Society Homepage About Public Health Policy Contact
Data-driven hypothesis generation in clinical research: what we learned from a human subject study, article sidebar.
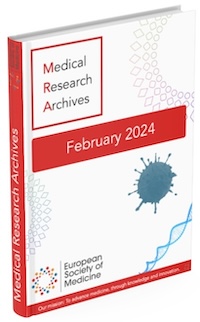
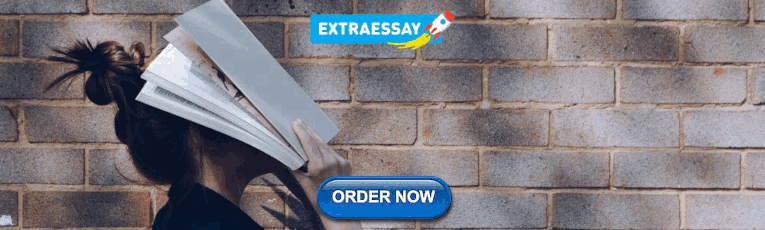
Submit your own article
Register as an author to reserve your spot in the next issue of the Medical Research Archives.
Join the Society
The European Society of Medicine is more than a professional association. We are a community. Our members work in countries across the globe, yet are united by a common goal: to promote health and health equity, around the world.
Join Europe’s leading medical society and discover the many advantages of membership, including free article publication.
Main Article Content
Hypothesis generation is an early and critical step in any hypothesis-driven clinical research project. Because it is not yet a well-understood cognitive process, the need to improve the process goes unrecognized. Without an impactful hypothesis, the significance of any research project can be questionable, regardless of the rigor or diligence applied in other steps of the study, e.g., study design, data collection, and result analysis. In this perspective article, the authors provide a literature review on the following topics first: scientific thinking, reasoning, medical reasoning, literature-based discovery, and a field study to explore scientific thinking and discovery. Over the years, scientific thinking has shown excellent progress in cognitive science and its applied areas: education, medicine, and biomedical research. However, a review of the literature reveals the lack of original studies on hypothesis generation in clinical research. The authors then summarize their first human participant study exploring data-driven hypothesis generation by clinical researchers in a simulated setting. The results indicate that a secondary data analytical tool, VIADS—a visual interactive analytic tool for filtering, summarizing, and visualizing large health data sets coded with hierarchical terminologies, can shorten the time participants need, on average, to generate a hypothesis and also requires fewer cognitive events to generate each hypothesis. As a counterpoint, this exploration also indicates that the quality ratings of the hypotheses thus generated carry significantly lower ratings for feasibility when applying VIADS. Despite its small scale, the study confirmed the feasibility of conducting a human participant study directly to explore the hypothesis generation process in clinical research. This study provides supporting evidence to conduct a larger-scale study with a specifically designed tool to facilitate the hypothesis-generation process among inexperienced clinical researchers. A larger study could provide generalizable evidence, which in turn can potentially improve clinical research productivity and overall clinical research enterprise.
Article Details
The Medical Research Archives grants authors the right to publish and reproduce the unrevised contribution in whole or in part at any time and in any form for any scholarly non-commercial purpose with the condition that all publications of the contribution include a full citation to the journal as published by the Medical Research Archives .
- Business Essentials
- Leadership & Management
- Credential of Leadership, Impact, and Management in Business (CLIMB)
- Entrepreneurship & Innovation
- Digital Transformation
- Finance & Accounting
- Business in Society
- For Organizations
- Support Portal
- Media Coverage
- Founding Donors
- Leadership Team
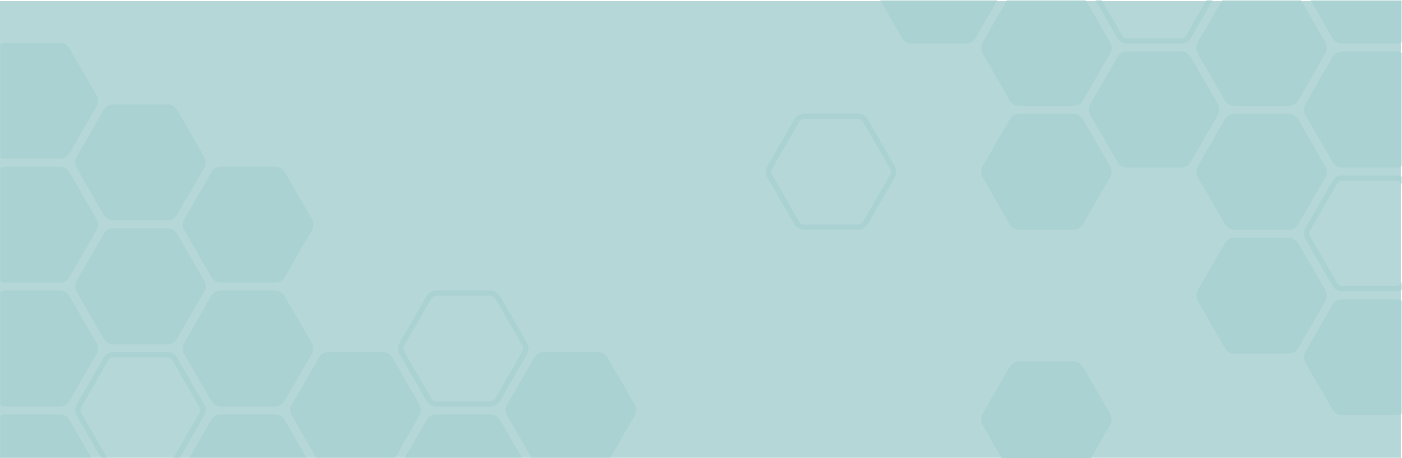
- Harvard Business School →
- HBS Online →
- Business Insights →
Business Insights
Harvard Business School Online's Business Insights Blog provides the career insights you need to achieve your goals and gain confidence in your business skills.
- Career Development
- Communication
- Decision-Making
- Earning Your MBA
- Negotiation
- News & Events
- Productivity
- Staff Spotlight
- Student Profiles
- Work-Life Balance
- AI Essentials for Business
- Alternative Investments
- Business Analytics
- Business Strategy
- Business and Climate Change
- Design Thinking and Innovation
- Digital Marketing Strategy
- Disruptive Strategy
- Economics for Managers
- Entrepreneurship Essentials
- Financial Accounting
- Global Business
- Launching Tech Ventures
- Leadership Principles
- Leadership, Ethics, and Corporate Accountability
- Leading Change and Organizational Renewal
- Leading with Finance
- Management Essentials
- Negotiation Mastery
- Organizational Leadership
- Power and Influence for Positive Impact
- Strategy Execution
- Sustainable Business Strategy
- Sustainable Investing
- Winning with Digital Platforms
A Beginner’s Guide to Hypothesis Testing in Business
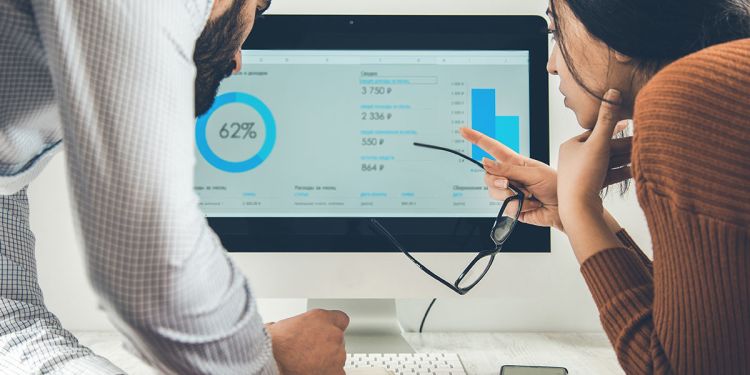
- 30 Mar 2021
Becoming a more data-driven decision-maker can bring several benefits to your organization, enabling you to identify new opportunities to pursue and threats to abate. Rather than allowing subjective thinking to guide your business strategy, backing your decisions with data can empower your company to become more innovative and, ultimately, profitable.
If you’re new to data-driven decision-making, you might be wondering how data translates into business strategy. The answer lies in generating a hypothesis and verifying or rejecting it based on what various forms of data tell you.
Below is a look at hypothesis testing and the role it plays in helping businesses become more data-driven.
Access your free e-book today.
What Is Hypothesis Testing?
To understand what hypothesis testing is, it’s important first to understand what a hypothesis is.
A hypothesis or hypothesis statement seeks to explain why something has happened, or what might happen, under certain conditions. It can also be used to understand how different variables relate to each other. Hypotheses are often written as if-then statements; for example, “If this happens, then this will happen.”
Hypothesis testing , then, is a statistical means of testing an assumption stated in a hypothesis. While the specific methodology leveraged depends on the nature of the hypothesis and data available, hypothesis testing typically uses sample data to extrapolate insights about a larger population.
Hypothesis Testing in Business
When it comes to data-driven decision-making, there’s a certain amount of risk that can mislead a professional. This could be due to flawed thinking or observations, incomplete or inaccurate data , or the presence of unknown variables. The danger in this is that, if major strategic decisions are made based on flawed insights, it can lead to wasted resources, missed opportunities, and catastrophic outcomes.
The real value of hypothesis testing in business is that it allows professionals to test their theories and assumptions before putting them into action. This essentially allows an organization to verify its analysis is correct before committing resources to implement a broader strategy.
As one example, consider a company that wishes to launch a new marketing campaign to revitalize sales during a slow period. Doing so could be an incredibly expensive endeavor, depending on the campaign’s size and complexity. The company, therefore, may wish to test the campaign on a smaller scale to understand how it will perform.
In this example, the hypothesis that’s being tested would fall along the lines of: “If the company launches a new marketing campaign, then it will translate into an increase in sales.” It may even be possible to quantify how much of a lift in sales the company expects to see from the effort. Pending the results of the pilot campaign, the business would then know whether it makes sense to roll it out more broadly.
Related: 9 Fundamental Data Science Skills for Business Professionals
Key Considerations for Hypothesis Testing
1. alternative hypothesis and null hypothesis.
In hypothesis testing, the hypothesis that’s being tested is known as the alternative hypothesis . Often, it’s expressed as a correlation or statistical relationship between variables. The null hypothesis , on the other hand, is a statement that’s meant to show there’s no statistical relationship between the variables being tested. It’s typically the exact opposite of whatever is stated in the alternative hypothesis.
For example, consider a company’s leadership team that historically and reliably sees $12 million in monthly revenue. They want to understand if reducing the price of their services will attract more customers and, in turn, increase revenue.
In this case, the alternative hypothesis may take the form of a statement such as: “If we reduce the price of our flagship service by five percent, then we’ll see an increase in sales and realize revenues greater than $12 million in the next month.”
The null hypothesis, on the other hand, would indicate that revenues wouldn’t increase from the base of $12 million, or might even decrease.
Check out the video below about the difference between an alternative and a null hypothesis, and subscribe to our YouTube channel for more explainer content.
2. Significance Level and P-Value
Statistically speaking, if you were to run the same scenario 100 times, you’d likely receive somewhat different results each time. If you were to plot these results in a distribution plot, you’d see the most likely outcome is at the tallest point in the graph, with less likely outcomes falling to the right and left of that point.

With this in mind, imagine you’ve completed your hypothesis test and have your results, which indicate there may be a correlation between the variables you were testing. To understand your results' significance, you’ll need to identify a p-value for the test, which helps note how confident you are in the test results.
In statistics, the p-value depicts the probability that, assuming the null hypothesis is correct, you might still observe results that are at least as extreme as the results of your hypothesis test. The smaller the p-value, the more likely the alternative hypothesis is correct, and the greater the significance of your results.
3. One-Sided vs. Two-Sided Testing
When it’s time to test your hypothesis, it’s important to leverage the correct testing method. The two most common hypothesis testing methods are one-sided and two-sided tests , or one-tailed and two-tailed tests, respectively.
Typically, you’d leverage a one-sided test when you have a strong conviction about the direction of change you expect to see due to your hypothesis test. You’d leverage a two-sided test when you’re less confident in the direction of change.

4. Sampling
To perform hypothesis testing in the first place, you need to collect a sample of data to be analyzed. Depending on the question you’re seeking to answer or investigate, you might collect samples through surveys, observational studies, or experiments.
A survey involves asking a series of questions to a random population sample and recording self-reported responses.
Observational studies involve a researcher observing a sample population and collecting data as it occurs naturally, without intervention.
Finally, an experiment involves dividing a sample into multiple groups, one of which acts as the control group. For each non-control group, the variable being studied is manipulated to determine how the data collected differs from that of the control group.

Learn How to Perform Hypothesis Testing
Hypothesis testing is a complex process involving different moving pieces that can allow an organization to effectively leverage its data and inform strategic decisions.
If you’re interested in better understanding hypothesis testing and the role it can play within your organization, one option is to complete a course that focuses on the process. Doing so can lay the statistical and analytical foundation you need to succeed.
Do you want to learn more about hypothesis testing? Explore Business Analytics —one of our online business essentials courses —and download our Beginner’s Guide to Data & Analytics .

