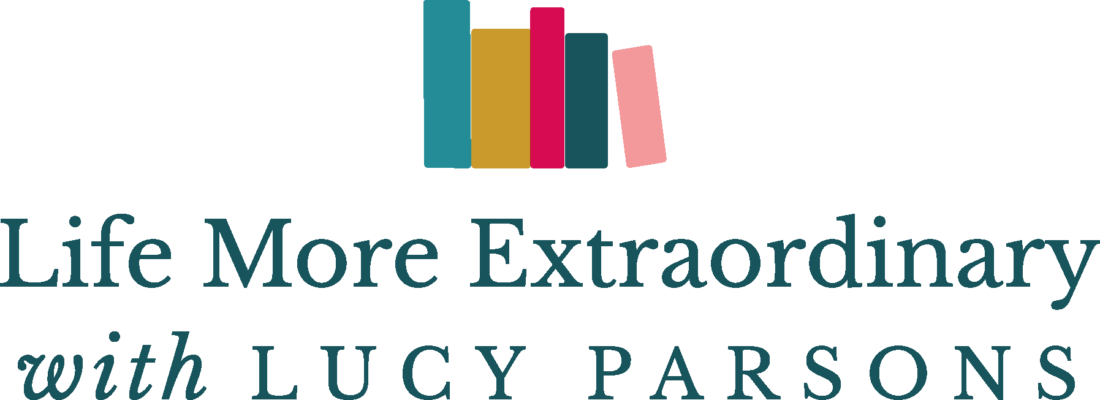
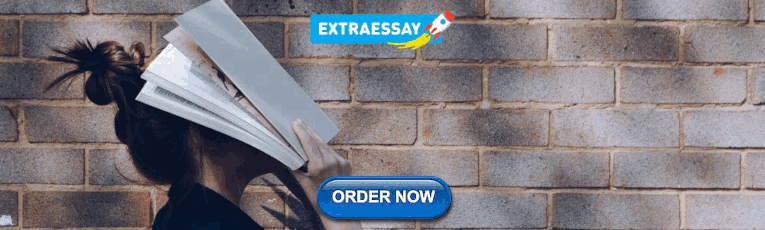
How to revise geography case studies
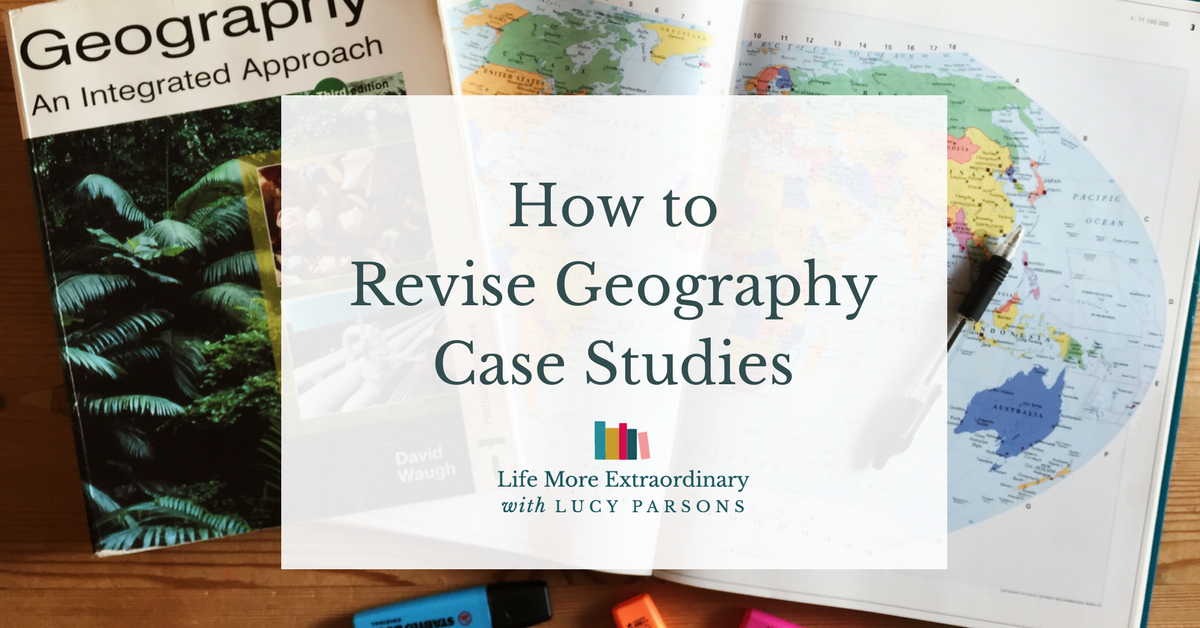
Molly, one of my readers, wrote to me to ask:
I was just wondering if you had any tips for revising and remembering geography case studies?
When I've asked around some of the things that students find most difficult about revising geography case studies are:
- Condensing all the information
- Remembering all the statistics
- Knowing what you need to know, and what you can afford to forget
Having been a bit of an ace at this kind of thing myself (I got an A* at GCSE, A at A-Level and a degree in the subject) I thought I'd share some of my top tips on how to revise geography case studies today.
1. Make sure you understand the case study
The first step in remembering anything is understanding it. You need to have a clear model in your mind of how the case study works. This includes how it's laid out in space (a mental map), who the people were who were involved and the context of the case study (historical, political, social, economic and environmental. These tips will help you with this:
- Make sure you've seen a map of the place. In this day and age this is easy with google maps, google earth and google streetview. All of these things can help you understand both the 2-D and 3-D landscape of the case study.
- Find newspaper articles and pictures to give you some background and also help you to visual the place
- Watch videos if they exist. For some case studies there are amazing clips of films (Kibera, the Nairobi shanty town at the beginning of The Constant Gardner springs to mind). For others there will be great video clips on YouTube to help you.
- If you can, visit the place. Nothing is as powerful as this in fully understanding a place.
2. Condense your notes
Once you've thoroughly understood the case study it's time to condense your notes. There are various ways you can do this.
- Create an A3 annotated map of the area. Colour code things like causes and effects or social, economic, environmental and political factors. Have a key. You can even have flaps. Stick the map up on the wall and look at it frequently. The great thing about this is that the finite size of the page forces you to condense the information.
- Create a table. You could put things like the social, economic, political and environmental factors along one side and background, causes and effects along the other. Inside The Extraordinaries Club I have some grids and guidelines for you to download and use. These are exclusively for members. Find out more about the club here .
- Create index cards. This was one of my favourites as it was quite a kinaesthetic way of separating the information into bite-sized chunks. It was a great format to give other people to test me so that I could learn all those facts and figures. You can even have different coloured index cards for different topics.
- Traditional revision notes . In my opinion this is a bit boring, and can also be a bit intimidating when you see reams of notes that you have to memorise. I'd go with one of the other options if I was you.
Download a free extract of my book, The Ten Step Guide to Acing Every Exam You Ever Take , to find out how you learn best. Enter your details in the box below to get your extract.
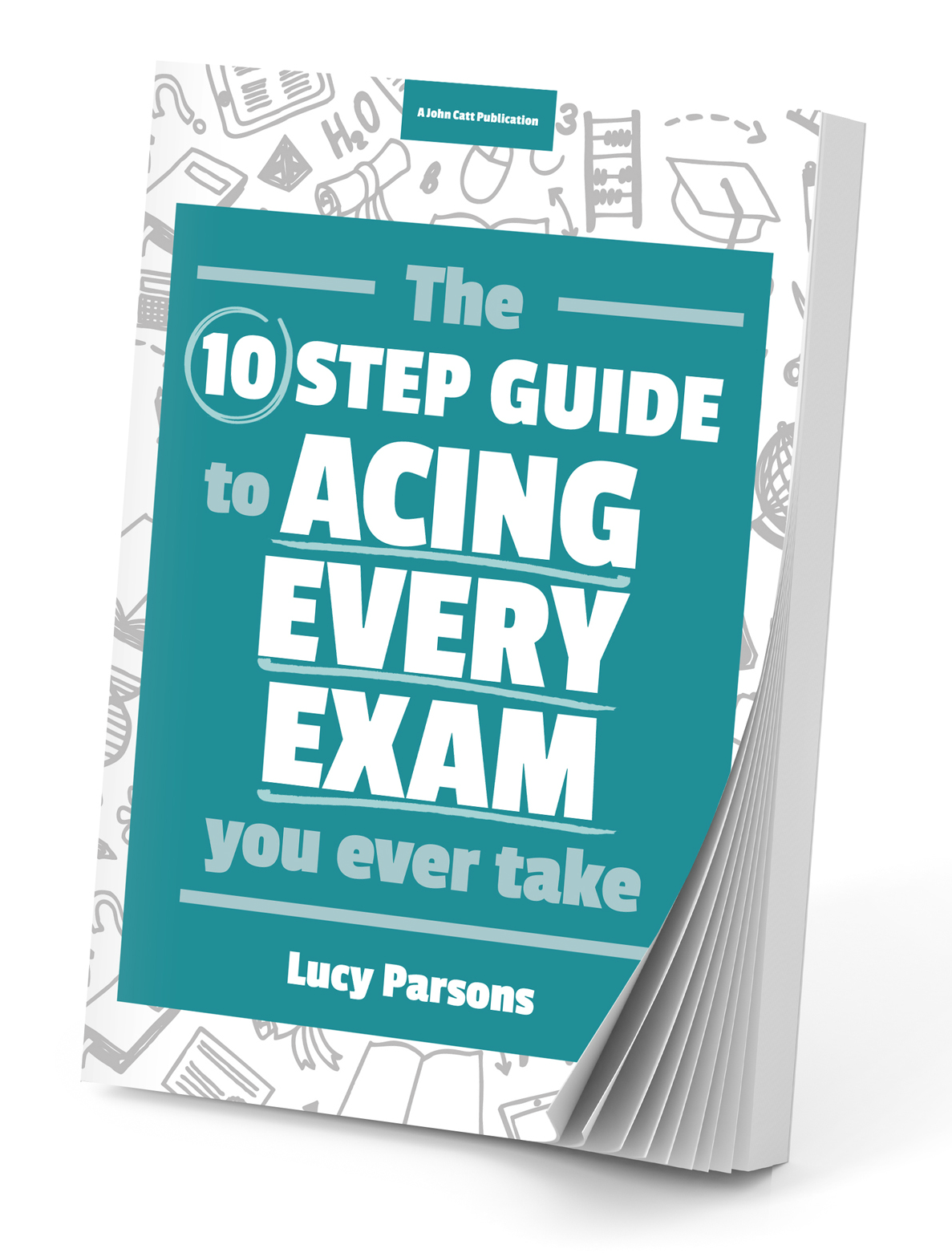
DOWNLOAD YOUR FREE CHAPTER
Of the ten step guide to acing every exam you ever take.
