
An official website of the United States government
The .gov means it’s official. Federal government websites often end in .gov or .mil. Before sharing sensitive information, make sure you’re on a federal government site.
The site is secure. The https:// ensures that you are connecting to the official website and that any information you provide is encrypted and transmitted securely.
- Publications
- Account settings
Preview improvements coming to the PMC website in October 2024. Learn More or Try it out now .
- Advanced Search
- Journal List

Factors impacting employee turnover intentions among professionals in Sri Lankan startups
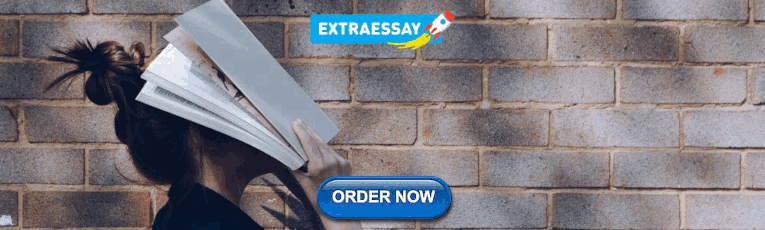
Lakshmi Kanchana
1 SLIIT Business School, Sri Lanka Institute of Information Technology, Malabe, Sri Lanka
2 Ceyentra Technologies, Panadura, Sri Lanka
Ruwan Jayathilaka
3 Department of Information Management, SLIIT Business School, Sri Lanka Institute of Information Technology, Malabe, Sri Lanka
Associated Data
All relevant data are within the paper and its Supporting Information files ( S2 Appendix . Data File).
Employee turnover is one of the topical issues worldwide. The impact of factors affecting employee turnover varies occasionally and new factors are considered. Many countries have examined various factors that affect employee turnover. The main objective of this research is to consider psychographics and socio-demographic factors in one study and analyse the impact on employee turnover. A Probit regression model through the stepwise technique was used to analyse the collected data. Using ventures in Sri Lanka as a case study, this study demonstrates that employee turnover occurs in different stages and independent factors impact differently in each stage. The study population was professionals who have been a key part of Sri Lankan startups, which involved 230 respondents. Data analysis was performed through a forward stepwise technique through STATA. The results verified that job satisfaction and co-worker support negatively impact employee turnover, whereas leader member exchange positively impacts employee turnover. This study also proved a significant positive relationship between male employees in their thirties and high employee turnover. This study’s findings help to identify the areas management should focus on to minimise employee turnover to retain experienced and skilled employees.
Introduction
Having the right combination of human resources/employees can assist firms to be effective in driving change, boosting business performance, as well as to achieving and sustaining a competitive edge. Companies need to give high priority to employee development and predict employee behaviour [ 1 ]. Organisations spend more time and take much effort to identify the good fit employees for the company. Companies invest in many ways for employees, as they are one of the organisation’s valuable assets [ 2 ]. Organisations conduct workshops for employees, buy online tutorials, evaluate employee performance, and provide feedback to them, which are some common types of investments in human resources. These processes sharpen employees’ skills and capabilities, directly affecting the organisation’s success. However, some organisations are weak in strategy adoption while not focusing constantly on these processes or employee voice. As such, these employees suddenly quit the company resulting in increased employee turnover. The issue of employee turnover is considered as one of the global obstacles for organisations worldwide, which directly and adversely affects strategic plans and opportunities of gaining competitive advantages [ 3 ]. As such, this issue can have massive effects on a company’s performance, especially for new businesses and startups. Therefore, it is essential to identify the factors that affect employee retention, which is also a topical issue worldwide. This type of approach enables businesses to achieve its strategic goals while retaining satisfied and skilful employees.
Many variables influence employee turnover intentions [ 4 – 6 ]. Previous studies imply that job satisfaction, work-life balance, trust, and management support are the critical factors that impact employee retention [ 7 – 9 ]. Further, promoting employee well-being leads to decrease employee turnover [ 10 ]. Providing psychological and social support through counselling promotes the quality of work-life [ 11 ]. With time, newly considered factors such as leader member exchange, workplace culture, happiness, joy in the workplace, career management, innovative work behaviour and employee delight are equally important and have been identified. As such, it is important to focus on these factors and build relationships between employees and the organisation.
Firm performance reflects the ability of an organisation to use its human resources and other material resources to achieve its goals and objectives. Firm performance belongs to the economic category, and it should consider the use of business means efficiently during the production and consumption process [ 12 ]. Employee retention is defined as encouraging employees to remain in the organisation for a long period or the organisation’s ability to minimised employee turnover [ 13 ]. Turnover intention is the intention of the employee to change the job or organisation voluntarily [ 14 ].
Sri Lankan business firms were chosen as a case study to examine this resarch problem. In Sri Lanka, over 1 million (Mn) businesses operate. By 2018, 10,510 new businesses had been registered in Sri Lanka. Among these companies, startup companies play a key role in the Sri Lankan economy. Startups come up with radical innovations and changes, and these disrupt the existing market with new products and services. Furthermore, Sri Lanka has a middle rank of ease of doing business. With these favourable conditions and educational and family backgrounds, many people like to apply their new idea and fill the market gap. The new generation in Sri Lanka are interested/are keen on innovations at work and being a part of unique products or services. Currently, most startups are technology-driven and do not have geographical limitations.
Startups are expanding day by day. These businesses are in different stages as ideation, traction, break-even, profit, scaling and stable. According to the “Sri Lanka Startup Report 2019” issued by PricewaterhouseCoopers (PWC), “55% of startups responded are in the growing revenue or expansion stage, 29% of respondents reported an annual revenue of more than Sri Lankan Rupees (LKR) 10 Mn, 40% are still in the less than LKR 1 Mn revenue category and 61% of respondents reported being profitable”. In this setting, employee turnover can be a setback for most startups yet to reach business stability.
Most startups are relatively new. According to (PWC) [ 15 ], 36% of the businesses have operated for less than a year, 44% have been in operation for 1–3 years and only 8% have operated for more than five years. These are still growing and in the early stages of executing their strategies. In this situation, most companies are willing to expand their staff strength. PricewaterhouseCoopers [ 15 ] evidenced that 82% of companies were willing to do so in the next year.
Studies conducted in Asian countries on this subject are assumably similar to the situation of Sri Lanka [ 4 , 5 , 16 ]. This study aims to create a model with critical and newly identified independent factors (job satisfaction, work-life balance, happiness, management support, career management, innovative work behaviour, leader member exchange, and co-worker support) influencing employee turnover in Sri Lankan startups.
Based on their knowledge and the existing literature, authors have considered widely used factors to investigate the employee turnover issue. Therefore, job satisfaction, happiness, work-life balance, career management, management support, innovative work behaviour, leader member exchange and co-worker support were selected based on previous literature findings [ 4 – 6 , 8 , 17 – 19 ]. As in the previous papers and along with the current study’s results, authors identified both positive and negative impacts on employee turnover among Sri Lankan startups.
This study aims to analyse the impact of job satisfaction, happiness, work-life balance, career management, management support, innovative work behaviour, leader member exchange, and co-worker support on employee turnover in startups in Sri Lanka. The present study’s scientific value can be elaborated by comparing it with previous studies. This study’s contribution can be explained in five ways. Firstly, the most critical and newly considered factors were identified together with the support of past literature. Secondly, the present study was classified into different levels of employee turnover. As such, by considering the various levels, the micro-level changes and probabilities of the impact on employee turnover can be better identified. Further, this study helps to reduce the methodological gap. Thirdly, the Sri Lankan context has been selected as the case study. This is because, to the best of the authors’ knowledge, there was no previous research done by local researchers that includes all the widely measured variables investigating the combined effect on employee turnover. Fourthly, the analysis results can be used to identify the strengths and weaknesses of startups in Sri Lanka. Finally, this study identifies the challenges faced by startups and identifies how policy modifications can strengthen the startup ecosystem.
The upcoming sections of this paper are structured as follows. Section 2 discusses the literature review, and section 3 explains data and methodology, Section 4 contains results and discussion highlighting how the research objectives are achieved. Section 5 marks the conclusion, with implications, research limitations and future research directions.
Literature review
As employee turnover is one of the most critical indicators for an organisation, many studies have been conducted on this topic with dissimilar demographical and geographical samples. The existing literature adds theoretical or methodological improvements to this topic. Accoridngly, this study included most variables that significantly impact employee turnover, summarising the independent variables that affect employee retention.
This study is based on the initially defined 47 journal articles through advanced filtration. Reputed journal databases, such as Emerald insight, Science Direct, Taylor & Francis, SAGE journals, ResearchGate, Sabinet, IEEE Xplore and Google Scholar were referred. Fig 1 below describes the literature search flow. Thirteen articles were excluded due to overlapping, insufficient information and irrelevant to the topic. The selected articles have been sorted according to the independent variables.

Source: Based on authors’ observations.
S4 Appendix contains the literature summary of the above presented literature search flow diagram. The following sections present the details of each category.
Job satisfaction
Job satisfaction refers to the employee’s positive emotions, feeling and attitudes on the job and workplace. Positive emotional experiences directly affect higher job satisfaction [ 7 ]. Kim, Knutson [ 7 ] found that satisfaction significantly affects employee turnover regardless of the generation of the employee. Gen Y employees do not easily build loyalty toward the organisation unlike older employees. Turnover intentions seem significantly higher in new generations compared to older generations. New generations are impatient with their organisation and older generations are more patient with it. However, even the new generation of employees tends to stay in their organisation if their level of satisfaction is acceptable. They found that newer the generation of employee, satisfaction level and loyalty is lower than the older generation. This shows that employee turnover is higher in newer generations. Feedback obtained from most employees in generations Y and Z in startups supports this finding.
Da Camara, Dulewicz [ 20 ] found that organisational emotional intelligence has a larger effect on employee satisfaction. Further, this study has discovered that organisational emotional intelligence helped improve job satisfaction and commitment, which reduced turnover intentions significantly. However, organisational commitment and satisfaction describe only 19% of the total intention to leave. Moreover, the descriptive statistics found a high level of job satisfaction and the intention to leave was at the mid or average level of the scale. Camara further stated that job satisfaction clearly implies the feeling about their job. But some research findings can be contradictory. Some employees are fully satisfied with the job and still want to leave the organisation for various reasons. However, this research focused only on charity workers. As such, it is important to gather many indicators that affect employee turnover and thereafter, one can analyse the real situation and generalise the findings.
Satisfaction also depends on the number of employees at the same level. When it gets higher, job satisfaction increases and reduces the intention to leave [ 8 ]. This study found that female employees are more satisfied with their jobs, while older employees are more likely to leave the organisation. However, this study focused only on online-level employees and supervisors.
Oosthuizen, Coetze and Munro studied the relationship between job satisfaction and turnover intention in the IT industry. Oosthuizen, Coetzee [ 6 ] revealed that job satisfaction significantly predicted employee turnover. The study also found that the work-home life balance has a major effect on job satisfaction. Predicting turnover intention based on overall work-life balance is a tough task. The findings further proved that white employees show less job satisfaction compared to black employees. However, they didn’t observe any significant interaction between overall work-life balance and job satisfaction in predicting employee turnover intention. With these results, this indicator must be examined further.
Considering the Asian context, Pakistan IT professionals’ turnover intentions were studied in a similar research [ 21 ]. Recruitment & section, team & management support, performance & career management, salary & compensation, employee commitment, job security, recognition, organisational demographics, and personal demographics have an effect on job satisfaction. However, this study suggested adding more factors, such as work-life balance and employee engagement, which may significantly impact employee retention. This means that human resource management has a significant influence on job satisfaction.
The study by Zeffane and Bani Melhem [ 22 ] investigated the turnover intention of public and private sector employees in the United Arab Emirates. Here, the researchers revealed that government employees are more satisfied with their job and are most unlikely to leave than private sector employees. The turnover intentions of private sector employees are not significantly affected by job satisfaction, whereas the public sector is almost affected by it. Kaur and Randhawa [ 16 ] examined the turnover intention of Indian private school teachers. It revealed that job satisfaction has a direct link with the civil status of the teachers, explaining that married teachers tend to have less job satisfaction. However, for unmarried teachers, there is more intention to leave organisations. Supervisor’s influence had indirect impacts on turnover intentions. However, this research limited the sample to private school female teachers. Here, the study highlighted the importance of having more influencing variables on employee retention and recommended considering these for a comprehensive analysis. Only then the model can be near to the real situation.
Thomas A. Wright [ 2 ] discovered that the employee’s well-being moderates the relationship between satisfaction and turnover intention. Satisfaction had a strong negative relationship with turnover intention, while well-being remained low. The study by Nae and Choi [ 23 ] evidenced the direct relationship between job satisfaction and employee turnover. However, this also pointed out that employee well-being moderates the indirect relationship between job satisfaction and turnover. However, this moderator was significant only for a few specified occasions, such as employees having a highly secure attachment, and low counter-dependent and over-dependent attachment styles.
As per the literature, job satisfaction is an important factor in determining the impact on employee turnover. Accordingly, hypothesis one has been developed.
Work-life balance
Work-life balance can be identified as the satisfactory co-existing of an employee’s work-life and personal life. On one hand. this led to a positive influence on both employees and the organisation. On the other hand, negative work-life-balance has harmful effects on employees. Most employees had abuse alcohol due to this issue in the hospitality industry, which indirectly influences the organisation’s productivity. Additionally, most women have suffered from depression due to poor work-life balance in the hospitality industry. Besides, burnout, exhaustion, and stress are common among employees with poor work-life balance. Therefore, the employee’s commitment heavily depends on work-life balance, an essential requirement for employee retention [ 24 ]. This study states that it can be developed by adding more independent variables such as commitment and job satisfaction.
The highly negative work-life interference has amplified the turnover intentions of IT employees in Pakistan. They also found that the organisation that invested heavily in creating proper work-life balance recorded the lowest turnover among other organisations in the IT industry in Pakistan. Oosthuizen, Coetzee [ 6 ] revealed that the overall work-life balance had no clear influence on the satisfaction of an employee’s current job. Gender was a primary separation point of work-life balance variation among employees. Female employees looked more satisfied with their work-life balance than male employees [ 6 ]. In this light, work-life balance is one part of quality work life other than career opportunities and job characteristics. Organisational embeddedness has a positive and strong relationship with work-life balance. Positive work-life balance has a negative relationship with turnover intention [ 25 ]. However, the sample of this research was based on two healthcare firms. Since the whole world is tech-driven, it is realistic to focus on the IT industry too for generalisability of findings.
According to this study, superiors’ influence on work-life balance highly impacts job satisfaction. Supportiveness and the supervisor’s flexibility on subordinates’ help achieve the desired work-life balance for employees. As noted before, the employee turnover intention is heavily dependent on work-life balance. As such, a study on work-life balance can predict the turnover intention of an employee accurately compared to other factors. Work-life balance can be measured and categorised into three. Interference of work on personal life, work and family conflict and facilitation of work and family are those categories that the researcher suggested. The sample for the study of Kaur and Randhawa [ 16 ] was Indian private school teachers. The researcher suggested that formulating teacher-friendly policies to enhance work-life balance will reduce teachers’ turnover intentions. The researcher also suggested that the imbalance workload on employees supports increasing employee turnover intentions. However, most of the employees in this study were females.
Organisations that focused on employees’ proper work-life balance have recorded better efficiency, innovation, and talent retention [ 26 ]. Employee engagement and life satisfaction have been significantly mediated by the work-life balance of restaurant employees in Nevada, USA [ 27 ]. However, there are not sufficient recent researchers in Sri Lanka on work-life balance and employee retention. Therefore, taking up this study as an opportunity to research is essential. According to the above literature, hypothesis two has been constructed; work-life balance has a negative impact on employee turnover.
Employee happiness is a psychological feeling they have with the workplace. This is an essential factor in maintaining a successful and profitable organisation.Wright and Cropanzano [ 17 ] described happiness as phycological well-being. Personal well-being is one better way to explain employee retention. By moderating this factor, firms can achieve better employee turnover.
