DataStax Astra Vectorize: Generate Vector Embeddings with 1 Line of Code
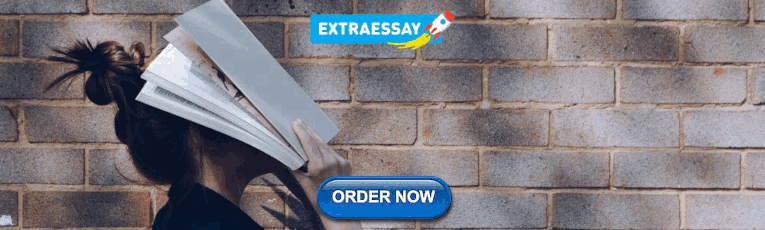
NoSQL Use Cases: When to Use a Non-Relational Database
Rich edwards.
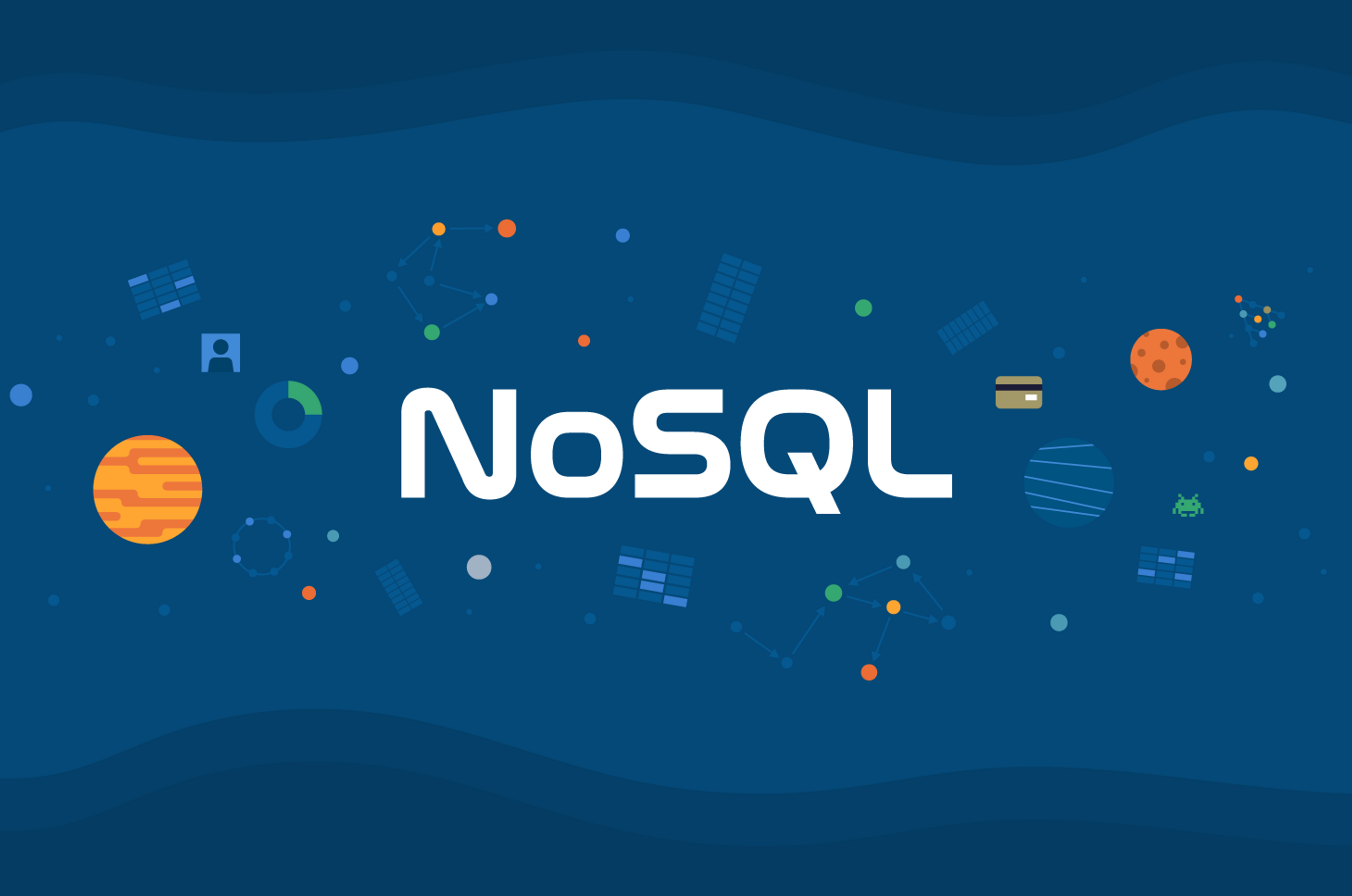
For decades, many companies have relied on relational databases to store, protect, and access their data. SQL databases, in particular, worked well for a long time and still do for many use cases. But, today, there are a wide range of situations where SQL databases can no longer satisfy the needs of modern enterprises, especially those that have made the move to the cloud. Increasingly, these companies are turning to NoSQL databases to meet their goals.
NoSQL databases are likely the better choice when:
- You have a large volume and variety of data
- Scalability is a top priority
- You need continuous availability
- Working with big data or performing real-time analytics
While this will often make a NoSQL database the right choice, there are many things to consider before making the move. In this post, we’ll explore when NoSQL use cases make sense. First, let’s take a closer look at NoSQL.
What is NoSQL?
NoSQL is short for “not only SQL,” or “non-SQL.” It’s a term used to describe databases that are not relational. To better understand NoSQL databases, let’s first take a look at their alternative, SQL databases.
Developed in the early 1970s, a time when data storage was extremely expensive, SQL databases attempt to minimize data duplication between tables. While extremely organized, this also makes them extremely inflexible and difficult to modify. Since then, the cost of storage has plummeted, while the cost of developer time has dramatically increased. With NoSQL databases, developers are no longer limited to the rigid, tabular approach of relational databases and have far more flexibility to do their best work.
NoSQL comes with many benefits, including:
- The choice of several database types— key-value, document, tabular (or wide column), graph, and multi-model —so you can find the best fit for your data.
- The flexibility to easily store and access a wide variety of data types together, without upfront planning. The data types can include structured, semi-structured, unstructured, and polymorphic.
- The ability to add new data types and fields to the database without having to redefine the data model.
- Built-in, horizontal scalability that can handle rapid growth and is much less costly than attempting to scale-out a SQL database.
- Continuous availability and strong resilience, due to its horizontal scaling approach.
- Ease-of-use for developers that fits well with modern, Agile teams.
Learn more about NoSQL .
Comparing NoSQL to SQL
While NoSQL databases have many advantages, they’re not the right choice for every situation. Sometimes sticking with a tried-and-true SQL database is the way to go. Let’s compare SQL and NoSQL databases across several factors. Think about how each would apply to your data profile and use cases.
NoSQL use cases
As you can see, making the choice between a SQL and NoSQL database is not always a straightforward decision. Each has its advantages and disadvantages. Making the right choice depends on your organization’s specific data environment, along with your current needs and future goals. Many development teams actually use both within their cloud data architecture, sometimes even within the same application—deploying each to cover the areas they handle best.
So, what are the non-relational use cases? Here are several where NoSQL has been proven to make sense:
Fraud detection and identity authentication
Inventory and catalog management.
- Personalization, recommendations and customer experience
- Internet of things (IoT) and sensor data
- Financial services and payments
- Logistics and asset management
- Content management systems
- Digital and media management
Let’s look at the first three NoSQL use cases more closely.
Protecting sensitive personal data and ensuring only real customers have access to applications is understandably a top priority. Of course, this is only heightened in areas such as financial services, banking, payments, and insurance.