Identify how YOU learn best and make your independent study as successful as it possibly can be
Your personal data will be treated with respect. View my privacy policy here .
3. Memorise
Now you've condensed your class notes you need to memorise them. Good memorisation, in my experience comes down to two things:
- Using the information in different formats.
I'd advise you to do a combination of the following:
- Read index cards out loud, cover and test yourself.
- Get other people to test you.
- Act it out.
- Make up songs or rhymes
- Whatever else works for you…
4. Teach someone else about the case study
Teaching someone else is one of the best ways there is to a) check your understanding (because they'll never understand it if you don't) and b) practice putting what you know into words so that someone else understands it.
5. Do Past Papers
The final step is to do past papers. I strongly recommend that you do this in the format of Revision Power Hours.
If you do power hours, and make a point of marking your work, you'll not only do lots of repetition of the case study you've been learning, you'll start to learn to think like an examiner and also get a brilliant insight into exactly what they expect you to know in terms of facts and statistics.
I will say this. I used to remember literally hundreds of stats for my case studies. When I became a teacher it surprised me how few students actually needed to know in order to get good marks. However, this comes with a word of warning. You need to have a good insight into what your exam board expects you to know.
Over to you
That's pretty much a masterclass in how to revise geography case studies. Now it's up to you to put it into practice
In the comments below I'd love to know:
- What you're finding difficult about revising geography case studies
- What other subjects you need revision help with
Need more help with your revision?
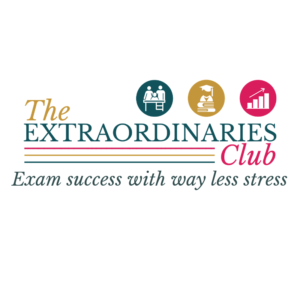
It works because you develop a personalised study system tailored to the way you work, what motivates you and your ambitions.
Exam success with way less stress.
You might also like...
Why your revision isn’t working
Why you need to make past paper practise part of your revision
3 Exam Technique Mistakes GCSE and A-Level Students Commonly Make
How to revise GCSE English with 7 weeks to go
“Is it too late for revision to make a difference for this year’s GCSEs and A-Levels?”
Why finding your study style is like finding your fashion sense
Lucy Parsons
Click here to leave a comment below, leave a reply:.
Save my name, email, and website in this browser for the next time I comment.
On a mission to end educational inequality for young people everywhere.
ZNotes Education Limited is incorporated and registered in England and Wales, under Registration number: 12520980 whose Registered office is at: Docklands Lodge Business Centre, 244 Poplar High Street, London, E14 0BB. “ZNotes” and the ZNotes logo are trademarks of ZNotes Education Limited (registration UK00003478331).
geographycasestudy.com Reviews
What is your feeling about geographycasestudy.com , geographycasestudy.com has an average to good trust score. why, consumer reviews about geographycasestudy.com, be the first one to review, advertorials, complete review geographycasestudy.com, company evaluation, webshop evaluation, technical evaluation, facts about geographycasestudy.com, popular stories, how to recognize a scam website, how do i get money back from a scammer, website alerts, help & info, scam trends, subscribe to our weekly newsletter.
- Free Samples
- Premium Essays
- Editing Services Editing Proofreading Rewriting
- Extra Tools Essay Topic Generator Thesis Generator Citation Generator GPA Calculator Study Guides Donate Paper
- Essay Writing Help
- About Us About Us Testimonials FAQ
- Geography Case Study
- Samples List
An case study examples on geography is a prosaic composition of a small volume and free composition, expressing individual impressions and thoughts on a specific occasion or issue and obviously not claiming a definitive or exhaustive interpretation of the subject.
Some signs of geography case study:
- the presence of a specific topic or question. A work devoted to the analysis of a wide range of problems in biology, by definition, cannot be performed in the genre of geography case study topic.
- The case study expresses individual impressions and thoughts on a specific occasion or issue, in this case, on geography and does not knowingly pretend to a definitive or exhaustive interpretation of the subject.
- As a rule, an essay suggests a new, subjectively colored word about something, such a work may have a philosophical, historical, biographical, journalistic, literary, critical, popular scientific or purely fiction character.
- in the content of an case study samples on geography , first of all, the author’s personality is assessed - his worldview, thoughts and feelings.
The goal of an case study in geography is to develop such skills as independent creative thinking and writing out your own thoughts.
Writing an case study is extremely useful, because it allows the author to learn to clearly and correctly formulate thoughts, structure information, use basic concepts, highlight causal relationships, illustrate experience with relevant examples, and substantiate his conclusions.
- Studentshare
Examples List on Geography Case Study
- TERMS & CONDITIONS
- PRIVACY POLICY
- COOKIES POLICY
- Find Flashcards
- Why It Works
- Tutors & resellers
- Content partnerships
- Teachers & professors
- Employee training
Brainscape's Knowledge Genome TM
- Entrance Exams
- Professional Certifications
- Foreign Languages
- Medical & Nursing
- Humanities & Social Studies
- Mathematics
- Health & Fitness
- Business & Finance
- Technology & Engineering
- Food & Beverage
- Random Knowledge
See full index
Learn geography case studies.

World Geography
1,050+ Adaptive world geography flashcards online for leveling up your knowledge of the world.

U.S. Geography
Master U.S. Geography with hundreds of the world's smartest mobile & web flashcards.
A-Level Geography Case Studies
By: james crowther, geography case studies., by: james atherton, gcse geography case studies, by: meg daniel.

AQA A Level Geography
By: aurelia nadal.

z - geography - case study
By: orna tighe, gcse geography case studies, by: vanshika chilukuri, case studies- geography gcse, by: emma larner, ib geography - case studies, by: ewa dun, geography case studies, by: ri downing, by: noah williams, by: deleted deleted.
Geography: Case Studies
By: elliot eadie, geography- case studies, by: ruby willis, geography case studies tectonic hazards, by: maciej zagdan, igcse geography case studies, by: isha rajadhyax, by: chris katsikaris, a level geography - case studies, by: charlie paterson, geography case studies, by: user unknown, aqa gcse geography case studies, by: aneesh punage, by: emma frost.
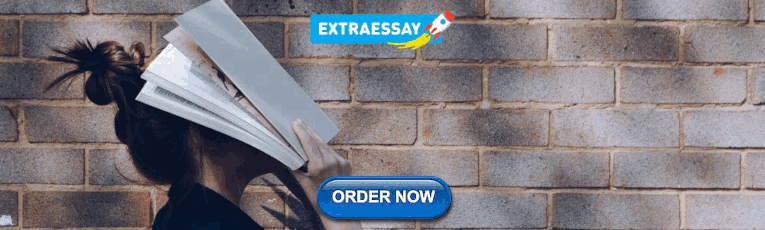
Z AQA GCSE Geography Case Study’s
By: simone laird, by: emma payne.
Geography Smith = Hazards = Seismicity + Case Study 🌋
By: sarah johnson, human geography case studies, by: caitlin o'regan, aa//geography case studies, by: abby marriott, by: heidi osmer, ce geography case study, geography - lagos and case studies, by: matthew humphreys.

A-Level Geography: Case Studies
By: callum haynes.
Geography Weston = Urban Policy - Case Studies 😑
Knowledge genome.
- Corporate Training
- Teachers & Schools
- Android App
- Help Center
- Law Education
- All Subjects A-Z
- All Certified Classes
- Earn Money!
Loading metrics
Open Access
Peer-reviewed
Research Article
Species distribution modeling for disease ecology: A multi-scale case study for schistosomiasis host snails in Brazil
Roles Conceptualization, Data curation, Formal analysis, Investigation, Methodology, Validation, Visualization, Writing – original draft, Writing – review & editing
* E-mail: [email protected]
Affiliation Emmett Interdisciplinary Program in Environment and Resources, Stanford University, Stanford, California, United States of America

Roles Conceptualization, Data curation, Methodology, Supervision, Validation, Writing – review & editing
Affiliations Department of Biology, Stanford University, Stanford, California, United States of America, Institute for Human-centered Artificial Intelligence, Stanford University, Stanford, California, United States of America
Roles Conceptualization, Validation, Writing – review & editing
Affiliation Department of Oceans, Hopkins Marine Station, Stanford University, Pacific Grove, California, United States of America
Roles Conceptualization, Data curation, Funding acquisition, Project administration, Resources, Supervision, Validation, Writing – review & editing
Affiliation Pasteur Institute, São Paulo, Brazil
Roles Conceptualization, Data curation, Resources, Validation, Writing – review & editing
Roles Data curation, Funding acquisition, Project administration
Roles Data curation, Funding acquisition, Project administration, Resources, Supervision, Validation, Writing – review & editing
Affiliation Fiocruz Minas/Belo Horizonte-Minas Gerais, Belo Horizonte, Brazil
Roles Data curation, Funding acquisition, Project administration, Resources
Roles Conceptualization, Funding acquisition, Methodology, Project administration, Resources, Supervision, Validation, Writing – review & editing
Affiliation Geoinformation & Earth Observation Division, National Institute for Space Research (INPE), São Paulo, Brazil
Roles Conceptualization, Methodology, Writing – review & editing
Affiliations Department of Biology, Stanford University, Stanford, California, United States of America, Harvard Medical School, Boston, Massachusetts, United States of America
Roles Funding acquisition, Project administration
Affiliations Department of Oceans, Hopkins Marine Station, Stanford University, Pacific Grove, California, United States of America, Marine Science Institute, University of California Santa Barbara, Santa Barbara, California, United States of America
Roles Conceptualization, Formal analysis, Resources, Supervision, Validation, Writing – review & editing
Affiliations Department of Biology, Stanford University, Stanford, California, United States of America, Woods Institute for the Environment, Stanford University, Stanford, California, United States of America
Roles Conceptualization, Funding acquisition, Project administration, Resources, Supervision, Validation, Writing – review & editing
Affiliations Department of Oceans, Hopkins Marine Station, Stanford University, Pacific Grove, California, United States of America, Woods Institute for the Environment, Stanford University, Stanford, California, United States of America
- Alyson L. Singleton,
- Caroline K. Glidden,
- Andrew J. Chamberlin,
- Roseli Tuan,
- Raquel G. S. Palasio,
- Adriano Pinter,
- Roberta L. Caldeira,
- Cristiane L. F. Mendonça,
- Omar S. Carvalho,
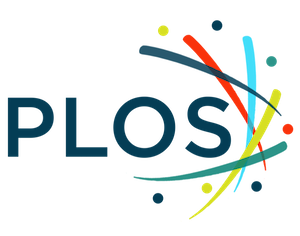
- Published: August 2, 2024
- https://doi.org/10.1371/journal.pgph.0002224
- Reader Comments
Species distribution models (SDMs) are increasingly popular tools for profiling disease risk in ecology, particularly for infectious diseases of public health importance that include an obligate non-human host in their transmission cycle. SDMs can create high-resolution maps of host distribution across geographical scales, reflecting baseline risk of disease. However, as SDM computational methods have rapidly expanded, there are many outstanding methodological questions. Here we address key questions about SDM application, using schistosomiasis risk in Brazil as a case study. Schistosomiasis is transmitted to humans through contact with the free-living infectious stage of Schistosoma spp. parasites released from freshwater snails, the parasite’s obligate intermediate hosts. In this study, we compared snail SDM performance across machine learning (ML) approaches (MaxEnt, Random Forest, and Boosted Regression Trees), geographic extents (national, regional, and state), types of presence data (expert-collected and publicly-available), and snail species ( Biomphalaria glabrata , B . straminea , and B . tenagophila ). We used high-resolution (1km) climate, hydrology, land-use/land-cover (LULC), and soil property data to describe the snails’ ecological niche and evaluated models on multiple criteria. Although all ML approaches produced comparable spatially cross-validated performance metrics, their suitability maps showed major qualitative differences that required validation based on local expert knowledge. Additionally, our findings revealed varying importance of LULC and bioclimatic variables for different snail species at different spatial scales. Finally, we found that models using publicly-available data predicted snail distribution with comparable AUC values to models using expert-collected data. This work serves as an instructional guide to SDM methods that can be applied to a range of vector-borne and zoonotic diseases. In addition, it advances our understanding of the relevant environment and bioclimatic determinants of schistosomiasis risk in Brazil.