The workplace must be a source of happiness for employees. Unhappy employees in a workplace tend to increase employee turnover, absenteeism, low productivity, and time wasted deadlines. Creating happiness within the workplace is not a simple process. It is a comprehensive and continuous process. Happy employees generally have a fair idea of the organisation’s vision, mission and values. Employees in each department should have a clear idea about their goals [ 28 ]. However, happy employees are not always productive. But they can guide and explore things without organisations forcing them. Those employees required proper career management and support to be productive.
The workplace’s physical environment plays a major role in employee happiness and cheerfulness and friendliness of the physical environment are fundamentals. Employee’s attitude also has a more significant effect on happiness. Gratitude, appreciation, servant leadership from the organisation, hope and interpersonal connection are the main factors that affect the employee’s positive attitude. Humour, fun and games also play a major role in keeping employees happy. Other than those factors, wellness activities, celebrations and compensation are the minor factors affecting employee happiness. Based on the above cited literature, hypothesis three can be developed; employee happiness has a negative impact on employee turnover.
Management support
Management support is a must in the move from a good to a great company. Management stands by employees and supports them mentally and physically. Van den Heuvel, Freese [ 29 ] conducted research from the data of 699 employees at three divisions within the Dutch subsidiary of a multinational organisation. Management increased employee autonomy by supporting them to work from anywhere at any hour. This positively affected employee engagement and was negatively related to employee retention. Trust in management is a critical factor in employee turnover.
A cross-sectional survey has been conducted for front-line healthcare staff in China by Li, Mohamed [ 30 ] to measure the impact of organisational support on employee turnover intention. This study’s results could verify that organisational support negatively affected employee turnover intention. Saoula and Johari [ 31 ] studied this area and determined a negative relationship between organisational support and employee turnover intention. As both of the above explained research have been conducted in non-Western countries, the findings help to complete the theoretical framework for the current study in the Sri Lankan context.
Wong and Wong [ 5 ] researched the world’s most populous county, China, to identify the relationship between perceived organisational support and employee turnover. The findings suggested that trust, job security and distributive justice negatively impact employee turnover. However, China is an Asian country, and these similarities may apply to specific research findings in the Sri Lankan context.
Employee perception of management support for employee health is a factor in employee retention. Xiu, Dauner [ 32 ] studied this area with employees’ data from a public university, which was the first empirical examination of organisational support for employee health and retention. This kind of approach leads to building trust with employees. Moreover, these findings are essential to human resource managers who are willing to promote employee well-being at the workplace. Hypothesis four has been developed based on above discussed literature.
Career management
Initiatives must carry out different strategies for old and young employees because their priorities are different. Digest [ 18 ] discloses that young employees are impressed by flexible working opportunities, career advancement, positive working relationships and inclusive management forms. Young employees are more likely to be talented, leading to an organisation’s success and they can also become key workers in the company.
Saoula and Johari [ 31 ] researched the effect of personality traits (big five) on employee turnover intention. The researchers state that the relationship between the big five personality traits and turnover intention will support early prediction of employee turnover intentions. Identifying employee’s personalities and helping them to find the most suitable job role is a long-term process, though it will be highly advantageous for both employees and the organisation.
Rawashdeh and Tamimi [ 33 ] focused on the latest management developments of leading organisations worldwide. They state that there is a strong relationship between the availability of training and supervisor support for training and organisational commitment. Further, they proved that there is a strong negative association between organisational commitment and employee retention. These research findings verify the social exchange theory [ 34 ]. However, the research suggested that the above study can improve by adding new factors like motivation and co-worker support for training. Hypothesis five has been developed by concluding the above explained literature.
Innovative work behaviour
Innovative behaviour is a leading factor in gaining a competitive advantage. Shih, Posthuma [ 35 ] investigated the negative impacts of innovative work behaviour on employee turnover and conflict with co-workers. According to the studies, there is a positive relationship between innovative work behaviour and employee turnover. Further, it found that perceived distributive fairness can negatively moderate this relationship. However, the writer has suggested extending the research to different geographical locations and industries.
The organisational learning culture is a key factor for innovative work behaviour. Saoula, Fareed [ 36 ] conducted research in Malayasia, a developing country in Asia to examine the relationship between organisational learning culture and employee turnover intention. The organisational learning culture improves learning capability, supports sustainable development, and affects organisation’s positive changes. As organisational learning culture and employee turnover intention have a negative relationship, the result helps to identify the impact of innovative work behaviour. According to the existing literature, limited studies have been conducted on this topic.
Agarwal, Datta [ 4 ] conducted research with managerial employees in India to examine the relationship between innovative work behaviour and employee turnover. This study asserted that the variables have an inverse relationship. As innovative work behaviour examinations in an Asian county country like India, it is important to consider this variable in this model. With the presence of the above mentioned literature, hypothesis six has been formulated.
Leader member exchange
As per many leadership methods, leader member exchange depends on the leadership style. Tobias M. Huning [ 37 ] conducted research to identify the effect of servant leadership on employee turnover. Servant leadership supports employee empowerment, development, interpersonal acceptance, and courage. This study found that servant leadership negatively impacts employee turnover. However, this leadership style does not directly affect employee retention. Gyensare, Kumedzro [ 38 ] studied the impact of transformational leadership on employee turnover. This type of leadership supports work engagement of the employee, and it negatively relates to employee retention. Considering both aspects, the study found that increasing work engagement is vital to curtail employee retention.
Leader support is an indirect factor in employee retention. According to the studies, employee engagement and work-life balance act as mediation for perceived supervisor support and employee turnover relationship [ 16 ]. The supervisor supports the career success of employees and it affects both directly and indirectly the career success of the employee and retention one year later [ 9 ]. Therefore, this study shows that co-worker support has a significantly positive impact on employee turnover. However, these results maintained the diversity of the sample. As this has been examined in India, a South Asian country, the same results can apply to the Sri Lankan context. Based on the above-mentioned literature, hypothesis seven has been developed; leader member exchange has a negative impact on employee turnover.
Co-worker support
Co-worker support will be in both formal and informal ways and in two different forms, emotional support, and instrumental support. The support of co-workers enhances the confidence level of the employee. Further, it helps to accept challenges in the work environment. Kmieciak [ 19 ] has worked on research to identify the effect of co-worker support on employee retention in the IT industry. However, a significantly negative impact was not evident on co-worker support. As this is a recently published research paper, the results are more valuable to the current research. The researcher has investigated more about the impact of subordinates’ support. Here, the analysis has been done only with 118 employees from a Polish software company. Considering the above limitations enables researchers to further study this topic with a larger sample size for generalisability of findings.
Abugre and Acquaah [ 39 ] researched in Ghana to identify the relationship between co-worker relationships and employee retention. The findings of this research imply that co-worker support is negatively associated with employee turnover. It further stated that cynicism of the employee is positively associated with employee turnover. The speciality of this research is identifying the importance of encouraging co-worker support rather than employee cynicism. These newly published research results can be used along with all other variables that affect employee turnover. According to the above literature, hypothesis eight has been constructed.
These studies have a common limitation in gathering more independent variables and analysing the impact. Therefore, a need exists to measure the effect of job satisfaction, work-life balance, happiness, management support, career management, innovative work behaviour, leader member exchange, and co-worker support together on employee turnover.
In Sri Lanka, no research has so far considered all eight factors affecting employee turnover in one study. With the above-mentioned literature findings, this study assists the government in identifying the impact of every factor on employee turnover in startups in Sri Lanka.
Data and methodology
This study was reviewed and approved by Sri Lanka Institute of Information Technology Business School and the Sri Lanka Institute of Information Technology ethical review board. Data were collected through a questionnaire using both online and manual channels. Each individual in this study gave verbal consent prior to the formal interview. The data was collected from August to September 2022 ( S1 Appendix ). The authors directly distributed the questionnaire. Moreover, authors could contact management in startups and distribute the questionnaire in their organisation. The questionnaire is composed of ten (10) sections. The first part of the questionnaire was designed to collect the demographic characteristics of the correspondents. The second to ninth sections focused on independent variables, job satisfaction, work-life balance, happiness, management support, career management, innovative work behaviour, leader member exchange, and co-worker support. Finally, the tenth section was designed to identify employee turnover indicator. A minimum of four questions was added under each indicator. The researchers facilitated anonymously answering all the questions in the questionnaire. The participants should be a part of startup and he/she should consider the behaviour and culture of that startup when answering the questions. All nine indicators were covered by Likert scale questions from 1 to 5 rating scale, depicting (1) strongly disagree to (5) strongly agree to collect respondents’ attitudes and opinions. Each respondent took about 10–15 minutes to complete answering the questionnaire and took approximately 5–7 minutes to fill out the questionnaire. Furthermore, the average values were calculated to measure the value given by respondents for each indicator. The data file used for the study is presented in S2 Appendix .
PricewaterhouseCoopers [ 15 ] statistics determined the study’s population and it explained the total number of elements to be focused on in this study. The researchers applied a random sampling method, mainly employees who are a part of or have been a part of the startup. This sampling technique was appropriate because it was free of bias. The sample size was selected by referencing the Krejcie and Morgan sampling table and Calculator.net [ 40 ] with a confidence level of 95% and 7% of margin of error. The calculation results indicated a minimum of 171 professionals. A stepwise ordered probit analysis method was used as the selected variables are widely used indicators for employee turnover; therefore, a micro-level analysis was required to study how these variables impact. A pilot survey was conducted to identify whether the purpose of the questions was clear to the respondents.
The data used for the estimation include 83 low employee turnover, 79 moderate employee turnover and 68 high employee turnovers of employees in Sri Lankan startups. The initial estimation results are presented in Table 1 .
Variable | Analytics sample (N = 230) | |
---|---|---|
(Means if numerical) | Standard deviations | |
Dependent variable—Employee Turnover (ET) | ||
Low | 36.09% | |
Moderate | 34.35% | |
High | 29.57% | |
Job Satisfaction (JS) | 3.8343 | 0.0639 |
Work-life Balance (WLB) | 4.0583 | 0.0642 |
Happiness (H) | 4.0017 | 0.0637 |
Management Support (MS) | 4.0235 | 0.0730 |
Career Management (CM) | 3.8989 | 0.0694 |
Innovative Work Behaviour (IWB) | 3.8565 | 0.0704 |
Leader Member Exchange (LMX) | 4.0924 | 0.0711 |
Co-Worker support (CWS) | 4.0913 | 0.0689 |
Male | 68.26% | |
Female | 31.74% | |
20–30 | 88.70% | |
31–40 | 7.39% | |
41–50 | 2.61% | |
Above 50 | 1.30% | |
Passed G.C.E. O/L or G.C.E. A/L or equivalent | 3.91% | |
Passed certificate or diploma level | 20.43% | |
Passed degree | 58.26% | |
Passed postgraduate | 17.39% | |
Full time | 93.04% | |
Part time | 6.96% | |
Ampara | 3.48% | |
Badulla | 0.87% | |
Colombo | 24.78% | |
Galle | 12.17% | |
Gampaha | 6.52% | |
Hambantota | 0.87% | |
Jaffna | 0.43% | |
Kalutara | 27.83% | |
Kandy | 1.30% | |
Kegalle | 0.43% | |
Kurunegala | 2.17% | |
Matale | 0.87% | |
Matara | 10.00% | |
Nuwara Eliya | 2.61% | |
Polonnaruwa | 0.43% | |
Puttalam | 1.30% | |
Rathnapura | 0.87% | |
Ratnapura | 2.17% | |
Trincomalee | 0.87% |
Source: Authors’ compilation based on survey data.
The mean values of all independent variables are greater than 2.5. Respondents were further grouped as per demographic and geographic characteristics. The respondents’ gender identity ratio is nearly 1: 2. When considering the age groups, most are in 20–30 years. Many employees in startup companies are in their twenties and are graduates. The respondents represent all the districts in Sri Lanka, most of which are from Kalutara, Colombo, Galle and Matara districts.
Research framework and hypothesis
The conceptual framework was developed with the literature review and existing knowledge, as illustrated in Fig 2 . This model was developed with the combination of eight hypotheses. These independent variables have been identified as critical factors that impact employee turnover.

Source: Authors’ compilation.
The following hypotheses have been developed in line with the research framework.
- Hypothesis 1 : Job satisfaction has a negative impact on employee turnover in startups in Sri Lanka.
- Hypothesis 2 : Work-life balance has a negative impact on employee turnover in startups in Sri Lanka.
- Hypothesis 3 : Happiness has a negative impact on employee turnover in startups in Sri Lanka.
- Hypothesis 4 : Management support has a negative impact on employee turnover in startups in Sri Lanka.
- Hypothesis 5 : Career management has a negative impact on employee turnover in startups in Sri Lanka.
- Hypothesis 6 : Innovative work behaviour has an impact on employee turnover in startups in Sri Lanka.
- Hypothesis 7 : Leader member exchange has an impact on employee turnover in startups in Sri Lanka.
- Hypothesis 8 : Co-worker support has a negative impact on employee turnover in startups in Sri Lanka.
Methodology
This study focuses on the demographical variables that affect employee turnover. For this, the present study’s authors considered employee feedback concerning Sri Lankan startups. The ordered probit regression determines the significant variables [ 41 ]. The probit model is an estimation technique for equations with dummy dependent variables that avoids the unboundedness problem of the linear probability model by using a variant of the cumulative normal distribution [ 42 ]. Further, this study examines the likelihood of three types of employee turnover. Accordingly, employee turnover is divided into three categories, considering the equality of data for each category based on employee turnover.
- Group 1 (y = 1): low = mean value of the employee turnover less than 1.50
- Group 2 (y = 2): moderate = mean value of the employee turnover greater than 1.5 and less than or equal to 2.25
- Group 3 (y = 3): high = mean value of the employee turnover greater than 2.25 and less than or equal to 5
The following equation represents the general form of the ordered probit model.
The y i value represents i th value of the dependent variable, employee turnover and x i represents the i th common independent variable. The β value is a vector parameter and ℇ i considered as the normally distributed random error term with a zero mean. The following ordered probit model has been developed by detailing the general equation.
Table 2 indicates the variables explained in previous literature and definitions of the previously mentioned equation that affects employee retention. The forward stepwise regression model has been used to analyse the data set.
Variable | Description | Expected sign(s) |
---|---|---|
Dummy variable to capture the turnover of employees in startups where low is denoted by 1, moderate as 2 and high as 3. | (-) | |
Job satisfaction. Five-point Likert scale variable with extremes “rarely– 1” to “always– 5” will be used. | (-) | |
Five-point Likert scale variable with extremes “rarely– 1” to “always– 5” to measure work-life balance of employees. | (-) | |
Employee’s happiness. Five-point Likert scale variable with extremes “rarely– 1” to “always– 5” will be used. | (-) | |
Five-point Likert scale variable with extremes “rarely– 1” to “always– 5” to measure management support to the employee. | (-) | |
Career management. Five-point Likert scale variable with extremes “rarely– 1” to “always– 5” will be used. | (-) | |
Five-point Likert scale variable with extremes “rarely– 1” to “always– 5” to measure innovative work behaviour of the employee. | (+/-) | |
Leader member exchange. Five-point Likert scale variable with extremes “rarely– 1” to “always– 5” will be used. | (+/-) | |
Five-point Likert scale variable with extremes “rarely– 1” to “always– 5” to measure employee’s co-worker support. | (-) |
Results and discussions
It is mandatory to test the internal consistency reliability before data analysis. The most common measure of reliability is Cronbach’s alpha (α) value, which determines whether the internal instruments are constant [ 43 ]. The reliability results for each indicator are presented in Table 3 . As all the Cronbach alpha values are greater than 0.6 scale reliability coefficients, all variables in this study are acceptable.