It’s a never-ending battle. Fraudsters are creative and nimble. They tirelessly look for new ways to break the seal and their attacks continue to rise at an alarming rate. Whether you’re trying to prevent illegitimate users from gaining access, or authenticating the identity of your customers, you have to lean heavily on your data.
It’s possible to identify patterns and anomalies to pinpoint fraud in real-time or, in some cases, even before it occurs. To do so, real-time analysis of a large volume of both historic and live data of all types is required, including but not limited to user profile, environment, geographic data, and perhaps even biometric data. And context matters. For example, a $500 withdrawal may not typically be a big deal for a particular customer, but it might raise a red flag if the attempt originates at 3 a.m. in a foreign country.
The stakes to your company’s reputation are higher than ever. One breach or mistake can be quickly amplified with the social media megaphone. It’s a balancing act because setting restrictions too narrowly could result in a false positive rate that can adversely impact the customer experience. You want to make it as easy as possible for customers to use your application or website, while ensuring they actually are who they say they are. It’s quite a tightrope to walk.
This combination of needs, including real-time analysis, large and growing datasets, numerous data types, along with the ability to continuously analyze and conduct machine learning and AI , makes the decision to use a NoSQL database a no-brainer for fraud detection and identity authentication.
Take the case of ACI Worldwide , a company that provides real-time payment capabilities to 19 of the top 20 banks in the world. Their transaction volume is astronomically high, processing trillions of dollars in payments every day, and their data analysis needs are complex.
While payment intelligence systems have used relational databases in the past, that approach struggles to handle growing, large-scale use cases that require complex data analysis. At some point, it becomes impractical and cost-prohibitive to build a relational database big enough to do the job. To have any chance at handling these needs, a SQL database would have to be partitioned. In addition to being extremely resource intensive and expensive, partitioning would have another drawback. For the fraud use case, all information across all dimensions is needed to make each transaction decision. To handle the ever-growing volume, inevitably, a partitioned relational database would have to decrease the window of time of past transactions evaluated. As that time window shrinks, so does the ability to detect fraud.
For effective fraud detection and identity authentication, the data types analyzed extend far beyond transactional information. They could include anything from demographic data, help desk information from the CRM system, website interactions, historical shopping data, and much, much more. It would be impossible to develop a schema upfront that would define everything customers might want to do in the future. This environment requires the flexibility of a NoSQL database where any type of data element can be quickly added to the mix.
Using DataStax Enterprise (DSE), ACI has improved its fraud detection rate and false positive rate, while saving their customers millions of dollars. And ACI’s call center is saving money as fewer false positive cases are routed there.
Read more about how ACI is battling fraud with a NoSQL solution .
NoSQL databases are known for their high availability and predictable, cost-effective, horizontal scalability. This makes them a great match for e-commerce companies with massive, growing online catalogs and loads of inventory to manage.
These organizations need the flexibility to quickly update their product mix, without volume limits. And the worst thing imaginable for them would be to have their site or application go down on Black Friday or during the Christmas holiday season.
For these reasons, Macy’s has made the journey from relational databases to NoSQL. One of the most prominent department stores in the world, Macy’s also has one of the largest e-commerce sites, with billions in annual sales. Like ACI, Macy’s handles a massive volume of data that is diverse and growing. Before the move to NoSQL, the company had a heavily normalized database that limited their ability to scale their catalog and online inventory. Now that DSE and a NoSQL database are in place, this is no longer a source of concern for the Macy’s team.
With their NoSQL database setup, Macy’s can now:
- Handle traffic growth and massive volumes of data
- Easily and cost-effectively scale
- Provide faster catalog refreshes
- Grow its online catalog and number of products
- Analyze its catalog and inventory in real time
Learn more about Macy’s move to NoSQL .
Personalization, recommendations, and customer experience
Providing a fast, personalized experience is no longer a differentiator. Today, it’s table stakes. Customers expect a consistent, high-quality, tailored experience from your brand, 24/7, across all devices.
They take it for granted. They demand near real-time interactions and relevant recommendations. While it’s still possible to carve out unique, memorable experiences, the first priority is to make sure you have these bases covered. If you don’t, that’s what they’ll remember. And, if that happens, you run the risk of them turning to Twitter or Facebook and amplifying your shortcomings. NoSQL databases are the answer to power the individualized experiences that will keep your customers happy.
That’s because NoSQL databases:
- Have fast response times with extremely low latency, even as a customer base expands
- Can handle all types of data, structured and unstructured, from a variety of sources
- Are built to cost-effectively scale, with the ability to store, manage, query, and modify extremely large volumes of data and concurrently deliver personalized experiences to millions of customers
- Are extremely flexible, so you can continuously innovate and improve the customer experience
- Can seamlessly capture, integrate, and analyze new data that is continuously flowing in
- Are adept at being the backbone for the machine learning and AI engine algorithms that provide recommendations and power personalization
By focusing on providing intuitive, superior online customer experiences from the start, Macquarie Bank , an Australian financial services company, was able to move from no retail banking presence to a top contender in the digital banking space in less than two years. Their focus on truly understanding customer behavior and prioritizing personalization has been a key to their success. So, it’s no surprise they use a NoSQL database (Apache Cassandra with DataStax Enterprise) to provide their customers with near real-time recommendations, interactions, and insights.
Read more about how MacQuarie uses NoSQL to provide personalization for their customers .
Do you have a NoSQL use case?
Hopefully, this post and the non-relational database examples above have provided some guidance about when using a NoSQL database would be the smart move. So, what’s the next step if you determine your company does indeed have NoSQL use cases?
A great place to start is to schedule a demo for DataStax Astra DB , a scale-out NoSQL database built on Apache CassandraTM.
Or, if you want to jump right in, you can get started with Astra DB for free .
More Technology
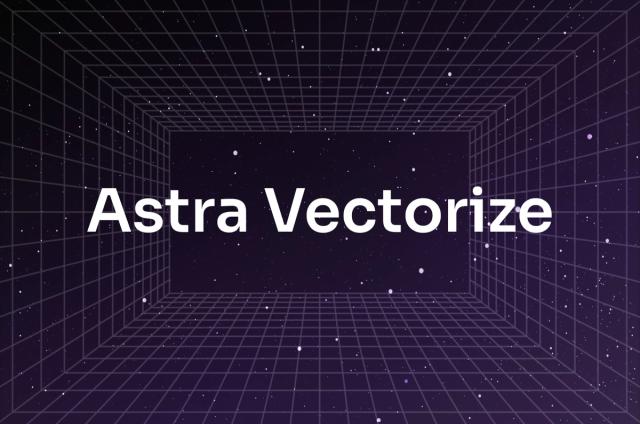
Astra Vectorize: Generate Vector Embeddings with 1 Line of Code
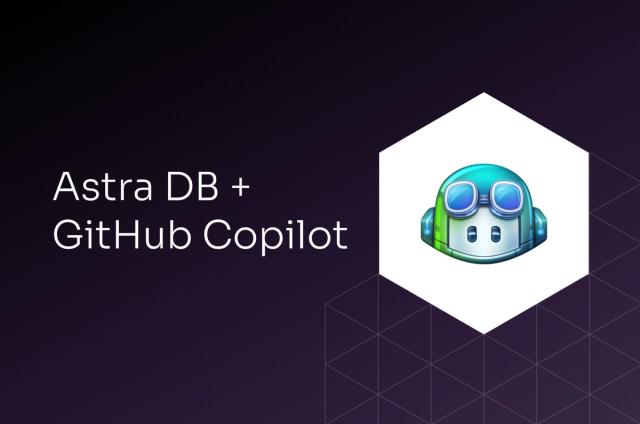
GitHub Copilot + DataStax Astra DB: Build GenAI Apps 100x Faster
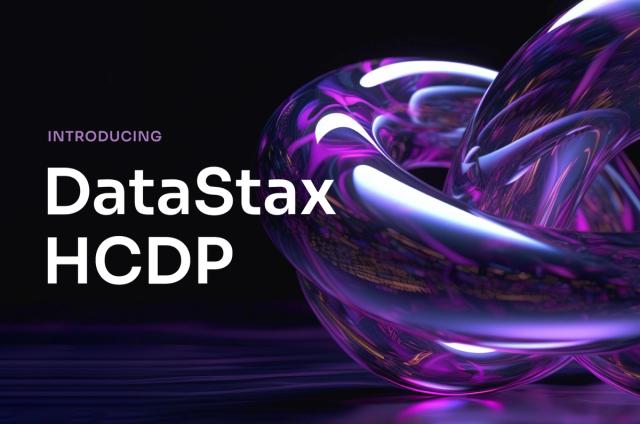
Empowering On-Premises Deployments with Generative AI: Introducing Vector Search for a Self-Managed Modern Architecture
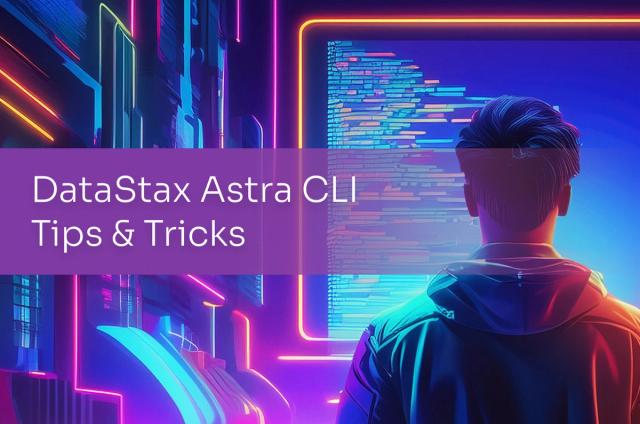
Tips and Tricks for the DataStax Astra CLI
One-stop data api for production genai.
