properly refined: theoretical design, data collection design, and statistical analysis design. ... While the theoretical design is a crucial step in research planning, it is often dealt ...
Step 4: Create a research design. The research design is a practical framework for answering your research questions. It involves making decisions about the type of data you need, the methods you'll use to collect and analyze it, and the location and timescale of your research. There are often many possible paths you can take to answering ...
While many books and articles guide various qualitative research methods and analyses, there is currently no concise resource that explains and differentiates among the most common qualitative approaches. We believe novice qualitative researchers, students planning the design of a qualitative study or taking an introductory qualitative research course, and faculty teaching such courses can ...
• Describe the steps of the research process and key components of designing a study. • Explain that the conceptual phase, including a conceptual or theoretical framework, underpins and organizes any research study, quantitative or qualitative. • Recognize that the study design and methods are further determined by the problem
Introduction. In clinical research, our aim is to design a study, which would be able to derive a valid and meaningful scientific conclusion using appropriate statistical methods that can be translated to the "real world" setting. 1 Before choosing a study design, one must establish aims and objectives of the study, and choose an appropriate target population that is most representative of ...
The steps of a research study include a number of elements which are described starting from generating a research idea to final publication. The journey of good clinical research goes through a systematic circle of generating a research idea and framing a research question, thorough literature review, designing the study, writing the research protocol and getting ethics approval, the actual ...
Abstract. In this chapter the key issues that have to be addressed when planning a research study are discussed. Planning a literature search and the key methodologies to consider are presented. The examples are applicable to both diagnostic and therapeutic practice. Insight into good practice measures when planning a research study is addressed.
Research design is the process to deliberately plan for your research. As mentioned before, identifying the key concepts related to the research problems is the initial step to success. Once the key concepts are identified and mapped to highlight the potential relationships, a literature review is imperative.
Planning Research. Figure 6. The Research Design Process. This diagram (taken from an archived Open University (UK) course entitled E891 Educational Inquiry) shows one way to schematise the research design process. Here, one begins with a research question and a context for the research (comprising policy and practice).
Table of contents. Step 1: Define your variables. Step 2: Write your hypothesis. Step 3: Design your experimental treatments. Step 4: Assign your subjects to treatment groups. Step 5: Measure your dependent variable. Other interesting articles. Frequently asked questions about experiments.
Clinical research can be completed in two major steps: study designing and study reporting. Three study designs should be planned in sequence and iterated until properly refined: theoretical design, data collection design, and statistical analysis design. The design of data collection could be further categorized into three facets: experimental ...
Research Design*. There are 10 steps to consider in any research study. These steps can be used to perform a critical appraisal of a published paper, or to design your study in the first place. The use of a simple system such as this can ensure that biases in study design are avoided and that an appropriate study is ….
It will form the foundations on which to design a research study that has the potential to deliver clinically useful results. As such, posing a well-considered research question is the first vital step in planning one's research. It is worthwhile looking at some well-conducted studies in the literature as an example of what a good research ...
Objective: To discuss common pitfalls and useful tips in designing a quantitative research study, the importance and process of ethical approval, and consideration of funding. Conclusions: Through careful planning, based on formulation of a research question, early career researchers can design and conduct quantitative research projects within the framework of the Scholarly Project or in their ...
Practical Research: A Basic Guide to Planning, Doing, and Writing. 2 Considerations in Designing Your Research Approach ... the next step is to design your study. This chapter will provide a basic overview of the considerations any researcher must think about as they design a research study. ...
A research design is a blueprint for the collection, measurement, and analysis of the data used to answer the stated research question. It should be economical and reflect complex research planning decisions that require compromises and trade-offs among the demands of resources, time, quality, and data access.
Planning and Designing research projects (The research. process) Introduction. This chapter explains the steps involved in conducting research projects. The. research process can be divided into ...
demiological study. Only general issues are covered. Each research project and each study setting presents unique problems which cannot be dealt with here. The first step in the design of any epidemiological study is to have an 'idea' for a study. This is a creative process for which no guidelines or advice can be given. The idea for a ...
The practical steps needed for planning and conducting an experiment include: recognizing the goal of the experiment, choice of factors, choice of response, choice of the design, analysis and then drawing conclusions. This pretty much covers the steps involved in the scientific method. What this course will deal with primarily is the choice of ...
The best way to accomplish any business or personal goal is to write out every possible step it takes to achieve the goal. Then, order those steps by what needs to happen first. Some steps may ...
The purpose of this article is to provide a broad overview of important decisions in planning a research study. The research process is reviewed, including the conceptual phase, the design and planning phase, the empirical phase, the analytic phase, and the dissemination phase. Issues unique to diabetes education research are highlighted.
Starting an online business can be a powerful way to launch a new product or service while reaching a wider audience. With market research, a solid business plan, a strong website, and a digital ...
This protocol must be approved by an Institutional Review Board (IRB) before the research starts off. The research protocol is a formal written document specifying the study design and the manner in which it will be conducted. It is the blueprint of the study and serves as a guideline throughout the implementation and analysis phases.
Download the Senior Leadership Success Plan Case Study Here. A global leader in design and manufacturing asked Clarkston Consulting to evaluate their strategic business needs and make recommendations for executive succession in conjunction with an enterprise strategy development project. The company required several aspects for its succession ...
Abstract. Public participation in heritage conservation is an area that lacks proper investigation in the urban planning and design discipline. To address this gap, this paper presents an empirical study of participatory design methods promoting social interaction in Liuzhi, a historical community with cultural and historical relics in rural China.
The lesson plan templates below are designed especially for preschool and kindergarten using colors and fun design elements. Kindergarten teachers can use these lesson plans to communicate key skills, concepts and topics to both parents and kids. Template #1: Illustrated Lesson Plan. This lesson plan template has a simple design with light colors.
Purpose According to the oncologist, a single medication is insufficient to completely cure the disease; as a result, most patients undergo treatment from two or more types of therapy, sometimes in succession, in order to control the progression of their cancer. Methods This comprehensive review explores the applications and challenges associated with multimodality treatment planning utilizing ...