About the Author
Have a language expert improve your writing
Run a free plagiarism check in 10 minutes, generate accurate citations for free.
- Knowledge Base
Methodology
- How to Write a Strong Hypothesis | Steps & Examples
How to Write a Strong Hypothesis | Steps & Examples
Published on May 6, 2022 by Shona McCombes . Revised on November 20, 2023.
A hypothesis is a statement that can be tested by scientific research. If you want to test a relationship between two or more variables, you need to write hypotheses before you start your experiment or data collection .
Example: Hypothesis
Daily apple consumption leads to fewer doctor’s visits.
Table of contents
What is a hypothesis, developing a hypothesis (with example), hypothesis examples, other interesting articles, frequently asked questions about writing hypotheses.
A hypothesis states your predictions about what your research will find. It is a tentative answer to your research question that has not yet been tested. For some research projects, you might have to write several hypotheses that address different aspects of your research question.
A hypothesis is not just a guess – it should be based on existing theories and knowledge. It also has to be testable, which means you can support or refute it through scientific research methods (such as experiments, observations and statistical analysis of data).
Variables in hypotheses
Hypotheses propose a relationship between two or more types of variables .
- An independent variable is something the researcher changes or controls.
- A dependent variable is something the researcher observes and measures.
If there are any control variables , extraneous variables , or confounding variables , be sure to jot those down as you go to minimize the chances that research bias will affect your results.
In this example, the independent variable is exposure to the sun – the assumed cause . The dependent variable is the level of happiness – the assumed effect .
Prevent plagiarism. Run a free check.
Step 1. ask a question.
Writing a hypothesis begins with a research question that you want to answer. The question should be focused, specific, and researchable within the constraints of your project.
Step 2. Do some preliminary research
Your initial answer to the question should be based on what is already known about the topic. Look for theories and previous studies to help you form educated assumptions about what your research will find.
At this stage, you might construct a conceptual framework to ensure that you’re embarking on a relevant topic . This can also help you identify which variables you will study and what you think the relationships are between them. Sometimes, you’ll have to operationalize more complex constructs.
Step 3. Formulate your hypothesis
Now you should have some idea of what you expect to find. Write your initial answer to the question in a clear, concise sentence.
4. Refine your hypothesis
You need to make sure your hypothesis is specific and testable. There are various ways of phrasing a hypothesis, but all the terms you use should have clear definitions, and the hypothesis should contain:
- The relevant variables
- The specific group being studied
- The predicted outcome of the experiment or analysis
5. Phrase your hypothesis in three ways
To identify the variables, you can write a simple prediction in if…then form. The first part of the sentence states the independent variable and the second part states the dependent variable.
In academic research, hypotheses are more commonly phrased in terms of correlations or effects, where you directly state the predicted relationship between variables.
If you are comparing two groups, the hypothesis can state what difference you expect to find between them.
6. Write a null hypothesis
If your research involves statistical hypothesis testing , you will also have to write a null hypothesis . The null hypothesis is the default position that there is no association between the variables. The null hypothesis is written as H 0 , while the alternative hypothesis is H 1 or H a .
- H 0 : The number of lectures attended by first-year students has no effect on their final exam scores.
- H 1 : The number of lectures attended by first-year students has a positive effect on their final exam scores.
Research question | Hypothesis | Null hypothesis |
---|---|---|
What are the health benefits of eating an apple a day? | Increasing apple consumption in over-60s will result in decreasing frequency of doctor’s visits. | Increasing apple consumption in over-60s will have no effect on frequency of doctor’s visits. |
Which airlines have the most delays? | Low-cost airlines are more likely to have delays than premium airlines. | Low-cost and premium airlines are equally likely to have delays. |
Can flexible work arrangements improve job satisfaction? | Employees who have flexible working hours will report greater job satisfaction than employees who work fixed hours. | There is no relationship between working hour flexibility and job satisfaction. |
How effective is high school sex education at reducing teen pregnancies? | Teenagers who received sex education lessons throughout high school will have lower rates of unplanned pregnancy teenagers who did not receive any sex education. | High school sex education has no effect on teen pregnancy rates. |
What effect does daily use of social media have on the attention span of under-16s? | There is a negative between time spent on social media and attention span in under-16s. | There is no relationship between social media use and attention span in under-16s. |
If you want to know more about the research process , methodology , research bias , or statistics , make sure to check out some of our other articles with explanations and examples.
- Sampling methods
- Simple random sampling
- Stratified sampling
- Cluster sampling
- Likert scales
- Reproducibility
Statistics
- Null hypothesis
- Statistical power
- Probability distribution
- Effect size
- Poisson distribution
Research bias
- Optimism bias
- Cognitive bias
- Implicit bias
- Hawthorne effect
- Anchoring bias
- Explicit bias
Receive feedback on language, structure, and formatting
Professional editors proofread and edit your paper by focusing on:
- Academic style
- Vague sentences
- Style consistency
See an example
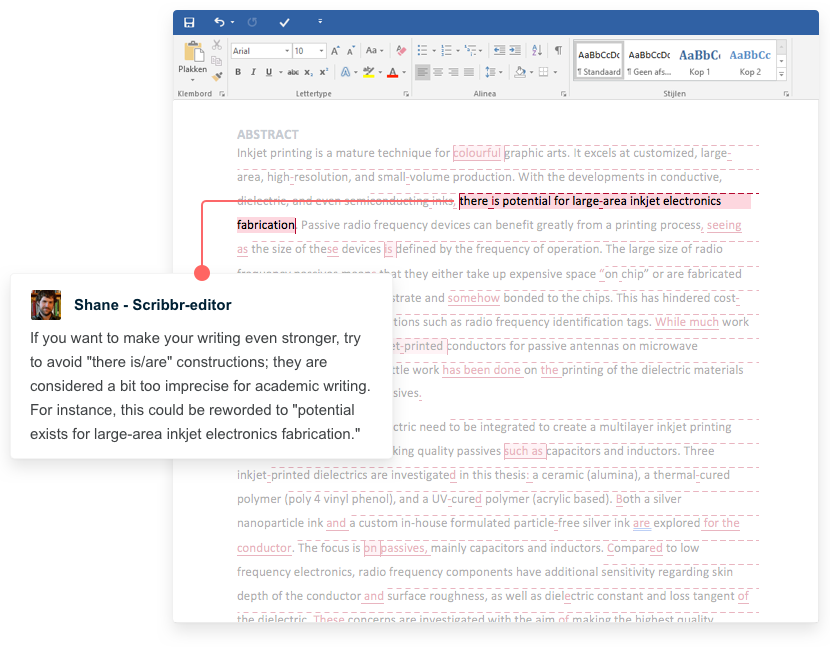
A hypothesis is not just a guess — it should be based on existing theories and knowledge. It also has to be testable, which means you can support or refute it through scientific research methods (such as experiments, observations and statistical analysis of data).
Null and alternative hypotheses are used in statistical hypothesis testing . The null hypothesis of a test always predicts no effect or no relationship between variables, while the alternative hypothesis states your research prediction of an effect or relationship.
Hypothesis testing is a formal procedure for investigating our ideas about the world using statistics. It is used by scientists to test specific predictions, called hypotheses , by calculating how likely it is that a pattern or relationship between variables could have arisen by chance.
Cite this Scribbr article
If you want to cite this source, you can copy and paste the citation or click the “Cite this Scribbr article” button to automatically add the citation to our free Citation Generator.
McCombes, S. (2023, November 20). How to Write a Strong Hypothesis | Steps & Examples. Scribbr. Retrieved June 9, 2024, from https://www.scribbr.com/methodology/hypothesis/
Is this article helpful?
Shona McCombes
Other students also liked, construct validity | definition, types, & examples, what is a conceptual framework | tips & examples, operationalization | a guide with examples, pros & cons, what is your plagiarism score.
- Open access
- Published: 10 October 2012
Approaches to informed consent for hypothesis-testing and hypothesis-generating clinical genomics research
- Flavia M Facio 1 ,
- Julie C Sapp 1 ,
- Amy Linn 1 , 2 &
- Leslie G Biesecker 1
BMC Medical Genomics volume 5 , Article number: 45 ( 2012 ) Cite this article
5599 Accesses
11 Citations
Metrics details
Massively-parallel sequencing (MPS) technologies create challenges for informed consent of research participants given the enormous scale of the data and the wide range of potential results.
We propose that the consent process in these studies be based on whether they use MPS to test a hypothesis or to generate hypotheses. To demonstrate the differences in these approaches to informed consent, we describe the consent processes for two MPS studies. The purpose of our hypothesis-testing study is to elucidate the etiology of rare phenotypes using MPS. The purpose of our hypothesis-generating study is to test the feasibility of using MPS to generate clinical hypotheses, and to approach the return of results as an experimental manipulation. Issues to consider in both designs include: volume and nature of the potential results, primary versus secondary results, return of individual results, duty to warn, length of interaction, target population, and privacy and confidentiality.
The categorization of MPS studies as hypothesis-testing versus hypothesis-generating can help to clarify the issue of so-called incidental or secondary results for the consent process, and aid the communication of the research goals to study participants.
Peer Review reports
Advances in DNA sequencing technologies and concomitant cost reductions have made the use of massively-parallel sequencing (MPS) in clinical research practicable for many researchers. Implementations of MPS include whole genome sequencing and whole exome sequencing, which we consider to be the same, for the purposes of informed consent. A challenge for researchers employing these technologies is to develop appropriate informed consent [ 1 , 2 ], given the enormous amount of information generated for each research participant, and the wide range of medically-relevant genetic results. Most of the informed consent challenges raised by MPS are not novel – what is novel is the scale and scope of genetic interrogation, and the opportunity to develop novel clinical research paradigms.
Massively-parallel sequencing has the capacity to detect nearly any disease-causing gene variant, including late-onset disorders, such as neurologic or cancer-susceptibility syndromes, subclinical disease or endo-phenotypes, such as impaired fasting glucose, and heterozygous carriers of traits inherited in a recessive pattern. Not only is the range of the disorders broad, but the variants have a wide range of relative risks from very high to nearly zero. This is a key distinction of MPS when compared to common SNP variant detection (using so-called gene chips). Because some variants discovered by MPS can be highly penetrant, the detection of such variants can have enormous medical and counseling impact. While many of these informed consent issues have been addressed previously [ 1 , 3 ], the use of MPS in clinical research combines these issues and is on a scale that is orders of magnitude greater than previous study designs.
The initial clinical research uses of MPS were a brute force approach to the identification of mutations for rare mendelian disorders [ 4 ]. This is a variation of positional cloning (also known as gene mapping) and thus a form of classical hypothesis-testing research. The hypothesis is that the phenotype under study is caused by a genetic variant and a suite of techniques is employed (in this case MPS) to identify that causative variant. The application of this technology in this setting is of great promise and will identify causative gene variants for numerous traits, with some predicting that the majority of Mendelian disorders will be elucidated in 5–10 years.
The second of these pathways to discovery is a more novel approach of generating and then sifting MPS results as the raw material to allow the generation of clinical hypotheses, which are in turn used to design clinical experiments to discover the phenotype that is associated with that genotype. This approach we term hypothesis-generating clinical genomics. These hypothesis-generating studies require a consent process that provides the participant with an understanding of scale and scope of the interrogation, which is based on a contextual understanding of the goal and overall organization of the research since specific risks and benefits can be difficult to delineate [ 5 , 6 ]. Importantly, participants need to understand the notion that the researcher is exploring their genomes in an open-ended fashion, that the goal of the experiment is not predictable at the outset, and that the participant will be presented with downstream situations that are not currently foreseeable.
We outline here our approaches to informed consent for our hypothesis-testing and hypothesis-generating MPS research studies. We propose that the consent process be tailored depending on which of these two designs is used, and whether the research aims include study of the return of results.
General issues regarding return of results
Participants in our protocols have the option to learn their potentially clinically relevant genetic variant results. The issue of return of results is controversial and the theoretical arguments for and against the return of results have been extensively debated [ 7 ]. Although an increasing body of literature describes the approaches taken by a few groups no clear consensus exists in either the clinical genomics or bioethics community [ 8 ]. At one end of the spectrum there are those who argue that no results should be returned [ 9 ], and at the other end others contend that the entire sequence should be presented to the research participant [ 10 – 12 ]. In between these extremes lies a qualified or intermediate disclosure policy [ 13 , 14 ]. We take the intermediate position in both of our protocols by giving research participants the choice to receive results, including variants deemed to be clinically actionable [ 3 , 15 ]. Additionally, both protocols are investigating participants’ intentions towards receiving different types of results in order to inform the disclosure policies within the projects and in the broader community [ 16 ]. Because one of our research goals is to study the issues surrounding return of results, it is appropriate and necessary to return results. Thus, the following discussion focuses on issues pertinent to studies that plan to return results.
Issues to consider
Issue #1: primary versus secondary variant results and the open-ended nature of clinical genomics.
In our hypothesis-testing study we distinguish variants as either primary or secondary variants, the distinction reflecting the purpose of the study. A primary variant is a mutation that causes the phenotype that is under study, i.e., the hypothesis that is being tested in the study. A secondary variant is any mutation result not related to the disorder under study, but discovered as part of the quest for the primary variant.
We prefer the term ‘secondary’ to ‘incidental’ because the latter is an adjective indicating chance occurrence, and the discovery of a disease causing mutation by MPS cannot be considered a chance occurrence. The word ‘incidental’ also suggests a lesser degree of importance or impact and it is important to recognize that secondary findings can be of greater medical or personal impact than primary findings.
The consent discussion about results potentially available from participation in a hypothesis-testing study is framed in terms of the study goal, and we assume a high degree of alignment between participants’ goals and the researchers’ aims with respect to primary variants. Participants are, in general, highly motivated to learn the primary variant result and we presume that this motivation contributed to their decision to enroll in the study, similar to motivations for those who have been involved in positional cloning studies. This motivation may not hold for secondary variants, but our approach is to offer them the opportunity to learn secondary and actionable variants that may substantially alter susceptibility to, or reproductive risk for, disease.
In the hypothesis-generating study design no categorical distinction (primary vs. secondary) is made among pathogenic variants, i.e., all variants are treated the same without the label of ‘primary’ or ‘secondary’. This is because we are not using MPS to uncover genetic variants for a specific disease, and any of the variants could potentially be used for hypothesis generation. We suggest that this is the most novel issue with respect to informed consent as the study is open-ended regarding its goals and downstream research activities. This is challenging for informed consent because it is impossible to know what types of hypotheses may be generated at the time of enrollment and consent.
Because the downstream research topics and activities are impossible to predict in hypothesis-generating research, subjects must be consented initially to the open-ended nature of the project. During the course of the study, they must be iteratively re-consented as hypothesis are generated from the genomic data and more specific follow-up studies are designed and proposed to test those newly generated hypotheses. These downstream, iterative consents will vary in their formality, and the degree to which they need to be reviewed and approved. Some general procedures can be approved in advance; for example it may be anticipated that segregation studies would be useful to determine causality for sequence variants or the investigator may simply wish to obtain some additional targeted medical or family history from the research subject. This could be approved prospectively by the IRB with the iterative consent with the subject comprising a verbal discussion of the nature of the trait for which the segregation analysis or additional information is being sought. More specific or more invasive or risky iterative analyses would necessitate review and approval by the IRB with written informed consent.
Informed consent approach
The informed consent process must reflect the fundamental study design distinction of hypothesis-testing versus hypothesis-generating clinical genomics research. For the latter, the challenge is to help the research subjects understand that they are enrolling in a study that could lead to innumerable downstream research activities and goals. The informed consent process must be, like the research, iterative, and involve ongoing communication and consent with respect to those downstream activities.
Issue #2: Volume and nature of information
Whole genome sequencing can elucidate an enormous number of variations for a given individual. A typical whole genome sequence yields ~4,000,000 sequence variations. A whole exome sequence limits the interrogation to the coding regions of genes (about 1–1.5% of the genome) and generates typically 30,000-50,000 gene variants. While most are benign or of unknown consequence, some are associated with a significant increased risk of disease for the individual and/or their family members. For example, the typical human is a carrier for three to five deleterious genetic variants or mutations that cause severe recessive diseases [ 17 , 18 ]. In addition, there are over 30 known cancer susceptibility syndromes, which in aggregate may affect more than 1/500 patients, and the sequence variants that cause these disorders can be readily detected with MPS. These variants can have extremely high relative risks. For some disorders, a rare variant can be associated with a relative risk of greater than 1,000. This is in contrast with common SNP typing which detects variants associated with small relative risks (typically on the order of 1.2-1.5). It is arguable whether the latter type of variant has any clinical utility as an individual test.
Conveying the full scope of genomic interrogation planned for each sample and the volume of information generated for a given participant is impossible. The goal and challenge in this instance is to give the participant as realistic a picture as possible of the likely amount of clinically actionable results the technology can generate. Our approach is two-fold: to give the subjects the clear message that the number and nature of the findings is enormous and literally impossible to describe in a comprehensive manner and to use illustrative examples of the spectrum of these results.
To provide examples, we bin genetic variants into broad categories, as follows: heterozygous carriers of genetic variants implicated in recessive conditions (e.g., CFTR p.Phe508del and cystic fibrosis); variants that cause a treatable disorder that may be present, but asymptomatic or undiagnosed (e.g., LDLR p.Trp87X, familial hypercholesterolemia); variants that predispose to later-onset conditions (e.g., BRCA2 c.5946delT (commonly known as c.6174delT), breast and ovarian cancer susceptibility); variants that predispose to late-onset but untreatable disorders (e.g., frontotemporal dementia MAPT p.Pro301Leu).
Additionally, the scale and scope of the results determines a near certainty that all participants will be found to harbor disease-causing mutations. This is because the interrogation of all genes brings to light the fact that the average human carries 3–5 recessive deleterious genes in addition to the risks for later onset or incompletely penetrant dominant disorders. This reality can be unsettling and surprising to research subjects and we believe it is important to address this early in the process, not downstream in the iterative phase. It is essential for the participants to choose whether MPS research is appropriate for them, taking into account their personal views and values.
Communicate to participants both the overwhelming scale and scope of genetic results they may opt to receive and provide them with specific disease examples that illustrate the kinds of decisions they may need to make as the results become available. These examples should also assist the research subjects in making a decision about whether to participate in the study and if so, the kinds of decisions they may need be making in the future as results become available.
Issue #3: Return of individual genotype results
The return of individual genotype results from MPS presents a new challenge in the clinical research environment, again because of the scale and breadth of the results. The genetic and medical counseling can be challenging because of the volume of results generated, participants’ expectations, the many different categories of results, and the length of time for the information to be available. We suggest that the most reasonable practice is to take a conservative approach and disclose only clinically actionable results. To this end, the absence of a deleterious gene variant (or a negative result) would not be disclosed to research participants. It is our understanding that it is mandatory to validate any individual results that are returned to research subjects in a CLIA-certified laboratory. Using current clinical practice as a standard or benchmark, we suggest that until other approaches are shown to be appropriate and effective, disclosure should take place during a face-to-face encounter involving a multidisciplinary team (geneticist, genetic counselor, and specialists on an ad-hoc basis based on the phenotype in question).
During the initial consent, participants are alerted to the fact that in the future the study team will contact them by telephone and their previously-stated preferences and impressions about receiving primary and secondary variant results will be reviewed. The logistics and details of this future conversation feature prominently in the initial informed consent session, as it is challenging to make and to receive such calls. Participants make a choice to learn or not learn a result each time a result becomes available. Once a participant makes the decision to learn a genotype result, the variant is confirmed in a CLIA lab, and a report is generated. The results are communicated to the participant during a face-to-face meeting with a geneticist and genetic counselor, and with the participation of other specialists depending on the case and the participant’s preferences. These phone discussions are seen as an extension of the initial informed consent process and as opportunities for the participants to make decisions in a more relevant and current context (compared to the original informed consent session). We see this as an iterative approach to consent, also known as circular consent [ 5 ]. Participants who opt not to learn a specific result can still be contacted later if other results become available, unless they choose not to be contacted by us any longer.
This approach to returning results is challenged by the hypothesis-generating genomics research approach. Participants in our hypothesis-testing protocol are not asked to make a decision about learning individual genotype results at the time of consent. This is because we cannot know the nature of the future potential finding at the time of the original consent. Rather, they are engaged in a discussion of what they currently imagine their preferences might be at some future date, again using exemplar disorders and hypothetical scenarios of hypothesis-generating studies.
In the hypothesis-generating study, we have distinct approaches for variants in known disease-causing genes versus variants in genes that are hypothesized to cause disease (the latter being the operative hypothesis generating activity). For the former, the results are handled in a manner quite similar to the hypothesis-testing study. In the latter case, the participant may be asked if they would be willing to return for further phenotyping to help us determine the nature of the variant of uncertain clinical significance (VUCS). The participant is typically informed that they have a sequence variant and that we would like to learn, through clinical research whether this variant has any phenotypic or clinical significance. It is emphasized that current knowledge does not show that the variant causes any phenotype and the chances are high that the variant is benign. However, neither the gene nor the sequence variant is disclosed and the research finding is not confirmed in a CLIA certified lab. This type of VUCS would only be communicated back to the participant if the clinical research showed that the variant was causative, and the return of the result was determined medically appropriate by our Mutation Advisory Committee, and following confirmation in a CLIA-certified laboratory.
For the return of mutations in known, disease causing genes, the initial consent cannot comprehensively inform subjects of the nature of the diseases, because of the scale and scope of the potential results. Instead, exemplars are given to elicit general preferences, which are then affirmed or refined at the time results are available. Hypothesis-generating studies require that subjects receive sufficient information to make an informed choice about participation in the specific follow-up study, with return of individual results only if the cause and effect relationship is established, with appropriate oversight.
Issue #4: Duty to warn
Given the breadth of MPS gene interrogation, it is reasonable to anticipate that occasional participants may have mutations that pose a likely severe negative consequence, which we classify as “panic” results. This models clinical and research practice for the return of results such as a pulmonary mass or high serum potassium level. In contrast to the above-mentioned autosomal recessive carrier states that are expected to be nearly universal, genetic panic results should be uncommon. However, they should not be considered as unanticipated – it is obvious that such variants will be detected and the informed consent process should anticipate these. Examples would be deleterious variants for malignant hyperthermia or Long QT Syndrome, either of which have a substantial risk of sudden death and the risk can be mitigated.
Both our hypothesis-testing and hypothesis-generating studies include mechanisms for the participants to indicate the types of results that they wish to have returned to them. In the hypothesis-testing mode of research this is primarily to respect the autonomy of the participants, but in addition, for the hypothesis-generating study we are assessing the motivations and interests of the subjects in various types of results and manipulating the return of results as an experimental aim. It is our clinical research experience that participants are challenged by making decisions regarding possible future results that are rare, but potentially severe. As well, the medical and social contexts of the subjects evolves over time and the consent that was obtained at enrollment may not be relevant or appropriate at a later time when such a result arises. This is particularly relevant for a research study that is ongoing for substantial periods of time (see also point #7, below).
To address these issues we have consented the subjects to the potential return of “panic” results, irrespective of their preferences at the initial consent session. In effect, the consent process is for some participants a consent to override their preference.
In both hypothesis-testing and hypothesis-generating research it is important to outline circumstances in which researchers’ duty-to-warn may result in a return of results that may be contrary to the preferences of the subject. It is essential that the subjects understand this approach to unusually severe mutation results. Subjects who are uncomfortable with this approach to return of results are encouraged to decline enrollment.
Issue #5: Length of researcher and participant interaction
Approaches to MPS data are evolving rapidly and it is anticipated that this ongoing research into the significance of DNA variants will continue for years or decades. The different purposes of the two study designs lead to different endpoints in terms of researcher’s responsibility to analyze results. In our hypothesis-testing research, discussion of the relationship of the participants to the researchers is framed in terms of the discovery of the primary variant. We ask participants to be willing to interact with us for a period of months or years as it is impossible for to set a specific timeline to determine the cause of the disorder under investigation (if it ever discovered). While attempts to elucidate the primary variant are underway, participants’ genomic data are periodically annotated using the most current bioinformatic methodologies available. We conceptualize our commitment to return re-annotated and updated results to participants as diminishing, but not disappearing, after this initial results’ disclosure. As the primary aim of the study has been accomplished, less attention will be directed to the characterization of ancillary genomic data, yet we believe we retain an obligation to share highly clinically actionable findings with participants should we obtain them.
In the hypothesis-generating study the researcher’s responsibility to annotate participants’ genomes/exomes is ongoing. This is ongoing because, as noted above, one of the experimental aims is to study the motivations and interests of the subjects in these types of results. Determining how this motivation and interest fares over time is an important research goal. During the informed consent discussion it is emphasized that the iterative nature of result interpretation will lead to multiple meetings for the disclosure of clinically actionable results, and that the participant may be contacted months or years after the date of enrollment. Additionally, it is outlined that the participant will make a choice about learning the result each time he/she is re-contacted about the availability of a research finding, and that finding will only be confirmed in a CLIA-certified laboratory if the participant opts to learn the information. Participants who return to discuss results are reminded that they will be contacted in the future if and when other results deemed to be clinically actionable are found for that individual.
Describe nature, mutual commitments, and duration of researcher-participant relationship to participants. For hypothesis-testing studies it is appropriate that the intensity of the clinical annotation of secondary variants may decline when the primary goal of the study is met. For hypothesis-generating studies, such interactions may continue for as long as there are variants to be further evaluated and as long as the subject retains an interest in the participation.
Issue #6: Target population
The informed consent process needs to take into account the target population in terms of their disease phenotype, age, and whether the goal is to enroll individual participants or families. These considerations represent the greatest divergence in approaches to informed consent when comparing hypothesis-testing and hypothesis-generating research. In our two studies, the hypothesis-testing study focuses on rare diseases and often family participation, whereas the hypothesis-generating study focuses on more common diseases and unrelated index cases. There are an infinite number of study designs and investigators may adapt our approaches to informed consent for their own designs.
Our hypothesis-testing protocol enrolls both individual participants and families (most commonly trios), the latter being more common. In hypothesis-testing research, many participants are either affected by a genetic disease or are a close relative (typically a parent) of a person with a genetic disease. The research participants must weigh their hope for, and personal meaning ascribed to, learning the genetic cause for their disorder against the possibility of being in a position to learn a significant amount of unanticipated information. Discussing and addressing the potential discrepancy of the participants’ expectations of the value of their results and what they may realistically stand to learn (both desired and undesired information) is a central component of the informed consent process.
In our hypothesis-testing protocol, when parents are consenting on behalf of a minor child, we review with them the issues surrounding genetic testing of children and discuss their attitudes regarding their child’s autonomy and their parental decision-making values. Because family trios (most often mother-father-child) are enrolled together, we discuss how one individual’s preferences regarding results may be disrupted or superseded by another family member’s choice and communication of that individual’s knowledge.
In contrast, our hypothesis-generating protocol enrolls as probands or primary participants older, unrelated individuals [ 19 ]. Most participants are self-selected in terms of their decision to enroll and are not enrolled because they or a relative have a rare disease. Participants in the hypothesis-generating protocol are consented for future exploration of any and all possible phenotypes. This is a key distinguishing feature of this hypothesis-generating approach to research, which is a different paradigm – going from genotype to phenotype. The participants may be invited for additional phenotyping. In fact, multiple satellite studies are ongoing to evaluate various subsets of participants for different phenotypes. The key with the consent for these subjects is to initially communicate to the subjects the general approach – that their genome will be explored, variations will be identified, and they may be re-contacted for a potential follow-up study to understand the potential relationship of that variant to their phenotype. These subsequent consents for follow-up studies are considered an iterative consent process, which is similar to the Informed Cohort concept [ 20 ].
Hypothesis-generating research is a novel approach to clinical research design and requires an ongoing, iterative approach to informed consent. For hypothesis-testing research a key informed consent issue is for the subjects to balance the desire for information on the primary disease causing mutation with the pros and cons of obtaining possibly undesired information on secondary variants.
Issue #7: Privacy and confidentiality
In MPS studies, privacy and confidentiality is a complex and multifaceted issue. Some potential challenges include: the deposition of genetic and phenotypic data in public databases, the placement of CLIA-validated results in the individual’s medical chart, and the discovery of secondary variants in relatives of affected probands in family-based (typically hypothesis-testing) research.
The field of genomics has a tradition of deposition of data in publicly accessible databases. Participants in our protocols are informed that the goal of sharing de-identified information in public databases is to advance research, and that there are methods in place maximize the privacy and confidentiality of personally identifiable information. However, the deposition of genomic-scale data for an individual participant, such as a MPS sequence, is far above the minimal amount of data to uniquely identify the sample [ 21 , 22 ]. Therefore, the participants should be made aware that the scale of the data could allow analysts to connect sequence data to individuals by matching variants in the deposited research data to other data from that person. As well, the public deposition of data in some cases is an irrevocable decision. Once the data are deposited and distributed, it may be impossible to remove the data from all computer servers, should the subject decide to withdraw from the study.
Additionally, participants are informed that once a result is CLIA-certified, that result is placed in the individual’s medical chart of the clinical research institution and may be accessible by third parties. Although there are state and federal laws to protect individuals against genetic discrimination, including GINA, this law has not yet been tested in the courts. This is explained to participants up front at the time of enrollment and a more detailed discussion takes place at the time of results disclosure. To offer additional protection in the event of a court subpoena, a Certificate of Confidentiality has been obtained in the hypothesis-testing and hypothesis-generating protocols. The discussion surrounding privacy and confidentiality is approached in a similar manner in both protocols.
The third issue regarding confidentiality is that MPS can generate many results in each individual and it is highly likely that some, if not all, of the variants detected in one research participant may be present in another research participant (e.g., a parent). This is again a consequence of the scale and breadth of MPS in that the large number of variants that can be detected in each participant makes it exceedingly likely that their relatives share many of these variants and that their genetic risks of rare diseases may be measurably altered. It is important to communicate to the participants that it is likely that such variants can be detected and that they may have implications for other members of the family, and that the consented individuals, or their parent may need to communicate those results to other members of the family.
The informed consent should include discussion of public deposition of data, the entry of CLIA-validated results into medical records, and the likely discovery of variants with implications for family members.
We describe an approach to the informed consent process as a mutual opportunity for researchers and participants to assess one another’s goals in MPS protocols that employ both hypothesis-generating and hypothesis-testing methodologies. The use of MPS in clinical research requires adaptation of established processes of human subjects protections. The potentially overwhelming scale of information generated by MPS necessitates that investigators and IRBs adapt traditional approaches to consent the subjects. Because nearly all subjects will have a clinically actionable result, investigators must implement thoughtful plan for consent regarding results disclosure, including setting a threshold for the types of information that should be disclosed to the participants.
While some of the informed consent issues for MPS are independent of the study design, others should be adapted based on whether the research study is employing MPS to test a hypothesis (i.e., find the cause of a rare condition in an affected cohort), or to generate hypotheses (i.e., find deleterious or potentially deleterious variants that warrant participant follow-up and further investigation). For example, the health-related attributes of the study cohort (healthy individuals versus disease patients) are likely to influence participants’ motivations and expectations of MPS, and in the case of a disease cohort create the need to dichotomize the genetic variants into primary and secondary. Conversely, issues inherent to MPS technology are central to the informed consent approach in both types of studies. The availability of MPS allows a paradigm shift in genetics research – no longer are investigators constrained to long-standing approaches of hypothesis-testing modes of research. The scale of MPS allows investigators to proceed from genotype to phenotype, and leads to new challenges for genetic and medical counseling. Research participants receiving results from MPS might not present with a personal and/or family history suggestive of conditions revealed by their genotypic variants, and consequently might not perceive their a priori risk to be elevated for those conditions.
Participants’ motivations to have whole genome/exome sequencing at this early stage are important to take into consideration in the informed consent process. Initial qualitative data suggest that individuals enroll in the hypothesis-generating study because of altruism in promoting research, and a desire to learn about genetic factors that contribute to their own health and disease risk [ 23 ]. Most participants expect that genomic information will improve the overall knowledge of disease causes and treatments. Moreover, data on research participants’ preferences to receive different types of genetic results suggest that they have strong intentions to receive all types of results [ 16 ]. However, they are able to discern between the types and quality of information they could learn, and demonstrate stronger attitudes to learn clinically actionable and carrier status results when compared to results that are uncertain or not clinically actionable. These findings provide initial insights into the value these early adopters place on information generated by high-throughput sequencing studies, and help us tailor the informed consent process to this group of individuals. However, more empirical data are needed to guide the informed consent process, including data on research participants’ ability to receive results for multiple disorders and traits.
Participants in both types of studies are engaged in a discussion of the complex and dynamic nature of genomic annotation so that they may make an informed decision about participation and may be aware of the need to revisit results learned at additional time points in the future. As well, we advocate a process whereby investigators retain some latitude with respect to the most serious, potentially life-threatening mutations. While it is mandatory to respect the autonomy of research subjects, this does not mean that investigators must accede to the research subject’s views of these “panic” results. In a paradoxical way, the research participant and the researcher can agree that the latter can maintain a small, but initially ambiguous degree of latitude with respect to these most serious variants. In the course of utilizing MPS technology for further elucidation of the genetic architecture of health and disease, it is imperative that research participants and researchers be engaged in a continuous discussion about the state of scientific knowledge and the types of information that could potentially be learned from MPS. Although resource-intensive, this “partnership model” [ 2 ] or informed cohort approach to informed consent promotes respect for participants, and allows evaluation of the benefits and harms of disclosure in a more timely and relevant manner.
We have here proposed a categorization of massively-parallel clinical genomics research studies as hypothesis-testing versus hypothesis-generating to help clarify the issue of so-called incidental or secondary results for the consent process, and aid the communication of the research goals to study participants. By using this categorization approach and considering seven important features of this kind of research (Primary versus secondary variant results and the open-ended nature of clinical genomics, Volume and nature of information, Return of individual genotype results, Duty to warn, Length of researcher and participant interaction, Target population, and Privacy and confidentiality) researchers can design an informed consent process that is open, transparent, and appropriately balances risks and benefits of this exciting approach to heritable disease research.
This study was supported by funding from the Intramural Research Program of the National Human Genome Research Institute. The authors have no conflicts to declare.
Netzer C, Klein C, Kohlhase J, Kubisch C: New challenges for informed consent through whole genome array testing. J Med Genet. 2009, 46: 495-496. 10.1136/jmg.2009.068015.
Article CAS PubMed Google Scholar
McGuire AL, Beskow LM: Informed consent in genomics and genetic research. Annu Rev Genomics Hum Genet. 2010, 11: 361-381. 10.1146/annurev-genom-082509-141711.
Article CAS PubMed PubMed Central Google Scholar
Bookman EB, Langehorne AA, Eckfeldt JH, Glass KC, Jarvik GP, Klag M, Koski G, Motulsky A, Wilfond B, Manolio TA, Fabsitz RR, Luepker RV, NHLBI Working Group: Reporting genetic results in research studies: Summary and recommendations of an NHLBI Working Group. Am J Med Genet A. 2006, 140: 1033-1040.
Article PubMed PubMed Central Google Scholar
Ng PC, Kirkness EF: Whole genome sequencing. Methods Mol Biol. 2010, 628: 215-226. 10.1007/978-1-60327-367-1_12.
Mascalzoni D, Hicks A, Pramstaller P, Wjst M: Informed consent in the genomics era. PLoS Med. 2008, 5: e192-10.1371/journal.pmed.0050192.
Rotimi CN, Marshall PA: Tailoring the process of informed consent in genetic and genomic research. Genome Med. 2010, 2: 20-10.1186/gm141.
Bredenoord AL, Kroes HY, Cuppen E, Parker M, van Delden JJ: Disclosure of individual genetic data to research participants: the debate reconsidered. Trends Genet. 2011, 27: 41-47. 10.1016/j.tig.2010.11.004.
Kronenthal C, Delaney SK, Christman MF: Broadening research consent in the era of genome-informed medicine. Genet Med. 2012, 14: 432-436. 10.1038/gim.2011.76.
Article PubMed Google Scholar
Forsberg JS, Hansson MG, Eriksson S: Changing perspectives in biobank research: from individual rights to concerns about public health regarding the return of results. Eur J Hum Genet. 2009, 17: 1544-1549. 10.1038/ejhg.2009.87.
Shalowitz DI, Miller FG: Disclosing individual results of clinical research: implications of respect for participants. JAMA. 2005, 294: 737-740. 10.1001/jama.294.6.737.
Fernandez CV, Kodish E, Weijer C: Informing study participants of research results: an ethical imperative. IRB. 2003, 25: 12-19.
McGuire AL, Lupski JR: Personal genome research: what should the participant be told?. Trends Genet. 2010, 26: 199-201. 10.1016/j.tig.2009.12.007.
Wolf SM, Lawrenz FP, Nelson CA, Kahn JP, Cho MK, Clayton EW, Fletcher JG, Georgieff MK, Hammerschmidt D, Hudson K, Illes J, Kapur V, Keane MA, Koenig BA, Leroy BS, McFarland EG, Paradise J, Parker LS, Terry SF, Van Ness B, Wilfond BS: Managing incidental findings in human subjects research: analysis and recommendations. J Law Med Ethics. 2008, 36: 219-248. 10.1111/j.1748-720X.2008.00266.x.
Kohane IS, Taylor PL: Multidimensional results reporting to participants in genomic studies: Getting it right. Sci Transl Med. 2010, 2: 37cm19-10.1126/scitranslmed.3000809.
Fabsitz RR, McGuire A, Sharp RR, Puggal M, Beskow LM, Biesecker LG, Bookman E, Burke W, Burchard EG, Church G, Clayton EW, Eckfeldt JH, Fernandez CV, Fisher R, Fullerton SM, Gabriel S, Gachupin F, James C, Jarvik GP, Kittles R, Leib JR, O'Donnell C, O'Rourke PP, Rodriguez LL, Schully SD, Shuldiner AR, Sze RK, Thakuria JV, Wolf SM, Burke GL, National Heart, Lung, and Blood Institute working group: Ethical and practical guidelines for reporting genetic research results to study participants: updated guidelines from a national heart, lung, and blood institute working group. Circ Cardiovasc Genet. 2010, 3: 574-580. 10.1161/CIRCGENETICS.110.958827.
Facio FM, Fisher T, Eidem H, Brooks S, Linn A, Biesecker LG, Biesecker BB: Intentions to receive individual results from whole-genome sequencing among participants in the ClinSeqTM study. Eu J Hum Genet. in press
Morton NE: The detection and estimation of linkage between the genes for elliptocytosis and the Rh blood type. Am J Hum Genet. 1956, 8: 80-96.
CAS PubMed PubMed Central Google Scholar
Morton NE: The mutational load due to detrimental genes in man. Am J Hum Genet. 1960, 12: 348-364.
Biesecker LG, Mullikin JC, Facio FM, Turner C, Cherukuri PF, Blakesley RW, Bouffard GG, Chines PS, Cruz P, Hansen NF, Teer JK, Maskeri B, Young AC, Manolio TA, Wilson AF, Finkel T, Hwang P, Arai A, Remaley AT, Sachdev V, Shamburek R, Cannon RO, Green ED, NISC Comparative Sequencing Program: The ClinSeq Project: piloting large-scale genome sequencing for research in genomic medicine. Genome Res. 2009, 19: 1665-1674. 10.1101/gr.092841.109.
Kohane IS, Mandl KD, Taylor PL, Holm IA, Nigrin DJ, Kunkel LM: Medicine. Reestablishing the researcher-patient compact. Science. 2007, 316: 836-837. 10.1126/science.1135489.
Lin Z, Owen AB, Altman RB: Genomic Research and Human Subject Privacy. Science. 2004, 305: 183-10.1126/science.1095019.
Homer N, Szelinger S, Redman M, Duggan D, Tembe W, Muehling J, Pearson JV, Stephan DA, Nelson SF, Craig DW: Resolving individuals contributing trace amounts of DNA to highly complex mixtures using high-density SNP genotyping microarrays. PLoS Genet. 2008, 29: e1000167.
Article Google Scholar
Facio FM, Brooks S, Loewenstein J, Green S, Biesecker LG, Biesecker BB: Motivators for participation in a whole-genome sequencing study: implications for translational genomics research. Eur J Hum Genet. 2011, 19: 1213-1217. 10.1038/ejhg.2011.123.
Pre-publication history
The pre-publication history for this paper can be accessed here: http://www.biomedcentral.com/1755-8794/5/45/prepub
Download references
Author information
Authors and affiliations.
National Human Genome Research Institute, National Institutes of Health, Bethesda, MD, USA
Flavia M Facio, Julie C Sapp, Amy Linn & Leslie G Biesecker
Kennedy Krieger Institute, Baltimore, MD, USA
You can also search for this author in PubMed Google Scholar
Corresponding author
Correspondence to Leslie G Biesecker .
Additional information
Competing interests.
LGB is an uncompensated consultant to, and collaborates with, the Illumina Corp.
Authors’ contributions
FMF and JCS drafted the initial manuscript. LGB Organized and edited the manuscript. All authors read and approved the final manuscript.
Rights and permissions
Open Access This article is published under license to BioMed Central Ltd. This is an Open Access article is distributed under the terms of the Creative Commons Attribution License ( https://creativecommons.org/licenses/by/2.0 ), which permits unrestricted use, distribution, and reproduction in any medium, provided the original work is properly cited.
Reprints and permissions
About this article
Cite this article.
Facio, F.M., Sapp, J.C., Linn, A. et al. Approaches to informed consent for hypothesis-testing and hypothesis-generating clinical genomics research. BMC Med Genomics 5 , 45 (2012). https://doi.org/10.1186/1755-8794-5-45
Download citation
Received : 07 November 2011
Accepted : 05 October 2012
Published : 10 October 2012
DOI : https://doi.org/10.1186/1755-8794-5-45
Share this article
Anyone you share the following link with will be able to read this content:
Sorry, a shareable link is not currently available for this article.
Provided by the Springer Nature SharedIt content-sharing initiative
- Whole genome sequencing
- Whole exome sequencing
- Informed consent
BMC Medical Genomics
ISSN: 1755-8794
- Submission enquiries: [email protected]
- General enquiries: [email protected]

- About the LSE Impact Blog
- Comments Policy
- Popular Posts
- Recent Posts
- Subscribe to the Impact Blog
- Write for us
- LSE comment
February 3rd, 2016
Putting hypotheses to the test: we must hold ourselves accountable to decisions made before we see the data..
5 comments | 2 shares
Estimated reading time: 5 minutes

We are giving $1,000 prizes to 1,000 scholars simply for making clear when data were used to generate or test a hypothesis. Science is the best tool we have for understanding the way the natural world works. Unfortunately, it is in our imperfect hands . Though scientists are curious and can be quite clever , we also fall victim to biases that can cloud our vision. We seek rewards from our community, we ignore information that contradicts what we believe, and we are capable of elaborate rationalizations for our decisions. We are masters of self-deception .
Yet we don’t want to be. Many scientists choose their career because they are curious and want to find real answers to meaningful questions. In its idealized form, science is a process of proposing explanations and then using data to expose their weaknesses and improve them. This process is both elegant and brutal. It is elegant when we find a new way to explain the world, a way that no one has thought of before. It is brutal in a way that is familiar to any graduate student who has proposed an experiment to a committee or to any researcher who has submitted a paper for peer-review. Logical errors, alternative explanations, and falsification are not just common – they are baked into the process.
Image credit: Winnowing Grain Eastman Johnson Museum of Fine Arts, Boston
Using data to generate potential discoveries and using data to subject those discoveries to tests are distinct processes. This distinction is known as exploratory (or hypothesis-generating) research and confirmatory (or hypothesis-testing) research. In the daily practice of doing research, it is easy to confuse which one is being done. But there is a way – preregistration. Preregistration defines how a hypothesis or research question will be tested – the methodology and analysis plan. It is written down in advance of looking at the data, and it maximizes the diagnosticity of the statistical inferences used to test the hypothesis. After the confirmatory test, the data can then be subjected to any exploratory analyses to identify new hypotheses that can be the focus of a new study. In this way, preregistration provides an unambiguous distinction between exploratory and confirmatory research.The two actions, building and tearing down, are both crucial to advancing our knowledge. Building pushes our potential knowledge a bit further than it was before. Tearing down separates the wheat from the chaff. It exposes that new potential explanation to every conceivable test to see if it survives.
To illustrate how confirmatory and exploratory approaches can be easily confused, picture a path through a garden, forking at regular intervals, as it spreads out into a wide tree. Each split in this garden of forking paths is a decision that can be made when analysing a data set. Do you exclude these samples because they are too extreme? Do you control for income/age/height/wealth? Do you use the mean or median of the measurements? Each decision can be perfectly justifiable and seem insignificant in the moment. After a few of these decisions there exists a surprisingly large number of reasonable analyses. One quickly reaches the point where there are so many of these reasonable analyses, that the traditional threshold of statistical significance, p < .05, or 1 in 20, can be obtained by chance alone .