Astra DB gives JavaScript developers a complete data API and out-of-the-box integrations that make it easier to build production RAG apps with high relevancy and low latency.
Reverse Engineering in a Client-Server Environment
Case Studies on Relational Database Design
Cite this chapter
- Brian Siu 2 &
- Joseph Fong 3
70 Accesses
1 Citations
Relational database management systems (RDBMS) share a high percentage of the database market. Coupled with client application development tools like PowerBuilder, * and data modeling and design tools like ERwin/ERX, rapid application development is made possible. However, due to the ease of use of these client-server tools, there is a tendency for application developers to quick start their application development process without going through a formal process of database design. Through the use of reverse engineering tools, physical database schemas can be converted into their entity-relationship (ER) model. By looking at the ER model we can evaluate whether the relational database design has been completee or not.
This paper first gives an overview of client-server computing, reengineering and reverse engineering, followed by an introduction of a database modeling and design tool which provides forward and reverse engineering capabilities in a client-server database environment. Using this tool, two case studies were carried out. In the first case, an incomplete database design was revealed. In the second case, the ER model showed that database design has been completed. An integrated approach is proposed for database modeling, design and database generation.
Article Footnote
PowerBuilder, Erwin/ERX, and other brand names are product names of their respective owners.
This is a preview of subscription content, log in via an institution to check access.
Access this chapter
- Available as PDF
- Read on any device
- Instant download
- Own it forever
- Compact, lightweight edition
- Dispatched in 3 to 5 business days
- Free shipping worldwide - see info
Tax calculation will be finalised at checkout
Purchases are for personal use only
Institutional subscriptions
Unable to display preview. Download preview PDF.
Cheung, To-yat (1993). “Temporal Databases - their present and future”, 5th International Hong Kong Computer Society Database Workshop, Next Generation Database Systems, Hong Kong Computer Society, Hong Kong, pp.29–46.
Google Scholar
Date, C.J. (1990). An Introduction to Database Systems, 5/e, Addison-Wesley.
Dewire, D.T. (1993). Client/Server Computing. McGraw Hill.
Khoshafian, S. (1993). Object-oriented Databases, Wiley.
Logic Works (1994). ERwin/ERX for PowerBuilder, Database Design Tool, Release 1.5c,User’s Guide. Princeton, Logic Works Inc. 1989–1994, NJ 08540.
Oracle Seminar (1994). Oracle CDE2 Tools, Maximizing Developer Productivity Seminar.Oracle Systems Hong Kong Limited, December 8, 1994.
PowerBuilder Watcom SQL, Version 3.0, PowerSoft Corporation, 1994.
Sybase (1993). Sybase OnmiSQL Gateway: A New Generation Gateway for the Distributed Enterprise. Sybase Corporation, April 1993.
Download references
Author information
Authors and affiliations.
Division of Technology, City University of Hong Kong, Tat Chee Avenue, Kowloon Tong, Hong Kong, China
Department of Computer Science, City University of Hong Kong, Tat Chee Avenue, Kowloon Tong, Hong Kong, China
Joseph Fong
You can also search for this author in PubMed Google Scholar
Editor information
Editors and affiliations.
City University of Hong Kong, Kowloon, Hong Kong, China
To-yat Cheung , Joseph Fong & Brian Siu , &
Rights and permissions
Reprints and permissions
Copyright information
© 1996 Springer Science+Business Media New York
About this chapter
Siu, B., Fong, J. (1996). Reverse Engineering in a Client-Server Environment. In: Cheung, Ty., Fong, J., Siu, B. (eds) Database Reengineering and Interoperability. Springer, Boston, MA. https://doi.org/10.1007/978-1-4615-1803-7_7
Download citation
DOI : https://doi.org/10.1007/978-1-4615-1803-7_7
Publisher Name : Springer, Boston, MA
Print ISBN : 978-1-4613-5728-5
Online ISBN : 978-1-4615-1803-7
eBook Packages : Springer Book Archive
Share this chapter
Anyone you share the following link with will be able to read this content:
Sorry, a shareable link is not currently available for this article.
Provided by the Springer Nature SharedIt content-sharing initiative
- Publish with us
Policies and ethics
- Find a journal
- Track your research
Need immediate assistance? Schedule a meeting with our Sales Team
- Explore All Solutions
- Platform Overview
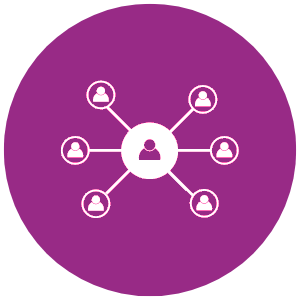
Explore Solutions
Read how you can revolutionize your operation with Knack.
Read Customer Stories
Knack builders are succeeding.
Leverage Knack AI to expedite your new app with just a few sentences and save hours of time.
Safeguard data, control access, and ensure robust system reliability for peace of mind.
Organize, import, and manipulate data efficiently to make informed decisions and drive success.
Integrate data and workflows to enhance productivity and expand functionality effortlessly.
Analyze data, create charts, track performance, and make data-driven decisions with ease.
Control permissions, grant or restrict access, and ensure data security effortlessly.
Sell products, manage inventory, and process payments smoothly for your e-commerce business.
Design your app with the best experience for your users and appearance for your brand.
Automate data handling, trigger notifications, and simplify actions for efficient operations.
Try Interactive Demo
Get a quick taste of Knack.
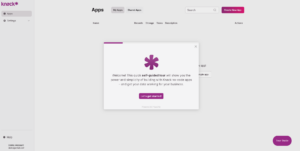
Expedite your app build with a pre-built template.
Discover valuable insights and tips.
Read Knack success stories.
Download and use a spreadsheet template.
Watch testimonials, tutorials, & expert reviews.
See why Knack is the best option.
Find an expert to build or expand your app.
Learn about how to set up and expand your app.
Become a certified Knack Expert.
Find comprehensive guides and documentation.
Discuss your build with other Knack builders.
Increase your revenue with Knack.
Get hired to build and expand apps.