Item | Number of items | Average interitem covariance | Scale reliability coefficient (Cronbach alpha for dimensions) |
---|---|---|---|
9 | 0.7698 | 0.9647 | |
2 | 0.7951 | 0.9145 | |
2 | 0.7444 | 0.8835 | |
2 | 0.9076 | 0.9134 | |
2 | 0.9840 | 0.9148 | |
2 | 0.9740 | 0.9284 | |
2 | 0.8397 | 0.8433 | |
2 | 0.9228 | 0.8997 | |
2 | 0.7654 | 0.8593 |
Analytical sample (N = 230)
Source: Authors’ calculation based on survey data
In the first step, the initial ordered probit model was executed, and this model explained 73% of the variation in employee retention by the variation in independent variables. S3 Appendix contains the table of the initial ordered probit regression model. The ordered probit model forwarded with the forward stepwise technique to identify the exact number of variables that impact employee turnover. A forward stepwise technique was adopted for the variable selection in each specification. Here, the new variables for selection were considered with a p-value < 0.20 and the previously selected variable for removal with a p-value ≥ 0.25. Three different model diagnostic criteria were considered in assessing the reliability of the results. The forward stepwise methodology suggested that the significance of the existing variables could be increased by adding more variables to the model. Marginal effects were separately calculated for low, moderate, and high employee turnover. Table 4 presents the final estimation results of the ordered probit model and illustrates the substantive effects of the independent variables. Here, 71.74% of the variation in employee turnover is explained by the variation in job satisfaction, LMX and co-worker support, considering the sample size and independent variables.
Marginal effects (in percentages) | |||||
---|---|---|---|---|---|
Variable | Estimate | Robust SE | Low ET ( ) | Moderate ET ( ) | High ET ( ) |
ln | -1.3829 | 0.6500 | 0.4716 | -0.0387 | -0.4329 |
ln | -0.8552 | 0.4856 | 0.2917 | 0-.0239 | -0.2677 |
ln | 1.3808 | 0.4127 | -0.4709 | 0.0386 | 0.4323 |
ln | -1.1872 | 0.4264 | 0.4049 | -0.0332 | -0.3717 |
ln | -0.6897 | 0. 4915 | 0.2352 | -0.0193 | -0.2159 |
G_Male | 0.4440 | 0.1723 | -0.1569 | 0.0264 | 0.1305 |
A_20_30 | 0.8527 | 0.5439 | -0.3220 | 0.1209 | 0.2011 |
A_31_40 | 1.6657 | 0.6027 | -0.3133 | -0.2817 | 0.5950 |
-2.9373 | 0.6738 | 0.2877 | 0.4691 | 0.2431 | |
-1.6810 | 0.6571 | ||||
Pseudo R | 0.7174 | ||||
Log likelihood | -197.1130 | ||||
Number of observations | 230 |
*** significant at the 1% level
** significant at the 5% level and * significant at the 10% level.
Source: Authors’ calculation based on surveying data.
Looking at the signs of the marginal effects in Table 4 , overall, high employee turnover is negatively associated with job satisfaction, co-worker support, and innovative work behaviour, whereas high employee turnover is positively associated with leader member exchange.
To control for the potential effect on different levels of employee turnover, the age factor was also included in the model, the coefficient of which implies that high employee turnover is 0.20 points and 0.60 points for the 20–30 years age range and 31–40 years age range, respectively. Employee turnover in 31–40 years age range employees is higher than that of other age ranges.
The marginal effects of the psychographic variables reveal that a 1% increase in job satisfaction increases the probability of low employee turnover by 0.47 percentage points. Similarly, 1% increase in job satisfaction decreases the probability for high employee turnover by 0.43 percentage points. With this observation, it can be stated that improving job satisfaction will highly affect to reduce high employee turnover. These results verify the existing statements indicating that job satisfaction has the highest significant and negative estimate value.
The estimated marginal effect of low employee turnover is 0.47 percentage points higher for employees in Sri Lankan Startups with a 1% increase in leader member exchange. High employee turnover is associated with leader member exchange increasing probability by 0.43. However, this study reflects similar findings to those of Tymon, Stumpf [ 9 ]. The reason behind the positive relation is employees learn fast and get qualified with the support of their leaders and then quit the company within the next few years.
Both leader member exchange and co-worker support are significant at the 99% level of employee turnover in the Sri Lankan context. When considering the independent variables for employee turnover in startups in Sri Lanka, co-worker support is a critical factor in determining the level of employee turnover. The 1% increase in co-worker support will also increase the probability of low employee turnover by 0.40 percentage points. But concurrently, change in co-worker support will negatively impact high employee turnover. The results ensure that encouraging co-worker support is crucial rather than employee cynicism.
Innovative work behaviour is one of the most critical factors in employee turnover. With a 1% increase in innovative work behaviour, the estimated marginal effect of high employee turnover is 0.27 percentage points lower for employees in Sri Lankan startups. The results of Shih, Posthuma [ 35 ] indicate a positive relationship exists between innovative work behaviour and employee turnover. However, this study concludes by emphasising the importance of retaining the innovative employees to remain competitive in the industry. For this, startups need to improve and enhance employees’ innovative behaviour and, concurrently, to prevent such employee retention.
Entrepreneurs are the founders of startups. Employees’ entrepreneurial dreams positively affect employee intention to startups. Employees in the startups also will have an ideation to start their own business. According to the study by Li, Li [ 44 ] the mediating role of employees’ entrepreneurial self-efficacy and the moderating role of job embeddedness in the influence of entrepreneurial dreams on employees’ turnover intention to startup.
The main objective of this research is to analyse the impact of critical and newly identified factors on employee turnover in one study. This issue occurs when employees leave the company by giving short notice or quitting unexpectedly. The analysis found that gender and age impact employee turnover in startups in Sri Lanka. In startups, many employees are in the 20 to 30 years age range. Employees between 31 and 40 years show a higher tendency to leave the startups. In Sri Lanka, only 8% of startups have been in operation for more than five years [ 15 ], indicating that the businesses are not stabilised and are still in its early stages. To prevent employee turnover, startups must improve employee job satisfaction. As per the findings, increasing job satisfaction has a significant impact on reducing employee turnover. For most employees in startups, it is their first job. During this time, employees gain work experience and become experts in the field. The leaders allocate much time to train their human resources and the company should gain strategic benefits from this investment. The results of the study prove that leader member exchange has a positive impact on employee turnover, as verified by Tymon, Stumpf [ 9 ] too about this relationship. To overcome this situation, as managers, it is vital to discuss with employees about their career paths, employee interests and company’s business plans while improving their technical skills and experience. This way, the mutual interest of both the employee and the company can be identified and handled. It also builds trust between the company and the employees. Regular support environment and ease of doing business is 66% highly important factor for the success of Sri Lankan startups [ 15 ]. This environment can be easily created with the level of co-worker support to the employee. Employee turnover can be more costly than a startup can imagine, with disruptions to business operations when their employees’ suddenly quit jobs. Therefore, it is must to attain above discussed facts. These results and discussions can be taken as insights to better understand and curtail employee turnover. This study will assist Sri Lankan startups where their skilled employees, who are also experts plausibly remain, enabling the businesses to expand to new markets. Usually, issues relevant to profit-making and business performance, such as a drop in sales and manufacturing are identified by startups. However, employee turnover is generally not identified as an organisational issue.
Theoretical implications
The current study empirically investigated the impact of job satisfaction, innovative work behaviour, co-worker support and leader member exchange on employee turnover. According to the authors’ knowledge, no prior studies were conducted considering the combined impact of all the independent variables on employee turnover. Therefore, this study strengthens the literature by demonstrating how job satisfaction, innovative work behaviour, co-worker support and leader member exchange impact employee turnover in Sri Lankan startups.
The findings reveal that job satisfaction has a negative impact on employee turnover. This finding is consistent with the previous study, job satisfaction significantly predicted employee turnover [ 6 ]. This study consolidates past findings that male employees have higher turnover intention than female employees. Female employees have comparatively higher-level job satisfaction [ 8 ]. This study implies that employees age 31 to 40 years have high employee turnover intention. The research findings are similar to Lu, Lu [ 8 ]; the older employees have high intentions to leave the company.
Practical implications
The study’s findings illustrate the importance of job satisfaction, innovative work behaviour, co-worker support and leader member exchange in affecting employee turnover in startups. This study provides managerial insights on lowering employee turnover in Sri Lankan startups. First, startups need to be aware that experienced employees in startups can be easily taken by well-established companies because, later, they have hand on experience and skills. Therefore, it is important to implement strategies for a solid career development plan, career growth, personal status, and employee recognition. As job satisfaction can predict employee turnover, it is a must to measure those indicators and maintain a favourable level at all times.
Innovative work behaviour is increasingly becoming a significant factor in employee retention. As good startups are a blend of creativity and competitive advantage, it is a must to focus on the IWB of the employee. LMX is a turning point for expanding the business. More importantly, healthy LMX can boost employees’ work engagement. This healthy level can maintain by conducting regular meetings, training programs and informal mentorship with employees’ immediate supervisors [ 8 ]. Further, management can allow employees at all levels to present their fresh ideas and incorporate them to influence organisation’s decision making process. These processes can lower employee hierarchy and build strong relationships while recognising them in the company.
It is important to retain trained and skilled employees who started their career paths in the organisation. Such employees can drive the organisation to success. While measuring employees’ job satisfaction, managers nee to conduct standard ways on performance and improvements of the organisation. It is better if companies can create their key performance indicators because it will help protect the organisation’s core values while expanding the company. Furthermore, having a flexible approach to work in an organisation culture will increase the trust between employees and the organisation. Giving the freedom to take risks and not allowing them to feel alone during work will give value to employees. Finally, all the above actions will strongly impact reducing employee intention to leave the organisation.
Research limitations and future research directions
Further research can improve the study as follows. First, this research includes feedback from 230 employees. More than one-third of these employees are from the IT industry. Since Sri Lankan startups are technology-driven, this ratio is more reliable. However, this research can be generalised by obtaining employees’ feedback from other industries. Secondly, in this questionnaire, the minimum number of questions for independent factors is four. This is to minimise the possibility of demotivating the employee by giving a lengthy and complex questionnaire. Therefore, in future, researchers can design questionnaires incorporating more questions to cover a wider range of independent factors, including open-ended ones. Thirdly, in this sample, many employees were in their twenties, and most hadn’t worked for more than two companies (i.e. employers). As such, it is reasonable to assume that participants’ response is somewhat limited to obtain the broader picture of the research problem. Future researchers can focus on different age groups and analyse the same factors concerning employee retention. Finally, new research can be executed by adopting a case study approach (including case studies representing various types of industries etc), such as employees in multinational companies.
Supporting information
S1 appendix, s2 appendix, s3 appendix, s4 appendix, acknowledgments.
The authors would like to thank Ms. Gayendri Karunarathne for proof-reading and editing this manuscript.
Funding Statement
The authors received no specific funding for this work.
Data Availability
- PLoS One. 2023; 18(2): e0281729.
Decision Letter 0
21 Dec 2022
PONE-D-22-30684How are employee turnover intentions created in Sri Lankan Startups?PLOS ONE
Dear Dr. Ruwan Jayathilaka,
Thank you for submitting your manuscript to PLOS ONE. After careful consideration, we feel that it has merit but does not fully meet PLOS ONE’s publication criteria as it currently stands. Therefore, we invite you to submit a revised version of the manuscript that addresses the points raised during the review process.
==============================Reviewer#1Abstract: Rewrite the abstract after manuscript correction and provide picture of whole study.
In the first paragraph of introduction used only this (2016) citation. This citation not justify the paragraph.
Introduction paragraph is not justifying the problem and bag-round of study. Revised the introduction and use the recent citations to justify and logically make connection with them.
In the introduction (second paragraph) , the contribution of study is confused with variable relationships; why are these relationships a contribution of study? Need strong justification.
Overall, I suggest a major rewrite of the introduction. It should provide an overview of and focus on one issue with recent citations.
Revised all literature variables and link with variables with new citation
In the literature, justify these hypotheses with literary support.
In literature, justify the conceptual model and theoretical gap.
Where is the total population? How did you choose the sample size? And how did you choose which method, unit of analysis, and research technique to use? Provide justification. Why is this method appropriate for this data set?
General: identifying flaws in the study's design (revised methodology section) and justifying technique
Write the theoretical contribution related to a model. Reviewer#2
Mention the scope of the study, the population, simple size, data collected from…..
Mention the analysis technique/ tool used in the study
Introduction:
The introduction is not clear and very less literature is used. Follow these instruction: The introduction should briefly place the study in a broad context and highlight why it is important. It should define the purpose of the work and its significance.
The current state of the research field should be reviewed carefully and key publications cited. Briefly mention the main aim of the work and highlight the main conclusions. Keep the introduction comprehensible to scientists working outside the topic of the paper.
What is the main research focus?? Firm performance? Employee retention? Employee turnover intention?
Focus more on the main issue of the study
Make the theoretical and practical gaps more clear ?
Why Sri Lanka?
Why stratup s in Sri Lanka? Why employee working in startups in Sri Lanka
What was the key motivation behind focusing on factors affecting employee turnover intention in stratups in Srilanka?
Please, properly justify why the selected variables are included in the model. How did you derive the 08 variables ??
As ,many studies conducted in the world and in Sri Lanka about this topic, what us the main contribution of your study?
The paper should incorporate a more solid argumentation that allows to justify the reason that allows to select the explanatory variables that are considered in the empirical analysis.
Literature and hypotheses development"
Improve the argumentation of hypothesis. Whether, the hypotheses are formulated separately or after the literature review of each section, it should be properly argued.
Each hypothesis should be formulated at the end of a literature section of the each variable presenting the different findings that have been made throughout the literature. With these arguments a reasoning should be developed in a certain direction and the conclusion of that reasoning should be the formulated hypothesis. In the current version of this manuscript the authors are including different aspects of previous literature, but it does not exist any convincing storyline in any direction.
Highlight controversial and diverging hypotheses when necessary.
Researcher should include a summary table / review on studies conducted on Employee Turnover Intention in Sri Lanka to support the literature and arguments.
Below papers has some interesting implications and understanding of concepts and relations that you could discuss in your introduction and literature review and how it relates to your work:
-Li, M., Li, J., Chen, X. “Employees’ Entrepreneurial Dreams and Turnover Intention to Start-Up: The Moderating Role of Job Embeddedness”, 2022, International Journal of Environmental Research and Public Health 19(15),9360
- Saoula, O.,Johari, H, “The mediating effect of organizational citizenship behavior on the relationship between perceived organizational support and turnover intention: A proposed framework” International Review of Management and Marketing, 2016, 6(7), pp. 345–354
- Saoula, O., Johari, H., Bhatti, M.A “The mediating effect of organizational citizenship behaviour on the relationship between personality traits (Big Five) and turnover intention: A proposed framework”, International Business Management, 2016, 10(20), pp. 4755–4766.
Zito, M., Emanuel, F., Molino, M., Cortese, C. G., Ghislieri, C., & Colombo, L. (2018). Turnover intentions in a call center: The role of emotional dissonance, job resources, and job satisfaction. PloS one, 13(2), e0192126.
- Saoula, O., Johari, H., Fareed, M, “A conceptualization of the role of organisational learning culture and organisational citizenship behaviour in reducing turnover intention”, Journal of Business and Retail Management Research, 2018, 12(4), pp. 126–133
- Saoula, O., Fareed, M., Ismail, S.A., Husin, N.S., Hamid, R.A, “A conceptualization of the effect of organisational justice on turnover intention: The mediating role of organisational citizenship behaviour”, International Journal of Financial Research, 2019, 10(5), pp. 327–337.
Poku, C. A., Alem, J. N., Poku, R. O., Osei, S. A., Amoah, E. O., & Ofei, A. M. A. (2022). Quality of work-life and turnover intentions among the Ghanaian nursing workforce: A multicentre study. PloS one, 17(9), e0272597.