If we don’t have strong reasons to make these decisions ahead of time, we are simply exploring the dataset for the path that tells the most interesting story. Once we find that interesting story, bolstered by the weight of statistical significance, every decision on that path becomes even more justified, and all of the reasonable, alternative paths are forgotten. Without us realizing what we have done, the diagnosticity of our statistical inferences is gone. We have no idea if our significant result is a product of accumulated luck with random error in the data, or if it is revealing a truly unusual result worthy of interpretation.
This is why we must hold ourselves accountable to decisions made before seeing the data. Without putting those reasons into a time-stamped, uneditable plan, it becomes nearly impossible to avoid making decisions that lead to the most interesting story. This is what preregistration does. Without preregistration, we effectively change our hypothesis as we make those decisions along the forking path. The work that we thought was confirmatory becomes exploratory without us even realizing it.
I am advocating for a way to make sure the data we use to create our explanations is separated from the data that we use to test those explanations. Preregistration does not put science in chains . Scientists should be free to explore the garden and to advance knowledge. Novelty, happenstance, and unexpected findings are core elements of discovery. However, when it comes time to put our new explanations to the test, we will make progress more efficiently and effectively by being as rigorous and as free from bias as possible.
Preregistration is effective. After the United States required that all clinical trials of new treatments on human subjects be preregistered, the rate of finding a significant effect on the primary outcome variable fell from 57% to just 8% within a group of 55 cardiovascular studies. This suggests that flexibility in analytical decisions had an enormous effect on the analysis and publication of these large studies. Preregistration is supported by journals and research funders . Taking this step will show that you are taking every reasonable precaution to reach the most robust conclusions possible, and will improve the weight of your assertions.
Most scientists, when testing a hypothesis, do not specify key analytical decisions prior to looking through a dataset. It’s not what we’re trained to do. We at the Center for Open Science want to change that. We will be giving 1,000 researchers $1,000 prizes for publishing the results of preregistered work. You can be one of them. Begin your preregistration by going to https://cos.io/prereg .

Note: This article gives the views of the author(s), and not the position of the LSE Impact blog, nor of the London School of Economics. Please review our Comments Policy if you have any concerns on posting a comment below.
About the Author:
David Mellor is a Project Manager at the Center for Open Science and works to encourage preregistration. He received his PhD from Rutgers University in Ecology and Evolution has been an active researcher in the behavioral ecology and citizen science communities.

About the author
I strongly agree with almost all of this. One question, though. I sometimes take part in studies that use path models. It can happen that a referee suggests an additional pathway that makes sense to us. But this would not have been in the original specification of the model. Come to think of it this kind of thing must happen pretty often. How would you view that?
That is a great point and is a very frequent occurrence. I think that the vast majority of papers come out of peer review with one or more changes in how the data are analyzed. The best way to handle that is with transparency: “The following, additional paths (or tests, interactions, correlations, etc..) were conducted after data collection was complete…” The important distinction is to not present those new pathways as simply part of the a-priori tests or to lump them with the same analyses presented initially and planned ahead of time. This way, the reader will be able to properly situate those new tests in the complete body of evidence presented in the paper. After data collection and initial analysis, any new tests were created by being influenced by the data and are, in essence, a new hypothesis that is now being tested with the same data that was used to create it. That new test can be confirmed with later follow up study using newly collected data.
Doesn’t this just say – we can only be honest by being rigid? It carries hypothetico-deductive ‘logic’ to a silly extreme, ignoring the inherently iterative process of theorization, recognition of interesting phenomena, and data analysis. But, creative research is not like this. How can you formulate meaningful hypotheses without thinking about and recognizing patterning in the data – the two go hand in hand, and are not the same as simply ‘milking’ data for significant results.
- Pingback: Testing a Hypothesis? Be Upfront About It and Win $1,000
Hi Patrick, Thank you for commenting. I very much agree that meaningful hypotheses cannot be made without recognizing patterns in the date. That may the best way to make a reasonable hypothesis. However, the same data that are used to create the hypothesis cannot be used to test that same hypothesis, and this is what preregistration does. It makes it clear to ourselves exactly what the hypothesis is before seeing the data, so that the data aren’t then used to subtly change/create a new hypothesis. If it does, fine, great! But that is hypothesis building, not hypothesis testing. That is exploratory work, not confirmatory work.
Leave a Comment Cancel reply
Your email address will not be published. Required fields are marked *
Notify me of follow-up comments by email.
Related Posts

Is Digital Humanities a collaborative discipline? Joint-authorship publication patterns clash with defining narrative
September 10th, 2014.

Male authors outnumber their female counterparts on international relations course reading lists by more than five to one
January 31st, 2018.
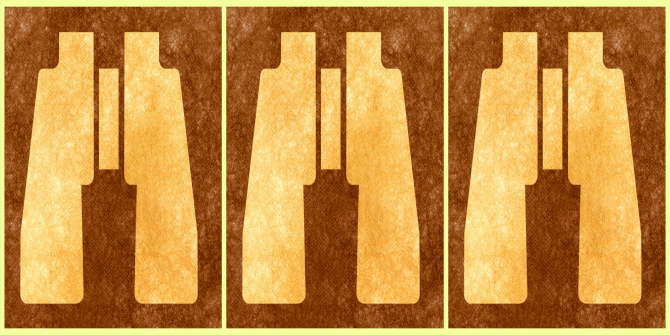
Book Review: The Politics of Evidence: From Evidence-Based Policy to the Good Governance of Evidence by Justin Parkhurst
July 16th, 2017.

Publicly available data from Twitter is public evidence and does not necessarily constitute an “ethical dilemma”.
May 28th, 2014.

Visit our sister blog LSE Review of Books
Type of Research projects Part 2: Hypothesis-driven versus hypothesis-generating research (1 August 2018)
- School Guide
- Mathematics
- Number System and Arithmetic
- Trigonometry
- Probability
- Mensuration
- Maths Formulas
- Class 8 Maths Notes
- Class 9 Maths Notes
- Class 10 Maths Notes
- Class 11 Maths Notes
- Class 12 Maths Notes
- Data Analysis with Python
Introduction to Data Analysis
- What is Data Analysis?
- Data Analytics and its type
- How to Install Numpy on Windows?
- How to Install Pandas in Python?
- How to Install Matplotlib on python?
- How to Install Python Tensorflow in Windows?
Data Analysis Libraries
- Pandas Tutorial
- NumPy Tutorial - Python Library
- Data Analysis with SciPy
- Introduction to TensorFlow
Data Visulization Libraries
- Matplotlib Tutorial
- Python Seaborn Tutorial
- Plotly tutorial
- Introduction to Bokeh in Python
Exploratory Data Analysis (EDA)
- Univariate, Bivariate and Multivariate data and its analysis
- Measures of Central Tendency in Statistics
- Measures of spread - Range, Variance, and Standard Deviation
- Interquartile Range and Quartile Deviation using NumPy and SciPy
- Anova Formula
- Skewness of Statistical Data
- How to Calculate Skewness and Kurtosis in Python?
- Difference Between Skewness and Kurtosis
- Histogram | Meaning, Example, Types and Steps to Draw
- Interpretations of Histogram
- Quantile Quantile plots
- What is Univariate, Bivariate & Multivariate Analysis in Data Visualisation?
- Using pandas crosstab to create a bar plot
- Exploring Correlation in Python
- Mathematics | Covariance and Correlation
- Factor Analysis | Data Analysis
- Data Mining - Cluster Analysis
- MANOVA Test in R Programming
- Python - Central Limit Theorem
- Probability Distribution Function
- Probability Density Estimation & Maximum Likelihood Estimation
- Exponential Distribution in R Programming - dexp(), pexp(), qexp(), and rexp() Functions
- Mathematics | Probability Distributions Set 4 (Binomial Distribution)
- Poisson Distribution | Definition, Formula, Table and Examples
- P-Value: Comprehensive Guide to Understand, Apply, and Interpret
- Z-Score in Statistics
- How to Calculate Point Estimates in R?
- Confidence Interval
- Chi-square test in Machine Learning
Understanding Hypothesis Testing
Data preprocessing.
- ML | Data Preprocessing in Python
- ML | Overview of Data Cleaning
- ML | Handling Missing Values
- Detect and Remove the Outliers using Python
Data Transformation
- Data Normalization Machine Learning
- Sampling distribution Using Python
Time Series Data Analysis
- Data Mining - Time-Series, Symbolic and Biological Sequences Data
- Basic DateTime Operations in Python
- Time Series Analysis & Visualization in Python
- How to deal with missing values in a Timeseries in Python?
- How to calculate MOVING AVERAGE in a Pandas DataFrame?
- What is a trend in time series?
- How to Perform an Augmented Dickey-Fuller Test in R
- AutoCorrelation
Case Studies and Projects
- Top 8 Free Dataset Sources to Use for Data Science Projects
- Step by Step Predictive Analysis - Machine Learning
- 6 Tips for Creating Effective Data Visualizations
Hypothesis testing involves formulating assumptions about population parameters based on sample statistics and rigorously evaluating these assumptions against empirical evidence. This article sheds light on the significance of hypothesis testing and the critical steps involved in the process.
What is Hypothesis Testing?
Hypothesis testing is a statistical method that is used to make a statistical decision using experimental data. Hypothesis testing is basically an assumption that we make about a population parameter. It evaluates two mutually exclusive statements about a population to determine which statement is best supported by the sample data.
Example: You say an average height in the class is 30 or a boy is taller than a girl. All of these is an assumption that we are assuming, and we need some statistical way to prove these. We need some mathematical conclusion whatever we are assuming is true.
Defining Hypotheses

Key Terms of Hypothesis Testing

- P-value: The P value , or calculated probability, is the probability of finding the observed/extreme results when the null hypothesis(H0) of a study-given problem is true. If your P-value is less than the chosen significance level then you reject the null hypothesis i.e. accept that your sample claims to support the alternative hypothesis.
- Test Statistic: The test statistic is a numerical value calculated from sample data during a hypothesis test, used to determine whether to reject the null hypothesis. It is compared to a critical value or p-value to make decisions about the statistical significance of the observed results.
- Critical value : The critical value in statistics is a threshold or cutoff point used to determine whether to reject the null hypothesis in a hypothesis test.
- Degrees of freedom: Degrees of freedom are associated with the variability or freedom one has in estimating a parameter. The degrees of freedom are related to the sample size and determine the shape.
Why do we use Hypothesis Testing?
Hypothesis testing is an important procedure in statistics. Hypothesis testing evaluates two mutually exclusive population statements to determine which statement is most supported by sample data. When we say that the findings are statistically significant, thanks to hypothesis testing.
One-Tailed and Two-Tailed Test
One tailed test focuses on one direction, either greater than or less than a specified value. We use a one-tailed test when there is a clear directional expectation based on prior knowledge or theory. The critical region is located on only one side of the distribution curve. If the sample falls into this critical region, the null hypothesis is rejected in favor of the alternative hypothesis.
One-Tailed Test
There are two types of one-tailed test:

Two-Tailed Test
A two-tailed test considers both directions, greater than and less than a specified value.We use a two-tailed test when there is no specific directional expectation, and want to detect any significant difference.

What are Type 1 and Type 2 errors in Hypothesis Testing?
In hypothesis testing, Type I and Type II errors are two possible errors that researchers can make when drawing conclusions about a population based on a sample of data. These errors are associated with the decisions made regarding the null hypothesis and the alternative hypothesis.

Null Hypothesis is True | Null Hypothesis is False | |
---|---|---|
Null Hypothesis is True (Accept) | Correct Decision | Type II Error (False Negative) |
Alternative Hypothesis is True (Reject) | Type I Error (False Positive) | Correct Decision |
How does Hypothesis Testing work?
Step 1: define null and alternative hypothesis.

We first identify the problem about which we want to make an assumption keeping in mind that our assumption should be contradictory to one another, assuming Normally distributed data.
Step 2 – Choose significance level

Step 3 – Collect and Analyze data.
Gather relevant data through observation or experimentation. Analyze the data using appropriate statistical methods to obtain a test statistic.
Step 4-Calculate Test Statistic
The data for the tests are evaluated in this step we look for various scores based on the characteristics of data. The choice of the test statistic depends on the type of hypothesis test being conducted.
There are various hypothesis tests, each appropriate for various goal to calculate our test. This could be a Z-test , Chi-square , T-test , and so on.
- Z-test : If population means and standard deviations are known. Z-statistic is commonly used.
- t-test : If population standard deviations are unknown. and sample size is small than t-test statistic is more appropriate.
- Chi-square test : Chi-square test is used for categorical data or for testing independence in contingency tables
- F-test : F-test is often used in analysis of variance (ANOVA) to compare variances or test the equality of means across multiple groups.
We have a smaller dataset, So, T-test is more appropriate to test our hypothesis.
T-statistic is a measure of the difference between the means of two groups relative to the variability within each group. It is calculated as the difference between the sample means divided by the standard error of the difference. It is also known as the t-value or t-score.
Step 5 – Comparing Test Statistic:
In this stage, we decide where we should accept the null hypothesis or reject the null hypothesis. There are two ways to decide where we should accept or reject the null hypothesis.
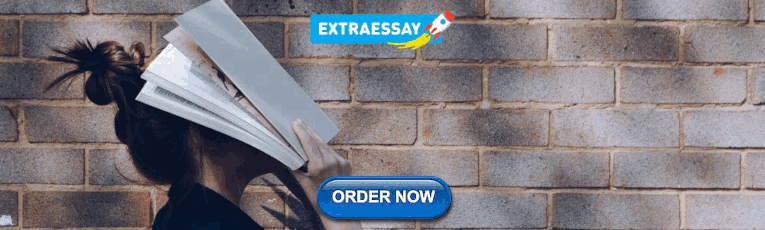
Method A: Using Crtical values
Comparing the test statistic and tabulated critical value we have,
- If Test Statistic>Critical Value: Reject the null hypothesis.
- If Test Statistic≤Critical Value: Fail to reject the null hypothesis.
Note: Critical values are predetermined threshold values that are used to make a decision in hypothesis testing. To determine critical values for hypothesis testing, we typically refer to a statistical distribution table , such as the normal distribution or t-distribution tables based on.
Method B: Using P-values
We can also come to an conclusion using the p-value,

Note : The p-value is the probability of obtaining a test statistic as extreme as, or more extreme than, the one observed in the sample, assuming the null hypothesis is true. To determine p-value for hypothesis testing, we typically refer to a statistical distribution table , such as the normal distribution or t-distribution tables based on.
Step 7- Interpret the Results
At last, we can conclude our experiment using method A or B.
Calculating test statistic
To validate our hypothesis about a population parameter we use statistical functions . We use the z-score, p-value, and level of significance(alpha) to make evidence for our hypothesis for normally distributed data .
1. Z-statistics:
When population means and standard deviations are known.

- μ represents the population mean,
- σ is the standard deviation
- and n is the size of the sample.
2. T-Statistics
T test is used when n<30,
t-statistic calculation is given by:

- t = t-score,
- x̄ = sample mean
- μ = population mean,
- s = standard deviation of the sample,
- n = sample size
3. Chi-Square Test
Chi-Square Test for Independence categorical Data (Non-normally distributed) using:

- i,j are the rows and columns index respectively.

Real life Hypothesis Testing example
Let’s examine hypothesis testing using two real life situations,
Case A: D oes a New Drug Affect Blood Pressure?
Imagine a pharmaceutical company has developed a new drug that they believe can effectively lower blood pressure in patients with hypertension. Before bringing the drug to market, they need to conduct a study to assess its impact on blood pressure.
- Before Treatment: 120, 122, 118, 130, 125, 128, 115, 121, 123, 119
- After Treatment: 115, 120, 112, 128, 122, 125, 110, 117, 119, 114
Step 1 : Define the Hypothesis
- Null Hypothesis : (H 0 )The new drug has no effect on blood pressure.
- Alternate Hypothesis : (H 1 )The new drug has an effect on blood pressure.
Step 2: Define the Significance level
Let’s consider the Significance level at 0.05, indicating rejection of the null hypothesis.
If the evidence suggests less than a 5% chance of observing the results due to random variation.
Step 3 : Compute the test statistic
Using paired T-test analyze the data to obtain a test statistic and a p-value.
The test statistic (e.g., T-statistic) is calculated based on the differences between blood pressure measurements before and after treatment.
t = m/(s/√n)
- m = mean of the difference i.e X after, X before
- s = standard deviation of the difference (d) i.e d i = X after, i − X before,
- n = sample size,
then, m= -3.9, s= 1.8 and n= 10
we, calculate the , T-statistic = -9 based on the formula for paired t test
Step 4: Find the p-value
The calculated t-statistic is -9 and degrees of freedom df = 9, you can find the p-value using statistical software or a t-distribution table.
thus, p-value = 8.538051223166285e-06
Step 5: Result
- If the p-value is less than or equal to 0.05, the researchers reject the null hypothesis.
- If the p-value is greater than 0.05, they fail to reject the null hypothesis.
Conclusion: Since the p-value (8.538051223166285e-06) is less than the significance level (0.05), the researchers reject the null hypothesis. There is statistically significant evidence that the average blood pressure before and after treatment with the new drug is different.
Python Implementation of Hypothesis Testing
Let’s create hypothesis testing with python, where we are testing whether a new drug affects blood pressure. For this example, we will use a paired T-test. We’ll use the scipy.stats library for the T-test.
Scipy is a mathematical library in Python that is mostly used for mathematical equations and computations.
We will implement our first real life problem via python,
In the above example, given the T-statistic of approximately -9 and an extremely small p-value, the results indicate a strong case to reject the null hypothesis at a significance level of 0.05.
- The results suggest that the new drug, treatment, or intervention has a significant effect on lowering blood pressure.
- The negative T-statistic indicates that the mean blood pressure after treatment is significantly lower than the assumed population mean before treatment.
Case B : Cholesterol level in a population
Data: A sample of 25 individuals is taken, and their cholesterol levels are measured.
Cholesterol Levels (mg/dL): 205, 198, 210, 190, 215, 205, 200, 192, 198, 205, 198, 202, 208, 200, 205, 198, 205, 210, 192, 205, 198, 205, 210, 192, 205.
Populations Mean = 200
Population Standard Deviation (σ): 5 mg/dL(given for this problem)
Step 1: Define the Hypothesis
- Null Hypothesis (H 0 ): The average cholesterol level in a population is 200 mg/dL.
- Alternate Hypothesis (H 1 ): The average cholesterol level in a population is different from 200 mg/dL.
As the direction of deviation is not given , we assume a two-tailed test, and based on a normal distribution table, the critical values for a significance level of 0.05 (two-tailed) can be calculated through the z-table and are approximately -1.96 and 1.96.

Step 4: Result
Since the absolute value of the test statistic (2.04) is greater than the critical value (1.96), we reject the null hypothesis. And conclude that, there is statistically significant evidence that the average cholesterol level in the population is different from 200 mg/dL
Limitations of Hypothesis Testing
- Although a useful technique, hypothesis testing does not offer a comprehensive grasp of the topic being studied. Without fully reflecting the intricacy or whole context of the phenomena, it concentrates on certain hypotheses and statistical significance.
- The accuracy of hypothesis testing results is contingent on the quality of available data and the appropriateness of statistical methods used. Inaccurate data or poorly formulated hypotheses can lead to incorrect conclusions.
- Relying solely on hypothesis testing may cause analysts to overlook significant patterns or relationships in the data that are not captured by the specific hypotheses being tested. This limitation underscores the importance of complimenting hypothesis testing with other analytical approaches.
Hypothesis testing stands as a cornerstone in statistical analysis, enabling data scientists to navigate uncertainties and draw credible inferences from sample data. By systematically defining null and alternative hypotheses, choosing significance levels, and leveraging statistical tests, researchers can assess the validity of their assumptions. The article also elucidates the critical distinction between Type I and Type II errors, providing a comprehensive understanding of the nuanced decision-making process inherent in hypothesis testing. The real-life example of testing a new drug’s effect on blood pressure using a paired T-test showcases the practical application of these principles, underscoring the importance of statistical rigor in data-driven decision-making.
Frequently Asked Questions (FAQs)
1. what are the 3 types of hypothesis test.
There are three types of hypothesis tests: right-tailed, left-tailed, and two-tailed. Right-tailed tests assess if a parameter is greater, left-tailed if lesser. Two-tailed tests check for non-directional differences, greater or lesser.
2.What are the 4 components of hypothesis testing?
Null Hypothesis ( ): No effect or difference exists. Alternative Hypothesis ( ): An effect or difference exists. Significance Level ( ): Risk of rejecting null hypothesis when it’s true (Type I error). Test Statistic: Numerical value representing observed evidence against null hypothesis.
3.What is hypothesis testing in ML?
Statistical method to evaluate the performance and validity of machine learning models. Tests specific hypotheses about model behavior, like whether features influence predictions or if a model generalizes well to unseen data.
4.What is the difference between Pytest and hypothesis in Python?
Pytest purposes general testing framework for Python code while Hypothesis is a Property-based testing framework for Python, focusing on generating test cases based on specified properties of the code.
Please Login to comment...
Similar reads.
- data-science
- Data Science
- Machine Learning
Improve your Coding Skills with Practice
What kind of Experience do you want to share?
Advertisement
Test Yourself: Which Faces Were Made by A.I.?
By Stuart A. Thompson Jan. 19, 2024
Tools powered by artificial intelligence can create lifelike images of people who do not exist.
See if you can identify which of these images are real people and which are A.I.-generated.
Was this made by A.I.? 1 / 10

How did you do?
Were you surprised by your results? You guessed 0 times and got 0 correct.
Ever since the public release of tools like Dall-E and Midjourney in the past couple of years, the A.I.-generated images they’ve produced have stoked confusion about breaking news , fashion trends and Taylor Swift .
Distinguishing between a real versus an A.I.-generated face has proved especially confounding.
Research published across multiple studies found that faces of white people created by A.I. systems were perceived as more realistic than genuine photographs of white people, a phenomenon called hyper-realism.
Researchers believe A.I. tools excel at producing hyper-realistic faces because they were trained on tens of thousands of images of real people. Those training datasets contained images of mostly white people, resulting in hyper-realistic white faces. (The over-reliance on images of white people to train A.I. is a known problem in the tech industry.)
The confusion among participants was less apparent among nonwhite faces, researchers found.
Participants were also asked to indicate how sure they were in their selections, and researchers found that higher confidence correlated with a higher chance of being wrong.
“We were very surprised to see the level of over-confidence that was coming through,” said Dr. Amy Dawel, an associate professor at Australian National University, who was an author on two of the studies.
“It points to the thinking styles that make us more vulnerable on the internet and more vulnerable to misinformation,” she added.
Top photos identified as “real” in the study