Template Marketplace
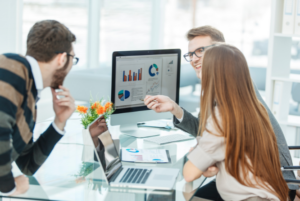
How to Build a Relational Database: A Complete Guide
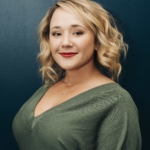
Written By: Cheyenne Kolosky
- April 21, 2024
- The Ultimate Guide to Business Process Automation
- The Comprehensive Guide to Document Management Systems
- How to Implement a Successful Business Process Automation Strategy
- Comparing CRM, Customer Service, and Customer Success: Which Is Right for Your Business?
Relational databases are the workhorses of data storage; they excel at organizing large amounts of information into a structured format, making it easy to store, retrieve, and manage. Whether you’re managing a company’s customer records, tracking inventory in a store, or building a personal library of movies, a relational database can be your secret weapon.
This comprehensive guide is designed to equip you with the knowledge and tools to build a relational database. We’ll outline the technical aspects of creating tables, defining relationships, and querying data and also explore the theoretical foundations of relational database design.
So, whether you’re a seasoned developer or just starting your journey into the world of data, this guide is here to empower you. Let’s dive in and build a solid foundation for your data management needs.
What is a Relational Database?
Definition and significance.
A relational database is a structured system for storing and organizing data in a way that allows for efficient retrieval and manipulation. It follows the relational model, which emphasizes data organization into tables and the establishment of relationships between those tables.
Here’s why relational databases are crucial for data management:
- Organization: They provide a clear and structured way to store large amounts of data, making it easy to find specific information quickly.
- Data Integrity: The relational model enforces data consistency and reduces redundancy, ensuring the accuracy and reliability of your information.
- Scalability: They can efficiently handle large datasets and accommodate growing data volumes.
- Data Sharing: The structured format allows for easy sharing and manipulation of data across different applications and processes.
The Mathematical Foundations
The concept of relational databases is rooted in the mathematical theory of relations. A relational database table can be seen as a mathematical relation, where each row is a tuple, and the columns represent the attributes of that data.
Understanding this connection helps us grasp the core principles of relational databases:
- Tables: Correspond to mathematical relations, with rows and columns representing tuples and attributes.
- Primary Keys: Uniquely identify each row in a table, similar to how mathematical relations avoid duplicate entries.
- Relationships: Established between tables using foreign keys, which link data points across tables, reflecting the connections between sets in mathematical relations.
By leveraging these mathematical concepts, relational databases ensure data organization, minimize redundancy, and enable powerful data manipulation techniques.
Establishing Goals for a Relational Database
Before getting involved in table structures and queries, it’s crucial to establish clear goals for your relational database. Your ideal database should be efficient, adaptable, and perfectly suited to the unique needs of your organization.
Here’s why setting goals is essential:
- Focus: Clearly defined goals help you focus on the data that truly matters for your organization’s needs.
- Efficiency: A targeted database avoids storing unnecessary information, streamlining storage requirements and retrieval processes.
- Scalability: Goals that consider future growth ensure your database can adapt to accommodate evolving data needs.
How to Define Your Database Goals:
- Identify Data Users: Who will be using this database, and for what purposes? Understanding their needs is key. (e.g., Marketing team, Sales department, Customer support)
- Data Requirements: What specific data points are essential to capture and manage?
- Desired Functionality: What kind of operations need to be performed on the data? (e.g., Reporting, Data Analysis, Searching)
- Future Considerations: How might your data needs change over time? Will you need to integrate with other systems?
Understanding Data Relationships
The true power of relational databases lies in their ability to establish connections between different tables.
Here’s a breakdown of the different types of data relationships:
- One-to-One (1:1) : In this relationship, a single record in one table corresponds to exactly one record in another table. This is less common but can be used in specific scenarios.
- One-to-Many (1:N) : This is the most fundamental and widely used relationship. A single record in one table (the “one” side) can be linked to multiple records in another table (the “many” side). This is often achieved through the use of a foreign key, which references the primary key of the “one” side table.
- Many-to-Many (N:N) : Here, multiple records in one table can be associated with multiple records in another table. Relational databases cannot directly represent this relationship, but we can create a workaround using an associative table. This associative table has foreign keys referencing both the original tables and establishes the many-to-many connection.
Understanding these relationships is key to designing an efficient and organized database structure. By properly defining relationships, you can:
- Minimize Data Redundancy: Store data only once and avoid duplication across tables.
- Maintain Data Integrity: Ensure consistency and accuracy of information by linking related data points.
- Simplify Data Retrieval: Perform complex queries that span multiple tables to retrieve the information you need.
Design Principles and Avoiding Common Pitfalls
Now that you understand the core concepts of relational databases, let’s explore some best practices for designing them effectively. Following these principles will ensure your database is functional, efficient, manageable, and future-proof.
Best Practices:
- Normalization: This is a set of techniques to minimize data redundancy and improve data integrity. Normalization involves breaking down tables into smaller, focused tables with well-defined relationships.
- Clear and Consistent Naming: Use descriptive and consistent names for tables, columns, and constraints. This enhances the readability and maintainability of your database.
- Data Types: Choose appropriate data types for each column, such as integers for numbers, dates for time-based data, and text for descriptive information. This ensures data accuracy and efficient storage.
- Constraints: Utilize constraints like primary keys and foreign keys to enforce data integrity and prevent invalid entries.
- Clear Documentation: Document your database design clearly, including table structures, relationships, and the purpose of each field. This is crucial for future maintenance and collaboration.
Common Pitfalls to Avoid:
- Data Duplication: Avoid storing the same data in multiple places. This can lead to inconsistencies and maintenance headaches.
- Poor Data Naming: Cryptic or inconsistent naming conventions can make the database difficult to understand and navigate.
- Inflexible Design: Don’t anticipate every future need, but design with some level of flexibility to accommodate potential growth and changes.
- Security Oversights: Implement proper access controls and security measures to safeguard your sensitive data.
- Lack of Testing: Thoroughly test your database design and queries before deploying them to real-world use cases.
The Role of SQL in Database Creation
SQL (Structured Query Language) is the cornerstone of interacting with relational databases. It’s a powerful and standardized language that allows you to create, manipulate, and retrieve data from your database.
Here’s a glimpse into how SQL empowers you to manage your relational database:
- Database Creation: SQL commands like CREATE TABLE enable you to define the structure of your tables, specifying columns, data types, and constraints.
- Data Manipulation: SQL provides a rich set of commands for inserting, updating, and deleting data within your tables. (e.g., INSERT, UPDATE, DELETE)
- Data Retrieval: The SELECT statement is the heart of data retrieval in SQL. You can use it to extract specific data points or entire rows based on various criteria and filter conditions.
- Data Relationships: SQL allows you to establish relationships between tables using foreign keys. This is often achieved through the FOREIGN KEY constraint within the CREATE TABLE statement.
How to Build a Relational Database
Now that we’ve explored the fundamental concepts and design principles, let’s build a simple relational database using SQL.
Step 1: Define Your Purpose and Data Needs
Here, you’ll identify the purpose of your database and the specific data points you want to manage.
- What kind of information will you be storing? (e.g., Customer information, Product inventory, Library of books)
- Who will be using this database, and how? (e.g., Sales team, Marketing department, Personal reference)
By answering these questions, you can determine the tables you need and the attributes (columns) within those tables.
Step 2: Entity-Relationship Model (ERM) Creation (Optional)
Creating an Entity-Relationship Model (ERM) can be a helpful visualization tool, especially for complex data structures. An ERM is a diagram that represents the entities (tables) in your database and the relationships between them.