Citation: Singleton AL, Glidden CK, Chamberlin AJ, Tuan R, Palasio RGS, Pinter A, et al. (2024) Species distribution modeling for disease ecology: A multi-scale case study for schistosomiasis host snails in Brazil. PLOS Glob Public Health 4(8): e0002224. https://doi.org/10.1371/journal.pgph.0002224
Editor: Kamran Shaukat Dar, The University of Newcastle, AUSTRALIA
Received: July 6, 2023; Accepted: July 17, 2024; Published: August 2, 2024
Copyright: © 2024 Singleton et al. This is an open access article distributed under the terms of the Creative Commons Attribution License , which permits unrestricted use, distribution, and reproduction in any medium, provided the original author and source are credited.
Data Availability: All data and methods used in this analysis are publicly available and can be found at https://github.com/alyson-singleton/sdm-disease-ecology-multi-scale .
Funding: This work was supported by the Belmont collaborative Forum on Climate, Environment, and Health (NSF-ICER 2024383 and NSF-ICER 2024385) and by FAPESP (2019/23593-3) in a collaborative research action with Belmont Forum. GDL and EM are also supported by the Stanford Center for Innovation in Global Health. GDL is also partially supported by NSF-DEB DEB-2011179 (EEID). EM is also supported by grants from the National Science Foundation (DEB-2011147, with Fogarty International Center), the National Institutes of Health (R35GM133439, R01AI168097, R01AI102918), and the Woods Institute for the Environment. TA is supported by the National Institute of General Medical Sciences (T32GM144273). RP is supported by FAPESP (2021/10212-1). The authors received no specific funding for this work. The funders had no role in study design, data collection and analysis, decision to publish, or preparation of the manuscript.
Competing interests: The authors have declared that no competing interests exist.
Introduction
Species distribution models (SDMs) have become increasingly popular tools in the field of disease ecology to profile transmission risk for vector-borne, zoonotic diseases, and environmentally-mediated diseases, i.e., diseases whose transmission involves a non-human host or vector species, such as mosquitoes (malaria, dengue, Zika), flies (leishmaniasis, sleeping sickness), ticks (Lyme disease), triatomine bugs (Chagas disease), and snails (schistosomiasis, fascioliasis) [ 1 – 6 ]. By using presence data of non-human hosts and remotely-sensed data of potential environmental covariates, SDMs are correlative models that can predict species habitat suitability across areas not sampled by field collection programs [ 7 – 9 ]. These models are typically used to create high-resolution maps of an inferred species distribution across a geographic area of interest, which can reflect areas where disease transmission may be possible. In combination with other processes that influence transmission, such as additional reservoir host distributions or other disease exposure variables, these predictions can directly inform the understanding of the pathogenic landscape of environmentally-mediated diseases [ 10 ].
SDMs are a powerful tool applied in a number of fields, including disease ecology [ 11 , 12 ], epidemiology [ 13 ], and conservation [ 14 , 15 ], among many others. Species distribution modeling works by using species presence/absence data to identify covariates that are predictive of a species presence. Because true absence data are not typically available, SDMs often use “background” or “pseudo-absence” data to simulate locations where an organism could have been sampled but was not [ 16 , 17 ]. SDMs use various machine learning methods to identify a suite of covariates that can accurately predict the presence or absence of the organism in geographic space, using flexible functional relationships between predictors and responses that can include nonlinearities and interactions [ 8 , 9 ]. Model inputs can vary in spatial and temporal resolution and extent. Many algorithms are available for model training and testing, and they differ in how they handle covariate-outcome relationships [ 18 ]. SDMs are cross-validated by leaving out part of the data in model training in order to assess model performance on out-of-sample data, often performed in a spatially-structured way [ 19 ]. The outputs of interest include geographic maps of species presence suitability, lists of variables selected as important predictors, and the functional forms of relationships between predictors and presence. A glossary of terms and concepts central to the SDM literature are summarized for reference in Table 1 .
- PPT PowerPoint slide
- PNG larger image
- TIFF original image
https://doi.org/10.1371/journal.pgph.0002224.t001
Increased access to large-scale, remotely-sensed environmental data [ 20 , 21 ] and species presence databases [ 22 ], such as the Global Biodiversity Information Facility [ 23 ], has spurred rapid expansion of these methods. Further, recent decades have brought rapid development of statistical models and machine learning algorithms that can be applied to species distribution models, such as regularized regression [ 24 ], decision tree [ 25 ], Bayesian [ 26 ], neural network [ 27 ], and ensemble methods [ 28 ], among many others. Although many machine learning methods have grown in popularity due to their flexibility, ability to model covariate interactions, and increasing accessibility in common programming languages like R, no single method has fully eclipsed its counterparts [ 18 , 29 ]. Due to both their popularity in the literature and their consistently high performance, we chose three modeling methods to investigate in this study: Maximum Entropy (MaxEnt), Random Forest (RF), and Boosted Regression Tree (BRT) models [ 18 ]. MaxEnt—a regularized, regression-based model—has long been a well-established method for presence-only applications [ 30 ], while flexible, decision tree model types such as RF and BRT have gained more recent popularity [ 31 ]. Along with the expansion of model types, there has been additional SDM methodological development, including optimization of sampling techniques for “background” or “pseudo-absence” points [ 17 , 32 ], increased rigor for input variable selection [ 33 , 34 ], investigation on resolution size [ 35 ], defense of spatial cross-validation techniques [ 19 ], integration of ecological theory [ 36 , 37 ], development of gold-standard model evaluation measures [ 38 ], and updated guidelines for method-specific reproducibility standards [ 39 , 40 ].
Despite these advances, many of these new methods have not been recently documented, especially not in a cohesive, accessible manner for scientists new to SDMs or those interested in adopting new methods [ 31 ]. To our knowledge, there has not been an analysis comparing machine learning algorithms, data sources, and geographic extents in combination and assessing the consequences for presence probabilities and covariate relationships. We hypothesize that algorithm performance will vary across geographic scales given differences in model structure, such as ability to handle covariate interactions and potential to overfit [ 18 ]. Additionally, there are very few analyses that directly compare the effects of using GBIF presence records versus records from expert-executed field collection programs. Given the known spatial bias in GBIF data, we ask how well GBIF data can approximate predictions created from expert-collected data sources [ 51 , 52 ]. Finally, although there has been discussion on the effect of resolution size [ 35 ], there has been limited discussion on how SDM performance varies across areas of differing geographic extent when resolution size is held constant.
In an effort to answer these methodological questions in a biologically and epidemiologically relevant study system, we will use the intermediate hosts of Schistosoma mansoni Sambon, 1907— Biomphalaria Preston, 1910 snails—as a case study. Simultaneously, we will make substantial contributions to knowledge on predicting schistosomiasis risk in Brazil. Schistosomiasis is a debilitating parasitic disease caused, in Brazil, by S . mansoni , a parasite that relies on both freshwater Biomphalaria snails and human beings to complete its life cycle [ 53 ]. In Brazil, approximately 6 million people are infected and 25 million live in areas where they are at risk of infection [ 54 ]. The disease predominantly impacts poor communities dependent on open water sources for occupational activities or other components of daily life [ 55 , 56 ]. More recently, schistosomiasis transmission has also been recorded in urban and peri-urban areas, impacting people who are either without access to basic sanitation services or whose sewage systems overflow in times of heavy rainfall [ 57 , 58 ].
Because Biomphalaria freshwater snails are obligate intermediate hosts of S . mansoni parasites, SDMs of the non-human hosts of schistosomiasis allow us to predict areas of suitable snail habitat where transmission may be possible. There are three competent Biomphalaria snail hosts in Brazil: Biomphalaria glabrata Say, 1818, Biomphalaria straminea Dunker, 1848, and Biomphalaria tenagophila D’Orbigny, 1835. Because snails are ectotherms (i.e., their body temperature is dependent on their environment), their reproduction, survival, and dispersal are strongly affected by their surrounding temperature [ 59 ]. The snails live in slow-moving freshwater, including permanent and temporary sources, which are both influenced strongly by precipitation and drainage patterns [ 60 ]. Land-use and land-cover (LULC) characteristics affect snail presence through multiple pathways, including affecting temperatures through changes in tree canopy and vegetation cover and influencing water patterns through deforestation and agriculture [ 61 ]. Finally, chemical factors and soil properties—such as pH and soil water content—are known to impact the survival of Biomphalaria snails, due to their impact on freshwater quality [ 62 ].
SDMs capture the snails’ biological relationships to these environmental factors and build predictive risk maps that can help to target disease intervention efforts such as mass drug administration [ 63 ]. There have been multiple studies using SDMs to predict suitable snail habitat across multiple geographical scales in Brazil, from national [ 64 – 66 ] to sub-national analyses, including those specific to areas within Pernambuco [ 67 ], São Paulo [ 68 ], and Minas Gerais [ 69 , 70 ]. However, all of these analyses test only MaxEnt models, with the exception of Guimarães et al., 2009 who used an indicator kriging procedure [ 69 ]. Moreover, with the exception of Palasio et al., 2021, the quality and quantity of accessible, remotely-sensed environmental data has grown substantially since time of publication [ 68 ]. Finally, our group has collected a large dataset of presence records throughout Brazil that reflect best expert knowledge of the constraints on snail habitat, presenting an alternative to publicly available GBIF presence data. Therefore, Biomphalaria snails in Brazil provide a ripe opportunity to compare and contrast current methods on SDMs, providing a rare comparative case study to guide SDM approaches for disease ecology and contributing updated risk models that can guide Brazil’s schistosomiasis elimination efforts [ 71 ].