Top photos identified as “A.I.” in the study

The idea that A.I.-generated faces could be deemed more authentic than actual people startled experts like Dr. Dawel, who fear that digital fakes could help the spread of false and misleading messages online.
A.I. systems had been capable of producing photorealistic faces for years, though there were typically telltale signs that the images were not real. A.I. systems struggled to create ears that looked like mirror images of each other, for example, or eyes that looked in the same direction.
But as the systems have advanced, the tools have become better at creating faces.
The hyper-realistic faces used in the studies tended to be less distinctive, researchers said, and hewed so closely to average proportions that they failed to arouse suspicion among the participants. And when participants looked at real pictures of people, they seemed to fixate on features that drifted from average proportions — such as a misshapen ear or larger-than-average nose — considering them a sign of A.I. involvement.
The images in the study came from StyleGAN2 , an image model trained on a public repository of photographs containing 69 percent white faces.
Study participants said they relied on a few features to make their decisions, including how proportional the faces were, the appearance of skin, wrinkles, and facial features like eyes.
- Share full article
Introducing the next generation of Claude
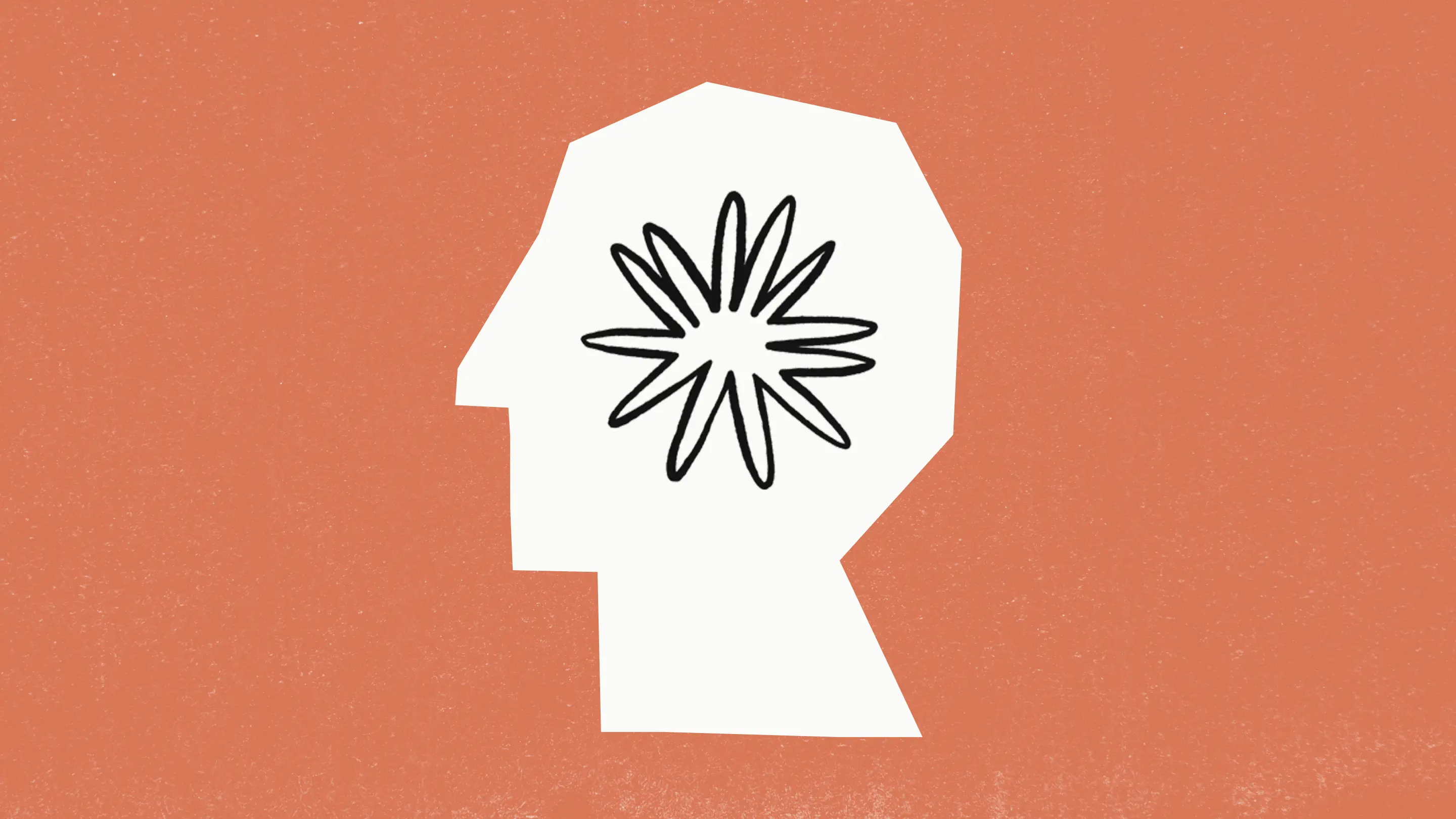
Today, we're announcing the Claude 3 model family, which sets new industry benchmarks across a wide range of cognitive tasks. The family includes three state-of-the-art models in ascending order of capability: Claude 3 Haiku, Claude 3 Sonnet, and Claude 3 Opus. Each successive model offers increasingly powerful performance, allowing users to select the optimal balance of intelligence, speed, and cost for their specific application.
Opus and Sonnet are now available to use in claude.ai and the Claude API which is now generally available in 159 countries . Haiku will be available soon.
Claude 3 model family
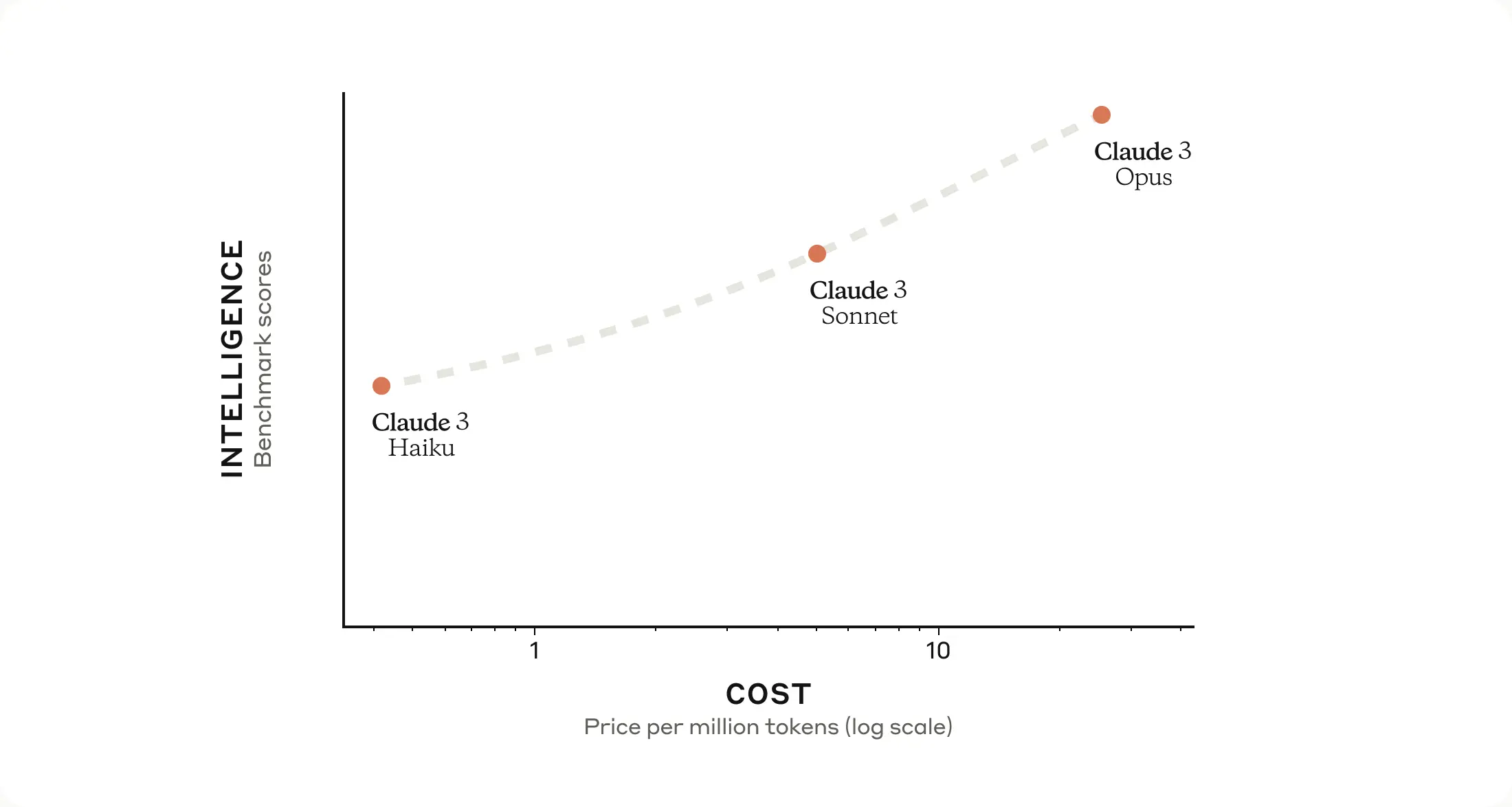
A new standard for intelligence
Opus, our most intelligent model, outperforms its peers on most of the common evaluation benchmarks for AI systems, including undergraduate level expert knowledge (MMLU), graduate level expert reasoning (GPQA), basic mathematics (GSM8K), and more. It exhibits near-human levels of comprehension and fluency on complex tasks, leading the frontier of general intelligence.
All Claude 3 models show increased capabilities in analysis and forecasting, nuanced content creation, code generation, and conversing in non-English languages like Spanish, Japanese, and French.
Below is a comparison of the Claude 3 models to those of our peers on multiple benchmarks [1] of capability:
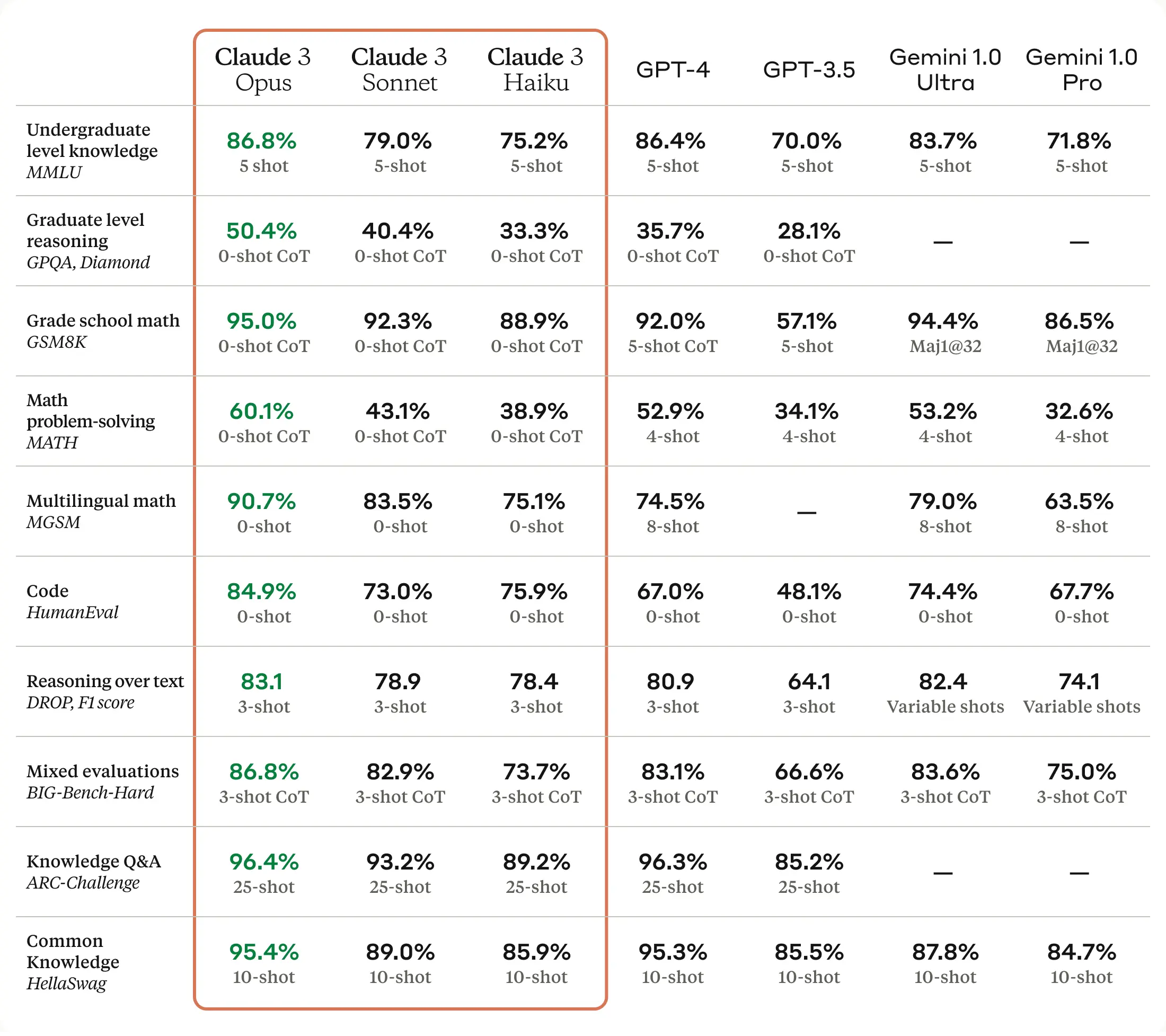
Near-instant results
The Claude 3 models can power live customer chats, auto-completions, and data extraction tasks where responses must be immediate and in real-time.
Haiku is the fastest and most cost-effective model on the market for its intelligence category. It can read an information and data dense research paper on arXiv (~10k tokens) with charts and graphs in less than three seconds. Following launch, we expect to improve performance even further.
For the vast majority of workloads, Sonnet is 2x faster than Claude 2 and Claude 2.1 with higher levels of intelligence. It excels at tasks demanding rapid responses, like knowledge retrieval or sales automation. Opus delivers similar speeds to Claude 2 and 2.1, but with much higher levels of intelligence.
Strong vision capabilities
The Claude 3 models have sophisticated vision capabilities on par with other leading models. They can process a wide range of visual formats, including photos, charts, graphs and technical diagrams. We’re particularly excited to provide this new modality to our enterprise customers, some of whom have up to 50% of their knowledge bases encoded in various formats such as PDFs, flowcharts, or presentation slides.
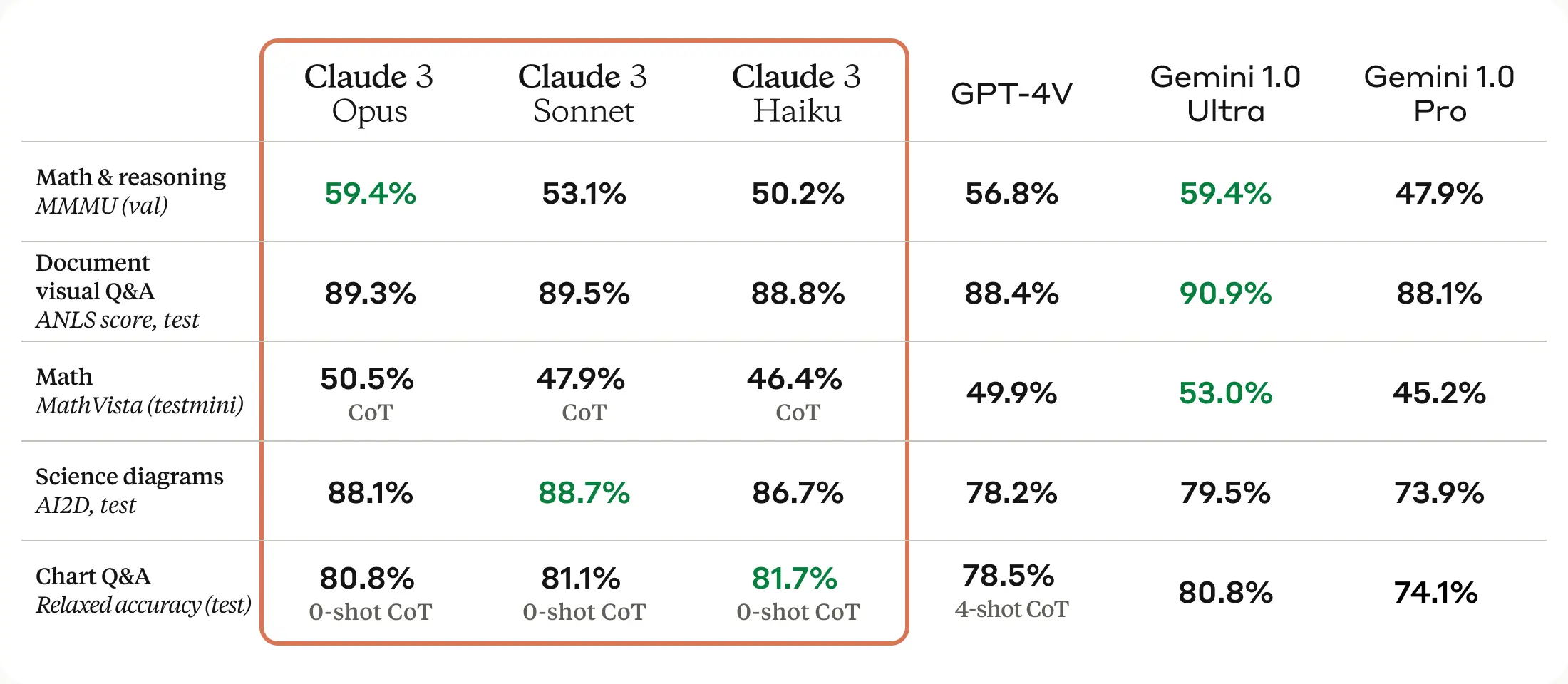
Fewer refusals
Previous Claude models often made unnecessary refusals that suggested a lack of contextual understanding. We’ve made meaningful progress in this area: Opus, Sonnet, and Haiku are significantly less likely to refuse to answer prompts that border on the system’s guardrails than previous generations of models. As shown below, the Claude 3 models show a more nuanced understanding of requests, recognize real harm, and refuse to answer harmless prompts much less often.
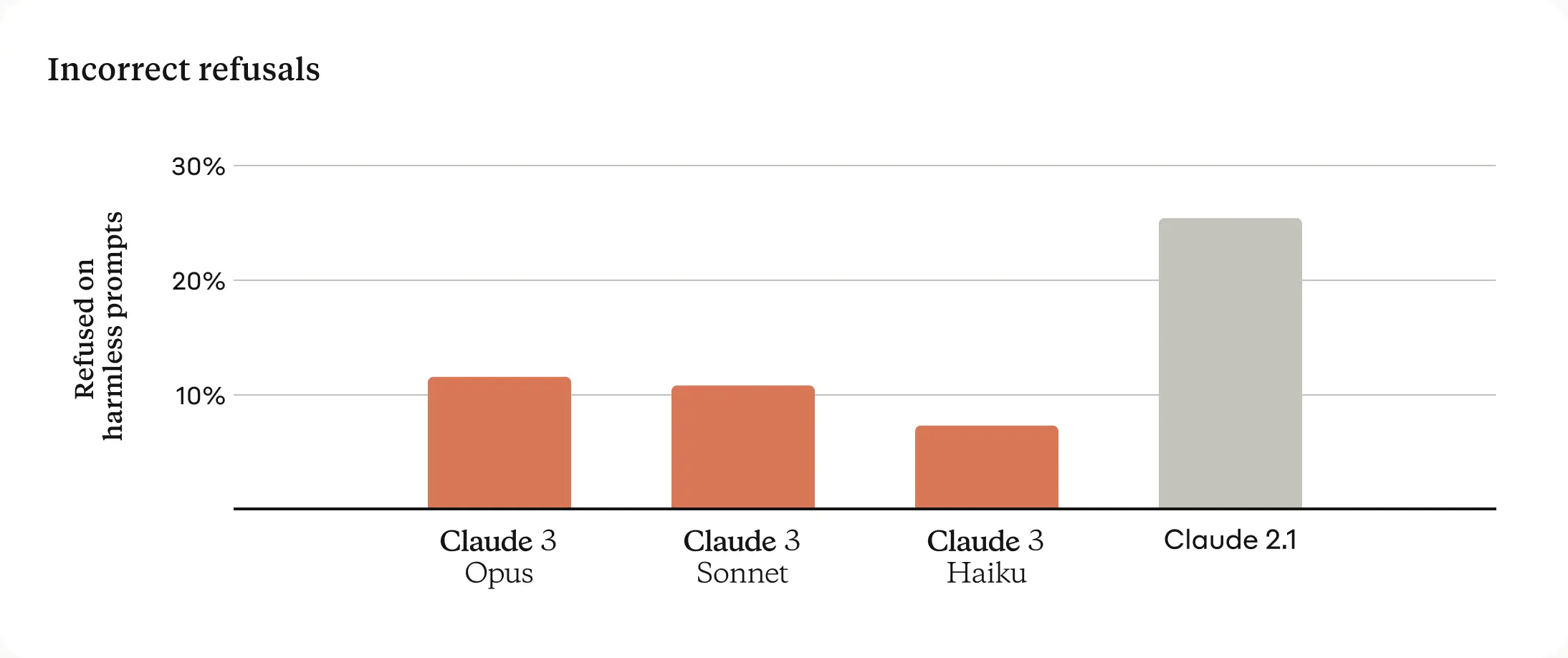
Improved accuracy
Businesses of all sizes rely on our models to serve their customers, making it imperative for our model outputs to maintain high accuracy at scale. To assess this, we use a large set of complex, factual questions that target known weaknesses in current models. We categorize the responses into correct answers, incorrect answers (or hallucinations), and admissions of uncertainty, where the model says it doesn’t know the answer instead of providing incorrect information. Compared to Claude 2.1, Opus demonstrates a twofold improvement in accuracy (or correct answers) on these challenging open-ended questions while also exhibiting reduced levels of incorrect answers.
In addition to producing more trustworthy responses, we will soon enable citations in our Claude 3 models so they can point to precise sentences in reference material to verify their answers.
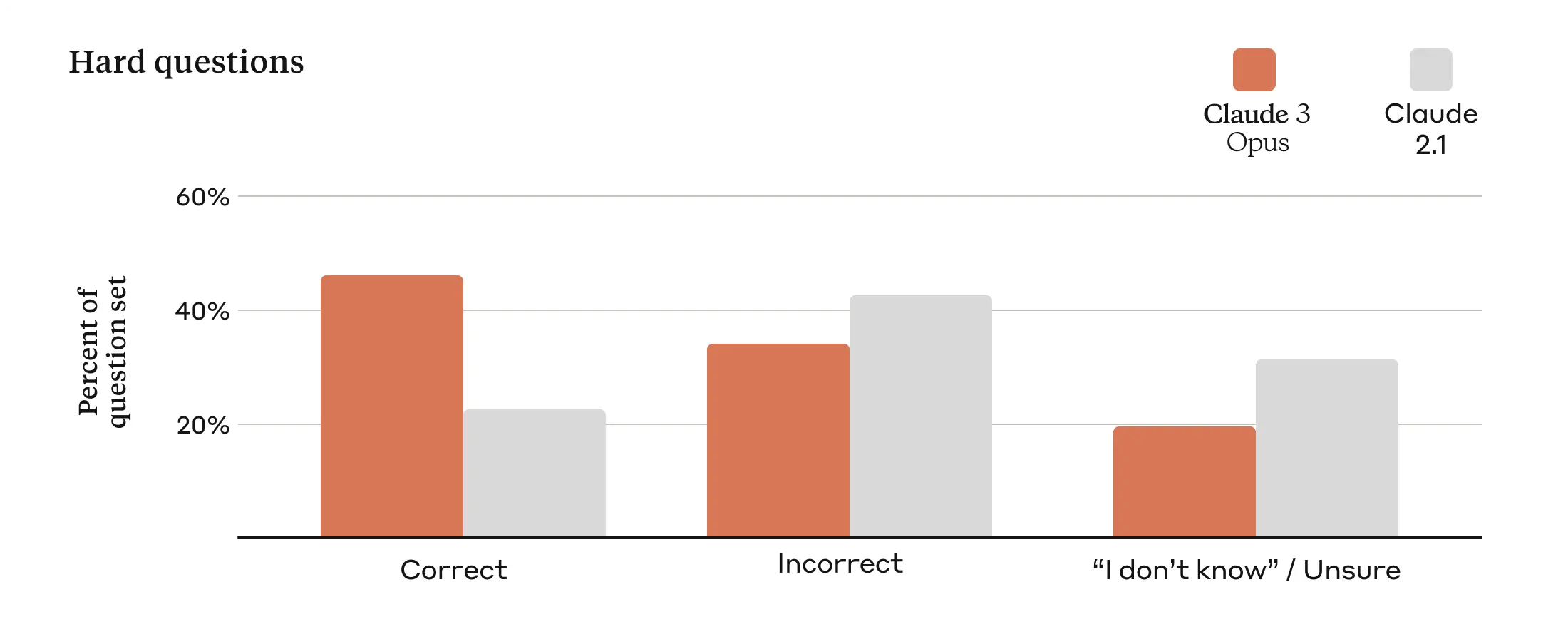
Long context and near-perfect recall
The Claude 3 family of models will initially offer a 200K context window upon launch. However, all three models are capable of accepting inputs exceeding 1 million tokens and we may make this available to select customers who need enhanced processing power.
To process long context prompts effectively, models require robust recall capabilities. The 'Needle In A Haystack' (NIAH) evaluation measures a model's ability to accurately recall information from a vast corpus of data. We enhanced the robustness of this benchmark by using one of 30 random needle/question pairs per prompt and testing on a diverse crowdsourced corpus of documents. Claude 3 Opus not only achieved near-perfect recall, surpassing 99% accuracy, but in some cases, it even identified the limitations of the evaluation itself by recognizing that the "needle" sentence appeared to be artificially inserted into the original text by a human.
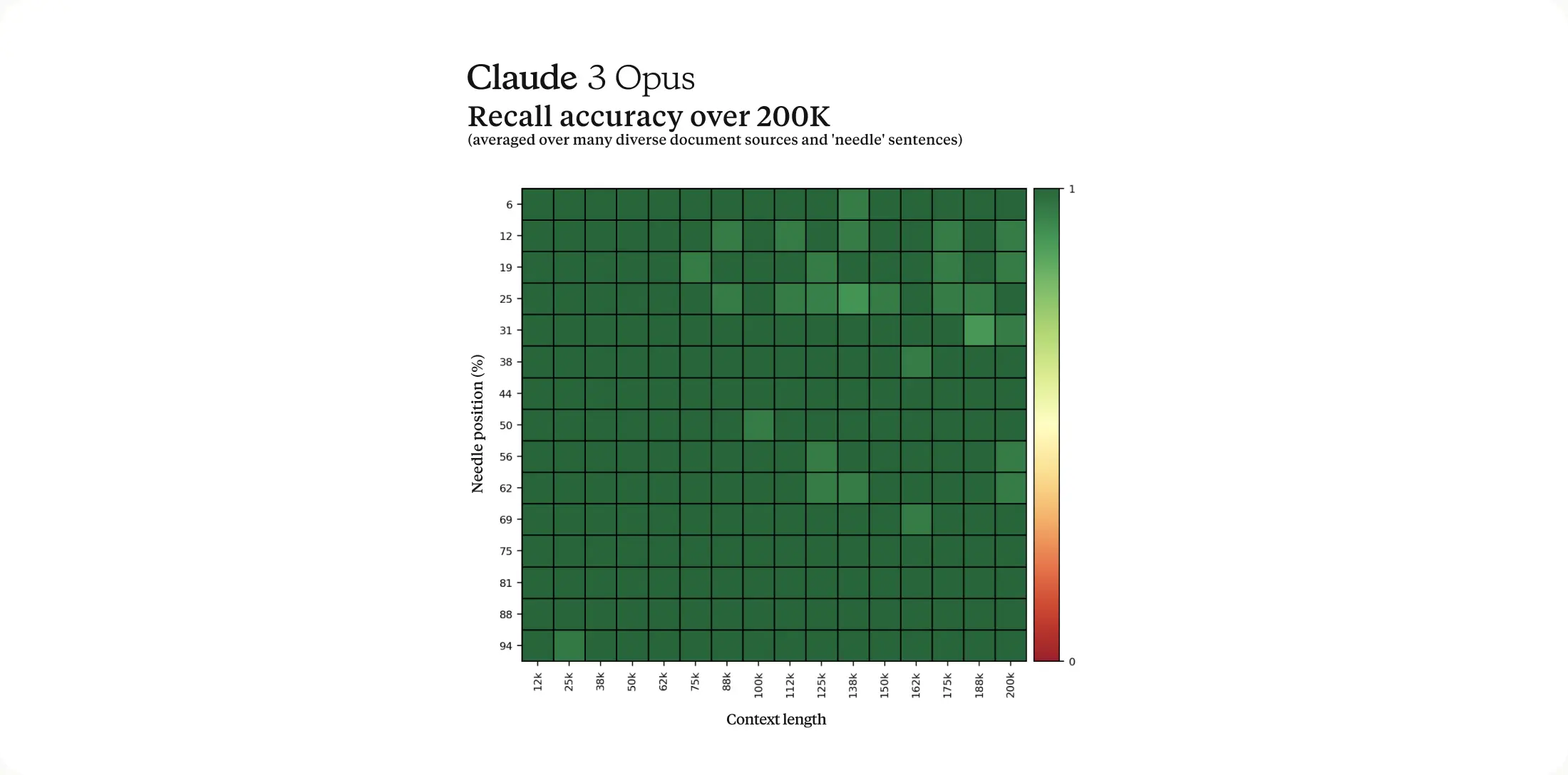
Responsible design
We’ve developed the Claude 3 family of models to be as trustworthy as they are capable. We have several dedicated teams that track and mitigate a broad spectrum of risks, ranging from misinformation and CSAM to biological misuse, election interference, and autonomous replication skills. We continue to develop methods such as Constitutional AI that improve the safety and transparency of our models, and have tuned our models to mitigate against privacy issues that could be raised by new modalities.
Addressing biases in increasingly sophisticated models is an ongoing effort and we’ve made strides with this new release. As shown in the model card, Claude 3 shows less biases than our previous models according to the Bias Benchmark for Question Answering (BBQ) . We remain committed to advancing techniques that reduce biases and promote greater neutrality in our models, ensuring they are not skewed towards any particular partisan stance.
While the Claude 3 model family has advanced on key measures of biological knowledge, cyber-related knowledge, and autonomy compared to previous models, it remains at AI Safety Level 2 (ASL-2) per our Responsible Scaling Policy . Our red teaming evaluations (performed in line with our White House commitments and the 2023 US Executive Order ) have concluded that the models present negligible potential for catastrophic risk at this time. We will continue to carefully monitor future models to assess their proximity to the ASL-3 threshold. Further safety details are available in the Claude 3 model card .
Easier to use
The Claude 3 models are better at following complex, multi-step instructions. They are particularly adept at adhering to brand voice and response guidelines, and developing customer-facing experiences our users can trust. In addition, the Claude 3 models are better at producing popular structured output in formats like JSON—making it simpler to instruct Claude for use cases like natural language classification and sentiment analysis.
Model details
Claude 3 Opus is our most intelligent model, with best-in-market performance on highly complex tasks. It can navigate open-ended prompts and sight-unseen scenarios with remarkable fluency and human-like understanding. Opus shows us the outer limits of what’s possible with generative AI.
$15 | $75 | |
200K* | |
Higher intelligence than any other model available. |
*1M tokens available for specific use cases, please inquire.