- Saoula, O., Fareed, M., Hamid, R.A., Al-Rejal, H.M.E.A., Ismail, S.A, “The moderating role of job embeddedness on the effect of organisational justice and organisational learning culture on turnover intention: A conceptual review”, Humanities and Social Sciences Reviews, 2019, 7(2), pp. 563–571
-Li, Q., Mohamed, R., Mahomed, A., & Khan, H. (2022). The Effect of Perceived Organizational Support and Employee Care on Turnover Intention and Work Engagement: A Mediated Moderation Model Using Age in the Post Pandemic Period. Sustainability, 14(15), 9125.
- Amin, M., Othman, S.Z., Saoula, O, “The Effect of Organizational Justice and Job Embeddedness on Turnover Intention in Textile Sector of Pakistan: The Mediating Role of Work Engagement” Central Asia and the Caucasus, 2021, 22(5), pp. 930–950
Methodology:
How experiment was conducted?
How participants were recruited?
What are the instructions of experiment?
How much was time given to each participant?
What are the theoretical implications of the study ?
Practical implications needs further discussion.
Add/ involve more recent citations/studies where necessary
Please submit your revised manuscript by Feb 04 2023 11:59PM. If you will need more time than this to complete your revisions, please reply to this message or contact the journal office at gro.solp@enosolp . When you're ready to submit your revision, log on to https://www.editorialmanager.com/pone/ and select the 'Submissions Needing Revision' folder to locate your manuscript file.
Please include the following items when submitting your revised manuscript:
- A rebuttal letter that responds to each point raised by the academic editor and reviewer(s). You should upload this letter as a separate file labeled 'Response to Reviewers'.
- A marked-up copy of your manuscript that highlights changes made to the original version. You should upload this as a separate file labeled 'Revised Manuscript with Track Changes'.
- An unmarked version of your revised paper without tracked changes. You should upload this as a separate file labeled 'Manuscript'.
If you would like to make changes to your financial disclosure, please include your updated statement in your cover letter. Guidelines for resubmitting your figure files are available below the reviewer comments at the end of this letter.
If applicable, we recommend that you deposit your laboratory protocols in protocols.io to enhance the reproducibility of your results. Protocols.io assigns your protocol its own identifier (DOI) so that it can be cited independently in the future. For instructions see: https://journals.plos.org/plosone/s/submission-guidelines#loc-laboratory-protocols . Additionally, PLOS ONE offers an option for publishing peer-reviewed Lab Protocol articles, which describe protocols hosted on protocols.io. Read more information on sharing protocols at https://plos.org/protocols?utm_medium=editorial-email&utm_source=authorletters&utm_campaign=protocols .
We look forward to receiving your revised manuscript.
Kind regards,
Muhammad Fareed, Ph.D
Academic Editor
Journal Requirements:
When submitting your revision, we need you to address these additional requirements.
1. Please ensure that your manuscript meets PLOS ONE's style requirements, including those for file naming. The PLOS ONE style templates can be found at
https://journals.plos.org/plosone/s/file?id=wjVg/PLOSOne_formatting_sample_main_body.pdf and
https://journals.plos.org/plosone/s/file?id=ba62/PLOSOne_formatting_sample_title_authors_affiliations.pdf
2. In the ethics statement in the Methods, you have specified that verbal consent was obtained. Please provide additional details regarding how this consent was documented and witnessed, and state whether this was approved by the IRB.
3. Please include your full ethics statement in the ‘Methods’ section of your manuscript file. In your statement, please include the full name of the IRB or ethics committee who approved or waived your study, as well as whether or not you obtained informed written or verbal consent. If consent was waived for your study, please include this information in your statement as well.
Additional Editor Comments:
The paper is generally well written and structured. However, I believe that paper has some shortcomings in terms of
Abstract: Rewrite the abstract after manuscript correction and provide picture of whole study.
In the introduction (second paragraph) , the contribution of study is confused with variable relationships; why are these relationships a contribution of study? Need strong justification.
�Overall, I suggest a major rewrite of the introduction. It should provide an overview of and focus on one issue with recent citations.
�Write the theoretical contribution related to a model.
[Note: HTML markup is below. Please do not edit.]
Reviewers' comments:
Reviewer's Responses to Questions
Comments to the Author
1. Is the manuscript technically sound, and do the data support the conclusions?
The manuscript must describe a technically sound piece of scientific research with data that supports the conclusions. Experiments must have been conducted rigorously, with appropriate controls, replication, and sample sizes. The conclusions must be drawn appropriately based on the data presented.
Reviewer #1: Partly
Reviewer #2: Partly
2. Has the statistical analysis been performed appropriately and rigorously?
Reviewer #1: Yes
Reviewer #2: I Don't Know
3. Have the authors made all data underlying the findings in their manuscript fully available?
The PLOS Data policy requires authors to make all data underlying the findings described in their manuscript fully available without restriction, with rare exception (please refer to the Data Availability Statement in the manuscript PDF file). The data should be provided as part of the manuscript or its supporting information, or deposited to a public repository. For example, in addition to summary statistics, the data points behind means, medians and variance measures should be available. If there are restrictions on publicly sharing data—e.g. participant privacy or use of data from a third party—those must be specified.
Reviewer #2: No
4. Is the manuscript presented in an intelligible fashion and written in standard English?
PLOS ONE does not copyedit accepted manuscripts, so the language in submitted articles must be clear, correct, and unambiguous. Any typographical or grammatical errors should be corrected at revision, so please note any specific errors here.
Reviewer #2: Yes
5. Review Comments to the Author
Please use the space provided to explain your answers to the questions above. You may also include additional comments for the author, including concerns about dual publication, research ethics, or publication ethics. (Please upload your review as an attachment if it exceeds 20,000 characters)
Reviewer #1: Abstract:
Reviewer #2: Revised topic after correction
The paper is generally well written and structured. However, I believe that paper has some shortcomings in terms of
6. PLOS authors have the option to publish the peer review history of their article ( what does this mean? ). If published, this will include your full peer review and any attached files.
If you choose “no”, your identity will remain anonymous but your review may still be made public.
Do you want your identity to be public for this peer review? For information about this choice, including consent withdrawal, please see our Privacy Policy .
Reviewer #1: Yes: Oussama Saoula
Reviewer #2: Yes: Munwar Hussain Pahi
[NOTE: If reviewer comments were submitted as an attachment file, they will be attached to this email and accessible via the submission site. Please log into your account, locate the manuscript record, and check for the action link "View Attachments". If this link does not appear, there are no attachment files.]
While revising your submission, please upload your figure files to the Preflight Analysis and Conversion Engine (PACE) digital diagnostic tool, https://pacev2.apexcovantage.com/ . PACE helps ensure that figures meet PLOS requirements. To use PACE, you must first register as a user. Registration is free. Then, login and navigate to the UPLOAD tab, where you will find detailed instructions on how to use the tool. If you encounter any issues or have any questions when using PACE, please email PLOS at gro.solp@serugif . Please note that Supporting Information files do not need this step.
Author response to Decision Letter 0
16 Jan 2023
Point by point response to editor and reviewers
Dear editor and the reviewers,
We would like to express our profound appreciation to the editor and the reviewers for the valuable comments and suggestions made on our manuscript which were very helpful in revising and improving it.
Please note that the line numbers referred in this document is aligned with the revised manuscript which has track changes.
Reviewer 1 Comment 1: Abstract: Mention the scope of the study, the population, simple size, data collected from…
Authors’ Response: Thank you very much for the valuable comment. The suggestions have been incorporated in the revised manuscript from lines 30 to 32.
“…The study population was professionals who have been a key part of Sri Lankan startups, which involved 230 respondents. …”
Reviewer 1 Comment 2: Abstract: Mention the analysis technique/ tool used in the study
Authors’ Response: Thank you very much. Your comment is well noted. The analysis technique was added in the abstract of the revised manuscript from lines 32 to 33.
“…Data analysis was performed through a forward stepwise technique through STATA …”
Reviewer 1 Comment 3: Introduction: The introduction is not clear and very less literature is used. Follow these instructions: The introduction should briefly place the study in a broad context and highlight why it is important. It should define the purpose of the work and its significance.
Authors’ Response: Thank you very much for the detailed comment. This helps to strength the introduction with recent literatures, better argument, and justifications. The following literature were added in the introduction section with citation nos. 10, 11, 1, and 3 of the revised manuscript.
Reviewer 1 Comment 4: Introduction: The current state of the research field should be reviewed carefully, and key publications cited. Briefly mention the main aim of the work and highlight the main conclusions. Keep the introduction comprehensible to scientists working outside the topic of the paper.
Authors’ Response: Well noted your comment. A major update has done in the introduction section of the study.
Reviewer 1 Comment 5: Introduction: What is the main research focus?? Firm performance? Employee retention? Employee turnover intention?
Authors’ Response: Thank you very much for your comment. This study focuses/ aims to analyse the impact of job satisfaction, happiness, work-life balance, career management, management support, innovative work behaviour, leader-member-exchange, and co-worker support on employee turnover in startups in Sri Lanka. New paragraph has been added in the revised manuscript to explain more about firm performance, employee retention, employee turnover intention from lines 74 to 80.
“Firm performance reflects the ability of an organisation to use its human resources and other material resources to achieve its goals and objectives. Firm performance belongs to the economic category, and it should consider the use of business means efficiently during the production and consumption process [12]. Employee retention is defined as encouraging employees to remain in the organisation for a long period or the organisation’s ability to minimised employee turnover [13]. Turnover intention is the intention of the employee to change the job or organisation voluntarily [14].”
Reviewer 1 Comment 6: Introduction: Focus more on the main issue of the study
Authors’ Response: Thank you for your valuable comment. More priority was given to discuss the main issue of the study. In the first paragraph of the introduction section have updated to highlight the main issue with latest literature. New content has been added from lines 55 to 58.
“…The issue of employee turnover is considered as one of the global obstacles for organisations worldwide, which directly and adversely affects strategic plans and opportunities of gaining competitive advantages [3].…”
Reviewer 1 Comment 7: Introduction: Make the theoretical and practical gaps more clear
Authors’ Response: Thank you very much for the comment. The revised manuscript has been updated by pointing out the existing research gaps. New content has been added from lines 128 to 131.
“…to the best of the authors’ knowledge, there was no previous research done by local researchers that includes all the widely measured variables investigating the combined effect on employee turnover…”
Reviewer 1 Comment 8: Introduction: Why Sri Lanka?
Authors’ Response: Thank you for the comment. Sri Lanka has selected as the case study because to the best of the authors’ knowledge, no any previous research has been done by local researchers considering all the widely affected eight variables together. It leads to improve the introduction part of the paper. Suggestions have been incorporated in the revised manuscript from lines 127 to 130.
“…Sri Lankan context has been selected as the case study. This is because, to the best of the authors’ knowledge, there was no previous research done by local researchers that includes all the widely measured variables investigating the combined effect on employee turnover…”
Reviewer 1 Comment 9: Introduction: Why stratup s in Sri Lanka? Why employee working in startups in Sri Lanka
Authors’ Response: Thank you very much for the comment and this is well noted. Suggestions have been incorporated in the revised manuscript from lines 85 to 89.
“…Sri Lanka has a middle rank of ease of doing business. With these favourable conditions and educational and family backgrounds, many people like to operate/apply their new idea and fill the market gap. The new generation in Sri Lanka are interested/are keen on innovations at work and being a part of unique products or services…”
Reviewer 1 Comment 10: Introduction: What was the key motivation behind focusing on factors affecting employee turnover intention in stratups in Sri Lanka?
Authors’ Response: Thank you very much for the valuable comment. The key motivation of focusing on factors affecting employee turnover intention was to gather widely affected factors together and measure the impact of each indicator at the micro level. The idea was added in revised manuscript from line 110 to 111.
“Based on their knowledge and the existing literature, authors have considered widely used factors to investigate the employee turnover issue …”
Reviewer 1 Comment 11: Introduction: Please, properly justify why the selected variables are included in the model. How did you derive the 08 variables?
Authors’ Response: Thank you for the comment. According to the past literature authors have selected widely used indicators for employee turnover and among these eight variables have been selected. The justification has included in the revised manuscript from line 110 to 117.
“Based on their knowledge and the existing literature, authors have considered widely used factors to investigate the employee turnover issue. Therefore, job satisfaction, happiness, work-life balance, career management, management support, innovative work behaviour, leader member exchange and co-worker support were selected based on previous literature findings [4-6, 8, 17-19]. As in the previous papers and along with the current study’s results, authors identified both positive and negative impacts on employee turnover among Sri Lankan startups..”
Reviewer 1 Comment 12: Introduction: As, many studies conducted in the world and in Sri Lanka about this topic, what us the main contribution of your study?
Authors’ Response: Well noted your comment. Thank you! The contribution of the study has highlighted in the revised manuscript from lines 120 to 133.
“…The present study’s scientific value can be elaborated by comparing it with previous studies. This study’s contribution can be explained in five ways. Firstly, the most critical and newly considered factors were identified together with the support of past literature. Secondly, the present study was divided/classified into different levels of employee turnover. As such, by y considering the various levels, the micro-level changes, and probabilities of the impact on employee turnover can be better identified. Further, this study helps to reduce the methodological gap. Thirdly, the Sri Lankan context has been selected as the case study. This is because, to the best of the authors’ knowledge, there was no previous research done by local researchers that includes all the widely measured variables investigating the combined effect on employee turnover. Fourthly, the analysis results can be used to identify the strengths and weaknesses of startups in Sri Lanka. Finally, this study identifies the challenges faced by startups and identifies how policy modifications can strengthen the startup ecosystem.”
Reviewer 1 Comment 13: Introduction: The paper should incorporate a more solid argumentation that allows to justify the reason that allows to select the explanatory variables that are considered in the empirical analysis.
Authors’ Response: Well noted your comment. Thank you! In the revised manuscript a paragraph was added to present the justification to select the variables in the empirical analysis from lines 110 to 117.
“Based on their knowledge and the existing literature, authors have considered widely used factors to investigate the employee turnover issue. Therefore, job satisfaction, happiness, work-life balance, career management, management support, innovative work behaviour, leader member exchange and co-worker support were selected based on previous literature findings [4-6, 8, 17-19]. As in the previous papers and along with the current study’s results, authors identified both positive and negative impacts on employee turnover among Sri Lankan startup.”
Reviewer 1 Comment 14: Literature and hypotheses development: Improve the argumentation of hypothesis. Whether the hypotheses are formulated separately or after the literature review of each section, it should be properly argued.
Authors’ Response: Thank you very much for your comment. The paper has been updated with the improved argument in literature review. The hypotheses have been formulated at the end of each sub section of literature review. New contents have incorporated as per the below line numbers.
(Line numbers 238 and 240)
“As per the literature, job satisfaction is an important factor in determining the impact on employee turnover. Accordingly, hypothesis one has been developed.”
(Line numbers from 283 to 284)
“…According to the above literature, hypothesis two has been constructed; work-life balance has a negative impact on employee turnover.”
(Line numbers from 306 to 308)
“…Based on the above cited literature, hypothesis three can be developed; employee happiness has a negative impact on employee turnover.”
(Line numbers from 336 to 337)
“…Hypothesis four has been developed based on above discussed literature.”
(Line numbers from 356 to 357)
“…Hypothesis five has been developed by concluding the above explained literature.”
(Line numbers 378 and 379)
“…With the presence of the above-mentioned literature, hypothesis six has been formulated.”
(Line numbers from 397 to 399)
“…Based on the above-mentioned literature, hypothesis seven has been developed; leader member exchange has a negative impact on employee turnover.”
(Line numbers from 417 to 419)
“…These newly published research results can be used along with all other variables that affect employee turnover. According to the above literature, hypothesis eight has been constructed.”
Reviewer 1 Comment 15: Literature and hypotheses development: Each hypothesis should be formulated at the end of a literature section of each variable presenting the different findings that have been made throughout the literature. With these arguments a reasoning should be developed in a certain direction and the conclusion of that reasoning should be the formulated hypothesis. In the current version of this manuscript the authors are including different aspects of previous literature, but it does not exist any convincing storyline in any direction.