We compare multiple combinations of SDM methods—three machine learning algorithms, two data sources, and three geographic extents—and assess the consequences for suitability probabilities and covariate relationships of three snail species. We address the questions: How do statistical/machine learning models compare depending on research question or application of interest? How do model accuracy, variable importance, and geographic predictions vary across spatial scales? How does model performance compare using expert-collected data versus publicly-available data?
All data and methods used in this analysis are publicly available and can be found at https://github.com/alyson-singleton/sdm-disease-ecology-multi-scale .
Species data and background sampling
We acquired B . glabrata , B . straminea , and B . tenagophila presence data from two main sources (1) an ongoing, Brazil-wide field program supported by multiple government-funded groups across Brazil, including the Coleção de Malacologia Médica, Fundação Oswaldo Cruz (CMM-Fiocruz) and the Coordination for Disease Control of the State Health Secretariat of São Paulo (CCD-SP) [ 72 – 79 ] and (2) the Global Biodiversity Information Facility (GBIF), a database of publicly available presence records commonly used to build SDMs [ 22 ].
The Brazil-wide field collection program, hereafter referred to as the expert-collected dataset, consisted of 11,299 total snail records that spanned 1992–2019 and included 25 species. As part of national efforts to control schistosomiasis, the Brazilian Ministry of Health has approved routine collection and monitoring of Biomphalaria snail species. Geographical coordinates of each collection site were acquired with a Garmin eTrex GPS device and species identification was done using morphological and molecular tools. Prior to model input, all records were spatially filtered such that only one presence record was retained for each 1km grid cell (i.e. “thinned to 1km”) to minimize pseudo-replication and oversampling bias [ 43 ]. After each species was separately thinned to 1km, the dataset was reduced to 972 records of our snail hosts of interest: 305 B . glabrata , 396 B . straminea , and 271 B . tenagophila presence points ( Fig 1 , Table 2 ).
A) National, B) Minas Gerais, C) São Paulo. Maps were built in R (version 4.2.2) using shapefiles from the geobr package [ 80 ].
https://doi.org/10.1371/journal.pgph.0002224.g001
https://doi.org/10.1371/journal.pgph.0002224.t002
To compare model performance between expert-collected and publicly available GBIF data and to create a background dataset (described below), we constructed a GBIF dataset by searching Brazil for all species included in the expert-collected dataset and records of all freshwater animals found in South America, as defined by the International Union for Conservation of Nature [ 81 ]. This resulted in a total of 74,960 records that spanned 1985–2020, included over 2,000 species, and reduced to 193 records of our snail hosts of interest—29 B . glabrata , 28 B . straminea , and 136 B . tenagophila —post thinning. Our inclusion criteria for GBIF records were (i) year was between 1985–2020, (ii) latitude and longitude each included at least three decimal places and (iii) basis of record excluded “fossil specimen” and “machine observation” to ensure that the record was field-collected at the latitude and longitude reported and was identified by a human. For our snail hosts of interest we also required a complete species taxonomic identification. We limited our comparison of expert-collected versus GBIF data to B . tenagophila in São Paulo due to lack of sufficient data availability in other areas.
Given a lack of true absence data, we constructed a background dataset of freshwater animals across Brazil as our comparison group, thereby representing the freshwater landscape in which snails could plausibly be sampled. Species distribution models are often constructed using presence data only, without data on true absences of the species. To do so, models typically calculate the probability of species presence relative to a set of randomly-sampled background points across an area in which a species hypothetically could have been sampled but was not. Instead of random sampling, we use presences of ecologically similar species (i.e., freshwater animals) as “pseudo-absence” or “background” points to control for sampling effort and to capture the relationships with environmental covariates that distinguish the presence of the species of interest from that of others [ 16 ]. We would expect sampling efforts of freshwater animals to be similar for that of our species of interest. By constructing a background dataset of freshwater animals, we are better able to represent typical sampling practices of freshwater species in Brazil, rather than selecting background points randomly throughout the study area. Using background records means the model will predict whether or not a record is a presence (labeled as 1) or a background point (labeled as 0), rather than 0’s representing true absences. The extent of the background dataset should be also chosen to represent the environmental variation of the study area [ 16 ]. Our background dataset was a combination of (1) the remaining expert-collected data after excluding our three species of interest (4.8%) and (2) the publicly-available GBIF data described above (95.2%), which included a total of 2,091 freshwater animal species and 77,785 presence records. Each background dataset was built by sampling two times the number of presence data points for each model (i.e., a model with 100 presence points was given 200 background points): this ratio was selected to balance the sample between groups [ 82 ], while providing sufficient data to represent all environments and promote model convergence [ 17 , 83 ]. Background points were sampled without replacement across a probability distribution that maintained the frequency of background points per 1km grid cell. Therefore, we retained a maximum of one record per grid cell, generating a “background mask” that helped address sampling bias concerns [ 16 , 84 ].
Environmental data and multicollinearity analysis
We used high-resolution (1km) climate, hydrology, soil property, and land-use/land-cover (LULC) data to describe the environmental conditions associated with each species presence record and background sample. We limited the number of covariates to variables previously found to impact snail presence for ease of interpretation and comparison between model design choices [ 44 ]. Climate data were obtained from CHELSA (version 2.1), a high resolution (1km 2 ) global downscaled climate data set [ 85 ]. Four climatology variables, averaged over thirty years (1981–2010), were included in the analysis: temperature seasonality (bio4), mean temperature of coldest quarter (bio11), mean precipitation of wettest quarter (bio16), and mean precipitation of driest quarter (bio17). Hydrology data (height above nearest drainage—HND—and soil water percentage) were obtained from the Merit Hydro data [ 86 ] and OpenLandMap Soil Water Content [ 87 ], respectively, and soil property data (pH and clay) was obtained from OpenLandMap Soil pH in H 2 O [ 88 ] and OpenLandMap Clay Content [ 89 ], respectively. Because hydrology and soil variables were measured at finer spatial resolution than the climate data, we scaled them up to the maximum value (HND) or mean value (water content, pH, clay content) for each 1km 2 grid cell. Finally, our two LULC covariates—distance to high population density and proportion of temporary crop cover during the year of sampling—were constructed from WorldPop [ 90 ] and MapBiomas [ 91 ], respectively. High population density was defined as a 1km grid cell with a density of at least 1500 inhabitants per km 2 , per the World Bank definition [ 92 ]. Proportion of temporary crop cover was defined—in natural areas—as farming areas where it was not possible to distinguish between pasture and agriculture and—in urban areas—as areas of urban vegetation, including cultivated vegetation, natural forest, and non-forest vegetation [ 91 ]. We selected these two LULC variables based on our team’s on-the-ground knowledge of snail presence [ 93 ]. In total, we provided our models with 12 environmental covariates ( Fig A in S1 Text ), none of which had pairwise Pearson correlation coefficients above 0.7 with any of the other covariates [ 44 ]. Although our studied models can handle multicollinearity when calculating probabilities, collinear variables obscure the variable importance and partial dependence plot interpretation [ 44 ].
Geographic extent
To investigate model performance across varying geographic extent, we created models spanning national, regional (Sudeste, composed of four states: Espírito Santo, Minas Gerais, Rio de Janeiro and São Paulo), and state (Minas Gerais and São Paulo) extents in Brazil. The region and states of interest were chosen based on the quantity of data available to input into the models. Past studies have shown that model performance substantially declines with fewer than 30–50 presence records [ 94 , 95 ]. We selected only states with greater than 100 presence records for a species of interest: Minas Gerais for B . glabrata and B . straminea and São Paulo for B . tenagophila ( Table 2 ).
Statistical model type
To compare between machine learning modeling methods, we built three model types: Maximum Entropy (MaxEnt), Random Forest (RF), and Boosted Regression Tree (BRT). All models were built using the R program (version 4.2.2).
MaxEnt uses a maximum-entropy approach to estimate a species’ relative probability distribution in response to environmental covariates [ 24 ]. MaxEnt models create smooth fitted curves, which can facilitate straightforward ecological interpretation [ 16 ]. The degree to which this “smoothness” is enforced can be controlled through choice of regularization settings and by which feature types are provided, where options include linear, quadratic, hinge, threshold, and product features [ 16 ]. Product features are equivalent to interaction terms in regression, and they allow for limited interactions between covariates [ 16 ]. We allow MaxEnt all five of these options and use the trainMaxNet function from the enmSdmX package, which includes an L1 regularization feature [ 96 ].
On the other hand, RF, BRT, and other tree-based methods provide enhanced flexibility that allow for automatic fitting of precise interactions between the environmental covariates [ 97 ]. RF models take bootstrap samples from the training data and fit a decision tree to each sample [ 83 ]. These individual trees can have high variance (i.e., depend heavily on the training data), but have strong generalizability when averaged together to make a prediction over all fitted trees [ 97 ]. RF models use random subsets of the available predictor variables (parameter mtry ) on each decision tree split, which results in decorrelated trees and subsequently improves model performance [ 83 , 98 ]. Due to its relative ease of implementation and conceptual simplicity, RF has become a common SDM approach [ 18 ]. However, RF models have the potential to overfit, especially when provided data with high class imbalance (e.g., many more background points than presences) [ 83 ]. We use the trainRF function from the enmSdmX package [ 96 ], which is a wrapper of the randomForest function from the randomForest package [ 99 ].
BRT is similar in structure to RF, but the decision trees are recursively updated as the algorithm learns. During each step of the learning process, BRT fits new trees to the residuals for the previously fitted trees, which allows the algorithm to improve on the observations that are not yet predicted correctly [ 25 ]. We use the trainBRT function from the enmSdmX package [ 96 ], which is a wrapper of the gbm . step function from the dismo package [ 100 ]. Similar to RF, BRT also has the potential to overfit to training data but can better handle class imbalance and missing data due to its additional hyperparameters [ 25 ]. While these hyperparameters make BRT the most flexible model of the three included in our analysis, they require an additional tuning step that can be computationally expensive [ 25 ]. As of now, no one model type has fully eclipsed the others as the SDM standard, but tree-based methods have been shown to improve performance in multiple settings [ 18 ].