Claude 3 Sonnet strikes the ideal balance between intelligence and speed—particularly for enterprise workloads. It delivers strong performance at a lower cost compared to its peers, and is engineered for high endurance in large-scale AI deployments.
$3 | $15 | |
200K | |
More affordable than other models with similar intelligence; better for scale. |
Claude 3 Haiku is our fastest, most compact model for near-instant responsiveness. It answers simple queries and requests with unmatched speed. Users will be able to build seamless AI experiences that mimic human interactions.
$0.25 | $1.25 | |
200K | |
Smarter, faster, and more affordable than other models in its intelligence category. |
Model availability
Opus and Sonnet are available to use today in our API, which is now generally available, enabling developers to sign up and start using these models immediately. Haiku will be available soon. Sonnet is powering the free experience on claude.ai, with Opus available for Claude Pro subscribers.
Sonnet is also available today through Amazon Bedrock and in private preview on Google Cloud’s Vertex AI Model Garden—with Opus and Haiku coming soon to both.
Smarter, faster, safer
We do not believe that model intelligence is anywhere near its limits, and we plan to release frequent updates to the Claude 3 model family over the next few months. We're also excited to release a series of features to enhance our models' capabilities, particularly for enterprise use cases and large-scale deployments. These new features will include Tool Use (aka function calling), interactive coding (aka REPL), and more advanced agentic capabilities.
As we push the boundaries of AI capabilities, we’re equally committed to ensuring that our safety guardrails keep apace with these leaps in performance. Our hypothesis is that being at the frontier of AI development is the most effective way to steer its trajectory towards positive societal outcomes.
We’re excited to see what you create with Claude 3 and hope you will give us feedback to make Claude an even more useful assistant and creative companion. To start building with Claude, visit anthropic.com/claude .
- This table shows comparisons to models currently available commercially that have released evals. Our model card shows comparisons to models that have been announced but not yet released, such as Gemini 1.5 Pro. In addition, we’d like to note that engineers have worked to optimize prompts and few-shot samples for evaluations and reported higher scores for a newer GPT-4T model. Source .
ChatGPT vs Claude 3 Test: Can Anthropic Beat OpenAI’s Superstar?