Authors’ Response: Thank you very much for this detailed comment. The revised version has strengthened the formulation of hypothesis. Hypothesises formulations has been incorporated at the end of each sub section in the literature review and the storyline has been built. New contents have been incorporated as per the below line numbers.
Reviewer 1 Comment 16: Literature and hypotheses development: Highlight controversial and diverging hypotheses when necessary.
Authors’ Response: Thank you for your valuable comment. This leads to build a discussion in literature review independent variables sub section. New contents have been included as per the below line numbers.
(Line numbers from 187 to 191)
“…Moreover, the descriptive statistics found a high level of job satisfaction and the intention to leave was at the mid or average level of the scale. Camara further stated that job satisfaction clearly implies the feeling about their job. But some research findings can be contradictory. Some employees are fully satisfied with the job and still want to leave the organisation for various reasons…”
(Line numbers from 205 to 207)
“…However, they didn’t observe any significant interaction between overall work-life balance and job satisfaction in predicting employee turnover intention. With these results, this indicator must be examined further.”
(Line numbers from 414 to 415)
“…It further stated that cynicism of the employee is positively associated with employee turnover…”
(Line numbers from 405 to 406)
“…However, a significantly negative impact was not evident on co-worker support.…”
Reviewer 1 Comment 17: Literature and hypotheses development: Researcher should include a summary table / review on studies conducted on Employee Turnover Intention in Sri Lanka to support the literature and arguments
Authors’ Response: Thank you very much for your comment, Literature summary table was added as an appendix, and it was cited in the revised manuscript from line numbers 168 to 169.
“Appendix S4 contains the literature summary of the above presented literature search flow diagram. The following sections present the details of each category.”
Reviewer 1 Comment 18: Literature and hypotheses development: Below papers has some interesting implications and understanding of concepts and relations that you could discuss in your introduction and literature review and how it relates to your work:
- Zito, M., Emanuel, F., Molino, M., Cortese, C. G., Ghislieri, C., & Colombo, L. (2018). Turnover intentions in a call center: The role of emotional dissonance, job resources, and job satisfaction. PloS one, 13(2), e0192126.
- Poku, C. A., Alem, J. N., Poku, R. O., Osei, S. A., Amoah, E. O., & Ofei, A. M. A. (2022). Quality of work-life and turnover intentions among the Ghanaian nursing workforce: A multicentre study. PloS one, 17(9), e0272597.
Authors’ Response: Thank you very much for the detailed comment and sharing the latest literature related to this paper. New literature has been incorporated in the introduction, literature review and results and discussion sections the paper as per the below line numbers.
(Line numbers from 45 to 46)
“…Companies need to give high priority to employee development and predict employee behaviour [1]…”
(Line numbers from 55 to 58)
“…The issue of employee turnover is considered as one of the global obstacles for organisations worldwide, which directly and adversely affects strategic plans and opportunities of gaining competitive advantages [3]…”
(Line numbers from 66 to 68)
“…Further, promoting employee well-being leads to decrease employee turnover [10]. Providing psychological and social support through counselling promotes the quality of work-life [11]...”
(Line numbers from 318 to 325)
“A cross-sectional survey has been conducted for front-line healthcare staff in China by Li, Mohamed [30] to measure the impact of organisational support on employee turnover intention. This study’s results could verify that organisational support negatively affected employee turnover intention. Saoula and Johari [31] studied this area and determined a negative relationship between organisational support and employee turnover intention. As both of the above explained research have been conducted in non-Western countries, the findings help to complete the theoretical framework for the current study in the Sri Lankan context.”
(Line numbers from 344 to 349)
“Saoula and Johari [31] researched the effect of personality traits (big five) on employee turnover intention. The researchers state that the relationship between the big five personality traits and turnover intention will support early prediction of employee turnover intentions. Identifying employee’s personalities and helping them to find the most suitable job role is a long-term process, though it will be highly advantageous for both employees and the organisation.”
(Line numbers from 365 to 373)
“The organisational learning culture is a key factor for innovative work behaviour. Saoula, Fareed [36] conducted research in Malaysia, a developing country in Asia to examine the relationship between organisational learning culture and employee turnover intention. The organisational learning culture improves learning capability, supports sustainable development, and affects organisation's positive changes. As organisational learning culture and employee turnover intention have a negative relationship, the result helps to identify the impact of innovative work behaviour. According to the existing/available literature, limited studies have been conducted on this topic.”
(Line numbers from 608 to 612)
“Entrepreneurs are the founders of startups. Employees’ entrepreneurial dreams positively affect employee intention to startups. Employees in the startups also will have an ideation to start their own business. According to the study by Li, Li [44] the mediating role of employees’ entrepreneurial self-efficacy and the moderating role of job embeddedness in the influence of entrepreneurial dreams on employees’ turnover intention to startup.”
Reviewer 1 Comment 19: Methodology: How experiment was conducted?
Authors’ Response: Thank you for the comment. The flow of methodology could improve with the help of the next three comments, including this. The experiment was conducted using both online and manual channels.
Accordingly, the revised manuscript is updated as follows in lines 432 and 435.
“…The authors directly distributed the questionnaire. Moreover, authors could contact management in startups and distribute the questionnaire in their organisation.…”
Reviewer 1 Comment 20: Methodology: How participants were recruited?
Authors’ Response: Duly noted with thanks! The participants were selected by random sampling method. Authors could contact the management of respective organisations to reach the respondents.
The methodology part has been written in descriptive manner in the revised document. From lines 432 to 435 and lines 457 to 458 were newly added.
“…The authors directly distributed the questionnaire. Moreover, authors could contact management in startups and distribute the questionnaire in their organisation….”
“…The researchers applied a random sampling method, mainly employees who are a part of or have been a part of the startup …”
Reviewer 1 Comment 21: Methodology: What are the instructions of experiment?
Authors’ Response: Thank you very much for the comment and well noted.
Instructions for the experiments were.
• Participants should be a part of the startup
• He/she should consider the behaviour and culture of that startup when answering the questions
• Respondent should answer all the questions
The instructions given in the questionnaire has included in the revised manuscript from lines 444 to 449.
“…A minimum of four questions was added under each indicator. The researchers facilitated anonymously answering all the questions in the questionnaire. The participants should be a part of startup and he/she should consider the behaviour and culture of that startup when answering the questions. All nine indicators were covered by Likert scale questions from 1 to 5 rating scale, depicting (1) strongly disagree to (5) strongly agree to collect respondents’ attitudes and opinions…”
Reviewer 1 Comment 22: Methodology: How much was time given to each participant?
Authors’ Response: Thank you very much for the comments. This helps to build the story line in methodology part. 15 minutes time were given to answer the questionnaire. New content has added from lines 449 to 452
“…Each respondent took about 10-15 minutes to complete answering the questionnaire and took approximately 5-7 minutes to fill out the questionnaire…”
Reviewer 1 Comment 23: What are the theoretical implications of the study?
Authors’ Response: Well noted your comment. Thank you very much! In revised manuscript has added new sub section to discuss theoretical implications from lines 648 to 654.
“The current study empirically investigated the impact of job satisfaction, innovative work behaviour, co-worker support and leader member exchange on employee turnover. According to the authors’ knowledge, no prior studies were conducted considering the combined impact of all the independent variables on employee turnover. Therefore, this study strengthens the literature by demonstrating how job satisfaction, innovative work behaviour, co-worker support and leader member exchange impact employee turnover in Sri Lankan startups.
The findings reveal that job satisfaction has a negative impact on employee turnover. This finding is consistent with the previous study, job satisfaction significantly predicted employee turnover [6]. This study consolidates past findings that male employees have higher turnover intention than female employees. Female employees have comparatively higher-level job satisfaction [8]. This study implies that employees age 31 to 40 years have high employee turnover intention. The research findings are similar to Lu, Lu [8]; the older employees have high intentions to leave the company.”
Reviewer 1 Comment 24: Practical implications need further discussion.
Authors’ Response: Thank you very much for your valuable comment. Practical implications section was improved with further discussion. New contents have been added from lines 665 to 671, 674 to 680, 686 to 690.
“…This study provides managerial insights on lowering employee turnover in Sri Lankan startups. First, startups need to be aware that experienced employees in startups can be easily taken by well-established companies because, later, they have hand on experience and skills. Therefore, it is important to implement strategies for a solid career development plan, career growth, personal status, and employee recognition. As job satisfaction can predict employee turnover, it is a must to measure those indicators and maintain a favourable level at all times.”
“…More importantly, healthy LMX can boost employees’ work engagement. This healthy level can maintain by conducting regular meetings, training programs and informal mentorship with employees’ immediate supervisors [8]. Further, management can allow employees at all levels to present their fresh ideas and incorporate them to influence organisation’s decision-making process. These processes can lower employee hierarchy and build strong relationships while recognising them in the company.”
“…Furthermore, having a flexible approach to work in an organisation culture will increase the trust between employees and the organisation. Giving the freedom to take risks and not allowing them to feel alone during work will give value to employees. Finally, all the above actions will strongly impact reducing employee intention to leave the organisation.”
Reviewer 1 Comment 25: Add/ involve more recent citations/studies where necessary
Authors’ Response: Thank you very much for the comment and this is well noted. New citations were added in revised manuscript in introduction, literature review and results and discussions sections as per the below line numbers.
(Line numbers from 612 to 616)
Reviewer 2 Comment 1: Abstract: Rewrite the abstract after manuscript correction and provide picture of whole study.
Authors’ Response: Thank you very much for your comment. Abstract has been rewritten after doing the manuscript corrections.
Reviewer 2 Comment 2: In the first paragraph of introduction used only this (2016) citation. This citation does not justify the paragraph.
Authors’ Response: Thank you very much for the comment. The 1st paragraph of introduction section has upgraded with recent literatures with the citation nos. 1, and 3.
New contents have included from lines 45 to 46, and lines 55 to 58.
Reviewer 2 Comment 3: Introduction paragraph is not justifying the problem and bag-round of study. Revised the introduction and use the recent citations to justify and logically make connection with them.
Authors’ Response: Thank you so much for the comment. The introduction section was upgraded with recent literatures with the citation nos. 10, 11, 1, and 3 and justifications.
Reviewer 2 Comment 4: In the introduction (second paragraph), the contribution of study is confused with variable relationships; why are these relationships a contribution of study? Need strong justification.
Authors’ Response: Thank you very much for your valuable comment. The content has been updated with recent citations in line 64.
“Many variables influence employee turnover intentions [4-6]…”
Reviewer 2 Comment 5: Overall, I suggest a major rewrite of the introduction. It should provide an overview of and focus on one issue with recent citations.
Authors’ Response: Thank you very much, the comment well noted. New literatures have added in revised manuscript and highlighted the main issues and the research gaps. Every paragraph of the introduction has updated according to the reviewers’ comments.
Reviewer 2 Comment 6: Revised all literature variables and link with variables with new citation.
Authors’ Response: Thank you very much for the comment and well noted. After adding new literatures, revised all literature variables and linked with variables with new citation.
Reviewer 2 Comment 7: In the literature, justify these hypotheses with literary support.
Authors’ Response: Thank you very much for the comment. A storyline was developed on hypothesis formulation. New contents have been incorporated as per the below line numbers.
Reviewer 2 Comment 8: In literature, justify the conceptual model and theoretical gap.
Authors’ Response: Well noted your comment. Thank you! Conceptual model and theoretical gap have justified in the revised manuscript from lines 420 to 424.
“These studies have a common limitation in gathering more independent variables and analysing the impact. Therefore, a need exists to measure the effect of job satisfaction, work-life balance, happiness, management support, career management, innovative work behaviour, leader member exchange, and co-worker support together on employee turnover.”
Reviewer 2 Comment 9: Where is the total population? How did you choose the sample size? And how did you choose which method, unit of analysis, and research technique to use? Provide justification. Why is this method appropriate for this data set?
Authors’ Response: Thank you very much for this valuable comment.
Total population was 1300 and sample size identified by referring calculator.net online sample size calculator. A stepwise ordered probit analysis method was used as the selected variables are widely used indicators for employee turnover therefore authors required to do a micro level analysis for these variables.
The details of sampling have added in revised manuscript from lines 459 to 465.
“…The sample size was selected by referencing the Krejcie and Morgan sampling table and Calculator.net [40] with a confidence level of 95% and 7% of margin of error. The calculation results indicated a minimum of 171 professionals. A stepwise ordered probit analysis method was used as the selected variables are widely used indicators for employee turnover; therefore, a micro-level analysis was required to study how these variables impact. A pilot survey was conducted to identify whether the purpose of the questions was clear to the respondents.”
Reviewer 2 Comment 10: General: Identifying flaws in the study's design (revised methodology section) and justifying technique.
Authors’ Response: Well noted your comment. Thank you! Methodology section has been updated in revised manuscript from line 520 to 522.
“…The probit model is an estimation technique for equations with dummy dependent variables that avoids the unboundedness problem of the linear probability model by using a variant of the cumulative normal distribution [42]…”
Reviewer 2 Comment 11: Discussion: Write the theoretical contribution related to a model.
Authors’ Response: Thank you very much for your valuable comment.
The revised manuscript has been updated with a new sub section to discuss theoretical implications from lines 648 to 661.
Reviewer 2 Comment 12: Revised topic after correction
Authors’ Response: Well noted your comment. The topic has been updated in lines 1 to 2 and 19 to 20, and the new topic is,
“Factors impacting employee turnover intentions among professionals in Sri Lankan startups”
Submitted filename: Response to the Reviewers.docx
Decision Letter 1
31 Jan 2023
PONE-D-22-30684R1
Dear Dr. Lakshmi Kanchana,
We’re pleased to inform you that your manuscript has been judged scientifically suitable for publication and will be formally accepted for publication once it meets all outstanding technical requirements.
Within one week, you’ll receive an e-mail detailing the required amendments. When these have been addressed, you’ll receive a formal acceptance letter and your manuscript will be scheduled for publication.
An invoice for payment will follow shortly after the formal acceptance. To ensure an efficient process, please log into Editorial Manager at http://www.editorialmanager.com/pone/ , click the 'Update My Information' link at the top of the page, and double check that your user information is up-to-date. If you have any billing related questions, please contact our Author Billing department directly at gro.solp@gnillibrohtua .
If your institution or institutions have a press office, please notify them about your upcoming paper to help maximize its impact. If they’ll be preparing press materials, please inform our press team as soon as possible -- no later than 48 hours after receiving the formal acceptance. Your manuscript will remain under strict press embargo until 2 pm Eastern Time on the date of publication. For more information, please contact gro.solp@sserpeno .
Additional Editor Comments (optional):
Dear Author/s,
Thank you for making all the corrections.
1. If the authors have adequately addressed your comments raised in a previous round of review and you feel that this manuscript is now acceptable for publication, you may indicate that here to bypass the “Comments to the Author” section, enter your conflict of interest statement in the “Confidential to Editor” section, and submit your "Accept" recommendation.
Reviewer #1: All comments have been addressed
Reviewer #2: All comments have been addressed
2. Is the manuscript technically sound, and do the data support the conclusions?
Reviewer #2: (No Response)
3. Has the statistical analysis been performed appropriately and rigorously?
4. Have the authors made all data underlying the findings in their manuscript fully available?
5. Is the manuscript presented in an intelligible fashion and written in standard English?
6. Review Comments to the Author
Reviewer #1: The authors have adequately addressed the comments raised in a previous round of review and I feel that this manuscript is now acceptable for publication
7. PLOS authors have the option to publish the peer review history of their article ( what does this mean? ). If published, this will include your full peer review and any attached files.
Acceptance letter
Dear Dr. Jayathilaka:
I'm pleased to inform you that your manuscript has been deemed suitable for publication in PLOS ONE. Congratulations! Your manuscript is now with our production department.
If your institution or institutions have a press office, please let them know about your upcoming paper now to help maximize its impact. If they'll be preparing press materials, please inform our press team within the next 48 hours. Your manuscript will remain under strict press embargo until 2 pm Eastern Time on the date of publication. For more information please contact gro.solp@sserpeno .