Model evaluation
Our goals in model evaluation were first to assess the accuracy of each model in classifying presence versus background (how well does each model classify snail distribution?), second to compare model accuracy among methods (which machine learning approach represents the data best?), third to assess the importance of different environmental covariates and the shapes of their relationships with presence (what environmental characteristics are associated with the observed snail distribution and with what functional form?), and fourth to compare this variable importance and functional form among model methods (are the relationships between predictors and snail presence consistent among models?). Before quantifying accuracy, we first assessed model biological realism qualitatively by using expert opinion to visually compare maps where each pixel shows the mean value across 10 bootstrapping iterations in which models were provided 80% of presence records available for each species at the scale of interest. Our group of experts consisted of scientists from CMM-Fiocruz and CCD-SP who have studied and organized field collection of Biomphalaria snails in Brazil for over three decades. Second, we assessed accuracy using four out-of-sample model performance metrics, as described below, calculated through ten-fold spatial cross-validation (a process where folds are divided in space instead of through random sampling, to avoid inflating SDM performance measures due to spatial autocorrelation of environmental covariates [ 19 ]). We determined the ten spatial folds using a k-means clustering algorithm where the size of folds was allowed to vary [ 101 ]. We choose this mode of data partitioning to prioritize the degree of spatial separation while also minimizing unnecessary computational time, as compared to checkerboard, n—1 jackknife, or block methodologies [ 101 , 102 ]. Each fold was required to have at least one presence and one background point.
To determine each model’s discrimination ability, we calculated sensitivity, specificity, the area under the receiver operator characteristic curve (ROC-AUC, often referred to as AUC), the partial ROC-AUC (pAUC), and true skill statistic (TSS). Sensitivity is the proportion of presences correctly identified as presences, and specificity is the number of background points correctly identified as background records. ROC-AUC measures the false positive rate (i.e., 1—specificity) versus sensitivity across all possible thresholds [ 45 ]. An AUC value of 1 indicates perfect discrimination and 0.5 or less indicates the performance is no better than random). We allowed AUC threshold values to vary across each fold for each model [ 103 ]. TSS is defined as sensitivity + specificity—1 (i.e., values of zero or less indicate the performance is no better than random) and is designed to be less sensitive to species prevalence values [ 38 ]. Given our interest in comparing each of our models’ ability to distinguish relative suitability of sites, output suitability probabilities were scaled such that all distributions ranged from 0 to 1 [ 48 , 95 ]. We also calculated partial ROC-AUC (pAUC) to better compare performance between model types, as pAUC calculates the AUC values bounded between each model’s range of predicted probabilities [ 46 ]. We implemented pAUC as described in Peterson et al., 2008 [ 46 ] using the NicheToolbox package [ 104 ], which also substitutes “proportion of area predicted as present” for the 1 –specificity x-axis. Background points are not actual absences; they can, in fact, represent areas of suitable species habitat. This substitution eliminates their impact on pAUC values. Instead, models are only evaluated on their ability to correctly identify presence points, while still being penalized for overprediction of presence areas [ 46 ]. With the intention of achieving an “out-of-sample” pAUC measure, we compared each test fold’s presence predictions with the training data’s total range of predictions. We also include a measure of pAUC significance to establish if models are performing better than random since pAUC null hypotheses can be <0.5 due to bounding between each model’s range of predicted probabilities [ 46 ]. AICc is another common, useful measure for balancing model complexity and goodness-of-fit during model selection [ 47 ]. However, we are unable to calculate AICc for two of our models (RF and BRT) due to their model structure (i.e., no obvious likelihood function or number of parameters) and therefore do not calculate the measure in this study. Calibration is a measure of how well the observed proportion of presence records in a grid cell equals the model estimated probability (i.e., 60% of grid cells predicted with a probability of 0.6 contain a presence record [ 48 ]). The main calibration evaluation technique is a calibration graph, which plots model probability estimates against the observed proportion of presences, and is predominantly used in studies with true absence data [ 48 ]. Although not applicable for this analysis, there are other situations where the calibration of the model is an additional aspect that should be tested, such as when evaluating estimates of true prevalence [ 48 ].
Finally, partial dependence plots and variable importance measures were calculated across the ten folds for each model to investigate each covariate’s contribution to model accuracy and functional relationship with presence probability. Partial dependence plots (PDP) were drawn using the pdp R package and show the marginal effect of each predictor on model probabilities [ 105 ]. Partial dependence plots allow for comparison of inferred relationships between covariates and suitability probability with a priori knowledge of factors that drive snail ecological niche suitability. Variable importance measures were calculated using the vi_shap function from the vip package [ 106 ], which calculates SHapley Additive exPlanations (SHAP) variable importance values (a method of calculating how much covariates contribute to model predictions) [ 49 , 107 ]. Notably, SHAP values are model agnostic and can estimate comparable values of variable contribution for both regression-based and tree-based methods [ 49 ].
Model types produce remarkably different national prediction maps for all species despite using the same presence and background records and environmental data ( Fig 2 ). Although probability prediction varies widely ( Fig 2 ), spatially cross-validated AUC ( Fig 3 ) and TSS ( Fig B in S1 Text ) values of national models do not substantially differ across model types. RF models tended to have somewhat higher sensitivity—they were more likely to accurately predict presence points—than MaxEnt and BRT across species ( Table 3 and Fig B in S1 Text ). RF models also had consistently higher pAUC values—they were more likely to correctly predict presence points relative to the proportion of area predicted as present—across all species and scales ( Table 3 and Fig B in S1 Text ). BRT models tended to have higher specificity—they were more likely to accurately predict background points—than MaxEnt and RF across species ( Table 3 and Fig B in S1 Text ). When comparing de-identified national prediction maps, expert opinion selected BRT maps for B . glabrata , B . straminea , and B . tenagophila as best matching a priori knowledge of current suitable snail habitat.
National prediction maps of B . glabrata (A–C), B . straminea (D–F), and B . tenagophila (G–I) suitability probabilities by model type (MaxEnt: A, D, G; Random Forest: B, E, H; Boosted Regression Tree: C, F, I). Each pixel shows the mean value across 10 bootstrapping iterations in which models were provided 80% of the available species presence records. Maps were built in R (version 4.2.2) using shapefiles from the geobr package [ 80 ].
https://doi.org/10.1371/journal.pgph.0002224.g002
Plots of ten-fold spatially cross-validated, out-of-sample AUC values across species (A, B, C), scales (panels), and model types (colors). Plots display mean (point) and +/- standard error (error bars).
https://doi.org/10.1371/journal.pgph.0002224.g003
https://doi.org/10.1371/journal.pgph.0002224.t003
Compared to these national models, model accuracy remained consistent at smaller geographic scales for B . glabrata and B . straminea and increased at smaller geographic scales for B . tenagophila ( Fig 3 and Fig B in S1 Text ), as measured by spatially cross-validated out-of-sample AUC, sensitivity, specificity, and TSS. Spatially cross-validated out-of-sample pAUC values decreased somewhat at smaller geographic scales for all species ( Fig B in S1 Text ). pAUC significance values indicate that almost all spatially cross-validated models were better than random at correctly classifying presence points, with the exception of B . glabrata MaxEnt and BRT models ( Table 3 ). However, when testing models fit to national-scale data at predicting state-level presences, all models for all species produced lower in-sample AUC and pAUC values than state models ( Table B in S1 Text ). State models also generally produced higher in-sample sensitivity and specificity values but the nationally-fit models occasionally produced higher sensitivity values (i.e., sometimes the nationally-fit models were able to correctly identify presence points that the state-specific models missed). Differences in predictive accuracy between models trained on state versus national data when tested on state data occurs due to differences in predicted suitability maps, which are visually apparent ( Fig 4 and Figs C and D in S1 Text ). We also directly measure the differences between state suitability maps produced by state and national models across species and model types by calculating pixel by pixel Pearson correlation coefficients and their significance ( Table 4 ). As evident in the predicted suitability maps, MaxEnt suitability maps remain most similar across scales for all species, while RF and BRT maps change more readily at smaller scales and produce larger improvements in model performance ( Table B in S1 Text ). Suitability maps of B . tenagophila were the most correlated for all model types ( Fig D in S1 Text ).
Minas Gerais prediction maps of B . glabrata suitability probabilities by model type (rows) and model geographic extent (columns) Each pixel shows the mean value across 10 bootstrapping iterations in which models were provided 80% of the species presence records available at a given scale. Parallel prediction maps of B . straminea in Minas Gerais and B . tenagophila in São Paulo can be found in Figs C and D in S1 Text . Compared to national models ( Fig 2 ), at smaller geographic scales it becomes more obvious that suitability probabilities can be highly localized, producing points of high suitability surrounded by areas with low suitability. Maps were built in R (version 4.2.2) using shapefiles from the geobr package [ 80 ].
https://doi.org/10.1371/journal.pgph.0002224.g004
https://doi.org/10.1371/journal.pgph.0002224.t004
Mean, 95% confidence intervals, and significance values of in-sample pixel by pixel Pearson correlation coefficients between the state suitability maps produced by state and national models across species and machine learning model type. Values are calculated across 10 bootstrapping iterations in which models were provided 80% of the species presence records available at the given scale. The values correspond to comparisons between the two columns of suitability maps in Fig 4 and Fig C in S1 Text , and Fig D in S1 Text , displaying the comparisons for B . glabrata , B . straminea , and B . tenagophila , respectively. P-values were calculated using a t-test, comparing the 10 bootstrapped values with correlations from 1000 randomly permuted maps.
Despite similar overall accuracy across machine learning model types within geographic extents (i.e., MaxEnt national compared to RF national ( Fig 3 )), the models infer strikingly different relationships between covariates and suitability probability, which imply distinct biological relationships. We use three specific examples to illustrate how responses differ across model types, spatial extents, and focal species, by comparing plots in each column of Fig 5 . First, model types produce different curve shapes: MaxEnt often fits smoother or linear forms in comparison to the flexible, nonlinear shapes produced by RF and BRT, as illustrated by distance to high population density ( Fig 5A ). Second, functional forms vary across scales: both B . glabrata and B . tenagophila responses to soil clay percentage are directionally opposite at national versus state scales for all model types ( Fig 5B ). It is important to note that the range of environmental covariates may differ remarkably across geographic extents. Third, species differ in the functional forms: B . glabrata and B . tenagophila suitability both respond nonlinearly to temperature in the coldest quarter, but with different functional responses that vary between scales ( Fig 5C ). By contrast, other functional forms remain relatively consistent across species and scale, such as the response to distance to high population density ( Fig 5A ). These differences in inferred biological relationships highlight the potential pitfalls of using SDMs to extrapolate environmental suitability beyond the scope of the data, and of assuming generality from a single modeling approach.
Examples of marginal effects of covariates on suitability probabilities that vary across model type (A), geographic scale (B), and species (C). Partial dependence plots for three covariates (columns) across model types (color), species (top two rows vs. bottom two rows), and scale (first row vs. second and third row vs. fourth).
https://doi.org/10.1371/journal.pgph.0002224.g005
Given the importance of understanding how Biomphalaria snails are responding to land use and land cover (LULC) change, we investigated how the relative importance of LULC variables changes with scale. We hypothesized that LULC variables would become increasingly important compared to climatic gradients at relatively smaller scales. Evidence for this prediction was mixed. Supporting this prediction, the relative importance of LULC variables increased consistently from national to regional to state scales for B . tenagophila models ( Fig 6C ). However, LULC variable importance for B . glabrata models ( Fig 6A ) remained more constant across scales and decreased at the state scale when using a MaxEnt model. Similar to B . glabrata , LULC variable importance for B . straminea models ( Fig 6B ) dipped in regional models and was equivalently high in national and state models.