Step 3: Building Tables with SQL
Once you have a clear understanding of your data, it’s time to translate that knowledge into SQL commands to create the tables in your database. Here’s a breakdown of the process:
- Use the CREATE TABLE statement: This command defines the structure of your table, specifying its name and the columns it will contain.
- Define Columns: For each column, specify its name, data type (e.g., text, integer, date), and any constraints like primary key or foreign key.
CREATE TABLE Customers (
customer_id INT PRIMARY KEY AUTO_INCREMENT,
first_name VARCHAR(50) NOT NULL,
last_name VARCHAR(50) NOT NULL,
email VARCHAR(100) UNIQUE
This code creates a table named “Customers” with four columns:
- customer_id: An auto-incrementing integer that uniquely identifies each customer (primary key).
- first_name: Customer’s first name (text, not null).
- last_name: Customer’s last name (text, not null).
- email: Customer’s email address (text, unique).
Step 4: Establishing Relationships with Foreign Keys
Now that you have your tables defined, it’s time to establish relationships between them if necessary. Foreign keys are used to link data points across tables, enforcing referential integrity and preventing inconsistencies.
CREATE TABLE Orders (
order_id INT PRIMARY KEY AUTO_INCREMENT,
customer_id INT NOT NULL,
order_date DATE,
FOREIGN KEY (customer_id) REFERENCES Customers(customer_id)
Here, the Orders table is created with a foreign key customer_id that references the primary key of the Customers table. This ensures that each order has a valid customer associated with it.
Step 5: Populating Your Database with Data
With your tables in place, you can start inserting data using the INSERT statement in SQL.
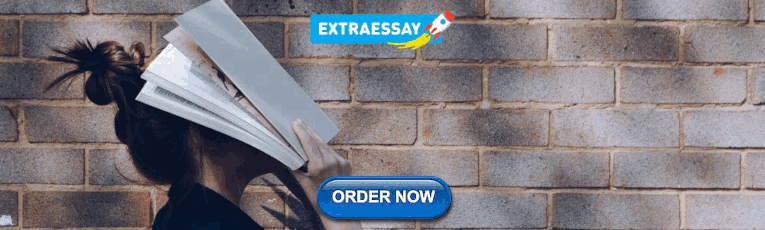
Step 6: Querying Your Database
Finally, the power of your relational database lies in its ability to retrieve specific data. SQL’s SELECT statement allows you to query your database based on various criteria, filtering and sorting data to answer your questions and generate reports.
Identifying Data Requirements:
It’s crucial to identify your data requirements in great detail . This initial phase allows for a structured and efficient database that perfectly aligns with your application or business needs.
Here, we’ll explore a step-by-step process to identify your data requirements:
1. Define the Purpose and Scope:
- What problem are you trying to solve, or what information do you need to manage? (e.g., Tracking customer orders for an e-commerce store, Managing employee information for a company)
- Who will be using this data, and for what purposes? (e.g., Sales team, Marketing department, Human Resources)
2. Identify Entities:
- Entities are the core building blocks of your data model. They represent the real-world objects or concepts you want to store information about. Think of them as the “things” in your data universe. (e.g., In an e-commerce store, entities could be Customers, Products, or Orders)
3. Define Attributes:
- Once you have your entities identified, it’s time to determine the specific characteristics or details you want to capture for each one. These characteristics become the attributes (columns) of your database tables. Ask yourself: What information do you need to know about each entity?
4. Identify Relationships:
- In the real world, entities rarely exist in isolation. They often have connections with each other. Your data model needs to reflect these relationships to accurately represent the information structure. Analyze how your entities interact and identify the nature of those relationships. A Product can be included in many Orders, and an Order can contain multiple Products (Many-to-Many relationship – typically requiring an associative table).)
Tips for Effective Data Requirement Identification:
- Involve Stakeholders: Get input from different departments or users who will be working with the data.
- Start Simple, Iterate Often: Begin with a core set of entities and attributes. As your understanding evolves, refine and expand your data model.
- Document Everything: Clearly document your data requirements, including entity definitions, attribute details, and relationship descriptions.
Defining Database Schema:
Knack’s no-code platform offers a user-friendly interface for building database schemas without writing code. Here’s a step-by-step guide on designing your schema using Knack:
1. Accessing the Knack Builder:
- Log in to your Knack account or create a free trial.
- Click on “ New App ” to initiate the app-building process.
2. Creating Tables:
- Knack uses tables to represent your data entities.
- Click on “ Add Table ” to create a new table.
- Give your table a descriptive name that reflects the entity it represents (e.g., Customers, Products, Orders).
3. Defining Fields (Columns):
- Each table will contain fields that represent the attributes of your entity.
- Click on “ Add Field ” to define a new field.
- Choose the appropriate data type for your field based on the information you want to store (e.g., Text for names, Number for prices, Date for order dates).
- Give your field a clear and concise name that reflects its purpose (e.g., first_name, product_price, order_date).
4. Setting Up Relationships:
- Knack allows you to establish relationships between tables using connected fields.
- To create a relationship, navigate to the table containing the “one” side of the relationship (e.g., the Customers table).
- Click on “ Add Connected Field “.
- Select the table representing the “many” side of the relationship (e.g., Orders table). This creates a foreign key connection.
- Choose the field in the “one” table that will be used for linking (e.g., customer_id in the Customers table).
Optimizing Performance and Scalability:
A well-designed relational database is a powerful tool, but like any tool, it needs proper maintenance. Here are best practices to keep your database running smoothly as your data volume and user base grow.
Enhancing Performance:
- Indexing Key Fields: Indexes act like reference catalogs in a library, allowing for faster data retrieval. Identify frequently used columns in your queries and create indexes on those fields. This significantly improves query execution speed.
- Optimize Queries: Write efficient SQL queries that avoid unnecessary operations or filtering conditions. Analyze slow queries and identify areas for improvement.
- Hardware Optimization: Ensure your database server has sufficient resources (CPU, RAM) to handle the workload. Consider upgrading hardware if performance bottlenecks arise.
Ensuring Scalability:
- Denormalization (Strategic): In some cases, denormalization can improve read performance by duplicating certain data points across tables.
- Archiving Old Data: Don’t overload your database with inactive or historical data. Regularly archive to keep your active tables lean and efficient.
- Horizontal Scaling (Sharding): For massive datasets, consider horizontal scaling. This involves distributing data across multiple servers.
Planning for the Future:
- Choose the Right Database Engine: Select a database engine that fits your specific needs and anticipated data growth. Consider factors like performance, scalability, and available features.
- Design for Growth: While building your schema, factor in potential future needs. Leave room for adding new tables or fields without compromising the overall structure.
- Regular Monitoring: Proactively monitor your database performance and identify potential bottlenecks before they become critical issues. Regularly analyze query execution times, storage usage, and user activity.
Testing and Iteration:
Building a relational database is an iterative process. Just like any software development project, thorough testing and continuous improvement can create a robust and user-friendly system.
Why Testing Matters
- Data Integrity: Testing helps identify data inconsistencies, invalid entries, and potential breaches of referential integrity. This ensures your data remains accurate and reliable.
- Functionality: Verify that your database functions as intended. Test different queries, data manipulation operations, and user workflows to identify any bugs or shortcomings.
- Performance: Evaluate the performance of your database under various load conditions. This helps pinpoint areas for optimization and ensures the system can handle real-world usage.
Testing Strategies:
- Unit Testing: Test individual components of your database schema, such as table structures and queries, in isolation. This helps isolate issues early in the development process.
- Integration Testing: Test how different parts of your database interact with each other, ensuring smooth data flow and consistency across tables.
- User Acceptance Testing (UAT): Involve your end-users in testing the database. Their feedback is invaluable for identifying usability issues and ensuring the system meets their needs effectively.
Choosing and Setting Up Primary Fields
The primary key is a fundamental concept in relational databases. It acts as a unique identifier for each row in a table, ensuring data integrity and efficient data retrieval. Choosing the right primary key is crucial for establishing a solid foundation for your database.