If we can help with anything else, please email us at gro.solp@enosolp .
Thank you for submitting your work to PLOS ONE and supporting open access.
PLOS ONE Editorial Office Staff
on behalf of
Dr. Muhammad Fareed
Predicting and explaining employee turnover intention
- Regular Paper
- Open access
- Published: 23 May 2022
- Volume 14 , pages 279–292, ( 2022 )
Cite this article
You have full access to this open access article
- Matilde Lazzari 1 ,
- Jose M. Alvarez ORCID: orcid.org/0000-0001-9412-9013 2 , 3 &
- Salvatore Ruggieri ORCID: orcid.org/0000-0002-1917-6087 3
37k Accesses
30 Citations
7 Altmetric
Explore all metrics
Turnover intention is an employee’s reported willingness to leave her organization within a given period of time and is often used for studying actual employee turnover. Since employee turnover can have a detrimental impact on business and the labor market at large, it is important to understand the determinants of such a choice. We describe and analyze a unique European-wide survey on employee turnover intention. A few baselines and state-of-the-art classification models are compared as per predictive performances. Logistic regression and LightGBM rank as the top two performing models. We investigate on the importance of the predictive features for these two models, as a means to rank the determinants of turnover intention. Further, we overcome the traditional correlation-based analysis of turnover intention by a novel causality-based approach to support potential policy interventions.
Similar content being viewed by others
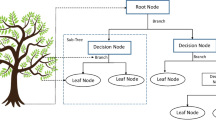
Employee turnover in multinational corporations: a supervised machine learning approach
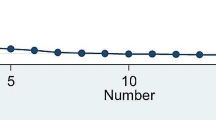
Assessing the Effects of Workplace Contextual Factors on Turnover Intention: Evidence from U.S. Federal Employees
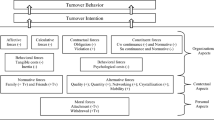
Models for antecedents of turnover intention and behavior among Brazilian employees
Avoid common mistakes on your manuscript.
1 Introduction
Employee turnover refers to the situation where an employee leaves an organization. It can be classified as voluntary , when it is the employee who decides to terminate the working relationship, or involuntary , when it is the employer who decides [ 33 ]. Voluntary turnover is divided further into functional and dysfunctional [ 26 ], which refer to, respectively, the exit of low-performing and high-performing workers. This paper focuses on voluntary dysfunctional employee turnover (henceforth, employee turnover) as the departure of a high-performing employee can have a detrimental impact on the organization itself [ 62 ] and the labor market at large [ 33 ].
It is important for organizations to be able to retain their talented workforce as this brings stability and growth [ 30 ]. It is also important for governments to monitor whether organizations are able to do so as changes in employee turnover can be symptomatic of an ailing economic sector. Footnote 1 For instance, the European Commission includes it in its annual joint employment report to the European Union (EU) [ 14 ]. Understanding why employees leave their jobs is crucial for both employers and policy makers, especially when the goal is to prevent this from happening.
Turnover intention, which is an employee’s reported willingness to leave the organization within a defined period of time, is considered the best predictor of actual employee turnover [ 34 ]. Although the link between the two has been questioned [ 13 ], it is still widely used for studying employee retention as detailed quit data is often unavailable due to, e.g., privacy policies. Moreover, since one precedes the other, the correct prediction of intended turnover enables employers and policy makers alike to intervene and thus prevent actual turnover.
In this paper, we model employee turnover intention using a set of traditional and state-of-the-art machine learning (ML) models and a unique cross-national survey collected by Effectory Footnote 2 , which contains individual-level information. The survey includes sets of questions (called items ) organized by themes that link an employee’s working environment to her willingness to leave her work. Our objective is to train accurate predictive models, and to extract from the best ones the most important features with a focus on such items and themes. This allows the potential employer/policy maker to better understand intended turnover and to identify areas of improvement within the organization to curtail actual employee turnover.
We train three interpretable (k-nearest neighbor, decision trees, and logistic regression) and four black-box (random forests, XGBoost, LightGBM, and TabNet) classifiers. We analyze the main features behind our two best performing models (logistic regression and LightGBM) across multiple folds on the training data for model robustness. We do so by ranking the features using a new procedure that aggregates their model importance across folds. Finally, we go beyond correlation-based techniques for feature importance by using a novel causal approach based on structural causal models and their link to partial dependence plots. This in turn provides an intuitive visual tool for interpreting our results.
In summary, the novel contributions of this paper are twofold. First, from a data science perspective:
we analyze a real-life, European-wide, and detailed survey dataset to test state-of-the-art ML techniques;
we find a new top-performing model (LightGBM) for predicting turnover intention;
we carefully study the importance of predictive features which have causal policy-making implications.
Second, method-wise:
we devise a robust ranking method for aggregating feature importance across many folds during cross-validation;
we are the first work in the employee turnover literature to use causality (in the form of structural causal models) for interventional (causal) analysis of ML model predictions.
The paper is organized as follows. First, we review related work in Sect. 2 . The Global Employee Engagement Index (GEEI) survey is described in Sect. 3 . The comparative analysis of predictive models is conducted in Sect. 4 , while Sect. 5 studies feature importance. Section 6 investigates the causal inference analysis. Finally, we summarize the contributions and limitations of our study in Sect. 7 .
2 Related work
We present the relevant literature around modeling and predicting turnover intention. Given our interdisciplinary approach, we group the related work by themes.
Turnover determinants . The study of both actual and intended employee turnover has had a long tradition within the fields of human resource management [ 45 ] and psychology [ 34 ]. The work has focused mostly on what factors influence and predict employee turnover [ 27 ]. Similarly, a complementary line of work has focused on job embeddedness, or why employees stay within a firm [ 42 , 60 ]. A number of determinants have been identified for losing, or conversely, retaining employees [ 56 ], including demographic ones (such as gender, age, marriage), economic ones (working time, wage, fringe benefits, firm size, carrier development expectations) and psychological ones (carrier commitment, job satisfaction, value attainment, positive mood, emotional exhaustion), among others. The items and themes along with employee contextual information reported in GEEI capture these determinants.
Most of this literature has centered on the United States or on just a few European countries. See, for instance, [ 56 ] and [ 57 ], respectively. Our analysis is the first to cover almost all of the European countries.
Modeling approaches . Traditional approaches for testing the determinants of employee turnover have focused largely on statistical significance tests via regression and ANOVA analysis, which are tools commonly used in applied econometrics. See, e.g., [ 27 , 56 ]. This line of work has embraced causal inference techniques as it works often with panel data, resorting to other econometric tools such as instrumental variables and random/fixed effects models. For a recent example see [ 31 ]. For an overview on these approaches see [ 5 ].
There has been a recent push for more advanced modeling approaches with the raise of human resource (HR) predictive analytics, where ML and data mining techniques are used to support HR teams [ 46 ]. This paper falls within this line of work. Most ML approaches use classification models to study the predictors of turnover. See, e.g., [ 2 , 20 , 24 , 36 ]. The common approach among papers in this line of work is to test many ML models and to find the best one for predicting employee turnover. However, despite the fact that some of these papers use the same datasets, there is no consensus around the best models. Using the same synthetic dataset, e.g., [ 2 ] finds the support vector machine (SVM) to be the best-performing model while [ 20 ] finds it to be the naive Bayes classifier. We note, however, that similar to [ 24 ] we find the logistic regression to be one of our top-performing models. This paper adds to the literature by introducing a new top-performing model to the list, the LightGBM.
Similarly, this line of work does not agree on the top data-driving factors behind employee turnover either. For instance, [ 2 ] identifies overtime as the main driver while [ 24 ] identifies it to be the salary level. This paper adds to this aspect in two ways. First, rather than reporting feature importance on a final model, we do so across many folds for the same model, which gives a more robust view on each feature’s importance within a specific model. Second, we go beyond the limited correlation-based analysis [ 3 ] by incorporating causality into our feature importance analysis.
Among the classification models used in the literature and from the recent state-of-the-art in ML, we will experiment with the following models: logistic regression [ 35 ], k-nearest neighbor [ 53 ], decision trees [ 11 ], random forests [ 10 ], XGBoost [ 12 ], and the more recent LightGBM [ 37 ], which is a gradient boosting method [ 23 ]. Ensemble of decision trees achieve very good performances in general, with few configuration parameters [ 16 ], and especially when the distribution of classes is imbalanced [ 9 ], which is typically the case for turnover data. Recent trends in (deep) neural networks are showing increasing performances of sub-symbolic models for tabular data (see the survey [ 7 ]). We will experiment with TabNet [ 6 ], which is one of the top recent approaches in this line. Implementations of all of the approaches are available in Python with uniform APIs.
Modeling intent . A parallel and growing line of research focuses on predicting individual desire or want (i.e., intent or intention) over time using graphical and deep learning models. These approaches require sequential data detailed per individual. The adopted models allow to account for temporal dependencies within and across individuals for identifying patterns of intent. Intention models have been used, for example, to predict driving routes for drivers [ 55 ], online consumer habits [ 58 , 59 ], and even for suggesting email [ 54 ] and chat bot responses [ 52 ]. Our survey data has a static nature, and therefore we cannot directly compare with those models, which would be appropriate for longitudinal survey data.
Determining feature importance . Beyond predictive performance, we are interested in determining the main features behind turnover. To this end, we build on the explainable AI (XAI) research [ 28 ], in particular XAI for tabular data [ 49 ], for extracting from ML models a ranking of the features used for making (accurate) predictions. ML models can either explain and present in understandable terms the logic of their predictions (white-boxes) or they can be obscure or too complex for human understanding (black-boxes). The k-nearest neighbor, logistic regression, and decision trees models we use are white-box models. All the other models are black-box models. For the latter group, we use the built-in model-specific methods for feature importance. We, however, add to this line of work in two ways. First, we device our own ranking procedure to aggregate each feature’s importance across many fold. Second, following [ 63 ] we use structural causal models (SCM) [ 47 ] to equip the partial dependence plot (PDP) [ 22 ] with causal inference properties. PDP is a common XAI visual tool for feature importance. Under our approach, we are able to test causal claims around drivers of turnover intention.
Turnover data . Predictive models are built from survey data (questionnaires) and/or from data about workers’ history and performances (roles covered, working times, productivity). Given its sensitive information, detailed data on actual and intended turnover is difficult to obtain. For instance, all of the advanced modeling approaches previously mentioned either use the IBM Watson synthetic data set Footnote 3 or the Kaggle HR Analytics dataset Footnote 4 . This paper contributes to the existing literature by applying and testing the latest in ML techniques to a unique, relevant survey data for turnover intention. The GEEI survey offers a level of granularity via the items and themes that is not present in the commonly used datasets. This is useful information to both employers and policy makers, which allows this paper to have a potential policy impact.
Causal analysis. We note that this is not the first paper to approach employee turnover from a causality perspective, but, to the best of our knowledge, it is the first to do so using SCM. Other papers such as [ 25 ] and [ 48 ] use causal graphs as conceptual tools to illustrate their views on the features behind employee turnover. However, these papers do not equip their causal models with any interventional properties. Some works, e.g., [ 4 , 21 , 61 ], go further by testing the consistency of their conceptual models with data using path analysis techniques. Still, none of these three papers use SCM, meaning that they cannot reason about causal interventions.
3 The GEEI survey and datasets
Effectory ran in 2018 the Global Employee Engagement Index (GEEI) survey, a labor market questionnaire that covered a sample of 18,322 employees from 56 countries. The survey is composed of 123 questions that inquire contextual information (socio-demographic, working and industry aspects), items related to a number of HR themes (also called, constructs), and a target question. The target question (or target variable , the one to be predicted) is the intention of the respondent to leave the organization within the next three months. It takes values leave (positive) and stay (negative). The design and validation of the GEEI questionnaire followed the approach of [ 18 ]. After reviewing the social science literature, the designers defined the relevant themes, and items for each theme. Then they ran a pilot study in order to validate psychometric properties of questions to assess their internal consistency, and to test convergent and discriminant validity Footnote 5 of questions.
Contextual information is reported in Table 1 , together with type of data encoded – binary for two-valued domains (male/female gender, profit/non-profit type of business, full/part time work status), nominal for multi-valued domains (e.g., country name), and ordinal for ranges of numeric values (e.g., age band) or for ordered values (e.g., primary/secondary/higher education level).
Items refer to questions related to a theme. The items for the Trust theme are shown in Table 2 . There are 112 items in total Footnote 6 . Each item admits answers in Likert scale. A score from 0 to 10 is assigned to an answer by a respondent as follows:
Strongly agree \(\rightarrow \) 10
Agree \(\rightarrow \) 7.5
Neither agree nor disagree \(\rightarrow \) 5
Disagree \(\rightarrow \) 2.5
Strongly disagree \(\rightarrow \) 0
The direction of the response scale is uniform throughout all the items [ 50 ]. Table 3 shows the list of all 23 themes. For a respondent, a score from 0 to 10 is also assigned to a theme as the average score of the items of the theme.
From the raw data of the GEEI survey, we constructed two Footnote 7 tabular datasets, both including the contextual information. The dataset with also the scores of the themes is called the themes dataset . The dataset with also the scores of the items is called the items dataset . The datasets are restricted to respondents from 30 countries in Europe. The GEEI survey includes 303 to 323 respondents per country, with the exception of Germany which has 1342 respondents. We sampled 323 German respondents stratifying by the target variable. Thus, the datasets have an approximately uniform distribution per country. Also, gender is uniformly distributed with 50.9% of males and 49.1% of females. These forms of selection bias do not take into account the (working) population size of countries. Caution will be mandatory when making conclusions about inferences on those datasets. Finally, Fig. 1 shows the distribution of respondents by age and gender.
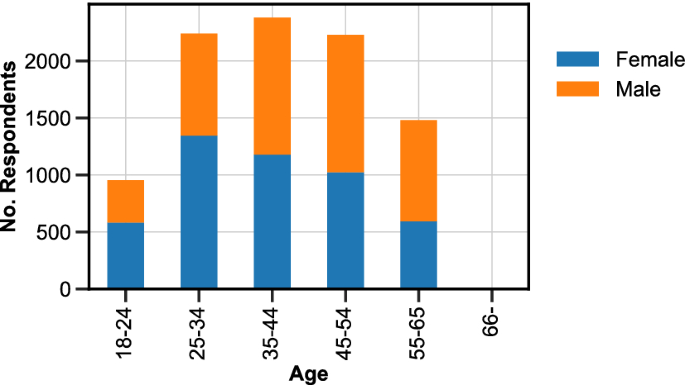
Distribution of respondents by Age and Gender
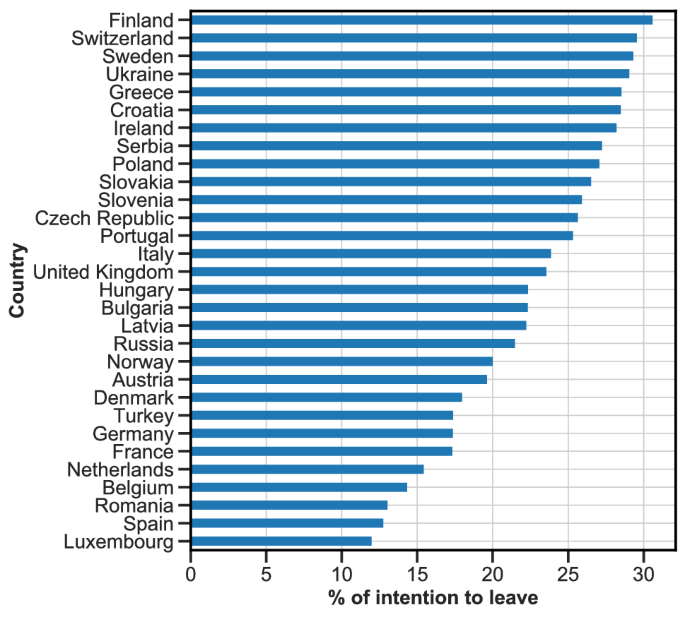
Target variable by Country
In summary, the two datasets have a total of 9,296 rows each, one row per respondent. Only 51 values are missing (out of a total of 1.1M cell values), and they have been replaced by the mode of the column they appear in. The positive rate is 22.5% on average, but it differs considerably across countries, as shown in Fig. 2 . In particular, it ranges from 12% of Luxemburg up to 30.6% of Finland.