Proportion of total variable importance averaged across all training folds attributable to distance to high population density and proportion of temporary crop cover. Displayed for all species (A,B, C) and model types (color).
https://doi.org/10.1371/journal.pgph.0002224.g006
To investigate impacts of using presence data from an expert-executed field collection program versus from a publicly-available species presence database, we constructed models using two distinct datasets: expert-collected and GBIF. As anticipated, each dataset produced distinct predictions of presence probability. Limiting these analyses to B . tenagophila in São Paulo, model accuracy was similar across both datasets (expert-collected mean AUC = 0.84,+/- standard errors: (0.82, 0.86, publicly-available GBIF mean AUC = 0.79, [0.77, 0.82]), yet the prediction maps show substantial variation regardless of model type ( Fig 7 ). Despite somewhat lower AUC values when the two datasets were combined (0.70, [0.68, 0.73]), experts judged the suitability maps as preferable when data from both sources is included, across all model types ( Fig 7C, 7F , and 7I ). Notably, the two data sets have different data quantities, with the expert-collected dataset ( n = 169) and combined dataset ( n = 234) containing more presence points than the GBIF dataset ( n = 115). The same maps and AUC comparisons for models with data quantity held constant ( n = 115) can be found in the ( Fig E in S1 Text ), with slightly more variation between model suitability maps but generally small changes to above results.
Predicted suitability maps with varying input data (columns) supplied to all model types (rows). Compared to national models ( Fig 2 ), at smaller geographic scales it becomes more obvious that suitability probabilities can be highly localized, producing points of high suitability surrounded by areas with low suitability. Maps were built in R (version 4.2.2) using shapefiles from the geobr package [ 80 ].
https://doi.org/10.1371/journal.pgph.0002224.g007
SDMs are increasingly used in disease ecology to understand environmental drivers of reservoir host or vector species distributions and to project how they might change with anthropogenic modification. We showed, by systematically comparing SDM approaches that employed different modeling techniques, spatial extents, data types, and species, that both the spatial predictions and the inferred relationships with environmental features can vary substantially across methods, even when performance measures (i.e., AUC, sensitivity, specificity, pAUC, and TSS) are very similar.
A first important result is that even when given the same presence, background, and covariate data, the three model types produce remarkably different suitability maps despite similar accuracy. Although differences in spatially-cross validated mean AUC values were minimal when compared within geographic extents (i.e., MaxEnt national compared to RF national), we found that RF models had higher pAUC and tended to have somewhat higher sensitivity, producing more ‘dense’ maps of predicted suitable habitat, than MaxEnt or BRT across species and scales ( Table 3 and Fig B in S1 Text ). Consistently higher pAUC values highlight that RF models are the best at predicting presence points correctly, even when controlling for overprediction of presence areas. On the other hand, BRT models tended to have higher specificity, producing more ‘sparse’ predictions, as compared to MaxEnt and RF across species and scales ( Table 3 and Fig B in S1 Text ).
Our analysis demonstrates the importance of individually investigating sensitivity, specificity, and pAUC (separate from AUC), especially if models are intended to inform disease control policy [ 108 , 109 ]. If total elimination is of high priority, high sensitivity and/or pAUC—the ability for models to accurately identify all presence locations—might be emphasized to safely capture all presence areas, with less concern for mistakenly implementing control interventions in places that actually contained only background records, which in this case would generally suggest using RF models for most species and scales ( Table 3 and Fig B in S1 Text ). Alternatively, with more limited resources, policymakers might prioritize models with high specificity (i.e., the ability to accurately identify locations where the species is not expected), such as the BRT models at all scales for B . tenagophila and B . straminea ( Table 3 and Fig B in S1 Text ). These models would minimize potential efficiency losses that could result from control programs deploying available resources in places that do not actually contain the species of interest. Notably, decisions regarding prevention and intervention efforts will change depending on the species of interest, and our discussion centers around snail control. Conservation efforts, for example, would likely consider different policy decisions based on modeled species distribution maps, as they are concerned with maintaining the presence of species as opposed to the absence. Finally, our experts consistently selected de-identified BRT models as producing maps that best aligned with their a priori knowledge of suitable snail habitat across multiple geographic contexts (national and São Paulo scales): these models tended to have higher specificity and lower sensitivity, making their suitability predictions relatively more sparse. Overall, our findings align with previous comparisons of statistical model types in the SDM literature: MaxEnt, RF, and BRT can all produce high model performance measures, although which is the best can vary across species types [ 18 , 41 ]. Therefore, we encourage modelers to use the suite of SDM resources (including the R packages dismo , enmSdmX , etc.) to draft multiple models for their application and explicitly test which model type is best suited for their question in close collaboration with experts in the field, particularly those who have extensive expertise in on-the-ground surveillance, as detailed further below.
When prediction maps are used to inform intervention and/or funding decisions, significant differences in the suitability maps could warrant radically differing deployment of control strategies [ 63 , 108 , 109 ]. Therefore, in addition to evaluating multiple model performance measures (AUC, sensitivity, specificity, pAUC, TSS, etc.), it is crucial to leverage local ecological knowledge to assess the biological realism of each model’s predicted suitability map, as well as of the estimated ecological relationships derived from partial dependence plots [ 93 ]. Other analyses have leveraged expert assessment of model outputs when AUC was unable to clearly rank models by performance [ 51 ]. This aligns with the well-known but underemployed guideline that remotely-sensed, big data models need to be integrated with local, on-the-ground knowledge to create the best understanding of the system of interest [ 93 ]. Fig 8 displays our recommended Standard Operating Procedure summarizing SDM model choice for disease vector and host control efforts ( Fig 8 ).
https://doi.org/10.1371/journal.pgph.0002224.g008
Subtle differences in performance across scales suggest that the most relevant geographic extent may depend on the application and the relative distribution of data at different geographical scales; yet we also found that model performance could be high from national down to state scales. Comparing across geographic scales, spatially cross-validated AUC values decreased at smaller geographic scales for B . glabrata and B . straminea , but increased at smaller geographic scales for B . tenagophila ( Fig 3 ; Fig B in S1 Text ). This phenomenon can likely be attributed to the varying proportion of presence data for each species within each state ( Table 2 ). While 89% of national B . tenagophila data is from within the Sudeste region and 65% is within São Paulo state, only 74% of national B . glabrata data is from the Sudeste region and 61% is from Minas Gerais. B . straminea had an even smaller proportion of total national data at the Sudeste (52%) and state level (39%). Accordingly, we hypothesize that larger amounts of localized data for B . tenagophila Sudeste and São Paulo models improved model accuracy, while limiting the ability for national models to capture ecological heterogeneity across the entirety of Brazil. On the other hand, B . glabrata and B . straminea records are more widely distributed across the nation, allowing for improved national predictions, whereas the smaller data set from Minas Gerais limits the relative performance of state and regional models. When specifically aiming to create best predictions for small geographic regions, we demonstrate that locally-fit SDMs moderately increase model discrimination ability ( Table B in S1 Text ) and create maps with substantially different predictions as compared to nationally-fit models ( Table 4 and Fig 4 and Figs C and D in S1 Text ). However, when data are more uniformly distributed at the national scale, national scale models can be cropped to smaller scales relatively effectively, indicating that building national models can also be warranted when needed for large-scale applications or when investigating smaller geographic regions that have limited local data. A final key factor affecting choice of geographic extent is whether the aim is to identify covariate relationships specific to a geographic area of interest or to see generalized covariate relationships that span heterogeneous habitats and geographies, including ranges not yet observed in a given geographic region. This is particularly important when researchers aim to use SDMs to project species distributions under scenarios of future climate change, which include temperature and precipitation patterns not yet experienced in a given region.
Covariate relationships not only varied depending on geographic extent, but also by species and machine learning model used. Even for two snail species in the same genus, their responses to environmental covariates varied in both magnitude and direction ( Fig 5 ), contributing to the large suitability map differences ( Fig 2 and Fig 7 ). Compounding these true biological differences among species is the fact that different model structures produce differently shaped partial dependence plot curves, weighting interpretability versus flexibility and differentially favoring nonlinearity and interactions [ 16 , 30 , 97 ]. For example, even when providing our MaxEnt models maximum flexibility in fitting the observed data, the resulting PDP curves still exhibit more limited shapes than RF or BRT. MaxEnt’s smooth curves offer simple, interpretable predictor relationships—potentially preferred for modelers whose primary interest is general mechanisms that underlie habitat suitability and/or ease of explanation for policymakers who need to make decisions with limited time [ 16 ]. On the other hand, the hyper-flexible curves produced by RF and BRT (and other tree-based methods) can produce improved model performance and variable interactions, especially when models include suites of variables known to interact in nonlinear ways, such as temperature and precipitation or sets of LULC variables [ 25 , 83 , 97 ]. If model classification ability is held at the highest priority and modelers believe it is ecologically feasible for predictors to have flexible relationships, partial dependence plots and the other model evaluation methods discussed here can assist in retaining clear model interpretation [ 49 , 107 ]. Finally, we note that SDMs are correlative analyses. Therefore, modeled covariate relationships may not be directly related to species presence but with other environmental variables not included in the model. SDMs should be followed by causal analyses if the goal is to understand true causes of species presence.
LULC variables became proportionally more important for predicting B . tenagophila snail presence at smaller geographic scales as compared to bioclimatic variables. However, LULC variable importance remained relatively constant across scales for both B . glabrata and B . straminea . Given that remotely-sensed bioclimatic variables predominantly change at larger spatial scales (i.e., they are highly spatially-autocorrelated), we expected that models of smaller geographic extent would rely more heavily on LULC variables, which contribute more localized variation ( Fig A in S1 Text ). This hypothesized effect may have been mitigated for two of the three snail species due to the fact that we held spatial resolution (1km 2 ) constant over the three geographic extents. Other analyses varying resolution size have shown that biotic interactions dominate at local scales, while abiotic factors dominate regionally [ 35 ]. Holding resolution constant likely allowed even national scale models to leverage localized variation derived from LULC variables.