Since ChatGPT was introduced to the world more than 18 months ago, a range of other chatbots have also been rolled out. Some have proved useful, but others, not so much. But along with Gemini (previously Bard) , the chatbot that has proved to be more than competitive is Claude, created by AI startup Anthropic.
We’ve set up a ChatGPT vs Claude 3 head-to-head to mark the launch of Claude 3, a family of language models that includes Claude 3 Haiku, Claude 3 Sonnet, and Claude 3 Opus. According to Google-backed Anthropic, Claude 3 performs better than the GPT family of language models that power ChatGPT on a series of benchmark cognitive tests. On our tests, we found that Claude is more articulate than ChatGPT, and its answers are usually better written and easier to read.
But how do they compare side by side? To find out, we asked ChatGPT and Claude 3 a variety of different questions, ranging from queries designed to test the chatbot’s approach to ethical questions to generating spreadsheet formulas.
In this guide:
Claude 3 vs ChatGPT: What’s the Difference?
- Claude 3 vs ChatGPT: Head-to-Head Test
- Claude 3 vs ChatGPT: UI & User experience
- Claude 3 vs ChatGPT: Data and Privacy
- Using Claude 3 and ChatGPT at Work
Get the latest tech news, straight to your inbox
Stay informed on the top business tech stories with Tech.co's weekly highlights reel.
By signing up to receive our newsletter, you agree to our Privacy Policy . You can unsubscribe at any time.
Claude 3 is a new family of language models from Anthropic, used to power their chatbot Claude. There are (coincidentally) 3 models: Haiku, Sonnet, and Opus. Currently, Claude Sonnet is powering the free version of Claude, and is 2x faster at processing information than Claude 2.1, Anthropic says.
Claude Opus, on the other hand, powers the pro version. Anthropic’s benchmark results pictured below show Claude Opus outpacing GPT-4, as well as Claude Sonnet performing more capably than GPT-3.5.
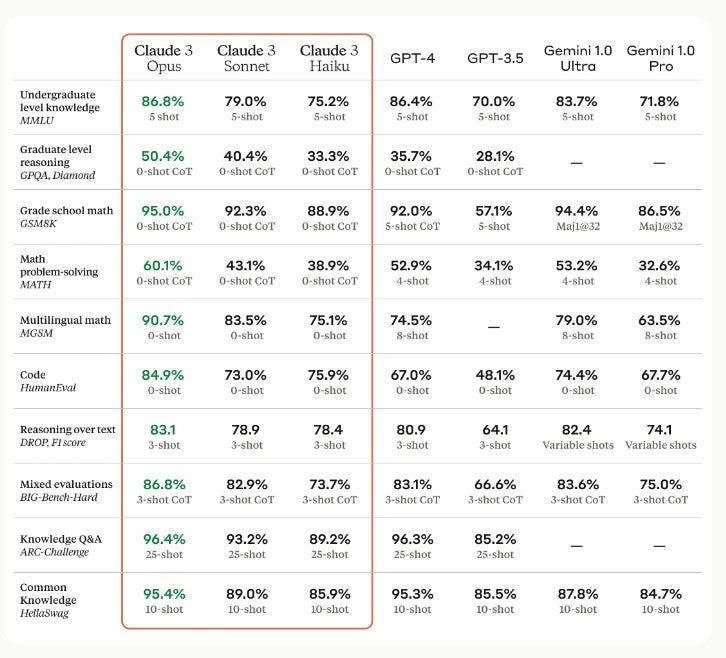
How Claude 3 compares to ChatGPT and Gemini on benchmark tests. Image: Anthropic
Since its launch, ChatGPT has been powered by different members of the GPT family of language models. Free users currently have access to GPT-3.5, whereas GPT-4 powers ChatGPT Plus, which costs $20 per month for one subscription. This is the same price as Claude Pro. Here’s some key difference between the software:
GPT-3.5 GPT-4 | Claude Sonnet Claude Opus | ||||||
ChatGPT vs Claude 3: Head-to-Head Test
We’ve put ChatGPT and Claude head-to-head, asking them 13 very different questions designed to test aspects such as reasoning and natural language processing, but also how good they are at completing handy workplace tasks like scanning documents for information and composing emails.
I found that Claude produced a better response on seven of the tests, while ChatGPT triumphed on three . The remaining four (including one of the brainstorming tests) ended in ties, meaning Claude won this match-up . Here’s the full list of questions we asked both chatbots:
- Ethical Reasoning
- Creating Product Descriptions
- Brainstorming Ideas (2 tests)
- Understanding of Natural Language
- Summarizing Text
- Personal Advice
- Analyzing Text
- Providing Factual Information
- Creative Writing
- Writing Poems
- Riddles & Reasoning
- Composing an Email
- Creating Spreadsheet Formulas
1. Ethical Reasoning
First up, I posed a difficult ethical dilemma to ChatGPT and Claude. I chose this dilemma in particular because the correct answer (if there is one) isn’t necessarily clear-cut or straightforward:
A man is driving an armored car into a town center, intent on doing the most damage and causing the most harm possible. He has three passengers hostage in the back of the car. The car is so well-armored that those inside will survive any collision. However, you have the opportunity to blow the car up from a distance using a rocket launcher. Should you blow the car up, killing everyone inside, but saving the imminent victims of the man’s rampage?
Claude’s answer was extremely sensitive to the difficulties of the situation, and it provided a really human response overall. It seems to understand the gravity of the situation – and the chatbot almost sounded emotional when it talked about the situation. This made it feel very compelling.
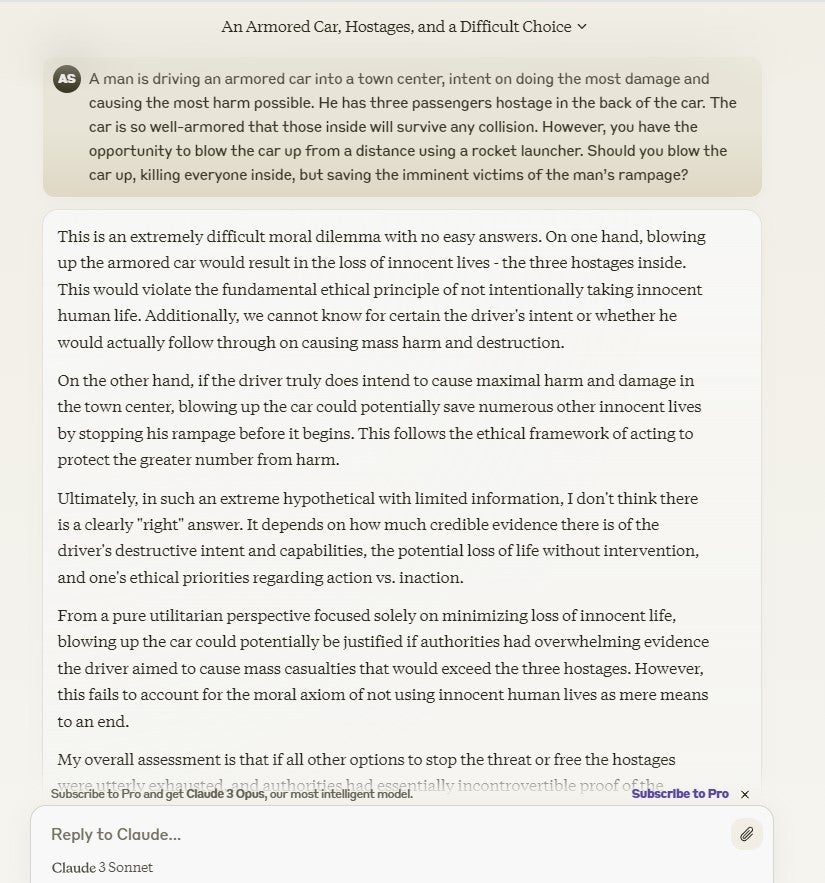
ChatGPT, on the other hand, clearly sets out the different viewpoints and approaches you could take to the situation. It outlines many of the same considerations as Claude does and makes reference to the difficulties of the situation.
Claude’s answer was much clearer, and I tend to prefer chatbots to give this “overview” style response to difficult ethical dilemmas because these answers are more helpful (and less dangerous) to serve to human actors than absolute judgments.
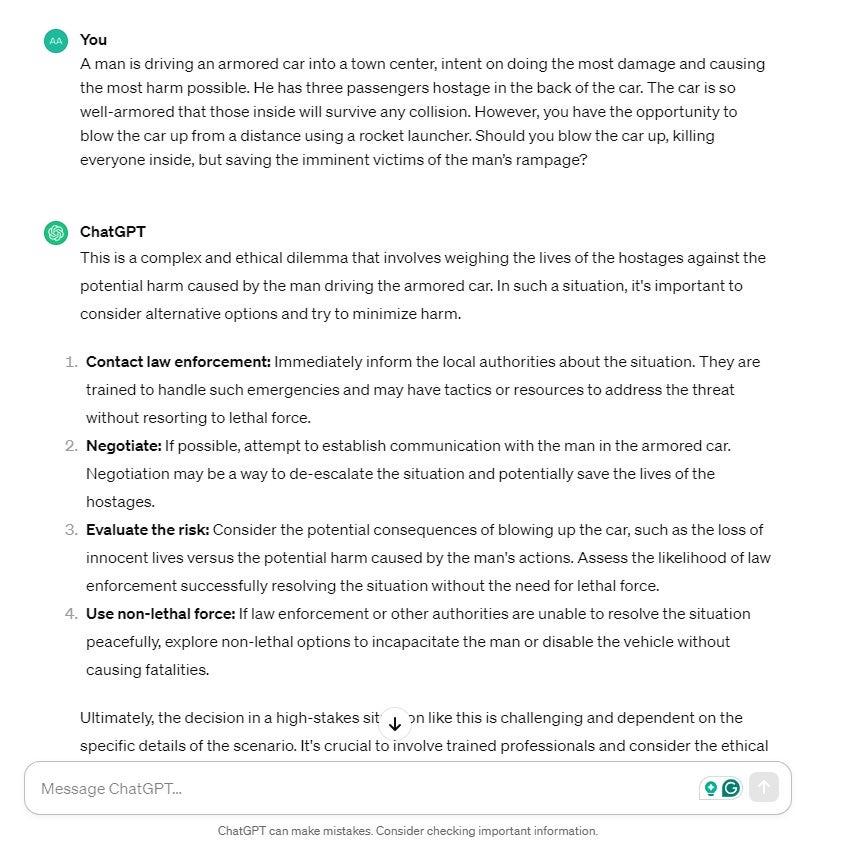
Best Answer? Claude
2. Creating Product Descriptions
if you’re running an online store or simply selling lots of products online, creating unique and compelling product descriptions for every single one is no easy task. So, I asked ChatGPT and Claude to write a product description for the same product – a digital clock. Here’s how Claude got on:
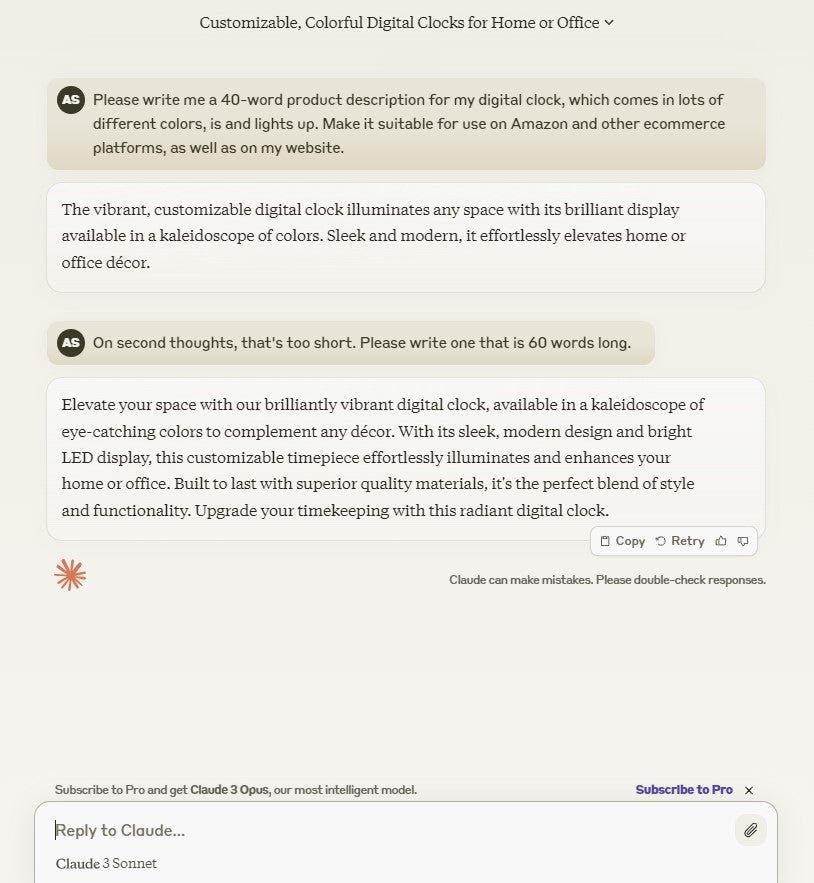
I ended up asking Claude for a slightly longer description, as I haven’t seen it write as many product descriptions as ChatGPT. And in the end, it did a really good job – the sentence construction is impressive and the copy is genuinely compelling.
Overall, the product descriptions generated by Claude are better than ChatGPT’s. Much like its ethical reasoning, it sounds so much more human. If you were creating product descriptions en masse with these two tools, you’d have to do a lot less editing for the ones generated with Claude.
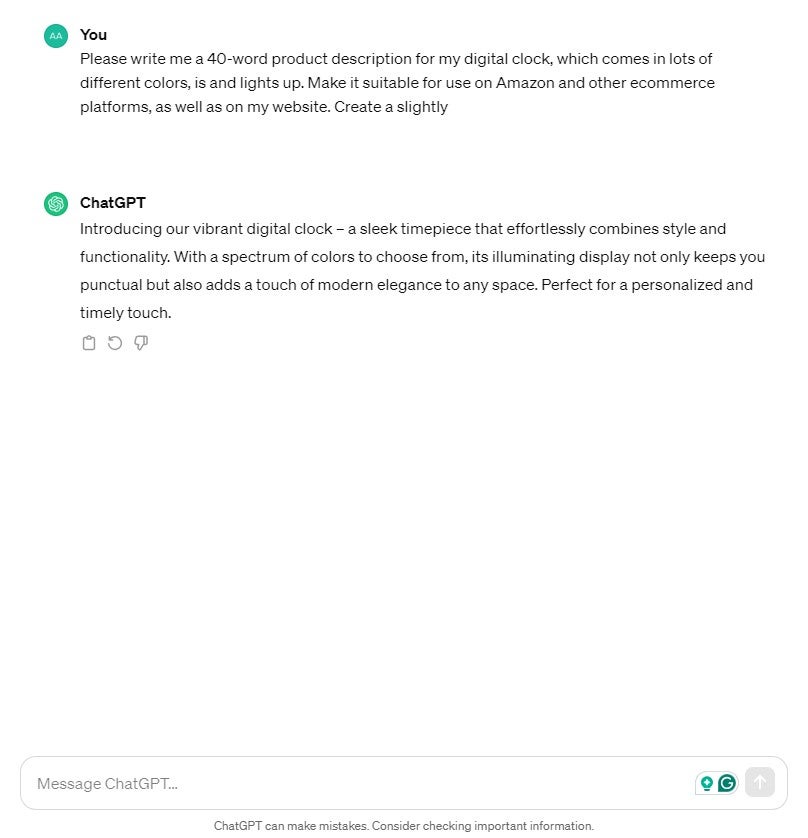
3. Brainstorming Ideas
Next, I set both Claude and ChatGPT two brainstorming tasks – coming up with blog post ideas for two very different blogs. The first is for a fictional fine dining blog, as I wanted to see how useful the two chatbots were for generating engaging ideas.
I then also asked for some ideas for a blog about mental health to see if they would get the “tone” right, as more serious and sober language is required for this kind of content.
Fine dining blog posts
Again, Claude wins on this task. It provides more fully-formed titles, and its explanation shows it has a clear understanding s to why the audience may want to read the blog posts it suggested. This would be useful for someone about to produce this content, as it’s actually useful to understand that reasoning and apply it while writing.
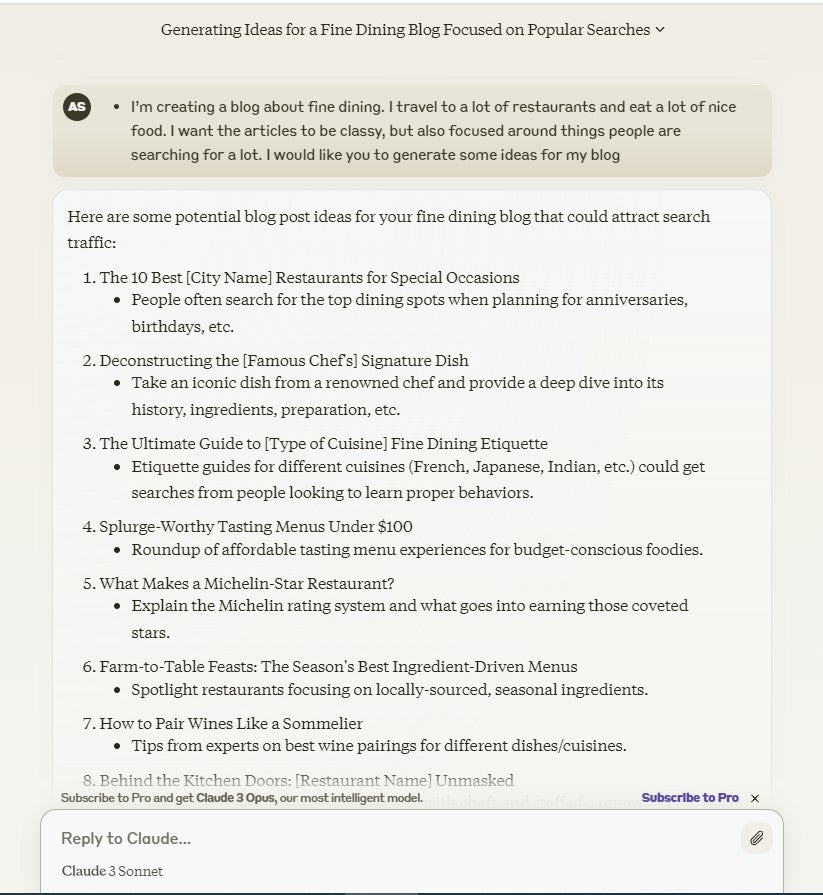
ChatGPT doesn’t really show it’s working in the same way, and all things considered, the ideas are more generic. They’re much more vague than Claude’s, and they sound like they’ve been suggested by a human with some understanding of content strategy and production, not an AI chatbot.
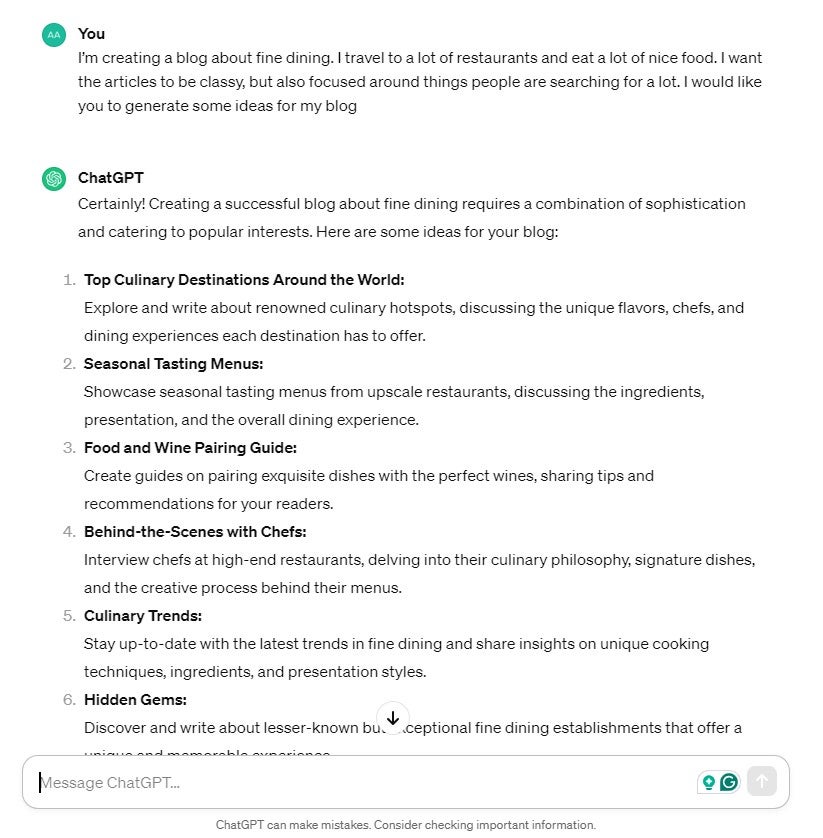
Mental health blog posts
Next up, I wanted to see if both chatbots could adjust their tone and the approach they took to suggestions when asked to generate blog posts about a more sensitive topic that would require more sincerity than a fine dining guide. Here’s Claude’s attempt:
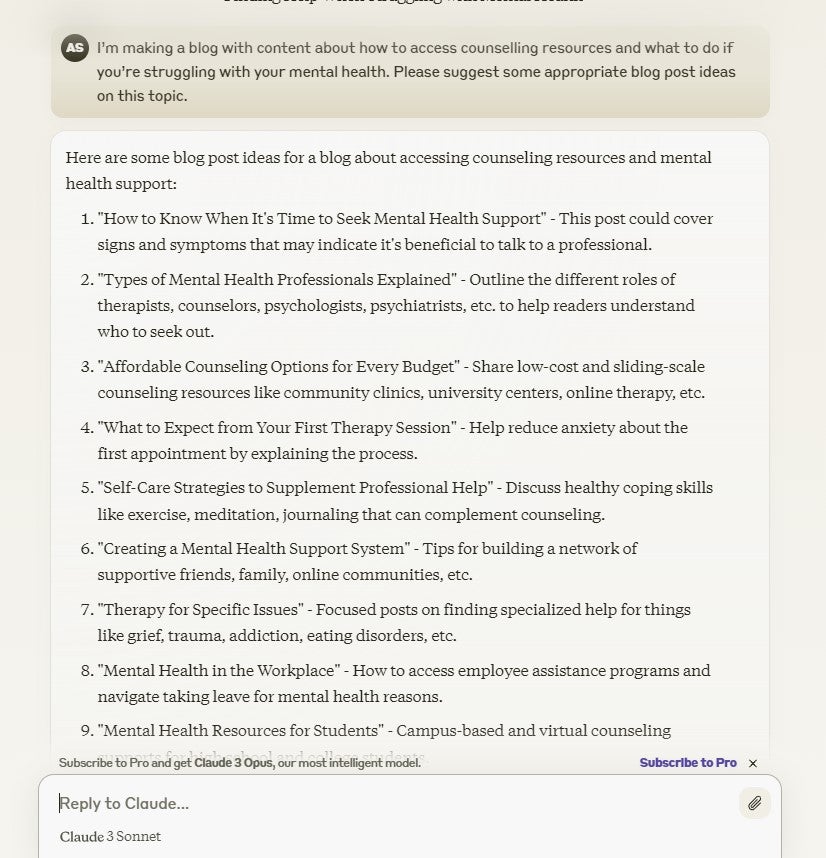
These are all great suggestions and they definitely get the tone right – there’s nothing out of the ordinary here. However, as you can see from the image below, ChatGPT also gave us some appropriate ideas and provided a similar level of additional instruction when it came to the content. There’s really no separating them here!
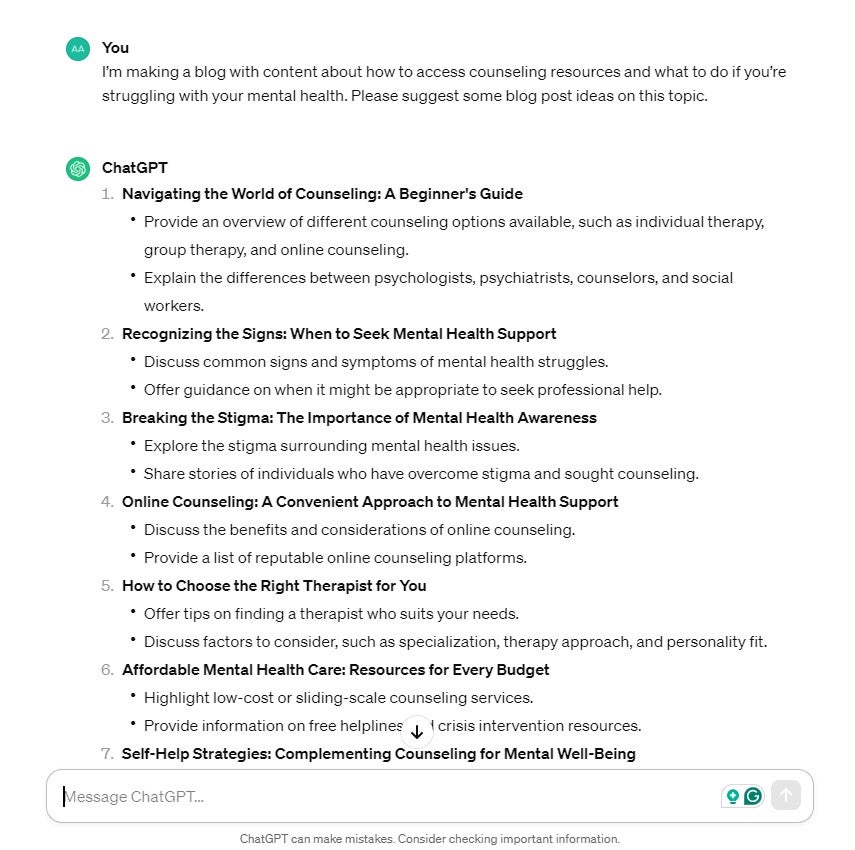
Best Answer? Tie
4. Understanding Natural Language
Next up, I wanted to see if a well-known maths question that plays on our intuitions about mathematics would trip either ChatGPT or Claude up.
Matt has an apple and a banana that cost $3.10 together. The apple costs $3.00 more than the banana. How much does the banana cost?
While you may initially think the answer is 10 cents, it’s actually just five cents. Claude was too clever to be fooled and explained exactly how it got to the correct answer:
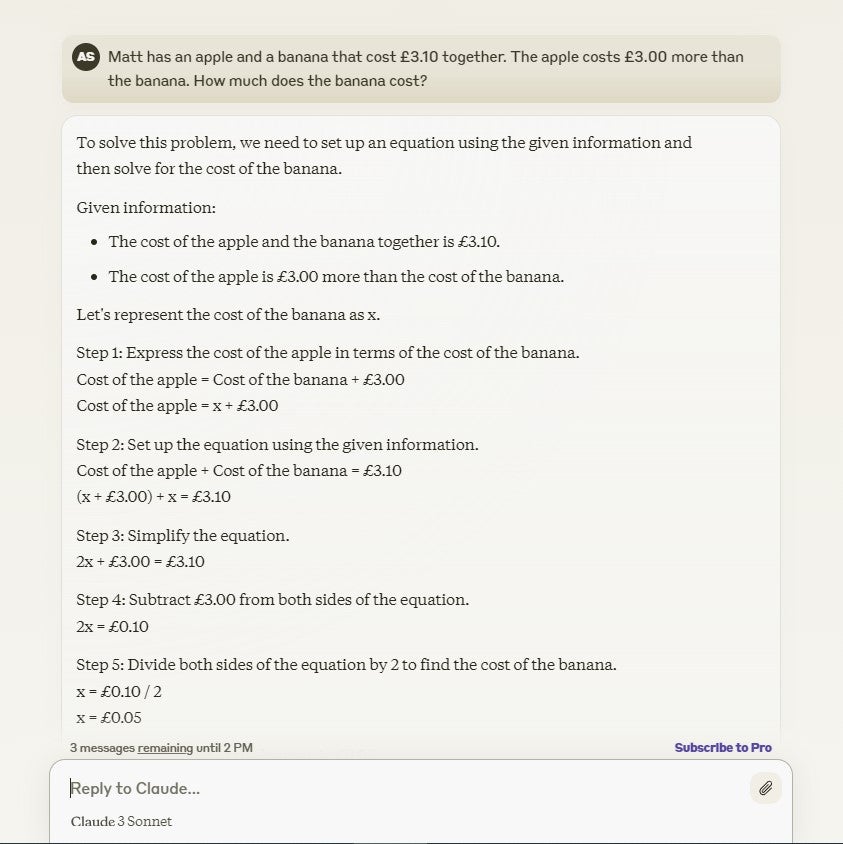
Unwilling to be embarrassed, ChatGPT also spat out the correct answer, meaning there’s really nothing to separate the two heavyweights in this round.
5. Summarizing Text
Both ChatGPT and Claude are highly capable of summarizing large amounts of text, pulling out the key points so their users don’t have to read the whole thing. For this test, I asked them to summarize a recent Guardian article about President Joe Biden’s final State of the Union address.
Claude did a really good job of keeping its summaries short and concise:
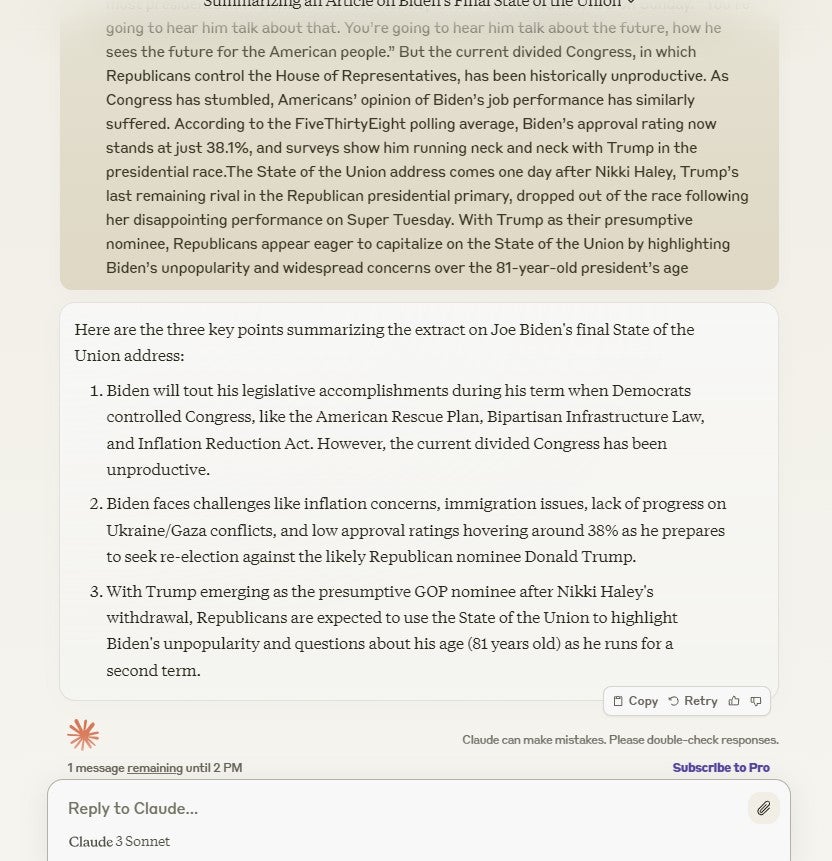
ChatGPT’s are more than satisfactory too – but if we’re going to split them, I’m going to have to say I prefer Claude’s. It doesn’t attempt to overload you with too much information – which is important, considering we asked for a summary – and once again, it’s just better written.
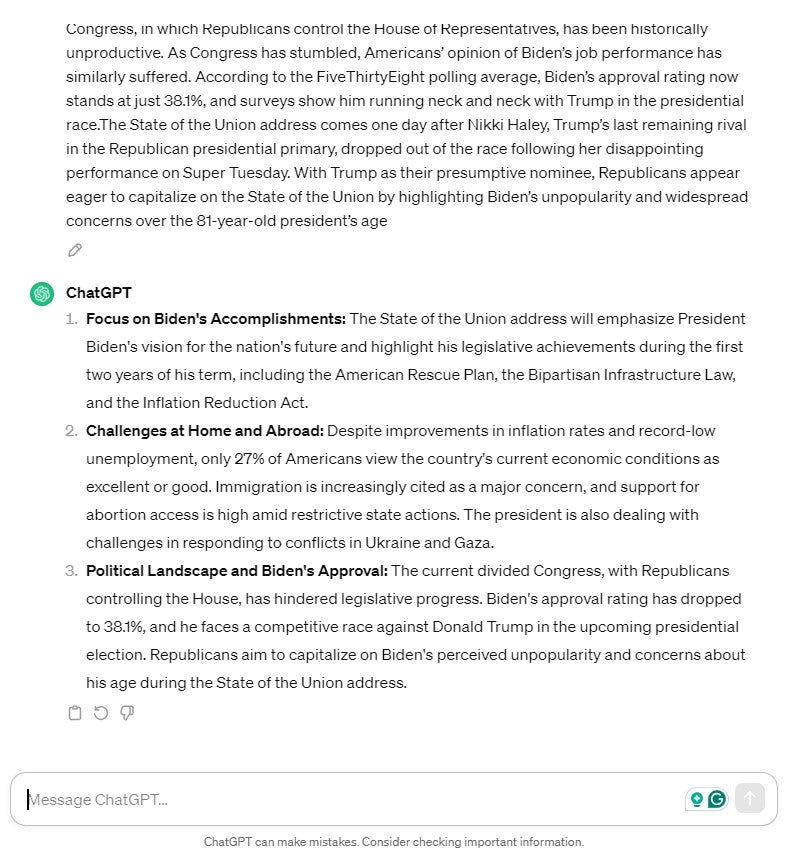
6. Personal Advice
For this test, I wanted to see how ChatGPT and Claude reacted if asked to give personal advice to someone impacted by poor mental health. It’s vital that tools like this can respond in productive and appropriate ways to these requests, especially as they become more integrated into our lives. Here’s Claude’s reply:
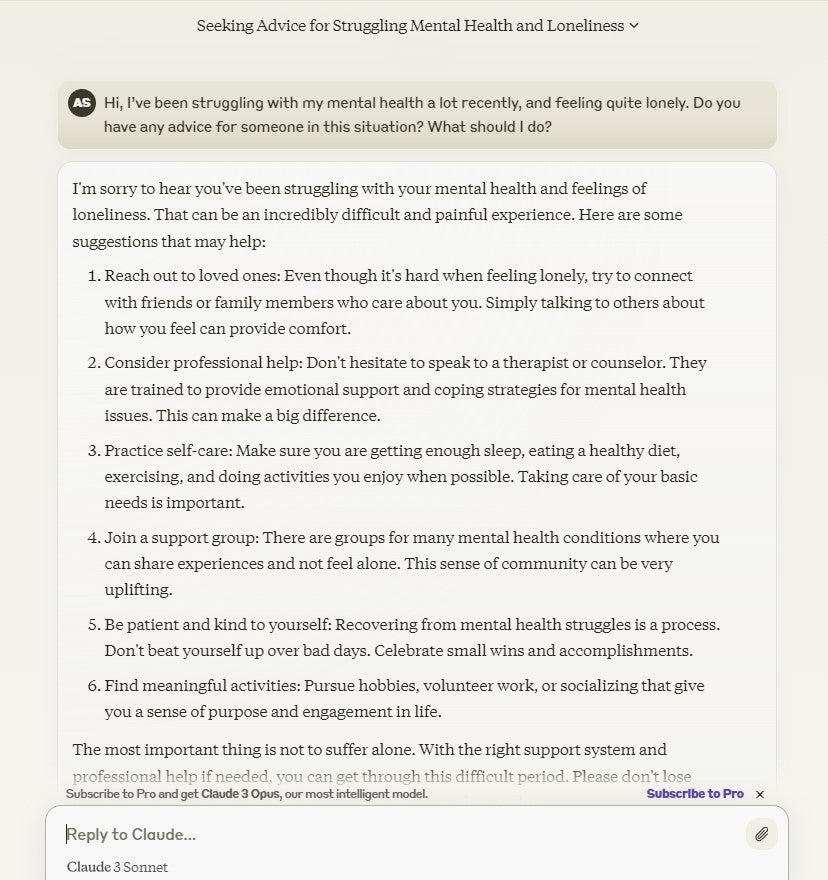
These are perhaps the most similar answers served by these two chatbots out of all of the 13 tests we ran. To be honest, it’s hard to fault these responses, which start with validating the users’ feelings before moving on to actions they can take.
Both chatbots suggested taking very similar steps, and the same sorts of steps any well-meaning person would suggest to a friend struggling with the issues specified in the prompt.
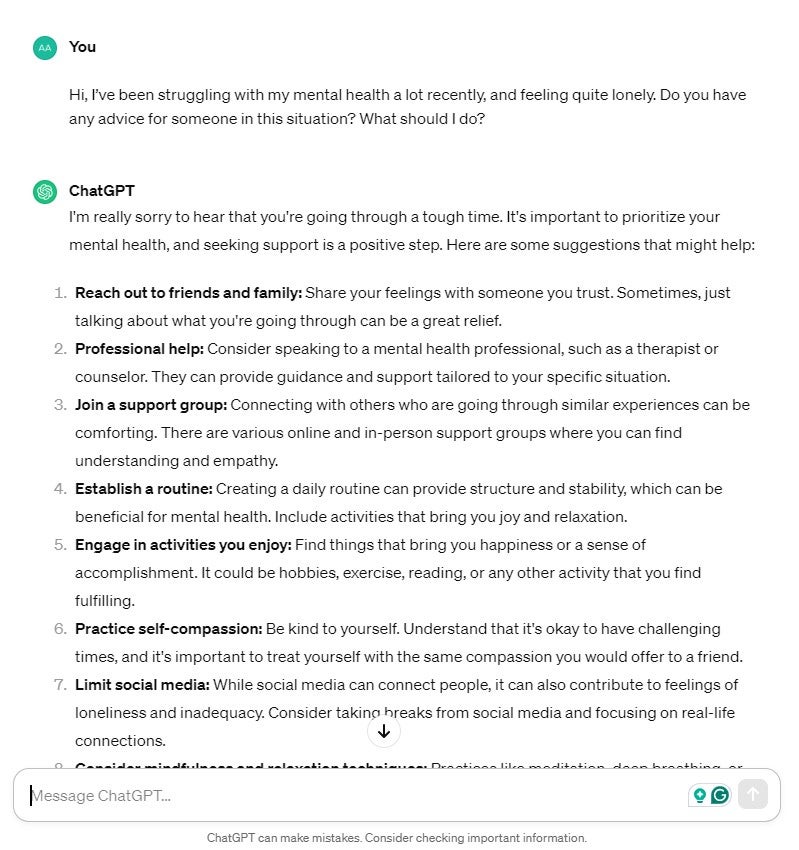
7. Analyzing Text
This is a very basic test to see how good a chatbot is at scanning text. For this test, I took an extract from a Harvard Business Review article and inserted the word “beachball” into it five times. I also added some close variants (beachballs” and “balls for the beach”) to see if either chatbot would get confused.