Criteria for Selecting Primary Keys:
- Uniqueness: The primary key value must be unique for every row in the table. No two rows can have the same primary key value.
- Not Null: The primary key field should not allow null values. Every row must have a defined primary key value.
- Simplicity and Efficiency: Ideally, the primary key should be concise and allow for efficient retrieval of data.
Common Primary Key Types:
- Auto-Incrementing Integers: This is a popular choice for primary keys. The database automatically generates a unique integer for each new row, ensuring uniqueness and simplicity. (e.g., customer_id in a Customers table)
- Unique Natural Keys: In some cases, a natural attribute of an entity can serve as a unique identifier. For example, a Social Security number (assuming appropriate privacy considerations) could be a primary key in an Employee table, provided duplicates are strictly controlled.
- Composite Keys: When no single attribute is inherently unique, a combination of two or more attributes can be used as a composite primary key. This is often used for tables linking multiple entities. (e.g., A combination of order_id and product_id in an Order_Details table linking Orders and Products tables)
Problem-Solving Within Database Construction
Relational databases require regular maintenance, so you may need to address issues in your design as your data needs evolve. here’s how:.
- Data Redundancy: Avoid storing the same data in multiple places. Normalize your tables and use foreign keys to create relationships.
- Performance Issues: Optimize queries, create indexes on frequently used fields, and consider hardware upgrades if needed.
- Scalability Challenges: Plan for growth! Denormalize strategically, archive old data, and explore horizontal scaling for massive datasets.
- Testing Oversights: Thoroughly test your database design! Involve users, identify bottlenecks, and iterate based on feedback.
Building a Relational Database with Knack
This comprehensive guide has equipped you with the knowledge and best practices to navigate the world of relational databases. You’ve learned how to:
- Define your data requirements and identify key entities and relationships.
- Design a well-structured schema using Knack’s no-code builder or traditional SQL.
- Optimize your database for performance and scalability to handle growing needs.
- Implement a rigorous testing and iteration process to ensure data integrity and user satisfaction.
Building a database with Knack is far simpler than doing this from scratch. Following our “ working with records ” guide will give you everything you need to know about building your table, fields, and records to start building custom software applications.
Knack uses tables and fields to define your data. Tables are used to separate your data into common groups. You can think of a table like a spreadsheet or a database table. Fields are used to define specific attributes of a table. Think of a field as a spreadsheet column. You’ll want to add a field for each attribute you want to store for a given table.
Once you’ve signed up for Knack, you can access your tables by clicking on the “Data” button in the top left of the Builder (inside your new project):
From here, you can start defining your database records, tables, fields, and overall schema to build your application. This makes the process of building relational databases much easier.
Start building your relational database for free today with Knack!
Relational Database FAQs
What is a relational database, and why is it important for businesses.
A relational database is a type of database that organizes data into tables with rows and columns and establishes relationships between these tables based on common fields. It’s important for businesses because it provides a structured and efficient way to store, manage, and retrieve data.
How does Knack facilitate the building of relational databases?
Knack provides a user-friendly platform that allows users to build custom relational databases without writing any code. With its intuitive drag-and-drop interface and customizable templates, Knack empowers users to design database schemas, define relationships between tables, and create forms and views for data entry and retrieval, all without the need for technical expertise.
What are the key components of a relational database built with Knack?
Key components of a relational database built with Knack include tables, which store data in rows and columns; fields, which represent the attributes of the data stored in each table; relationships, which define connections between tables based on common fields; forms, which allow users to enter and edit data; and views, which display data in different formats for analysis and reporting.
Can I import existing data into a relational database built with Knack?
Yes, Knack allows users to import existing data from various sources, including spreadsheets, CSV files, and other databases, into their relational databases. Users can map fields from their data sources to fields in their Knack databases, enabling them to quickly populate their databases with existing data and start using Knack’s features for data management and analysis.
How scalable are relational databases built with Knack?
Relational databases built with Knack are highly scalable and can accommodate growing data volumes and user bases. Knack offers flexible pricing plans that allow users to scale their databases and applications as needed, with options for additional storage, users, and features.
Create your free account and join thousands of professionals running their businesses with Knack.
The best no-code platform for building custom online databases and applications.
- Data Management
- Integrations
- User Access
- Construction
- Customer Portal
- Event Management
- Grants Management
- Inventory Management
- Online Database
- Project Management
- Case Studies
- Hire an Expert
- Learning Center
- Spreadsheet Templates
- Template Apps
- Community Forum
- Developer Docs
We use cookies to understand how you use our site and to improve your experience. This includes personalizing content and advertising. By continuing to use our site, you accept our use of cookies. To learn more, go to: https://www.knack.com/cookies/
Navigation Menu
Search code, repositories, users, issues, pull requests..., provide feedback.
We read every piece of feedback, and take your input very seriously.
Saved searches
Use saved searches to filter your results more quickly.
To see all available qualifiers, see our documentation .
- Notifications You must be signed in to change notification settings
Welcome to this case study on dissecting and designing products for top leading platforms. In this case study, i will delve into the intriguing world of schema design for a prominent platform linkedin of my choice
Ilu27/Product-Dissection-Relational-Database-SQL---Schema-design-Linkedin
Folders and files.
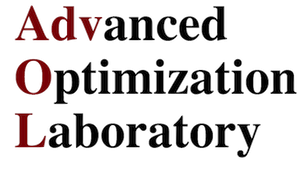
- Publications
- Photogallery
- Internal Pages
- Forgot your username?
- Forgot your password?
- A Suite of Case Studies In Relational Database Design
M.Sc Thesis by Weiguang Zhang(January 2012)
- You are here:

McMaster University
Faculty of Engineering
Faculty of Science
Department of Computing and Software
School Of Computational Science & Engineering
Relational Database Design Case Studies
Here are eight case studies in relational database design. Each case includes a complete explanation and illustrations of fields, tables, key fields, and relationships.
Get full access to Relational Database Design and Implementation, 4th Edition and 60K+ other titles, with a free 10-day trial of O'Reilly.
There are also live events, courses curated by job role, and more.
Relational Database Design and Implementation, 4th Edition
Read it now on the O’Reilly learning platform with a 10-day free trial.
O’Reilly members get unlimited access to books, live events, courses curated by job role, and more from O’Reilly and nearly 200 top publishers.
Book description
Relational Database Design and Implementation: Clearly Explained, Fourth Edition , provides the conceptual and practical information necessary to develop a database design and management scheme that ensures data accuracy and user satisfaction while optimizing performance.
Database systems underlie the large majority of business information systems. Most of those in use today are based on the relational data model, a way of representing data and data relationships using only two-dimensional tables. This book covers relational database theory as well as providing a solid introduction to SQL, the international standard for the relational database data manipulation language.
The book begins by reviewing basic concepts of databases and database design, then turns to creating, populating, and retrieving data using SQL. Topics such as the relational data model, normalization, data entities, and Codd's Rules (and why they are important) are covered clearly and concisely. In addition, the book looks at the impact of big data on relational databases and the option of using NoSQL databases for that purpose.