Given an adequate number of presence points, publicly-available GBIF data creates models with comparable snail distribution predictions and model performance measures as models given an expert-collected dataset. This is a very encouraging finding given that expert-executed field collection programs can be logistically infeasible and public species presence resources have grown in size and popularity [ 22 ]. Moreover, even when expert field collection is feasible, it is often not possible to execute surveillance programs across large areas, such as the entirety of Brazil. GBIF cannot always guarantee the same level of species identification accuracy as the morphological and molecular tools often used in expert-executed field sampling, but the accessibility of large amounts of species data has dramatically increased the potential for species distribution analyses [ 22 ]. Although only a singular case-study, our findings support the utility of GBIF data for producing accurate SDMs without targeted field collection programs. It is critical to employ methods to overcome spatial biases inherent in these publicly available data sources [ 51 , 52 ], such as through geographically stratified background sampling and careful inclusion criteria, but our findings support the growing use of these resources [ 34 ]. Importantly, many of these conclusions rest on our example where there was sufficient quantity of GBIF data, which was only true of one species in São Paulo state. Our findings demonstrate the value that GBIF data can offer to disease control and elimination efforts and we support ongoing initiatives working to increase access, precision, and quality of GBIF data across all species and geographies [ 110 ].
Although our analysis contributes substantially to describing and quantifying current best practices in the SDM literature, there are several limitations. First, the smallest geographic extent we investigated was at the state level, which is still a large area. Other modeling studies, including some of specific Biomphalaria species, have been conducted at the municipality or intra-municipality scale [ 67 , 68 ]. Although infeasible due to data quantity constraints across species for this study, it is possible that our comparisons could have been augmented for local specificity if we had included models built for specific municipalities. Secondly, we included a limited number of predictors in this case study for ease of interpretation, especially given our plan to compare models across geographic extents, machine learning models, and data sources. However, some of our findings could be sensitive to the number, resolution, and/or spatial-autocorrelation of predictors included [ 111 ]. For example, a set of predictors dominated by LULC variables—rather than our models that included only two—could come to differing conclusions on changes in variable importance or partial dependence relationships. However, our set of predictors was chosen to be biologically relevant, sufficient to capture ecological relationships, and sufficiently general to be representative for other species distribution modeling studies. A combination of bioclimatic, LULC, and other variables is very common in the body of literature informing this analysis [ 41 ]. Lastly, while this analysis does compare results across three species of snails, the species are very similar in that they are all from the same genus and are all freshwater mollusks. Other species, even among those relevant to disease ecology, could vary in their response to our analyses across machine learning models, spatial extents, and data sources [ 18 ]. However, our analysis shows that even species in the same genus may have significantly different ecological niches, indicating that modeling decisions need to be grounded in system-specific ecological and biological knowledge.
There rightfully remains no single gold-standard of SDM methods suitable for all species, geographic locations, and applications because differing contexts and intended uses warrant differing modeling decisions. Making species distribution models that are useful and accurate for a given question of interest requires careful design and in-depth evaluation. This paper aims to serve as a resource and reference for current methods in species distribution modeling, with applications to disease ecology. Given the extent to which these models are used to inform fieldwork, policy, funding, and intervention strategies, continuous assessment and model evaluation are imperative. Species distribution models are powerful tools if used appropriately, and this work illustrates the importance of three key dimensions of variation—model type, spatial extent, and data source—highlighting that the former two can have large implications for model predictions and interpretation.
Supporting information
https://doi.org/10.1371/journal.pgph.0002224.s001
Acknowledgments
We would like to thank the Medical Malacology Collection of Fiocruz Minas for sharing Biomphalaria data used in this study.
- View Article
- PubMed/NCBI
- Google Scholar
- 23. GBIF. GBIF [Internet]. GBIF. [cited 2023 Jul 5]. Available from: https://www.gbif.org/
- 34. Smith AM, Capinha C, Kramer AM. Predicting species distributions with environmental time series data and deep learning [Internet]. bioRxiv; 2022 [cited 2023 Mar 24]. p.2022.10.26.513922. Available from: https://www.biorxiv.org/content/10.1101/2022.10.26.513922v1
- 49. Lundberg SM, Lee SI. A unified approach to interpreting model predictions. In: Advances in Neural Information Processing Systems [Internet]. Curran Associates, Inc.; 2017 [cited 2023 Mar 26]. Available from: https://proceedings.neurips.cc/paper_files/paper/2017/hash/8a20a8621978632d76c43dfd28b67767-Abstract.html
- 50. Greenwell BM, Boehmke BC, McCarthy AJ. A simple and effective model-based variable importance measure [Internet]. arXiv; 2018 [cited 2023 Mar 26]. Available from: http://arxiv.org/abs/1805.04755
- 54. Mitchell C. PAHO/WHO | Schistosomiasis [Internet]. Pan American Health Organization / World Health Organization. 2014 [cited 2023 May 26]. Available from: https://www3.paho.org/hq/index.php?option=com_content&view=article&id=9474:schistosomiasis-factsheet&Itemid=0&lang=en#gsc.tab=0
- 72. Tuan R, Pires F, Sanches Palasio RG, Dalla R, Almeida Guimaraes MCD. Pattern of Genetic Divergence of Mitochondrial DNA Sequences in Biomphalaria tenagophila Complex Species Based on Barcode and Morphological Analysis. In: Rokni MB, editor. Schistosomiasis [Internet]. InTech; 2012 [cited 2023 Apr 17]. Available from: http://www.intechopen.com/books/schistosomiasis/pattern-of-genetic-divergence-of-mitochondrial-dna-sequences-in-biomphalaria-tenagophila-complex-spe
- 81. IUCN. IUCN [Internet]. [cited 2023 Jul 5]. Available from: https://www.iucn.org/
- 85. CHELSA. CHELSA [Internet]. Chelsa Climate. 2020 [cited 2023 May 26]. Available from: https://chelsa-climate.org/downloads/
- 87. Hengl T, Gupta S. Soil water content (volumetric %) for 33kPa and 1500kPa suctions predicted at 6 standard depths (0, 10, 30, 60, 100 and 200 cm) at 250 m resolution [Internet]. Zenodo; 2019 [cited 2023 Jul 5]. Available from: https://zenodo.org/record/2784001
- 88. Hengl T. Soil pH in H2O at 6 standard depths (0, 10, 30, 60, 100 and 200 cm) at 250 m resolution [Internet]. Zenodo; 2018 [cited 2023 Jul 5]. Available from: https://zenodo.org/record/2525664
- 89. Hengl T. Clay content in % (kg / kg) at 6 standard depths (0, 10, 30, 60, 100 and 200 cm) at 250 m resolution [Internet]. Zenodo; 2018 [cited 2023 Jul 5]. Available from: https://zenodo.org/record/2525663
- 90. Open Spatial Demographic Data and Research. WorldPop [Internet]. WorldPop. [cited 2023 Jul 5]. Available from: https://www.worldpop.org/
- 94. Guisan A, Thuiller W, Zimmermann NE. Habitat suitability and distribution models: with applications in R [Internet]. Cambridge: Cambridge University Press; 2017 [cited 2023 Mar 25]. (Ecology, Biodiversity and Conservation). Available from: https://www.cambridge.org/core/books/habitat-suitability-and-distribution-models/A17F74A3418DBF9ADA191A04C35187F9
- 98. Hastie T, Friedman J, Tibshirani R. The Elements of Statistical Learning [Internet]. New York, NY: Springer; 2001 [cited 2023 Mar 27]. (Springer Series in Statistics). Available from: http://link.springer.com/10.1007/978-0-387-21606-5
- 0 Shopping Cart
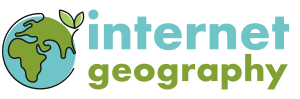
Hurricane Katrina Case Study
Hurricane Katrina is tied with Hurricane Harvey (2017) as the costliest hurricane on record. Although not the strongest in recorded history, the hurricane caused an estimated $125 billion worth of damage. The category five hurricane is the joint eight strongest ever recorded, with sustained winds of 175 mph (280 km/h).
The hurricane began as a very low-pressure system over the Atlantic Ocean. The system strengthened, forming a hurricane that moved west, approaching the Florida coast on the evening of the 25th August 2005.
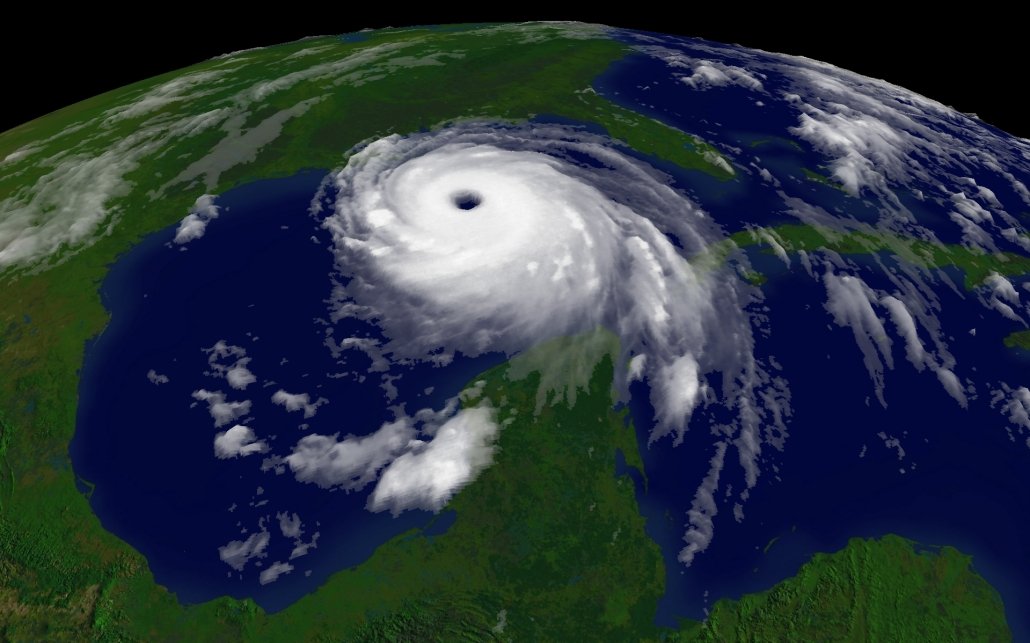
A satellite image of Hurricane Katrina.
Hurricane Katrina was an extremely destructive and deadly Category 5 hurricane. It made landfall on Florida and Louisiana, particularly the city of New Orleans and surrounding areas, in August 2005, causing catastrophic damage from central Florida to eastern Texas. Fatal flaws in flood engineering protection led to a significant loss of life in New Orleans. The levees, designed to cope with category three storm surges, failed to lead to catastrophic flooding and loss of life.
What were the impacts of Hurricane Katrina?
Hurricane Katrina was a category five tropical storm. The hurricane caused storm surges over six metres in height. The city of New Orleans was one of the worst affected areas. This is because it lies below sea level and is protected by levees. The levees protect the city from the Mississippi River and Lake Ponchartrain. However, these were unable to cope with the storm surge, and water flooded the city.
$105 billion was sought by The Bush Administration for repairs and reconstruction in the region. This funding did not include potential interruption of the oil supply, destruction of the Gulf Coast’s highway infrastructure, and exports of commodities such as grain.
Although the state made an evacuation order, many of the poorest people remained in New Orleans because they either wanted to protect their property or could not afford to leave.