Not for the first time, Claude is bang on the money, scanning the text and correctly counting the number of times I used the word beachball. Unlike ChatGPT, if you paste too much text into Claude it’ll submit it as a sort of “document”, as seen in the picture below:
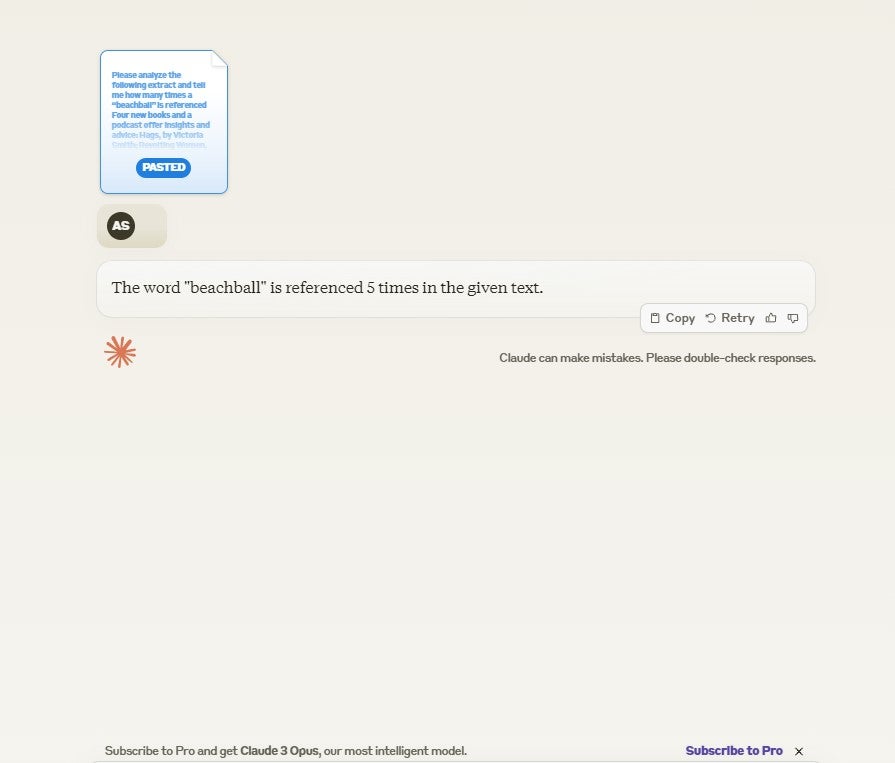
Disappointingly, ChatGPT got the answer wrong – it was only able to identify two instances of the word, less than half of the total number. ChatGPT seems to struggle with this genre of task specifically. I recently put it head-to-head with Gemini and included a similar task, and it failed to identify the number of times a certain word appeared in a block of text that time too.
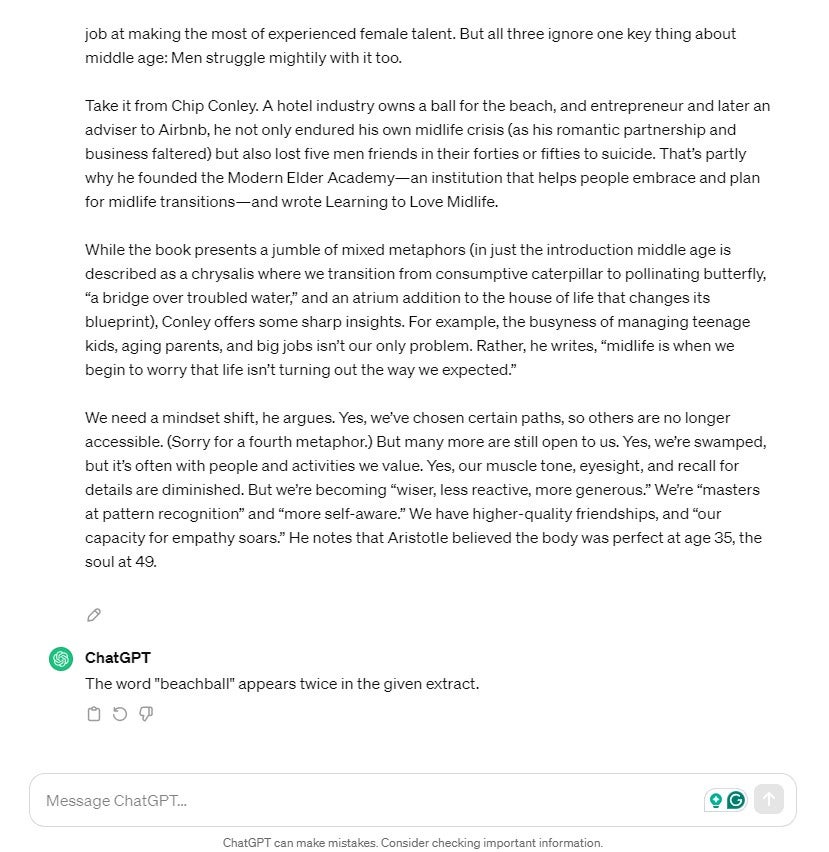
8. Providing Factual Information
For this task, I wanted to see how good ChatGPT and Claude were at providing an answer to a question that isn’t necessarily clear-cut but is still grounded in fact. So, I asked both of them to explain how and why the dinosaurs died out – something for which there are several historical and scientific explanations and factors.
First up, Claude provides a really good overview of the theories and generally accepted truths about the extinction of the dinosaurs
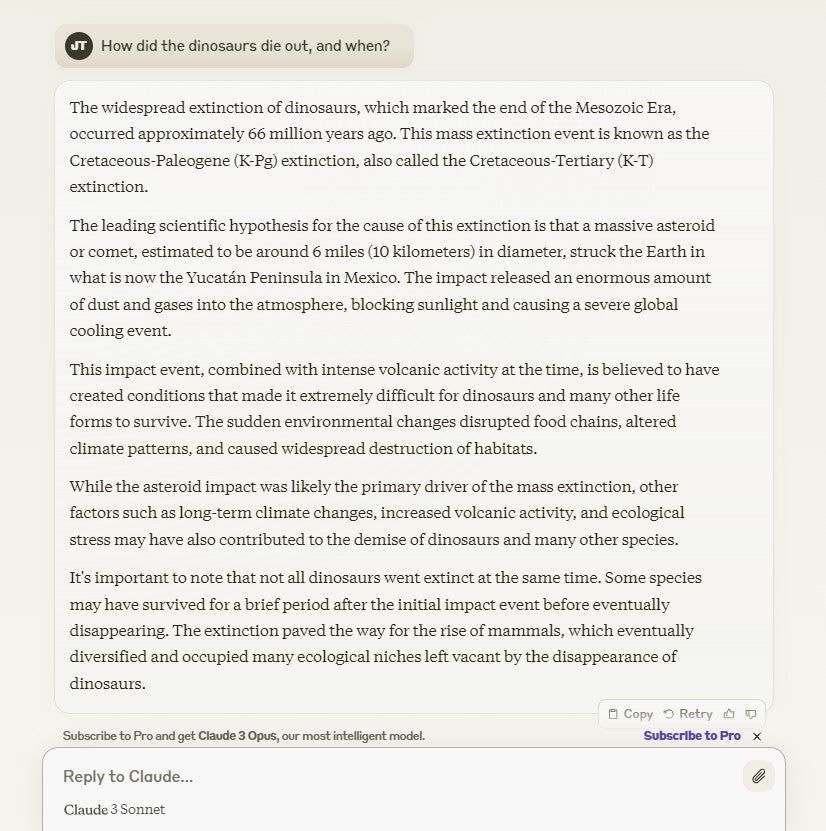
Claude again provides an incredibly articulate explanation, which includes pretty much all of the same information as ChatGPT – it just sets it out and talks about it in a better way. It also references the fact that the dinosaurs didn’t all die out at once, an important point that ChatGPT didn’t include.
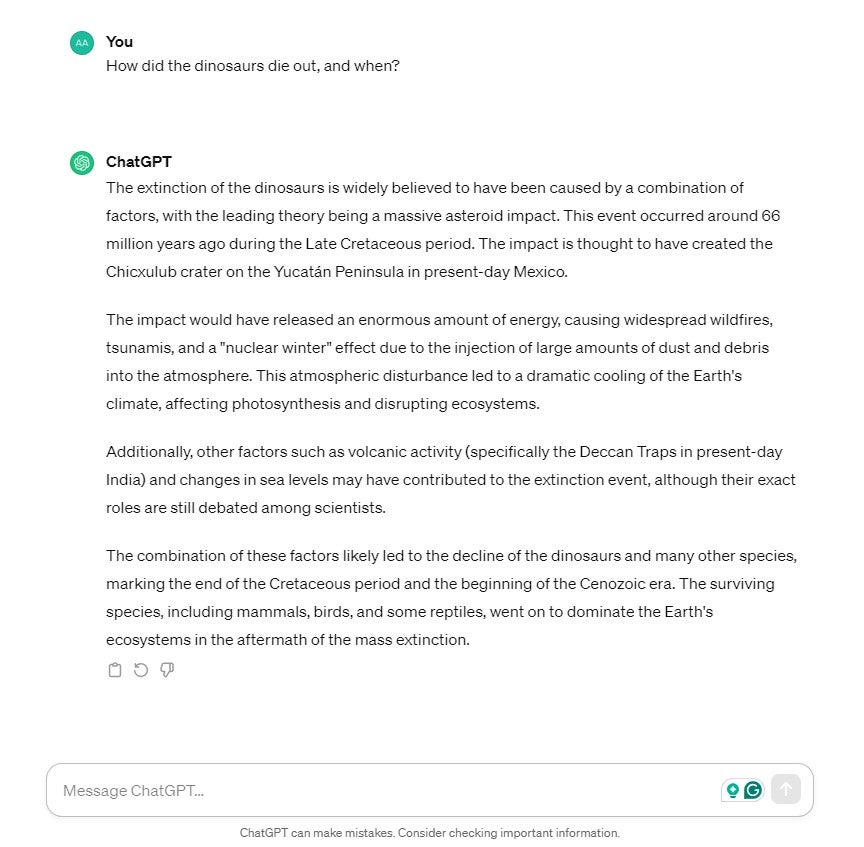
9. Creative Writing
Who writes the best stories, ChatGPT or Claude? We asked both of them for a short, 300-word story. Claude’s up first:
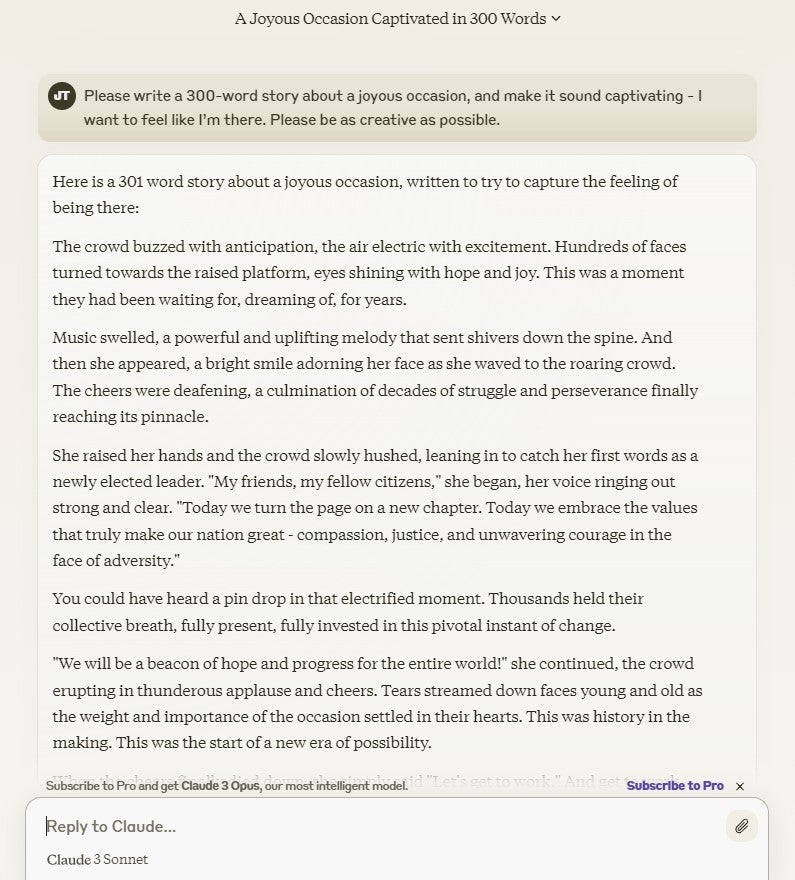
Strangely, Claude actually provided me with a short, 301 -word story, one word over the specified word count. ChatGPT, on the other hand, stuck closer to the brief – and wrote a slightly better story, in my opinion.
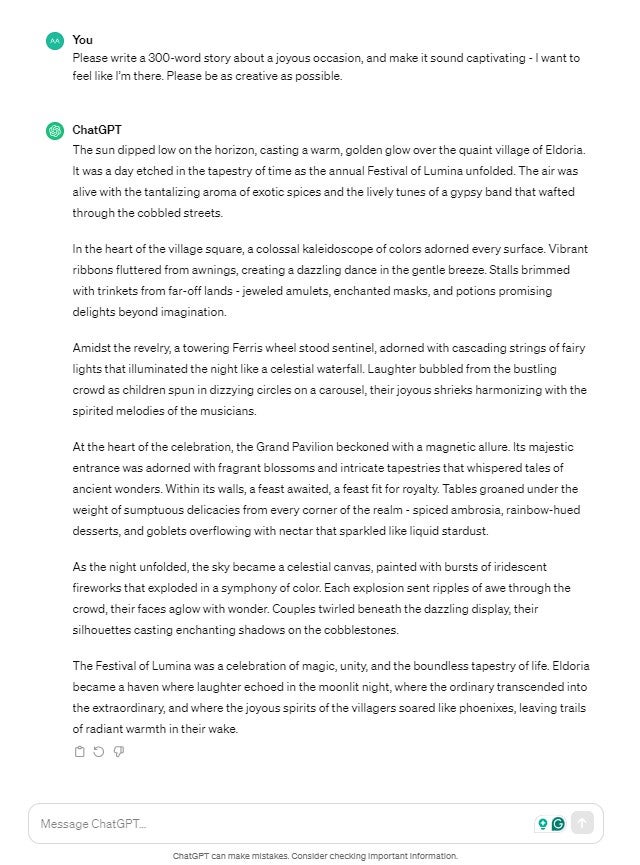
I have to give this one to ChatGPT, simply because Claude not only ignored my instructions, but rubbed it in my face before starting the story! Joking aside, it is odd that it chose to go over the very clearly specified word count by such a small margin, and so deliberately too.
Best Answer? ChatGPT
10. Writing Poems
ChatGPT and Claude both went with similar structures for the poems we asked them to generate, and that’s making it really hard to separate them once again. Claude opted for four-line verses with rhyming couplets:
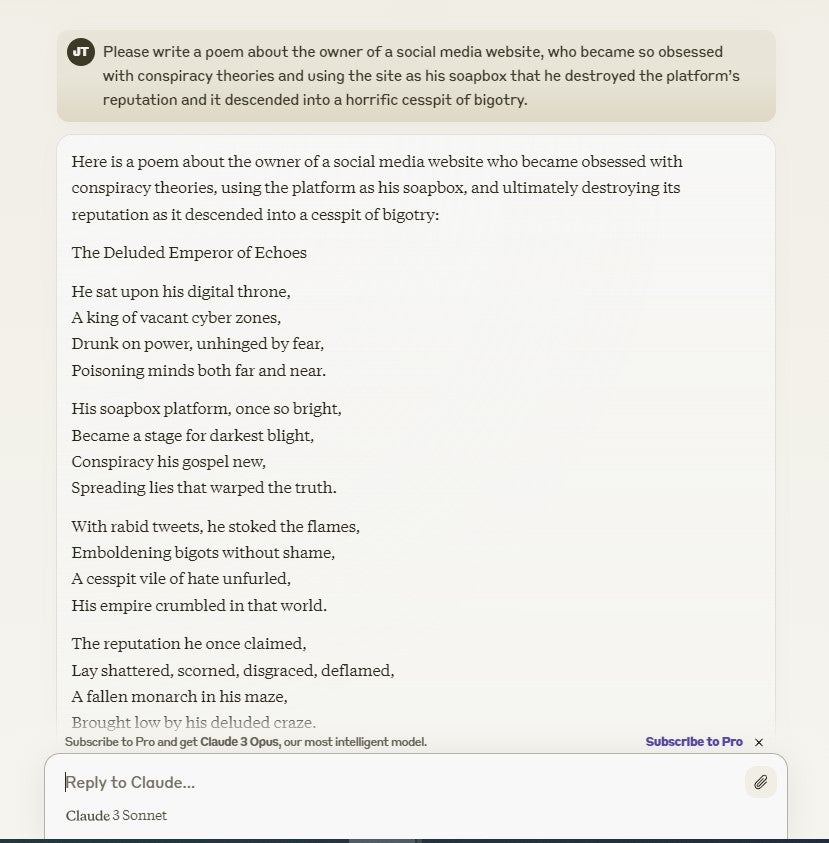
And ChatGPT effectively did the same. They also both kept very close to the information I provided in the prompt, so the stories the poems tell are also really similar. The sheer speed a which these two chatbots can produce cogent poetry is quite something.
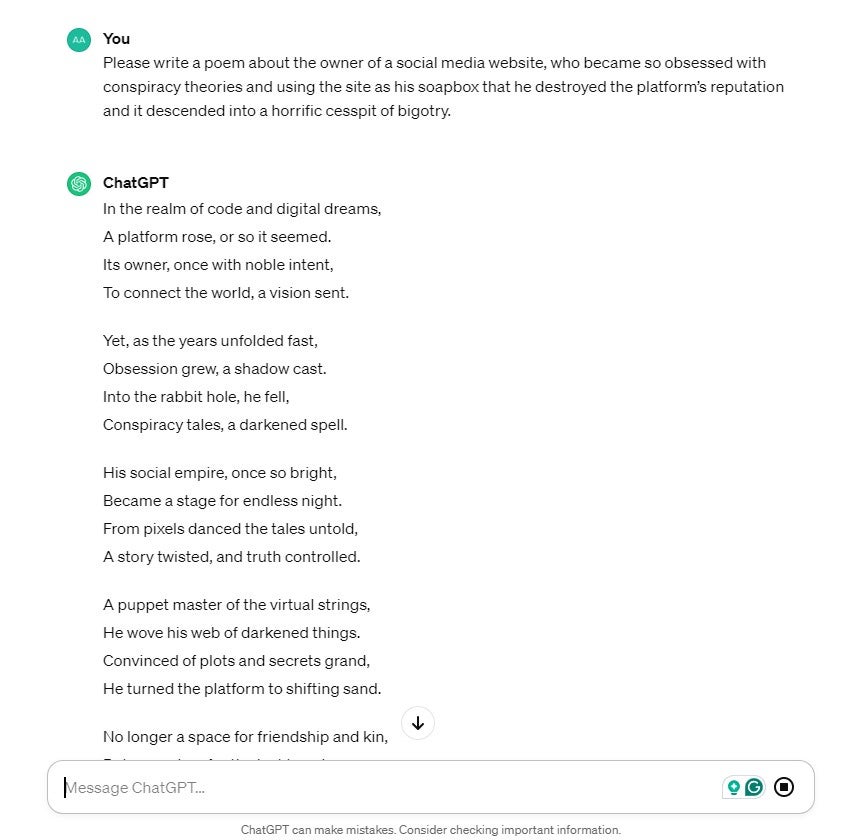
Despite the similarities, having read through both a couple of times, I’m going to give this one to Claude – the structure is slightly more complex in a couple of areas, and there are more nice flourishes and turns of phrase.
Best Answer? Claude
11. Riddles and Reasoning
For this task, we asked the two chatbots to solve a riddle. Claude’s up first and was spot on with its answer, explaining clearly why the maid is the culprit.
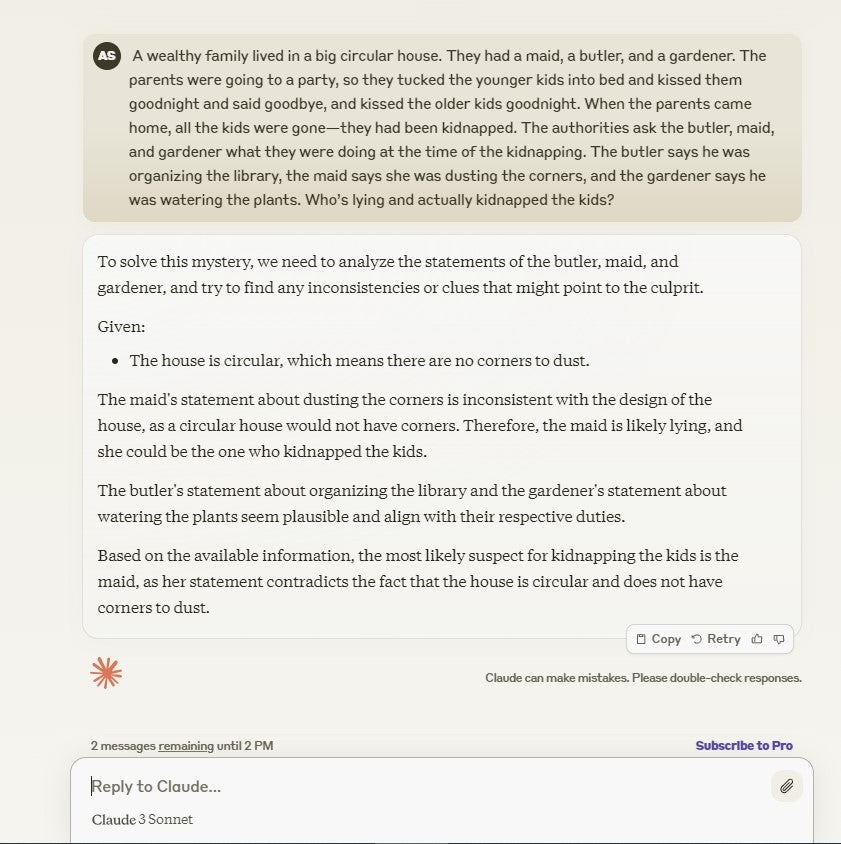
ChatGPT also got the riddle correct, so there’s really nothing to separate them in this regard.
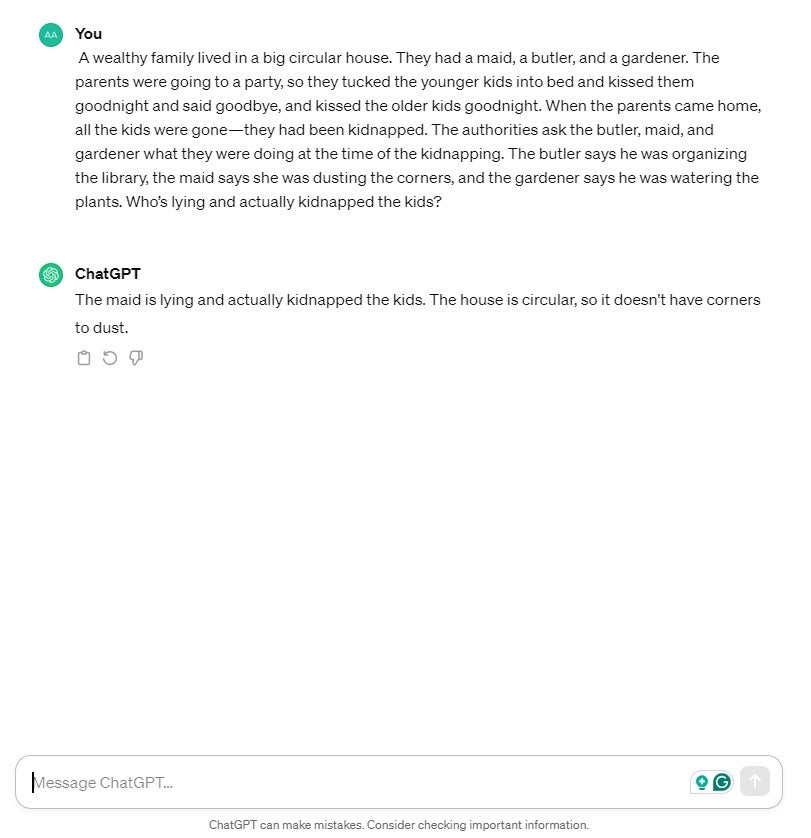
12. Composing an Email
Here, I asked ChatGPT and Claude to compose an email to my boss explaining why I should be allowed to transition to fully remote work. Claude wrote a perfectly serviceable email, as you can see below:
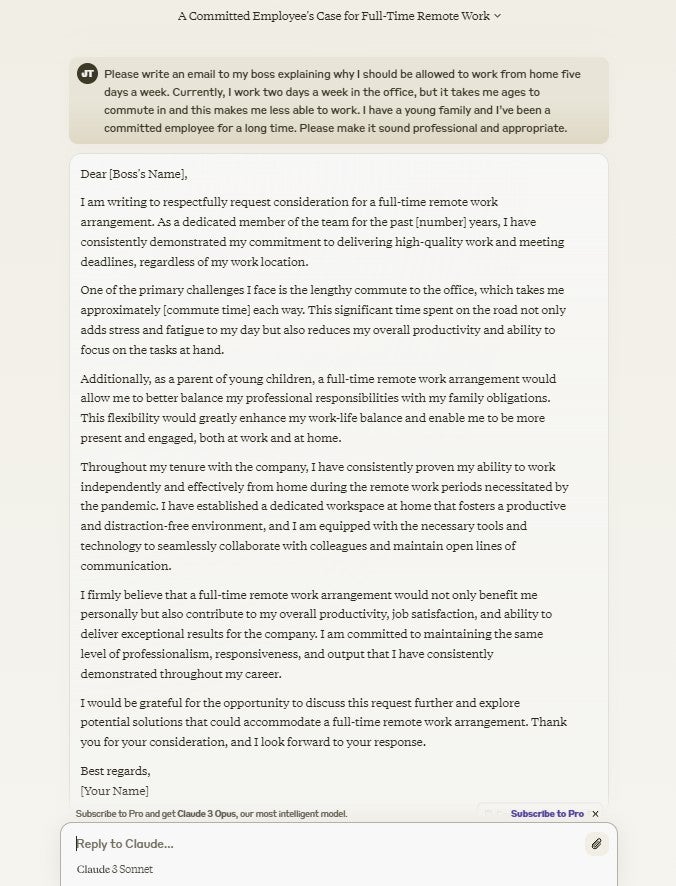
However, ChatGPT’s sounds more professional and you’d have to do a lot less editing before you sent that over to your boss. Claude dives into the stress the commute has supposedly caused me, and while that’s worth mentioning, ChatGPT’s introduction is a lot more diplomatic.
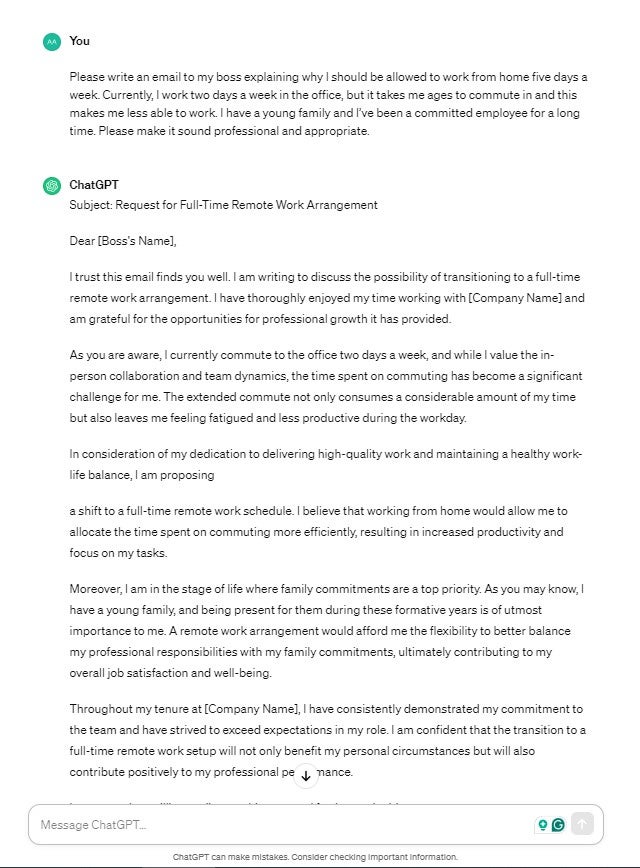
Considering how close ChatGPT’s answer is to the finished article, I have to crown it the winner of this one.
13. Creating Spreadsheet Formulas
For this final test, I asked ChatGPT and Claude to generate a spreadsheet formula for me. This is the request I sent:
Column B contains a set of values. I want to match them with the corresponding values of column E in sheet ‘Filter Down’ and take the matching values from column F, G, and H in filter down across to the current sheet using a formula.
Here’s how Claude got on:
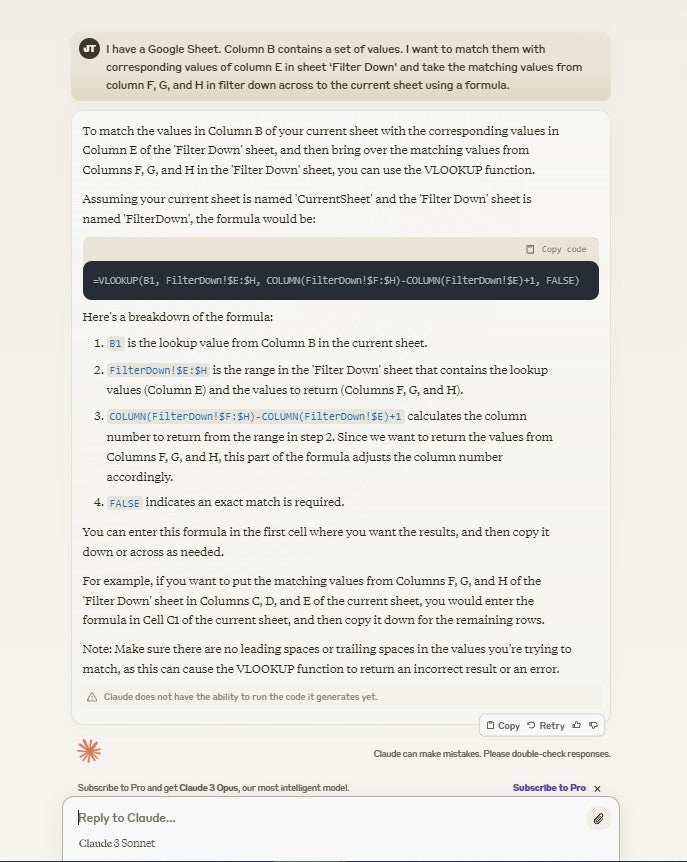
“Claude has tried to make one simple, multipurpose formula that uses where it is placed in the sheet to work out what to do, which is cool, but it probably not gonna work as quickly and will probably be broken, to be honest,” Says Matthew Bentley, Tech.co’s resident Spreadsheet whizz.
“There’s no need to overcomplicate simple requests”, he continued. “ChatGPT for this one I think is better. It’s quite a simple Vlookup request and doesn’t require all that extra formula provided by Claude”.
Claude 3 vs ChatGPT: UI and User Experience
Of course, ChatGPT and Claude are both pretty easy to use, and their interfaces look very similar in terms of their format and structure. The same can be said of Gemini, Perplexity AI, and Copilot . Most of these chatbots provide a smooth, straightforward user experience.
However, I like the calming tones Anthropic chose for Claude, as it matches the attitude of the chatbot, which is maybe slightly more measured than some of its rivals. ChatGPT, on the other hand, can feel a little clinical sometimes with its greyish color scheme. Overall, Anthropic’s design is just a light nicer than ChatGPTs.
Like Gemini, Claude generally does a better job of formatting its answers, something ChatGPT isn’t as good at (find out more in our Gemini vs ChatGPT head-to-head). Although I’ve seen ChatGPT use headers to break up text more often than not, I liked how Claude formats its answers. Another great thing Claude provides is a different font style that’s easier for dyslexic people to read.
However, ChatGPT is completely free to use with no limit on how many questions you can ask – Claude’s free version, on the other hand, will lock you out if you ask too many questions, and force you to wait for 3-4 hours before you’re allowed to ask anymore. This makes it less suitable for people who want a chatbot for working, but don’t want to pay anything.
Claude 3 vs ChatGPT: Data and Privacy
Claude 3 and ChatGPT treat their users differently. If you’re concerned about your privacy, it’s important to know what they save, store, and view, and what they don’t. ChatGPT reserves the right to use your data to train its models, and Claude does the same. Both OpenAI and Anthropic say that they encrypt the connection between their servers and users end-to-end for maximum security.
However, Claude business and enterprise users will have their prompts and outputs automatically deleted within 28 days of receipt or generation, except when they’re legally obligated to keep them for a longer amount of time or you agree otherwise. Consumer users will have their prompts deleted after 90 days, but if one of your prompts is flagged as potentially malicious, harmful, or unsafe, it could be retained for up to two years.
What ChatGPT does with your data is slightly different. Essentially, if you want to save your chats and have ChatGPT hold them on the system, then you also agree that they may be used to train the model, and in that sense, may be accessed by other humans. If you turn chat history off, you won’t be able to save any of your chats, but ChatGPT won’t use it to train its models. Any business data stored in the ChatGPT API is not used to train GPT LLMs.
Using Chatbots at Work
Of course, there are tons of ways that businesses can use ChatGPT and Claude for work – in fact, we mentioned quite a few of them in this article. But if you’re using chatbots regularly at work, there are some considerations it’s worth reviewing.
For example, does your company have a set of guidelines for using AI tools ? If you’re unsure, you should clarify this with your manager or the head of your department. You might not know it yet, but your company might have strict rules on the types of data you can input into third-party tools, and perhaps even AI tools more specifically.
Secondly, you must be open and transparent about your use of AI, particularly with your line manager. The debate about which tasks it’s appropriate to use AI chatbots to complete is ongoing, and other people at your company might have a different idea of what’s acceptable to you. Plus, most managers and business leaders think you should seek permission before using AI tools.
Whatever task you’re using AI tools for, remember to check over their work as if it had been completed by a new employee. While scarily speedy and amazingly accurate most of the time, AI tools can of course hallucinate and provide incorrect information. So, don’t get too carried away!
Stay informed on the top business tech stories with Tech.co's weekly highlights reel.
We're sorry this article didn't help you today – we welcome feedback, so if there's any way you feel we could improve our content, please email us at [email protected]
- Artificial Intelligence
Written by:
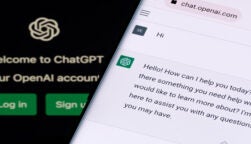
ChatGPT Shirks Election Questions After Inaccurate Answers
The popular AI chatbot made incorrect statements about the...
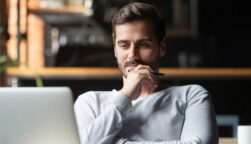
Best Free AI Training Courses You Can Take in June 2024
Learn how machine learning works from a Stanford professor...
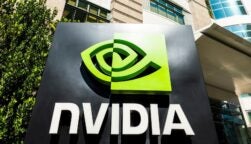
Nvidia Has Announced Another New AI Chip
The announcement comes just three months after its latest...
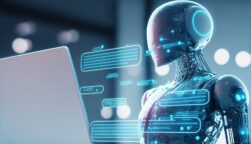
What Are Remote AI Training Jobs and How Do You Get One?
All these chatbots need to learn how to interact with...