- Features updated and expanded coverage of SQL and new material on big data, cloud computing, and object-relational databases
- Presents design approaches that ensure data accuracy and consistency and help boost performance
- Includes three case studies, each illustrating a different database design challenge
- Reviews the basic concepts of databases and database design, then turns to creating, populating, and retrieving data using SQL
Table of contents
- Table of Contents
- Preface to the Fourth Edition
- Acknowledgments
- Introduction
- Defining a Database
- Systems that Use Databases
- Data “Ownership”
- Database Software: DBMSs
- Database Hardware Architecture
- Other Factors in the Database Environment
- Open Source Relational DBMSs
- Dealing with Resistance to Change
- The Structured Design Life Cycle
- Conducting the Needs Assessment
- Assessing Feasibility
- Generating Alternatives
- Evaluating and Choosing an Alternative
- Creating Design Requirements
- Alternative Analysis Methods
- Effects of Poor Database Design
- Unnecessary Duplicated Data and Data Consistency
- Data Insertion Problems
- Data Deletion Problems
- Meaningful Identifiers
- The Bottom Line
- Entities and Their Attributes
- Basic Data Relationships
- Documenting Relationships
- Dealing with Many-to-Many Relationships
- Relationships and Business Rules
- Data Modeling Versus Data Flow
- Understanding Relations
- Primary Keys
- Representing Data Relationships
- The Data Dictionary
- A Bit of History
- The Relational Algebra and SQL Example Database: Rare Books
- The Sample Data
- Making Vertical Subsets: Project
- Making Horizontal Subsets: Restrict
- Choosing Columns and Rows: Restrict and Then Project
- Translating an ER Diagram into Relations
- Normal Forms
- First Normal Form
- Second Normal Form
- Third Normal Form
- Boyce–Codd Normal Form
- Fourth Normal Form
- Fifth Normal Form
- Sixth Normal Form
- Partitioning
- Rule 0: The Foundation Rule
- Rule 1: The Information Rule
- Rule 2: The Guaranteed Access Rule
- Rule 3: Systematic Treatment of Null Values
- Rule 4: Dynamic Online Catalog Based on the Relational Model
- Rule 5: The Comprehensive Data Sublanguage Rule
- Rule 6: The View Updating Rule
- Rule 7: High-Level Insert, Update, Delete
- Rule 8: Physical Data Independence
- Rule 9: Logical Data Independence
- Rule 10: Integrity Independence
- Rule 11: Distribution Independence
- Rule 12: Nonsubversion Rule
- A Bit of SQL History
- Conformance Levels
- SQL Environments
- Elements of a SQL Statement
- Database Structure Hierarchy
- Modifying Database Elements
- Deleting Database Elements
- CASE Capabilities
- ER Diagram Reports
- Data Flow Diagrams
- Code Generation
- Sample Input and Output Designs
- The Drawing Environment
- Corporate Overview
- Designing the Database
- Organizational Overview
- The Volunteers Database
- The Animal Tracking Database
- The Merchandising Environment
- Putting Together an ERD
- Creating the Tables
- Generating the SQL
- Revisiting the Sample Data
- Choosing Columns
- Ordering the Result Table
- Choosing Rows
- Nulls and Retrieval: Three-Valued Logic
- SQL Syntax for Inner Joins
- Finding Multiple Rows in One Table: Joining a Table to Itself
- Outer Joins
- Table Constructors in Queries
- Avoiding Joins with Uncorrelated Subqueries
- Negative Queries
- The EXISTS Operator
- The EXCEPT and INTERSECT Operators
- Performing Arithmetic
- String Manipulation
- Date and Time Manipulation
- CASE Expressions
- Set Functions
- Changing Data Types: CAST
- Grouping Queries
- Windowing and Window Functions
- Inserting Rows
- Updating Data
- Deleting Rows
- Inserting, Updating, or Deleting on a Condition: MERGE
- Temporary Tables
- Common Table Expressions (CTEs)
- Creating Indexes
- The Multiuser Environment
- Problems with Concurrent Use
- Solution #1: Classic Locking
- Solution #2: Optimistic Concurrency Control (Optimistic Locking)
- Solution #3: Multiversion Concurrency Control (Timestamping)
- Transaction Isolation Levels
- Web Database Concurrency Control Issues
- Distributed Database Issues
- Sources of External Security Threats
- Sources of Internal Threats
- External Remedies
- Internal Solutions
- Backup and Recovery
- The Bottom Line: How Much Security Do You Need?
- Scope and Purpose of a Data Warehouse
- Obtaining and Preparing the Data
- Data Modeling for the Data Warehouse
- Data Warehouse Appliances
- Why Data Quality Matters
- Recognizing and Handling Incomplete Data
- Recognizing and Handling Incorrect Data
- Recognizing and Handling Incomprehensible Data
- Recognizing and Handling Inconsistent Data
- Employees and Data Quality
- The XML Data Type
- Getting Started: Object-Orientation without Computing
- Basic OO Concepts
- Benefits of Object-Orientation
- Limitations of Pure Object-Oriented DBMSs
- The Object-Relational Data Model
- SQL Support for the OR Data Model
- An Additional Sample Database
- SQL Data Types for Object-Relational Support
- User-Defined Data Types and Typed Tables
- Types of NoSQL Databases
- Other Differences Between NoSQL Databases and Relational Databases
- Benefits of NoSQL Databases
- Problems with NoSQL Databases
- Open Source NoSQL Products
- Appendix A: Historical Antecedents
- Appendix B: SQL Programming
- Appendix C: SQL Syntax Summary
- Subject Index
Product information
- Title: Relational Database Design and Implementation, 4th Edition
- Author(s): Jan L. Harrington
- Release date: April 2016
- Publisher(s): Morgan Kaufmann
- ISBN: 9780128499023
You might also like
Database design for mere mortals®: a hands-on guide to relational database design, third edition.
by Michael J. Hernandez
The #1 Easy, Commonsense Guide to Database Design! Michael J. Hernandez’s best-selling Database Design for Mere …
Database Design for Mere Mortals™: A Hands-On Guide to Relational Database Design, Second Edition
“This book takes the somewhat daunting process of database design and breaks it into completely manageable …
Database Design for Mere Mortals: 25th Anniversary Edition, 4th Edition
The #1 Easy, Commonsense Guide to Database DesignNow Updated Foreword by Michelle Poolet, Mount Vernon Data …
The Data Warehouse Toolkit: The Definitive Guide to Dimensional Modeling, 3rd Edition
by Ralph Kimball, Margy Ross
Updated new edition of Ralph Kimball's groundbreaking book on dimensional modeling for data warehousing and business …
Don’t leave empty-handed
Get Mark Richards’s Software Architecture Patterns ebook to better understand how to design components—and how they should interact.
It’s yours, free.
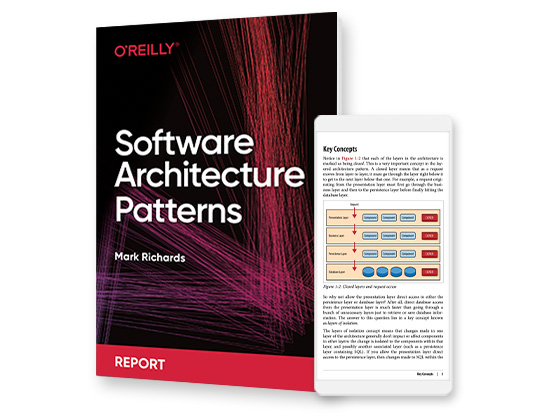
Check it out now on O’Reilly
Dive in for free with a 10-day trial of the O’Reilly learning platform—then explore all the other resources our members count on to build skills and solve problems every day.