The Superdome stadium was set up as a centre for people who could not escape the storm. There was a shortage of food, and the conditions were unhygienic.
Looting occurred throughout the city, and tensions were high as people felt unsafe. 1,200 people drowned in the floods, and 1 million people were made homeless. Oil facilities were damaged, and as a result, the price of petrol rose in the UK and USA.
80% of the city of New Orleans and large neighbouring parishes became flooded, and the floodwaters remained for weeks. Most of the transportation and communication networks servicing New Orleans were damaged or disabled by the flooding, and tens of thousands of people who had not evacuated the city before landfall became stranded with little access to food, shelter or basic necessities.
The storm surge caused substantial beach erosion , in some cases completely devastating coastal areas.
Katrina also produced massive tree loss along the Gulf Coast, particularly in Louisiana’s Pearl River Basin and among bottomland hardwood forests.
The storm caused oil spills from 44 facilities throughout southeastern Louisiana. This resulted in over 7 million US gallons (26,000 m 3 ) of oil being leaked. Some spills were only a few hundred gallons, and most were contained on-site, though some oil entered the ecosystem and residential areas.
Some New Orleans residents are no longer able to get home insurance to cover them from the impact of hurricanes.
What was the response to Hurricane Katrina?
The US Government was heavily criticised for its handling of the disaster. Despite many people being evacuated, it was a very slow process. The poorest and most vulnerable were left behind.
The government provided $50 billion in aid.
During the early stages of the recovery process, the UK government sent food aid.
The National Guard was mobilised to restore law and order in New Orleans.
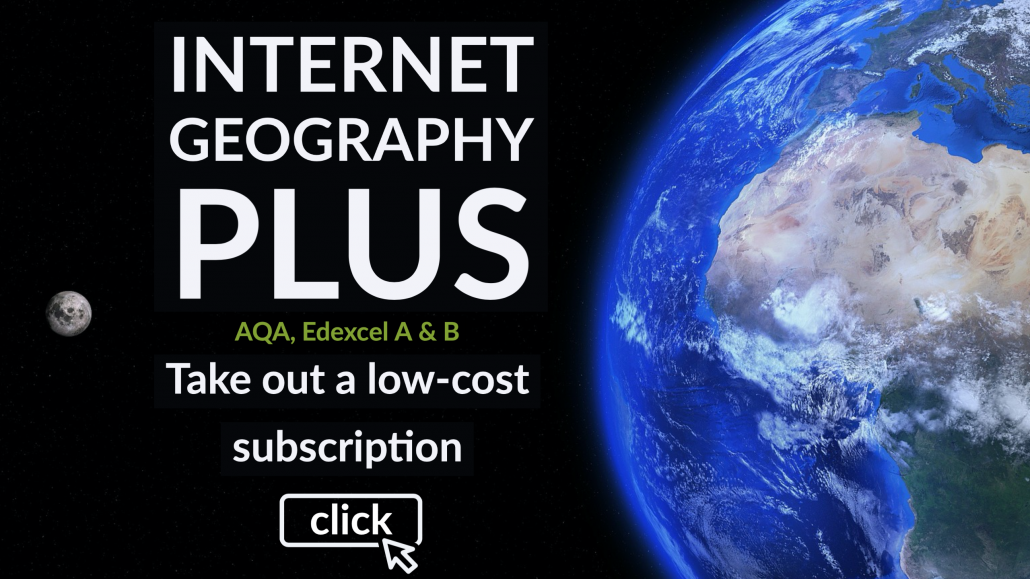
Premium Resources
Please support internet geography.
If you've found the resources on this page useful please consider making a secure donation via PayPal to support the development of the site. The site is self-funded and your support is really appreciated.
Related Topics
Use the images below to explore related GeoTopics.
Hurricane Florence
Topic home, hurricane michael, share this:.
- Click to share on Twitter (Opens in new window)
- Click to share on Facebook (Opens in new window)
- Click to share on Pinterest (Opens in new window)
- Click to email a link to a friend (Opens in new window)
- Click to share on WhatsApp (Opens in new window)
- Click to print (Opens in new window)
If you've found the resources on this site useful please consider making a secure donation via PayPal to support the development of the site. The site is self-funded and your support is really appreciated.
Search Internet Geography

Latest Blog Entries

Pin It on Pinterest
- Click to share
- Print Friendly
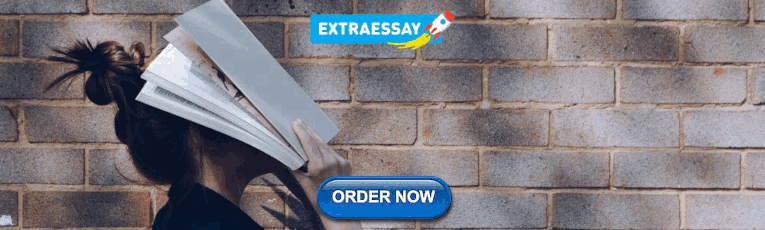
IMAGES
VIDEO
COMMENTS
Welcome to GeographyCaseStudy.Com - a site for International Baccalaureate (IB) Diploma Programme (DP) Geography teachers and students. Use the 'Topics' menu at the top of every page to navigate to detailed explanations of IBDP Geography units such as Urban Environments, Global Climate Change, Leisure Tourism and Sport and more, with a full references list and questions at…
By Matt Burdett, 10 March 2020. This article is about how to plan your revision for Geography in one week. Even with just a week, you can still make revision highly effective if you follow a condensed version of my '6-point plan to stress-free revision'. If you don't have time for that - maybe because you're still in school, or have to work a part-time job - I recommend two simple ...
The main urban land uses are: Residential. Industrial. Commercial and administrative. Infrastructure (including transport) Open space (including planned open space like parks, and derelict space) Urban land use is generally described as the land use at ground level. This is typically what is shown on maps.
Share this: Geography Case Studies - A wide selection of geography case studies to support you with GCSE Geography revision, homework and research.
Welcome! I am a Head of Geography, with a Masters Degree in Education and 15+ years experience helping students achieve amazing results at GCSE. I am busy creating a huge bank of resources for my ...
Click on the map below that shows you the spread of current case studies for the IB DP Geography course. Part 1 - Themes. Theme A - Freshwater (blue tap) Theme D - Geophysical Hazards (blue earthquake trace) Theme E - Leisure, Sport, Tourism (brown binoculars) Theme G - Urban Environments (purple skyscrapers) Part 2 - Perspectives.
How to revise geography case studies. 1. Make sure you understand the case study. The first step in remembering anything is understanding it. You need to have a clear model in your mind of how the case study works. This includes how it's laid out in space (a mental map), who the people were who were involved and the context of the case study ...
Best free resources for CAIE IGCSE Geography 0460 Case Studies including summarized notes, topical and past paper walk through videos by top students.
geographycasestudy.com has an average to good trust score. Why? It seems that geographycasestudy.com is legit and safe to use and not a scam website. The review of geographycasestudy.com is positive. The positive trust score is based on an automated analysis of 40 different data sources we checked online such as the technology used, the location of the company, other websites found on the same ...
AQA GCSE Geography Urban Issues and Challenges Urbanisation in the UK: London Case Study. London is the capital city of the United Kingdom and stands as one of the world's dominant economic hubs. Since the late 18th century, it has experienced rapid growth in size and population, driven by increasing rates of urbanisation.
Case Study - Rio de Janeiro. Rio de Janeiro is a city located on Brazil's south-east coast. It is one of Brazil's largest settlements with a population of approximately 11.7 million people. The population of Rio de Janeiro has grown for a number of reasons. Natural Increase is one reason for its growth (this is when the birth rate is ...
Role of TNCs. The role of TNCs in expanding international tourism destinations, including the costs and benefits of TNC involvement for different stakeholders. Use this material to make sure you fully understand the role played by TNCs in expanding tourism worldwide. Make notes which summarise the ways in which tourism has been driven by TNCs.
In our online database you can find free Geography Case Study work for every taste: thesis, essays, dissertations, assignments, research and term papers etc. - easy and free. Choose any document below and bravely use it as an example to make your own work perfect! An case study examples on geography is a prosaic composition of a small volume ...
Essential infrastructure includes the following: Transport. Sanitation. Water supplies. Waste disposal. Telecommunications. These infrastructure are almost always run on a network system in urban areas. This means they have inputs, outputs, nodal points and links. Inputs: the material or energy coming into the urban system.
Over 230,000 people killed. 11⁄2 million people were made homeless. 50% of buildings were destroyed because they were made mainly of concrete and poorly built. 250,000 buildings razed. Cost of the earthquake was $7.8billion. Water supplies were destroyed, and bodies lay in the streets unable to be buried.
Geography Weston = Urban Policy - Case Studies 😑. Property Led Inititaives, London Docklands Developemnt Corporation, Partnership Schemes ... Study Geography Case Studies using smart web & mobile flashcards created by top students, teachers, and professors. Prep for a quiz or learn for fun!
Thar Desert Case Study. The Thar Desert, also known as the Great Indian Desert, is located in northwest India and stretches into Pakistan. It covers 200,000 square kilometres and is the most densely populated desert in the world. The desert forms a natural boundary between India and Pakistan. A map to show the location of the Thar Desert in India.
About. Welcome to my website - a kind of online Geography textbook. I started writing it because I needed it. I hope it's helping you too. If you find the site useful, I'd love to hear how you're using it and what you like about it. And if you have any suggestions for improvements or corrections, or think I've used something I shouldn ...
Location of teams and their grounds. There are several geographical factors that affect the location of sports teams. These include. In most sports leagues, the teams towards the top of the league hierarchy are from larger, wealthier settlements. These teams generally have more supporters and more income from selling tickets and merchandise.
Forget what you learnt in elementary school geography, Earth apparently doesn't have seven continents after all. From a young age, we're taught that the world is made up of Africa, Antarctica ...
Growth forecasts. Tourism is predicted to increase in the future. The number of international tourists is predicted to increase by 3.3% per year up to 2030, reaching 1.8 billion tourist arrivals (Lee, 2017). Although all areas are set to grow, the fastest growth will be in emerging economies in Asia, Africa and the Middle East.
Nigeria - A case study of a NEE experiencing rapid economic development which leads to significant social, environmental and cultural change. What is the role of transnational companies in Nigeria?
Species distribution models (SDMs) are increasingly popular tools for profiling disease risk in ecology, particularly for infectious diseases of public health importance that include an obligate non-human host in their transmission cycle. SDMs can create high-resolution maps of host distribution across geographical scales, reflecting baseline risk of disease. However, as SDM computational ...
Hurricane Katrina was an extremely destructive and deadly Category 5 hurricane. It made landfall on Florida and Louisiana, particularly the city of New Orleans and surrounding areas, in August 2005, causing catastrophic damage from central Florida to eastern Texas. Fatal flaws in flood engineering protection led to a significant loss of life in ...