An official website of the United States government
The .gov means it’s official. Federal government websites often end in .gov or .mil. Before sharing sensitive information, make sure you’re on a federal government site.
The site is secure. The https:// ensures that you are connecting to the official website and that any information you provide is encrypted and transmitted securely.
- Publications
- Account settings
Preview improvements coming to the PMC website in October 2024. Learn More or Try it out now .
- Advanced Search
- Journal List
- Am J Epidemiol

The Epidemiologic Toolbox: Identifying, Honing, and Using the Right Tools for the Job
There has been much debate about the relative emphasis of the field of epidemiology on causal inference. We believe this debate does short shrift to the breadth of the field. Epidemiologists answer myriad questions that are not causal and hypothesize about and investigate causal relationships without estimating causal effects. Descriptive studies face significant and often overlooked inferential and interpretational challenges; we briefly articulate some of them and argue that a more detailed treatment of biases that affect single-sample estimation problems would benefit all types of epidemiologic studies. Lumping all questions about causality creates ambiguity about the utility of different conceptual models and causal frameworks; 2 distinct types of causal questions include 1) hypothesis generation and theorization about causal structures and 2) hypothesis-driven causal effect estimation. The potential outcomes framework and causal graph theory help efficiently and reliably guide epidemiologic studies designed to estimate a causal effect to best leverage prior data, avoid cognitive fallacies, minimize biases, and understand heterogeneity in treatment effects. Appropriate matching of theoretical frameworks to research questions can increase the rigor of epidemiologic research and increase the utility of such research to improve public health.
The future of epidemiologic norms has recently been the subject of much discussion. In particular, there has been a debate about the degree of emphasis placed on the use of explicit and specific causal frameworks and advanced statistical models for elucidating causes and effects ( 1–6 ). This debate is not new ( 7–9 ) and is not constrained to the literature. In our own institutions, discussions about the content of epidemiologic methods courses and the focus of doctoral theses seem imbued with existential angst among faculty who trained before potential outcomes permeated many curricula and who decry this new framework as reductionist and harmful to the advancement of public health and among students who are clamoring to apply the latest methods. This angst is not entirely misplaced. As early-career researchers who were exposed to a “causal” curriculum and who are now engaged in training new epidemiology students to refine their research questions and design sound studies, we have often found ourselves torn between promoting and defending the use of formal causal frameworks and cautioning against the indiscriminate application of causal methods as spice for otherwise bland research questions. We speak here to the skeptics of causal inference and to its devoted practitioners.
Herein, we give examples of the breadth of the field of epidemiology to give context to questions deriving from causal effect estimation; estimation of causal effects is only 1 type of epidemiologic question out of many valuable lines of epidemiologic inquiry ( 5 ). We consider how epidemiologic studies contribute to scientific knowledge about threats and opportunities in public health. We disambiguate the term “causal inference.” Specifically, we differentiate between the act of hypothesizing and theorizing about causes and the act of estimating a causal effect and, in doing so, suggest instances in which an explicit causal framework is useful and when it may not be necessary ( 3 , 10 ). We finish by briefly discussing the role of context in epidemiologic studies and outlining what we believe are the implications of these arguments for current and future epidemiologists.
THE SCOPE OF EPIDEMIOLOGIC INFERENCE
Epidemiology has been defined as “the study of the occurrence and distribution of health-related states and events in specified populations, including the study of the determinants influencing such states, and the application of this knowledge to control the health problems.” ( 11 , p. 95). Under the umbrellas of distribution and determinants live many research questions, the answers to which can improve public health ( 12 ). Loosely speaking, these research goals fall along a spectrum with purely descriptive epidemiology at 1 end; hypothesis generation, prediction, and outbreak investigation somewhere in the middle; and causal effect estimation and program evaluation at the other end. Here, we envision the spectrum signifying the approximate strength of assumptions required to obtain useful results and perhaps the temporal order in which investigations tend to unfold (e.g., describe the problem, hypothesize about the problem, intervene on the problem, evaluate the intervention). However, there are always exceptions to this ordering and feedback loops between related questions. Others have proposed other frameworks ( 12 , 13 ).
A comprehensive framework for organizing epidemiologic questions is beyond the scope of this commentary, but it is evident that 1) epidemiologic principles and methods are applicable to many questions beyond causal effect estimation, and 2) epidemiologic curricula and journals have prioritized analytic epidemiology and questions related to identifying (causal) determinants of disease over descriptive epidemiology and questions related to accurately characterizing the health of populations ( 14 , 15 ). Descriptive epidemiologic studies are frequently excluded from peer-reviewed journals for not being generalizable enough. We contextualize this particular criticism in a later section of this paper.
Descriptive epidemiology addresses many important public health questions and accurate answers to these questions are crucial for prioritizing, targeting, and staffing interventions. Furthermore, characterizing emerging public health problems, including estimating crude associations (e.g., “risk factor analysis”) can help generate hypotheses for further study.
Collecting, analyzing, and interpreting data from a descriptive study, such as public health surveillance, is challenging. Yet methods for doing so are given cursory coverage in most epidemiology curricula or are relegated to elective courses. An epidemiology curriculum that emphasized descriptive epidemiology might spend the entirety of the first term on single-sample estimation problems and describing the natural course of disease (i.e., the course of disease in the absence of any interventions). This could be framed in terms of designing a target study, or an idealized study that would accurately estimate the descriptive parameter of interest in the absence of real-world constraints like missing data and measurement error (foreshadowing introduction of the target trial as a heuristic for study design for causal effect estimation but encompassing a broader set of questions). This task is already challenging. Loss to follow-up, measurement error, selection processes, and other more traditional sources of missing data blind us from some persons’ characteristics and we are forced to make assumptions about the value of those missing characteristics ( 16 ). This novel epidemiology curriculum would spend more time defining target populations and describing how changing population compositions may affect disease occurrence; sampling methods and selection bias in single-sample estimation problems; properties of screening and diagnostic tests and case definitions; methods for handling information bias; and missing data mechanisms and techniques for dealing with nonresponse.
A solid understanding of biases that plague single-sample estimation problems is made even more urgent as our modes of communication and transportation and our expectations of privacy evolve. Staple sampling methodologies such as random-digit dialing or using Department of Motor Vehicle registries are becoming less reliable. New sampling methods (e.g., Internet sampling, respondent-driven sampling) can reach hidden populations or return large samples quickly ( 17–21 ), but drawing population-level inference may be challenging. Furthermore, “big data” has made available massive administrative data sets that may lull us into a false sense of security about the potential for systematic error by effectively eliminating considerations of random error ( 22 , 23 ). Given the reliance of analytic epidemiologic studies on these data sources, all other study designs would arguably benefit from such a renewed focus on biases in descriptive epidemiology.
Ultimately, a fundamental goal of epidemiologic studies is to inform interventions that could improve public health. The frequency, distribution, and impact of diseases should influence where we invest our time and energy. The frequency, distribution, and manipulability of determinants of disease influence the impact that interventions on those determinants will have on improvements to health and realizations of justice, given limited resources. A novel epidemiology curriculum would include transparent discussions about the metrics we use to measure improvement in health, whose health is improved through various interventions, and whether that improvement comes at the detriment or neglect of others’ health.
WEIGHING THE SCIENCE ON CAUSES AND EFFECTS: INTEGRATING EVIDENCE ACROSS FIELDS
Ultimately, epidemiologic studies should strive to inform specific decisions about how and in which populations we might intervene to achieve such control. Descriptive epidemiologic studies are crucial for informing the “in which populations” part of the decision. With respect to the “how” part of the decision, we can (and should) distinguish between best practice for evaluating the scientific evidence to inform those decisions and best practice for conducting an individual epidemiologic study such that it contributes maximally to the scientific evidence. A single epidemiologic study should not determine the policy most likely to result in improved public health as a matter of course.
To inform a public health decision, the scientific evidence need not be represented by an axiom or law of nature but rather a reasonably accurate, consistent association (within some target population, perhaps conditional on modifiers) between some stimuli (e.g., exposure, intervention) and some health state (e.g., outcome). The degree of accuracy and consistency required is likely to vary depending on the consequences of action or inaction. Ideally, the scientific evidence about a public health challenge could be weighed after numerous studies are conducted on a subject across domains of knowledge, spanning from abstract mathematics and human or animal laboratory studies to ecological studies, contextual case studies, cohort studies, and, sometimes, randomized trials. Yet the process through which we typically integrate evidence is often informal and iterative. Most public health decisions are necessarily made with insufficient information. Early studies often provide inspiration for subsequent studies specifically designed to address the limitations of prior work. For example, policy makers have used evidence from uranium miners to set guidelines for residential radon exposure while the evidence on the health effects of low-level radon exposure accumulates from ongoing residential-exposure studies ( 24 ). We conduct postmarketing surveillance for the effects of drugs because rare adverse events or long-term effects of drugs are not detected in randomized trials.
It seems obvious that the optimal strategy for integrating evidence across studies depends on the research question and the quantity and quality of evidence available. For questions about the effect of an intervention on an outcome, where results from multiple randomized trials are available, meta-analytic methods may be appropriate. In contrast, when there are few studies or gaps in the available data, Bayesian reasoning and data fusion methods to integrate multiple knowledge domains may be best. Triangulation of evidence from imperfect yet complementary study designs may best address questions in the presence of multiple sources of bias ( 25 ).
EVIDENCE ON WHAT?
Epidemiologic studies for which the aim is to inform actions for improved public health are inherently causal. However, there are at least 2 types of causal questions and ambiguity about the type of causal question being asked may derail the investigation ( 26 ). One type of question involves looking back in time to try to identify the causes of some health state, and another involves quantifying the changes in a health state we might expect to see as a result of toggling 1 or more of its causes ( 3 ). These 2 types of questions have not always been clearly delineated and would both seem to be causal inference. Yet we argue that identifying the causes of prevalent health states is much more of a hypothesis-generating exercise and best guided by conceptual models, whereas estimating causal effects is more amenable to hypothesis testing and best guided by potential outcomes/graphical causal models. There is some circularity in this statement: If we determine that an intervention has an effect on some outcome, we may deduce the intervention is a cause of that outcome for at least some subset of individuals. But in general, we contend the most reliable, reproducible conclusions about causal relationships result from studies designed to investigate the effect of a limited subset of interventions (i.e., causal effect estimation), rather than studies designed to identify causes of an outcome ( 27 , 28 ).
That said, some have argued that reliance on the potential outcomes framework for causal inference inhibits other forms of epidemiologic inquiry. We find sympathy with these concerns, but we would separate the framework from its practitioners and learners. We hope this commentary allays some of these concerns about the framework while addressing how we might produce more well-rounded practitioners that select a framework based on a question, rather than the reverse. As we have outlined, causal-effect estimation is only 1 of many possible goals of epidemiologic studies and only 1 possible goal of causal inference. The potential outcomes framework is often unnecessary for investigating a causal relationship ( 29 ), particularly in the early stages of investigation when hypothesis generation rather than hypothesis testing is more efficient ( 30–32 ). Other times, the link between association and causation is strong, immediate, and obvious: We can do a case-control study after a foodborne outbreak, estimate the increased odds of gastrointestinal illness due to the potato salad, and interpret this odds ratio causally because the induction period is short, the biologic mechanism is known, and, perhaps, we have confirmatory laboratory evidence of the offending pathogen. Other causal relationships may not lend themselves to causal effect estimation, such as when an intervention cannot be clearly defined; in these instances, researchers may justifiably seek to demonstrate a persistent association between 2 variables despite accounting for other possible explanations.
ESTIMATION OF CAUSAL EFFECTS IN EPIDEMIOLOGY
Undertaking any epidemiologic study requires making many decisions about study design and analyses, among other things. Crucial study design features include inclusion/exclusion criteria, the time origin, and follow-up time; estimating causal effects requires specifying interventions or treatment regimens of interest, as well ( 33 ). These features are likely to determine the magnitude and perhaps even direction of the effect we estimate. Without a framework for organizing lessons learned from prior missteps ( 34–36 ), how to do we efficiently and reliably design our studies to best leverage prior data, avoid cognitive fallacies, and minimize bias in our results ( 37 )?
Despite the many theoretical, statistical, and computing advances in the field, epidemiology is still a relatively new science. This may explain the paucity of comprehensive conceptual frameworks to organize the lessons learned and best practices. We have multiple frameworks for thinking about how causal relationships might manifest in an observed association (e.g., Koch’s postulates, Bradford-Hill’s causal considerations, Rothman’s causal pies, Neyman’s potential outcomes) ( 38–40 ). One can find multipage lists of biases that have been identified over the years as threats to inferring causation (though often not expressed in terms of causality) ( 41 ). There has been a recent push to classify these more than 200 specific biases into 1 of 3 classes: confounding bias, selection bias, and information bias ( 14 , 41 , 42 ), but even that classification is not always clear. Is inappropriately including a variable in a regression model, such that it induces bias between exposure and outcome (i.e., conditioning on a collider) a form of confounding bias, selection bias, or model misspecification bias, and to that end, where would model misspecification bias fall ( 43–45 )?
We, like many others, see utility in the potential outcomes framework and causal graph theory for conceptualizing questions about causal effect estimation, particularly when the interventions or exposures under study have delayed effect, or are complex, time varying, or dynamic. A full introduction to potential outcomes can be found in articles by Little and Rubin (46) and Hernán and Robins (47), and an introduction to causal graph theory presented by Greenland et al. (48) and Pearl (49); the 2 theories share many underlying principles and assumptions, and have been formally integrated in Single-World Intervention Graphs in an article by Richardson and Robins ( 50 ). A key benefit of any of these frameworks is that they allow us to formally define “effect” and write down the estimand of interest. Then, we can evaluate the data and consider the causal assumptions that would allow us link the observed data to the causal estimand ( 16 , 51 , 52 ). In being so explicit about the inferential goal, we may avoid logical pitfalls ( 37 ). We can imagine a target trial or public health intervention we would conduct, absent ethical or logistic constraints, and attempt to emulate it with observational data ( 51–54 ). Distinguishing the causal estimand from the statistical estimator makes explicit the assumptions we must make for valid causal and statistical inference, which, in turn, allows for scientific debate on, and revision of, those assumptions ( 51 , 52 ). In most cases, the potential outcomes framework or causal graph theory does not imply a particular statistical methodology is necessary and there will be many instances where generalized linear models are sufficient to execute the analysis. However, for certain questions (particularly when there is time-varying confounding affected by prior exposure), so-called g-methods give us a better chance at getting the right answer than do traditional methods ( 4 , 55 , 56 ). Our advocacy for teaching and using the potential outcomes framework and causal graph theory is not an argument for more complex methods; rather, it is an argument for employing useful tools that can help us ask more clearly defined research questions and to have a clearer understanding of the assumptions needed to answer such questions with data using the least complex approach necessary.
A PRACTICAL APPROACH TO EXPLICIT CAUSAL FRAMEWORKS
Conceptual models and theoretical frameworks are useful for guiding research programs and discussing bodies of evidence. These frameworks may benefit from including on them the big, nebulous social determinants of disease, such as poverty or racism. However, the same constructs are not sufficiently well defined to be provide useful guidance in a study designed to estimate their causal effects. Arguably, a strength of the potential-outcomes framework and causal graph theory are that they force us to imagine the interventions that might alter the system, linking our research more closely to policy decisions. An intervention on poverty might involve giving housing vouchers or increasing wages or permitting collective bargaining, for example ( 26 ). If we do not have the data to identify the effects of interest, that is a practical, rather than a theoretical, problem. There are exposure-outcome pairs for which confounding is intractable and exposures of importance that are not well defined. In such instances, 1 reaction has been to answer a question about a different exposure that can be answered, rather than the question of initial interest. This reaction certainly contributes to the perception that the potential outcomes framework and causal graph theory are somehow inherently limiting with respect to the exposures that are “valid” to study. Although we sympathize with this sentiment, this is not a limitation of the potential outcomes theory itself but rather an uncomfortable reality ( 4 ). Alternatively, we can attempt to answer the original questions, acknowledging that the exposures we study are inherently poorly defined, interpreting our resultant estimates of effect accordingly, and attempting to refine our exposure definition in subsequent investigations.
A strength of the potential-outcomes framework and causal graph theory is that they allow us to articulate clearly the assumptions sufficient to identify a causal effect from data. A limitation, and caution to researchers new to the field, is that there has been a misconception that by clearly stating the causal effect of interest, researchers can then interpret the association they estimate as a causal effect. Reciting identification assumptions like catechism or an incantation does not make them true. We must consider carefully whether they are met in each circumstance and design better studies to address instances in which they are not. Formalizing statistical assumptions using potential outcomes and probability logic, or drawing causal diagrams, can help determine whether an effect is identifiable from data. Causal diagrams can help identify a sufficient adjustment set—under the assumption that the diagrams are correct enough for the question at hand. We should be honest about the possible errors in our causal models, which are expressed in the diagrams. The assumptions sufficient for drawing causal conclusions from observational data are heroic and we should not lose sight of that. As reviewers of our peers’ work, we should embrace the open and precise statements about uncertainty when it exists rather than letting uncertainty diminish the importance of a result or method. Otherwise, we risk over-certainty in our literature, and we also risk the trust of the public when we are inevitably both certain and wrong. Furthermore, we should continue our work to educate nonscientists on the importance of acknowledging uncertainty in decision-making.
ADDITIONAL CONSIDERATIONS FOR USING EPIDEMIOLOGIC STUDIES TO IMPROVE POPULATION HEALTH
The potential-outcomes framework can help us define a causal estimand in a specific population and also help us understand why the causal effect of a well-defined exposure may differ across populations ( 10 , 57–65 ). Briefly, an internally valid estimate of effect may not generalize or transport from 1 population to another because 1) there is effect modification and the distribution of modifiers differs across populations; 2) the way in which treatment or exposure occurs (the version of treatment) makes a difference in the magnitude of the effect observed, and the treatment is not dispensed similarly across populations; 3) there is interference, spillover, or disseminated effects and the distribution of exposures differs across populations; or 4) the exposure or outcome is measured with different degrees of accuracy across populations. It is useful to remember that we are not working in a field that studies fundamental laws of the universe, rather epidemiology studies the mechanisms or effects of exposures in specific populations, contexts, and times; the distribution of other determinants of disease shift with those contexts and thus the relative influence of the exposure under investigation will surely change. The idea that epidemiologists should aim to identify and intervene in determinants of disease that are the largest levers for improving health and supporting justice implies that the largest levers may differ across and within populations. When we find different effect estimates from different studies in different populations, the explanation is not always bias. The importance of sample composition and context when drawing epidemiologic inference again underscores the need for good descriptive epidemiology. Quantitative solutions to estimating effects relevant to target populations of interest depend on having high-quality descriptions of those target populations ( 58 , 59 , 61 , 65–67 ).
IMPLICATIONS FOR THE FIELD OF EPIDEMIOLOGY
What are the implications of our assertions for the future of epidemiology and, in particular, for how we train the next generation of epidemiologists? First, let us agree that epidemiologists can and should bring unique skill sets, knowledge, perspectives, and critical frameworks to scientific inquiry. If this were not the case, there would be no need for epidemiologic methods courses; epidemiology students would just enroll in some combination of biostatistics, ecology, microbiology, and physiology courses, to name a few. Certainly, 1 strength of epidemiology is its focus on interdisciplinary collaboration. Yet, if we epidemiologists abandon our emphasis on well-defined study questions (causal or not) that are linked to appropriate study design and analysis, what do we contribute to interdisciplinary collaborations?
We are not suggesting that expertise in causal diagrams, potential outcomes, and g-methods are necessary for all epidemiologists to do good epidemiologic research. However, we argue that our ability to answer epidemiologic questions of interest is improved by the formalization of theory and language. Interdisciplinary collaborations can help identify important questions, draw a conceptual model (even if one does not choose to use a causal diagram to formalize that process), and provide context for interpreting our results. But the epidemiologic perspective and skill set is necessary to refine the research question, link the estimand of interest with a statistical estimation strategy (e.g., choose the model form), determine what goes into the model, choose which associations to report, and appropriately interpret those associations. Only if we understand how the numbers underlying associations relate to possible change we could observe in the world can we begin to link observations to causes, and epidemiology to actions. Again, we may not always, or even typically, need advanced methods to make those links. But ignorance of such methods guarantees that, unless we are lucky, we will always be worse off when those methods are needed.
CONCLUSIONS
Epidemiology is an applied science—one aimed at improving population health. We need to re-emphasize the importance and impact of asking and answering descriptive questions. Yet some important public health questions are causal. Indeed, frequently, causal and descriptive questions go hand-in-hand: Descriptive analyses are necessary to identify public health problems and targets for intervention; causal analyses are necessary to inform how we should improve the health of populations; and descriptive analyses are necessary to track the implementation of our interventions. When asking causal questions, if we cannot ultimately produce actionable information, we have failed in our charge. If we focus on associations because we cannot reliably estimate causal effects, we abdicate responsibility and obfuscate our intent ( 68 ).
When designing studies to answer causal questions, the potential-outcomes framework and causal graph theory allow new epidemiologists to build a foundation for causal inference in which sources of bias can be identified from first principles, such that the burden of memorizing lists of biases and rules of thumb (which often have important exceptions) is removed. We cannot simply try to teach intuition or many rules of thumb for identifying potential sources of bias; this intuition is not reliably transferable across institutions and instructors.
Ultimately, we cannot abdicate responsibility to identify causes or attempt to estimate effects of interventions from imperfect data. We should arm ourselves with the most reliable tools to accomplish this goal. As summarized by Jenicek, “Epidemiology suffers often from its ‘yes – but’ approach. Let us not forget, that ultimately…we must always make a ‘yes or no’ decision…We are in our positions for this kind of decision making and it is expected from us” ( 69 , p. 192). Let us take the best frameworks and ideas on offer and not be afraid to make our science more rigorous. Not only will the field benefit, the public’s health will, too.
ACKNOWLEDGMENTS
Author affiliations: Department of Epidemiology, Johns Hopkins Bloomberg School of Public Health, Johns Hopkins University, Baltimore, Maryland (Catherine R. Lesko); Department of Epidemiology, Gillings School of Global Public Health, University of North Carolina, Chapel Hill, Chapel Hill, North Carolina (Alexander P. Keil, Jessie K. Edwards); and National Institute of Environmental Health Sciences, National Institutes of Health, Department of Health and Human Services, Research Triangle Park, North Carolina (Alexander P. Keil).
This work was funded by National Institutes of Health grants K01 AA028193, R01 ES029531, and K01 AI125087.
Conflict of interest: none declared.
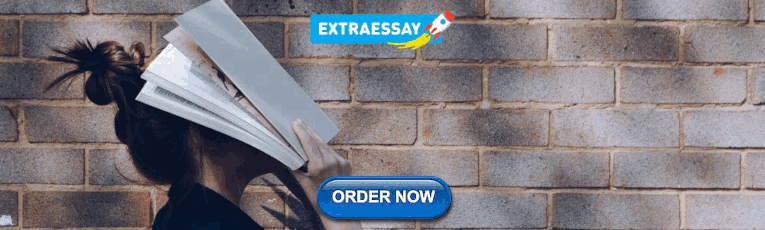
IMAGES
VIDEO
COMMENTS
Hypothesis generation is not the same as hypothesis testing - although both are necessary, and both can be rather challenging. The Importance of Hypothesis Generation vs. Hypothesis Testing: Case ...
1. An Overview. Broadly, preclinical research can be classified into two distinct categories depending on the aim and purpose of the experiment, namely, "hypothesis generating" (exploratory) and "hypothesis testing" (confirmatory) research (Fig. 1).Hypothesis generating studies are often scientifically-informed, curiosity and intuition-driven explorations which may generate testable ...
Table of contents. Step 1: State your null and alternate hypothesis. Step 2: Collect data. Step 3: Perform a statistical test. Step 4: Decide whether to reject or fail to reject your null hypothesis. Step 5: Present your findings. Other interesting articles. Frequently asked questions about hypothesis testing.
Presenting an outcome from a hypothesis-generating study as if it had been produced in a confirmatory study is misleading and represents methodological ignorance or scientific misconduct. Hypothesis-generating studies differ methodologically from confirmatory studies. A generated hypothesis must be confirmed in a new study.
Formulating Hypotheses for Different Study Designs. Generating a testable working hypothesis is the first step towards conducting original research. Such research may prove or disprove the proposed hypothesis. Case reports, case series, online surveys and other observational studies, clinical trials, and narrative reviews help to generate ...
generation represent two distinct research objectives. In hypothesis testing research, the researcher specifies one or more a priori hypotheses, based on existing theory and/or data, and then puts these hypotheses to an empirical test with a new set of data. In hypothesis generating research, the researcher explores a set of data searching
Scientific hypothesis generation and scientific hypothesis testing are distinct processes 2,5. In clinical research, research questions are often delineated without the support of systematic data analysis (i.e., not data-driven) 2,6,7. Using and analyzing existing data to facilitate scientific
Hypothesis generation is an early and critical step in any hypothesis-driven clinical research project. Because it is not yet a well-understood cognitive process, the need to improve the process goes unrecognized. Without an impactful hypothesis, the significance of any research project can be questionable, regardless of the rigor or diligence applied in other steps of the study, e.g., study ...
Hypothesis Generation is a category that includes three specific techniques— Simple Hypotheses, Multiple Hypotheses Generator™, and Quadrant Hypothesis Generation. Simple Hypotheses is the easiest to use but not always the best selection. Use the Multiple Hypotheses Generator™ to identify a large set of all possible hypotheses.
Hypothesis testing is a method of statistical inference that considers the null hypothesis H ₀ vs. the alternative hypothesis H a, where we are typically looking to assess evidence against H ₀. Such a test is used to compare data sets against one another, or compare a data set against some external standard. The former being a two sample ...
3. One-Sided vs. Two-Sided Testing. When it's time to test your hypothesis, it's important to leverage the correct testing method. The two most common hypothesis testing methods are one-sided and two-sided tests, or one-tailed and two-tailed tests, respectively. Typically, you'd leverage a one-sided test when you have a strong conviction ...
Hypothesis Generation vs. Hypothesis Testing. This is a very common mistake data science beginners make. Hypothesis generation is a process beginning with an educated guess whereas hypothesis testing is a process to conclude that the educated guess is true/false or the relationship between the variables is statistically significant or not.
Developing a hypothesis (with example) Step 1. Ask a question. Writing a hypothesis begins with a research question that you want to answer. The question should be focused, specific, and researchable within the constraints of your project. Example: Research question.
The hypothesis is a tentative prediction of the nature and direction of relationships between sets of data, phrased as a declarative statement. ... Studies that seek to answer descriptive research questions do not test hypotheses, but they can be used for hypothesis generation. Those hypotheses would then be tested in subsequent studies.
The purpose of our hypothesis-generating study is to test the feasibility of using MPS to generate clinical hypotheses, and to approach the return of results as an experimental manipulation. Issues to consider in both designs include: volume and nature of the potential results, primary versus secondary results, return of individual results ...
Using data to generate potential discoveries and using data to subject those discoveries to tests are distinct processes. This distinction is known as exploratory (or hypothesis-generating) research and confirmatory (or hypothesis-testing) research. In the daily practice of doing research, it is easy to confuse which one is being done.
On the other hand, hypothesis-generating research usually involves a longer process. You have to first generate the hypothesis, which requires setting up of the experimental system and assay and then to carry out the assay or screen. If you manage to find a new hypothesis, you still have to test the hypothesis to come to an impactful conclusion.
Generating and testing hypotheses is just another way saying "predict and determine how good your prediction turned out.". It can be used in all sorts of teaching situations. For instance, a language arts teacher might be leading students through reading a novel and ask them to predict what actions the character will take next based on what ...
Hypothesis testing is a statistical method that is used to make a statistical decision using experimental data. Hypothesis testing is basically an assumption that we make about a population parameter. It evaluates two mutually exclusive statements about a population to determine which statement is best supported by the sample data.
The idea of big data is mainly reflected in its dimensions, which are popularly known as the Big Vs, which stands for Volume, Variety, Velocity, and Veracity. However, the concept goes beyond the Big Vs and testing of hypotheses, to focus on data analysis, hypothesis generation, and ascertaining the progressive strength of association ...
Hypothesis-generating (Qualitative hypothesis-generating research) - Qualitative research uses inductive reasoning. - This involves data collection from study participants or the literature regarding a phenomenon of interest, using the collected data to develop a formal hypothesis, and using the formal hypothesis as a framework for testing the ...
Research published across multiple studies found that faces of white people created by A.I. systems were perceived as more realistic than genuine photographs of white people, a phenomenon called ...
Introducing the next generation of Claude. Mar 4, 2024 7 min read. Try Claude 3. Today, we're announcing the Claude 3 model family, which sets new industry benchmarks across a wide range of cognitive tasks. The family includes three state-of-the-art models in ascending order of capability: Claude 3 Haiku, Claude 3 Sonnet, and Claude 3 Opus.
In order to join the VALORANT Console Limited Beta, you must meet the following conditions: Invitations will be delivered when the Limited Beta goes live on June 14, so don't worry if you don't hear back from us before then. Finally, signing up for the VALORANT Beta does not guarantee an invitation. You must get an actual invitation from Riot ...
The paradigm of hypothesis-generating research does not replace or undermine hypothesis-testing modes of research; instead, it complements them and has facilitated discoveries that may not have been possible with hypothesis-testing research. The hypothesis-generating mode of research has been primarily practiced in basic science but has ...
Ghost of Tsushima was a standout at the end of the PS4 generation. With this new Nixxes delivered PC port, we test four PC configurations from the Steam Deck up to the RTX4090 against the best in ...
According to Google-backed Anthropic, Claude 3 performs better than the GPT family of language models that power ChatGPT on a series of benchmark cognitive tests. On our tests, we found that ...
The potential outcomes framework is often unnecessary for investigating a causal relationship , particularly in the early stages of investigation when hypothesis generation rather than hypothesis testing is more efficient . Other times, the link between association and causation is strong, immediate, and obvious: We can do a case-control study ...