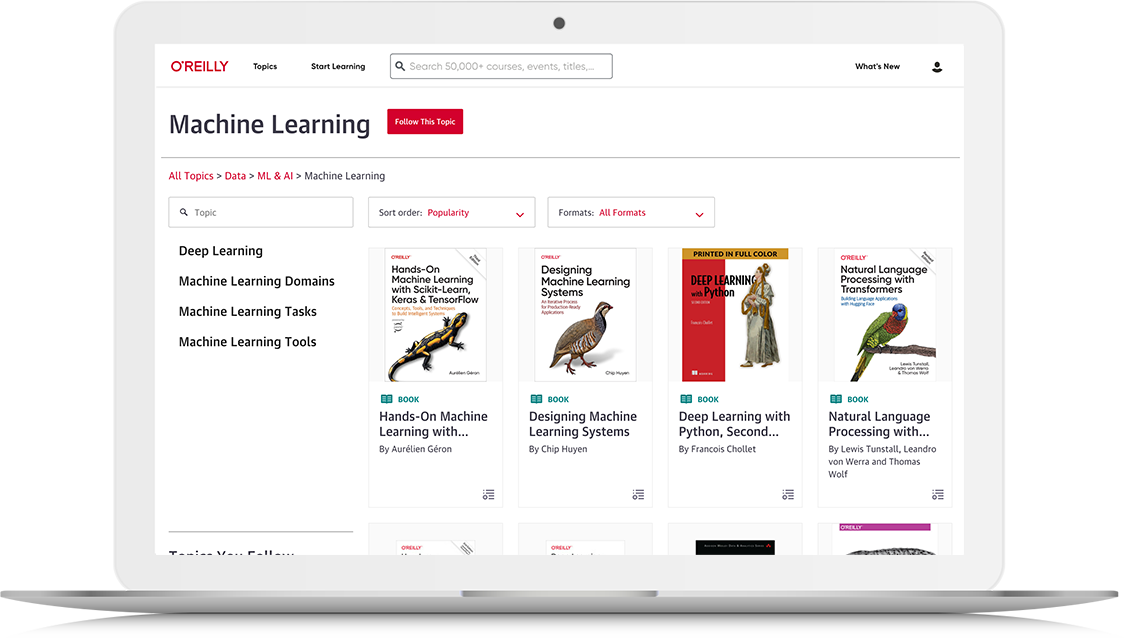
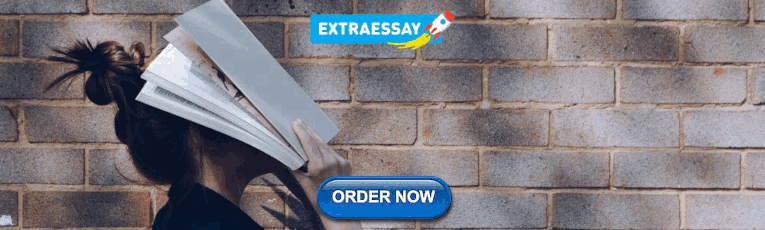
IMAGES
VIDEO
COMMENTS
In this section, we will give a brief overview of the basic concepts about Nosql databases and other aspects that are relevant to our work. 2.1 NoSQL Databases. The problems of the main data are part of a complex context, at the crossroads of 2 major concerns: the adoption of new mass storage solutions, high-speed information collection, preferably in real time.
NoSQL Use Cases: When to Use a Non-Relational Database. For decades, many companies have relied on relational databases to store, protect, and access their data. SQL databases, in particular, worked well for a long time and still do for many use cases. But, today, there are a wide range of situations where SQL databases can no longer satisfy ...
assess its suitability as an alternative to a relational database. The research will look at a case study of a bulletin board application that uses a relational database for data storage and evaluate how such an application can be converted to using a NoSQL database. This case study will also be used to assess the performance attributes of a ...
A typical database management course covers mostly the relational database systems and a significant portion of the practical aspect of any such course deals with the modeling, design and installation of relational databases, and querying of databases using SQL both interactively and via application programs.
Abstract. Relational Database Design and Implementation: Clearly Explained, Fourth Edition, provides the conceptual and practical information necessary to develop a database design and management scheme that ensures data accuracy and user satisfaction while optimizing performance. Database systems underlie the large majority of business ...
A case study in relation data bases. Ce'sar Rodrigues. CCTC. 1 Introduction. This chapter applies the principles behind data refinememt to data base. normalization theory, ie. each normal form ...
In this unit, we learn the semantics of specifying a relational database, later we will learn the syntax of SQL for doing this The basic "datatype", or "variable" of a relational database is a relation. In this unit, such a variable will be a set. Later, we will extend this, and such a variable will be a.
3.1 Reverse Engineering Case Study 1 - Incomplete Database Design A Foreign Exchange (FOREIGN) database resided on a server. Both ER model and database design documentation were not available. A reverse engineering process was carried out on it. A total of 29 entities (database tables) were generated, as shown in Figure 2. Result -Incomplete ...
No double-diamonds here because train number + time uniquely determine a stop. Database design steps: review. •Understand the real-world domain being modeled •Specify it using a database design model (e.g., E/R) •Translate specification to the data model of DBMS (e.g., relational) •Create DBMS schema Next: translating E/R design to ...
Database Design: Case Studies 22.1 INTRODUCTION. From Chapter 6 to Chapter 10, we discussed the concepts of relational database and database design steps. This chapter deals with some of the practical database design projects as case studies using the concepts used in them. Different types of case studies have been considered, covering several ...
Browse; Top Courses; Log In
Relational Database Design and Implementation: Clearly Explained, Fourth Edition, provides the conceptual and practical information necessary to develop a database design and management scheme that ensures data accuracy and user satisfaction while optimizing performance. Database systems underlie the large majority of business information systems.
Click on " New App " to initiate the app-building process. 2. Creating Tables: Knack uses tables to represent your data entities. Click on " Add Table " to create a new table. Give your table a descriptive name that reflects the entity it represents (e.g., Customers, Products, Orders). 3.
A Suite of Case Studies in Relational Database Design. Weiguang Zhang. Published 1 April 2012. Computer Science. TLDR. This chapter discusses the development of ERwin IE Format and its use in the classroom, as well as some of the techniques used to develop and test the system. Expand.
Welcome to this case study on dissecting and designing products for top leading platforms. In this case study, i will delve into the intriguing world of schema design for a prominent platform linkedin of my choice - Ilu27/Product-Dissection-Relational-Database-SQL---Schema-design-Linkedin
Chapter 13 Database Design Case Study #1: Mighty-Mite Motors Abstract This chapter presents the first of three major case studies in the book. ... Get Relational Database Design and Implementation, 4th Edition now with the O'Reilly learning platform. O'Reilly members experience books, live events, courses curated by job role, and more from ...
Size 1.66 MB. Created by Super User. Changed by. Downloads 41. License. Price. Back. Powered by jDownloads. M.Sc Thesis by Weiguang Zhang (January 2012)
After a brief overview of the six-step database design process, three case studies are used to demonstrate how the technique works. Each case study starts with a concise statement of the problem and then goes through each of the six steps that are part of the six-step database design technique, ending with a Crow's Foot relational database model.
Relational databases are tools for storing various types of information that are related to each other in some way. Data engineers build and design relational databases (and other data management systems) to assist organizations in collecting, storing, and analyzing data. Then, data analysts and data scientists use them for digesting large ...
4 Mapping Relational Design to ER/EER Case Study . 3 Session Agenda Session Overview ... Design a relational database schema Based on a conceptual schema design Seven-step algorithm to convert the basic ER model constructs into relations Additional steps for EER model . 8
Here are eight case studies in relational database design. Each case includes a complete explanation and illustrations of fields, tables, key fields, and relationships. Case 1: MC Club Membership (2 tables) Case 2: Social Security Contributions (3 tables) Case 3: Breakfast Cereal Marketing Research (3 tables) Case 4: Boat Rental Reservations (3 ...
Case study 2 •Design a database consistent with the following: •A station has a unique name and an address, and is either an express station or a local station •A train has a unique number and an engineer, and is either an express train or a local train •A local train can stop at any station •An express train only stops at express ...
Book description. Relational Database Design and Implementation: Clearly Explained, Fourth Edition, provides the conceptual and practical information necessary to develop a database design and management scheme that ensures data accuracy and user satisfaction while optimizing performance.. Database systems underlie the large majority of business information systems.
The document provides 4 case studies on database design for different domains: a general case study, banking sector, library management. It includes steps to analyze requirements, identify entities/attributes, develop E-R and R-M diagrams, and transform the models into a relational database design. Students are assigned to identify the key elements - entities, attributes, relationships, keys ...