4 Predictive modeling
Our first objective is to compare the predictive performances of a few state-of-the-art machine learning classifiers on both the datasets, which, as observed, are quite imbalanced [ 9 ]. We experiment with interpretable classifiers, namely k-nearest neighbors (KNN), decision trees (DT) and ridge logistic regression (LR), and with black-box classifiers, namely random forests (RF), XGBoost (XGB), LightGBM (LGBM), and TabNet (TABNET). We use the scikit-learn Footnote 8 implementation of LR, DT, and RF, and the xgboost Footnote 9 , lightgbm Footnote 10 , and pytorch-tabnet Footnote 11 Python packages of XGB, LGBM, and TABNET. Parameters are left as default except for the ones set by hyper-parameter search (see later on).
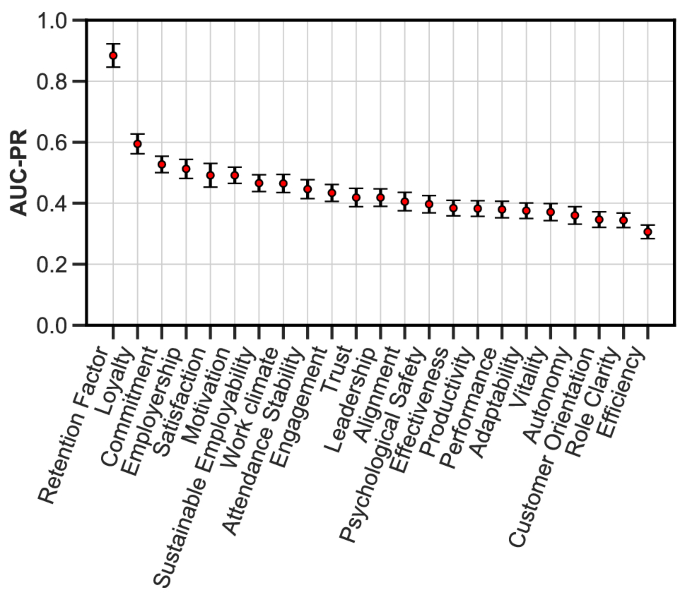
AUC-PR of logistic regression on a single theme. Bars show mean ± stdev over \(10 \times 10\) cross-validation folds
We adopt repeated stratified 10-fold cross validation as testing procedure to estimate the performance of classifiers. Cross-validation is a nearly unbiased estimator of the generalization error [ 40 ], yet highly variable for small datasets. Kohavi recommends to adopt a stratified version of it. Variability of the estimator is accounted for by adopting repetitions [ 39 ]. Cross-validation is repeated 10 times. At each repetition, the available dataset is split into 10 folds, using stratified random sampling. An evaluation metric is calculated on each fold for the classifier built on the remaining 9 folds used as training set. The performance of the classifier is then estimated as the average evaluation metric over the 100 classification models (10 models times 10 repetitions). An hyper-parameter search is performed on each training set by means of the Optuna Footnote 12 library [ 1 ] through a maximum of 50 trials of hyper-parameter settings. Each trial is a further 3-fold cross-validation of the training set to evaluate a given setting of hyper-parameters. The following hyper-parameters are searched for: (LR) the inverse of regularization strength; (DT) the maximum tree depth; (RF) the number of trees and their maximum depth; (XGBoost) the number of trees, number of leaves in trees, the stopping parameter of minimum child instances, and the re-balancing of class weights; (LightGBM) minimum child instances, L1 and L2 regularization coefficients, number of leaves in trees, feature fraction for each tree, data (bagging) fraction, and frequency of bagging; (TABNET) the number of shared Gated Linear Units.
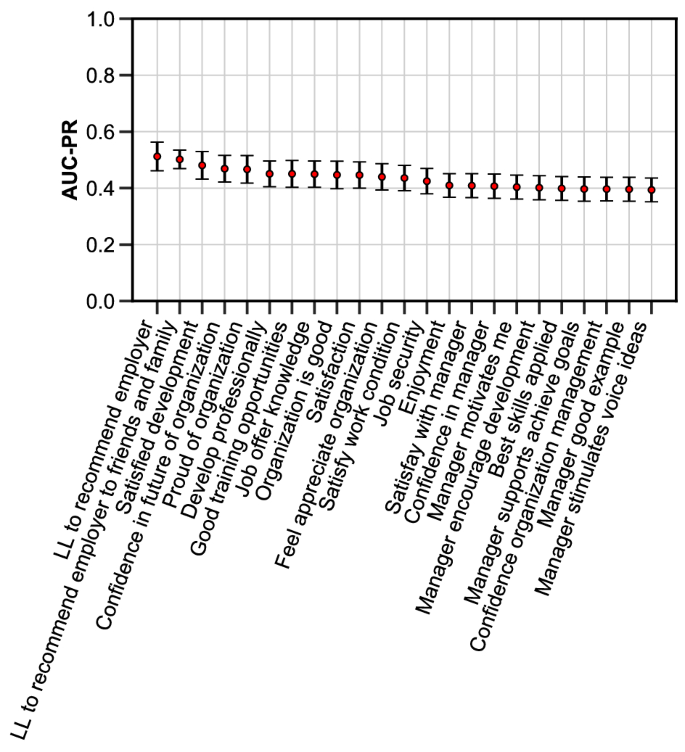
AUC-PR of logistic regression on a single item. Bars show mean ± stdev over \(10 \times 10\) cross-validation folds
As evaluation metric, we consider the Area Under the Precision-Recall Curve (AUC-PR) [ 38 ], which is more informative than the Area Under the Curve of the Receiver operating characteristic (AUC-ROC) on imbalanced datasets [ 15 , 51 ]. A random classifier achieves an AUC-PR of 0.225 (positive rate), which is then the reference baseline. A point estimate of the AUC-PR is the mean average precision over the 100 folds [ 8 ]. Confidence intervals are calculated using a normal approximation over the 100 folds [ 19 ]. We refer to [ 8 ] for details and for a comparison with alternative confidence interval methods.
Let us first concentrate on the case of the themes dataset. As a feature selection pre-processing step, we run a logistic regression for each theme, with the theme as the only predictive feature. Fig. 3 reports the achieved AUC-PRs (mean ± stdev over the \(10 \times 10\) cross-validation folds). It turns out that the top three themes (Retention factor, Loyalty, and Commitment) include among their items a question close or exactly the same as the target question. For this reason, we removed these themes (and their items, for the item dataset) from the set of predictive features. The nominal contextual features from Table 1 , namely Country, Industry, and Job function, are one-hot encoded.
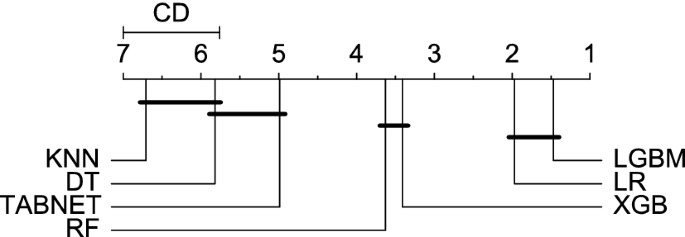
(Unweighted) items dataset: Critical Difference (CD) diagram for the post hoc Nemenyi test at 99.9% confidence level [ 17 ]
The performances of the experimented classifiers are shown in Table 4 (top). It includes the AUC-PR (mean ± stdev), the 95% confidence interval of the AUC-PR, and the elapsed time Footnote 13 (mean ± stdev), including hyper-parameter search, over the \(10 \times 10\) cross-validation folds. AUC-PRs for all classifiers are considerably better than the baseline (more than twice the baseline even for the lower limit of the confidence interval). DT is the fastest classifier Footnote 14 , but, together with KNN, also the one with lowest predictive performance. LGBM has the best AUC-PR values and an acceptable elapsed time. LR is runned up, but it is almost as fast as DT. RF has a performance close to LGBM and LR but it slower. XGB is in the middle as per AUC-PR and elapsed time. Finally, TABNET has intermediate performances, but it is two orders of magnitude slower than its competitors.
The statistical significance of the difference of mean performances of classifiers is assessed with two-way ANOVA if values are normally distributed (Shapiro’s test) and homoscedastic (Bartlett’s test). Otherwise, the nonparametric Friedman test is adopted [ 17 , 32 ]. For the theme dataset, ANOVA was used. The test shows a statistically significant difference among the mean values (family-wise significance level \(\alpha = 0.001\) ). The post hoc Tukey HSD test shows a no significant difference between LGBM and LR. All other differences are significant, as shown in Table 4 (top).
As a natural question, one may wonder how the performance would change if the datasets were weighted to reflect the workforce of each country. We collected the employment figures for all the countries in our training dataset for 2018, which was when the survey was carried out. The country-specific employment data was obtained from Eurostat Footnote 15 (for the EU member states as well as for the United Kingdom) and from the World Bank Footnote 16 (for Russia and Ukraine). The numbers correspond to the country’s total employed population between the ages of 15 and 74. For Russia and Ukraine, however, the number corresponds to the total employed population at any age. We assigned a weight to each instance in our datasets proportional to the workforce in the country of the employee. Weights are considered both in training of classifiers and in the evaluation metric (weighted average precision). The weighted positive rate is 20%. Table 4 (bottom) shows the performances of the classifiers over the weighted dataset. The mean AUC-PR is now smaller for most classifier, the same for LGBM, and slightly better for RF. Standard deviation has increased in all cases. The post hoc Tukey HSD test now shows a small significant difference between LGBM and LR.
Let us now consider the items dataset. Figure 4 shows the predictive performances of single-feature logistic regressions. Table 5 reports the performances of classifiers on all features for both the unweighted and the weighted data. Overall performances of each classifier improve over the theme dataset. Elapsed times also increase due to the larger dimensionality of the dataset. Differences are statistically significant. LGBM and LR are the best classifiers for both the unweighted and the weighted datasets. Figure 5 shows the critical difference diagram for the post hoc Nemenyi test for the unweighted dataset following a significant Friedman test. An horizontal line that crosses two or more classifier lines means that the mean performances of those features are not statistically different. In summary, we conclude that the LR and LGBM classifiers have highest predictive power of the turnover intention.
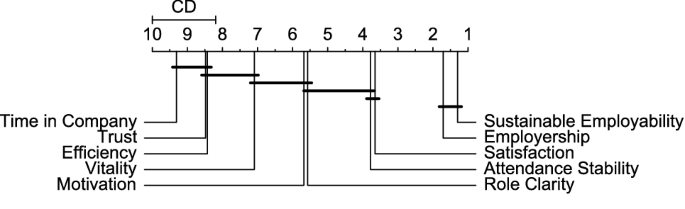
Weighted theme dataset: CD diagram for the post hoc Nemenyi test at 99.9% confidence level for the top-10 LR feature importances

Weighted item dataset: CD diagram for the post hoc Nemenyi test at 99.9% confidence level for the top-10 LR feature importances
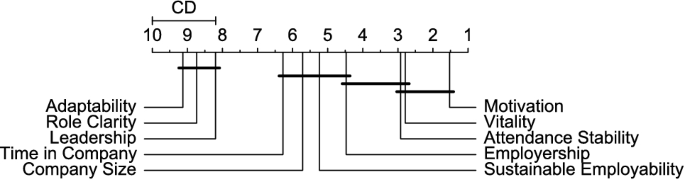
Weighted theme dataset: CD diagram for the post hoc Nemenyi test at 99.9% confidence level for the top-10 LGBM feature importances
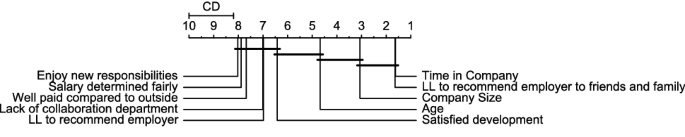
Weighted item dataset: CD diagram for the post hoc Nemenyi test at 99.9% confidence level for the top-10 LGBM feature importances
5 Explanatory factors
We examine the driving features behind the two top-performing models found in Sect. 4 : the LGBM and the LR. We use each model’s specific method for determining feature importance and aggregate the importance ranks over the 100 experimental folds. This novel approach yields more robust estimates (a.k.a., lower variance) of importance ranks than using a single hold-out set. We do so for the weighted version of both the theme and item datasets.
For a fixed fold, feature importance of the LR model is determined as the absolute value of the feature’s coefficient in the model. The importance of a feature in the LGBM model is measured as the number of times the feature is used in a split of a tree in the model. We aggregate feature importance using their ranks, as in nonparametric tests statistical [ 32 ]. For instance, LR absolute coefficients \((|\beta _1|, |\beta _2|, |\beta _3|, |\beta _4|) = (1, 2, 3, 0.5)\) lead to the ranking (3, 2, 1, 4).
The top-10 features w.r.t. the mean rank over the 100 folds are shown in Fig. 6 to Fig. 9 for the theme/item datasets and LR/LGBM models. For the theme dataset (resp., the item dataset), LR and LGBM share almost the same set of top features with slight differences in the mean ranks. For example, the Sustainable Employability , Employership , and Attendance Stability themes are all within the top-five features for both LR and LGBM. For the item dataset, we observe Time in Company , Satisfied Development , and Likelihood to Recommend Employer to Friends and Family to be among the top-five shared features. Interestingly, Gender , a well-recognized determinant of turnover intention, is not among the top features for both datasets. Also, no country-specific effect emerges.
The Friedman test shows significant differences among the importance measures in all four cases in Fig. 6 to Fig. 9 .
Further, the figures show the critical difference diagrams for the post hoc Nemenyi test, thus answering the question whether there is any statistical difference among them. An horizontal line that crosses two or more feature lines means that the mean importances of those features are not statistically different. In Fig. 8 , for example, the Motivation , Vitality , and Attendance Stability themes are grouped together.
Statistical significance of different feature importance is valuable information when drawing potential policy recommendations as we are able to prioritize policy interventions. For example, given these results, a company interested in employee retention could focus on improving either motivation or vitality, as they strongly influence LGBM predictions and, a fortiori , turnover intention. However, the magnitude and direction of the influence is not accounted for in the feature importance plots of Fig. 6 to Fig. 9 . This is not actually a limitation of our (nonparametric) approach. Any association measure between features and predictions (such as the coefficients in regression models) does not allow for causal conclusions. We intend to overcome correlation analysis, as a means to support policy intervention, thought an explicit causal approach.
6 Causal analysis
In Sect. 4 , we found LGBM and LR to be the best performing models for predicting turnover intention, and in Sect. 5 we studied the driving features behind the two models. Now we want to assess whether a specific theme T has a causal effect on the target variable, written \(T \rightarrow Y\) , given the trained model b (as in b lack-box) and the contextual attributes in Table 1 . We use \(T^*\) to denote the set of remaining themes and \(\tau \) to denote the set of all themes, such that \(\tau = \{T\} \cup T^*\) . Establishing evidence for a direct causal link between T and Y would allow our model b to answer intervention-policy questions related to the theme scores. Given our focus on T , in this section we work only with the theme dataset.
We divide all contextual attributes into three distinct groups based on their level of specificity: individual-specific attributes, I , where we include attributes such as Age and Gender ; work-specific attributes, W , where we include attributes such as Work Status and Industry ; and geography-specific attributes, G , where we include the attribute Country . Footnote 17 We summarize the causal relationships across the contextual attributes, a given theme’s score T , the remaining themes \(T^*\) , and the target variable Y using the causal graph \({\mathcal {G}}\) in Fig. 10 . The nodes on the graph represent groupings of random variables, while the edges represent causal claims across the variable groupings. Within each of these contextual nodes, we picture the corresponding variables as their own individual nodes independent from each other but with the same causal effects with respect to the other groupings. Footnote 18
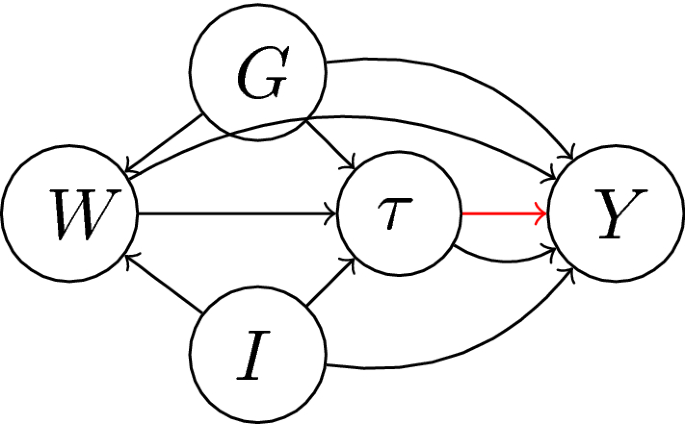
Causal graph \({\mathcal {G}}\) showing the three groups of contextual attributes (individual I , geographic G , and working W ), the collection of themes ( \(\tau \) ) and the target variable Y . We are interested in the edge going from \(\tau \) into Y
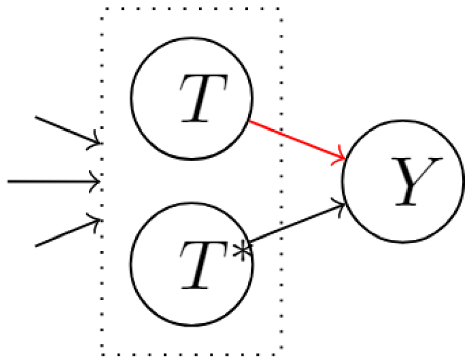
A more detailed look into \(\tau \) (dashed black-rectangle) where we can see the distinct edges going from T and \(T^*\) into Y . The three incoming edges represent the information flow from W , G , and I into \(\tau \) . Here, for illustrative purposes, we have ignored those same edges going into Y
Notice that in Fig. 10 two edges go from \(\tau \) to Y . This is because we have defined \(\tau = \{T\} \cup T^*\) and are interested in identifying the edge between T and Y (marked in red), while controlling for the edges from \(T^*\) to Y (marked in black as the rest). This becomes clearer in Fig. 11 where we detailed the internal structure of \(\tau \) . Here, we assume independence between whatever theme is chosen as T and the remaining themes in \(T^*\) . Footnote 19 Further, as with the contextual nodes representing the variable groupings, \(T^*\) represents the grouping of all themes in \(\tau \) but T where each theme is its own node and independent of each other while have the same inward and outward causal effects. Footnote 20
Under \({\mathcal {G}}\) , all three contextual attribute groups act as confounders between T and Y and thus need to be controlled for along with \(T^*\) to be able to identify the causal effect of T on Y . Otherwise, for example, observing a change in Y cannot be attributed to changes in T as G (or, similarly, I or W ) could have influenced both simultaneously, resulting in an observed association that is not rooted on a causal relationship. Therefore, controlling for G , as for the rest of the contextual attributes insures the identification of \(T \rightarrow Y\) . This is formalized by the back-door adjustment formula [ 47 ], where \(X_{C} = I \cup W \cup G \cup T^*\) is the set for all contextual attributes:
In ( 1 ), the term \(P(X_{C}=x_{C})\) is thus shorthand for \(P(I=i, W=w, G=g, T^*=t^*)\) . The set \(X_{C}\) satisfies the back-door criterion as none of its nodes are descendants of T and it blocks all back-door paths between T and Y [ 47 ]. Given \(X_{C}\) , under the back-door criterion, the direct causal effect \(T \rightarrow Y\) is identifiable. Further, ( 1 ) represents the joint distribution of the nodes in Fig. 10 after a t intervention on T , which is illustrated by the \( do \) -operator. If T has a causal effect on Y , then the original distribution P ( Y ) and the new distribution \(P(Y| do (T:=t))\) should differ over different values of t . The goal of such interventions is to mimic what would happen to the system if we were to intervene it in practice. For example, consider a European-wide initiative to improve confidence among colleagues, such as providing subsidies to team-building courses at companies. Then the objective of this action would be to improve the Trust theme’s score to a level t with the hopes of affecting Y .
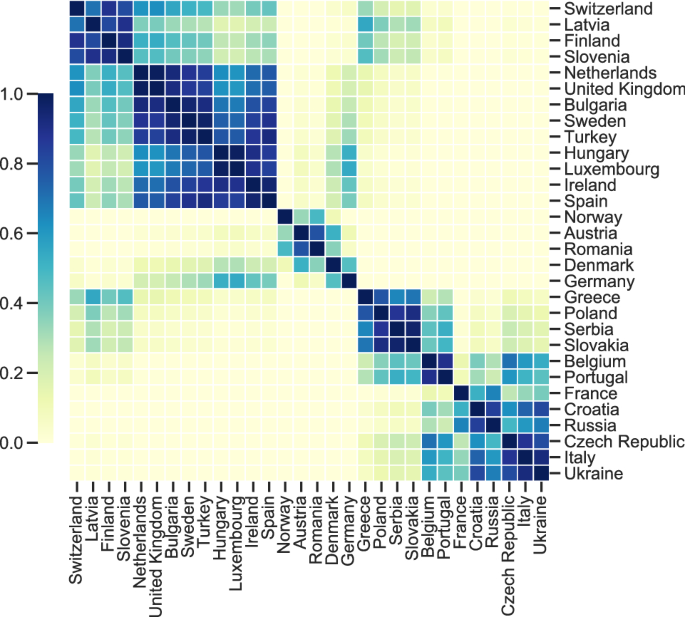
Pairwise Conover-Iman post hoc test p-value for Trust theme vs Country in a clustered map. The map clusters together countries whose score distributions are similar
The structure of the causal graph \({\mathcal {G}}\) in Fig. 10 is motivated both from the data and from expert knowledge. Here we argue that I , W , and G are potential confounders of T and Y . For instance, consider the Country attribute, which belongs to G . It is sensible to picture that Country affects T as employees from different cultures can have different views on the same theme. Similarly, Country can affect Y as different countries have different labor laws that could make some labor markets more dynamic (reflected in the form of higher turnover rates) than others. We also observe this in the data. In particular, the Country attribute is correlated to each of the themes: the nonparametric Kruskal–Wallis H test [ 32 ] shows a p-value close to 0 for all themes, which means that we reject the null hypothesis that the scores of a theme in all countries originate from the same distribution. Consider the Trust theme. To understand which pair of countries have similar/different Trust score distributions, we run the Conover-Iman post hoc test pairwise. The p-values are shown in the clustered map of Fig. 12 . The groups of countries highlighted Footnote 21 by dark colors (e.g., Switzerland, Latvia, Finland, Slovenia) are similar among them in the distribution of Trust scores, and dissimilar from the countries not in the group. Such clustering shows that the societal environment of a specific country has some effect on the respondents’ scores of the Trust theme. Similar conclusions hold for all other themes.
Further, both G and I have a direct effect also on W . We argue that country-specific traits, from location to internal politics, will affect the type of industries that developed nationally. For example, countries with limited natural resources will prioritize non-commodity-intensive industries. Similarly, individual-specific attributes will determine the type of work that an individual can perform. For example, individuals with higher education, where education is among the attributes in I , can apply to a wider range of industries than an individual with lower levels of educational attainment.
To summarize thus far, our goal in this section is to test the claim that a given T causes Y given our model b and our theme dataset. To do so we have defined the causal graph \({\mathcal {G}}\) in Fig. 10 and defined the corresponding set \(X_{C}\) that satisfies the back-door criterion that would allow us to test \(T \rightarrow Y\) using ( 1 ). What we are missing then is a procedure for estimating ( 1 ) over our sample to test our causal claim.
For estimating ( 1 ) we follow the procedure in [ 63 ] and use the partial dependence plot (PDP) [ 22 ] to test visually the causal claim. The PDP is a model-agnostic XAI method that shows the marginal effect one feature has on the predicted outcomes generated by the model [ 43 ]. If changing the former leads to changes in the latter, then we have evidence of a partial dependency between the feature of interest and the outcome variable that is manifested through the model output. Footnote 22 We define formally the partial dependence of feature T on the outcome variable Y given the model b and the complementary set \(X_C\) as:
If there exist a partial dependence between T and Y , then \(b_{T}(t)\) should vary over different values of T , which could be visually inspected by plotting the values via the PDP. If \(X_C\) satisfies the back-door criterion, [ 63 ] argues, then ( 2 ) is equivalent to ( 1 ), Footnote 23 and we can use the PDP to check visually our causal claim. Under this scenario, the PDP would have a stronger claim than partial dependence between T and Y , as it would also allow for causal claims of the sort \(T \rightarrow Y\) . Footnote 24 Therefore, we could assess the claim \(T \rightarrow Y\) by estimating ( 2 ) over our sample of n respondents using:
Using ( 3 ), we can now visually assess the causal effect of T on Y by plotting \({\hat{b}}_T\) against values of T . If \({\hat{b}}_T\) varies across values of t , i.e. \({\hat{b}}_T\) is indeed a function of t , then we have evidence for \(T \rightarrow Y\) [ 63 ].
However, before turning to the estimation of ( 3 ), we address the issue of representativeness (or lack thereof) in our dataset. One implicit assumption used in ( 3 ) is that any j element in \(X_C^{(j)}\) is equiprobable. Footnote 25 This is often assumed because we expect random sampling (or, in practice, proper sampling techniques) when creating our dataset. For example, the probability of sampling a German worker and a Belgian worker would be same. This is a very strong assumption (and one that is hard to prove or disprove), which can become an issue if we were to deploy the trained model b as it may suffer from selection bias and could hinder the policy maker’s decisions.
To account for this potential issue, one approach is to estimate \(P(X_C=x_c)\) from other data sources such as official statistics. This is why, for example, we created the country weighted versions of the theme and item datasets back in Sect. 4 . Here it would be better to do the same not just for country, but to weight across the entirety of the complementary set. Footnote 26 However, this was not possible. The main complication we found for estimating the weight of the complementary set was that there is no one-to-one match between the categories used in the survey and the EU official statistics. Therefore, it is important to keep this in mind when interpreting the results beyond the context of the paper. By using the (country-)weighted theme dataset, we can rewrite ( 3 ) as a country-specific weighted average:
where \(\alpha _j\) is the weight assigned to j ’s country, and \(\alpha = \sum _{j=1}^n \alpha ^{(j)}\) . Under this approach, we are still using the causal graph \({\mathcal {G}}\) in Fig. 10 .
We proceed by estimating the PDP using ( 4 ). We define as T our top feature from the LGBM model in the weighted theme dataset, which was the Motivation theme as seen in Fig. 8 . We then use the corresponding top LGBM hyper-parameters and retrain the classifier on the entire dataset. Footnote 27 Finally, we compute the PDP for Motivation theme as shown in Fig. 13 . We do the same for the LR model for comparison.
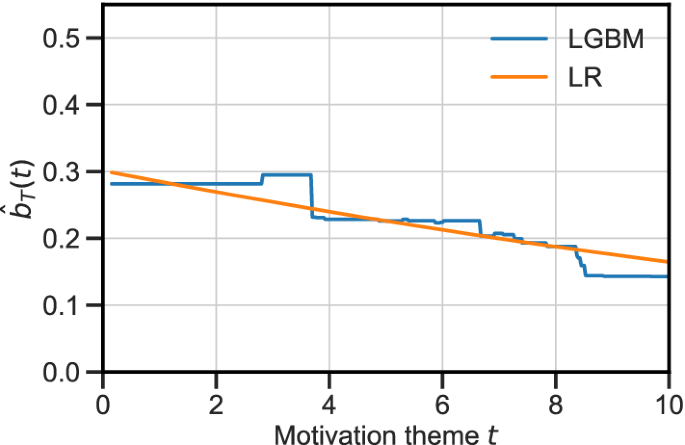
PDP for the Motivation theme for both LGBM and LR models using the weighted theme dataset
From Fig. 13 , under the causal graph \({\mathcal {G}}\) , we can conclude that there is evidence for the causal claim \(T \rightarrow Y\) for the Motivation theme. For the LGBM model, the theme score ( x-axis ), which ranges from 0 to 10, as it increases the corresponding predicted probabilities of employee turnover decrease, meaning that a higher motivation score leads to a lower employee turnover intention. We see a similar, though smoother, behaviour with the LR model. This is expected as the LGBM can capture non-linear relationships between the variables better than the LR.
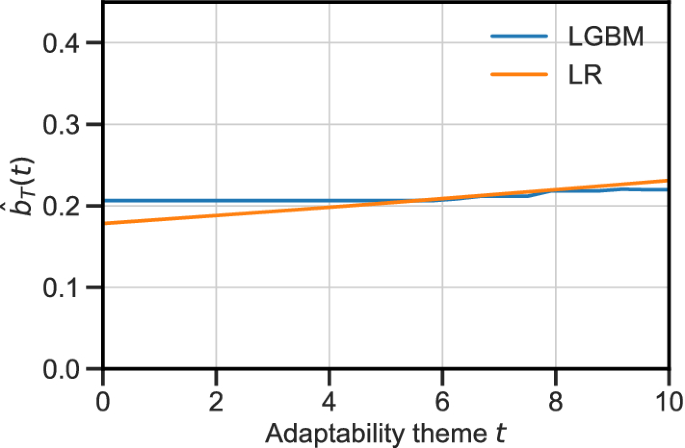
PDP for the Adaptability theme for both LGBM and LR models using the weighted theme dataset
We repeat the procedure on a non-top-ranked theme for both models, namely the Adaptability theme (the capability to adapt to changes), to see how the PDPs compare. The results are shown in Fig. 14 . In the case of the LGBM, the PDP is essentially flat and implies a potential non-causal relationship between this theme and employee turnover intention. For the LR, however, we see a non-flat yet narrower PDP, which also seems to support a potential non-causal link. This might be due again to the non-linearity in the data, where the more flexible model (LGBM) can better capture the effects in the changes of T than the less flexible one (LR) that can tend to overestimate them.
To summarize this approach for all themes, we calculate the change in PDP, which we define as:
and do this for all themes across the LGBM and LR models. The results are shown in Table 6 . Themes are ordered based on the LGBM’s deltas. We note that the deltas across models tend to agree: the signs (and for some themes like Motivation even the magnitudes) coincide. This is inline with previous results in other sections where the LR’s behaviour is comparable to the LGBM’s. Further, comparing the ordering of the themes in Table 6 with the feature rankings in Fig. 6 and 8 , we note that some of the theme’s with the largest deltas (such as Sustainable Emp. and Employership ) are also among the top-ranked features. Although there is no clear one-to-one relationship between the two approaches, it is comforting to see the top-ranked themes also having the higher causal impact on employee turnover as it implies some potential shared underlying mechanism.
Table 6 also provides a view on how each theme causally affects employee turnover, where themes with a positive delta cause a decrease in employee turnover. As the theme’s score increases, the probability of turnover decreases. The reverse holds for negative deltas. We recognize that some of these results are not fully aligned with findings by other papers, mainly from the managerial and human resources fields. For example, we find Role Clarity to cause employee turnover to increase, which is the opposite effect found in other studies [ 29 ]. These other claims, we note, are not causal. Moreover, such discrepancies are possible already by taking into account that those findings are based on US data while ours on European data. As we argued when motivating Fig. 10 , we believe the interaction between geographical and work variables (such as in the form of country-specific labor laws or the health of its economy) affect employee turnover. Hence, the transportability of these previous results into a European context was not expected.
Overall, Table 6 along with both Fig. 13 and Fig. 14 can be very useful to inform a policy maker as they can serve as evidence for justifying a specific policy intervention. For example, here we would advised for prioritizing policies that foster employee motivation over policies that focus on employee and organization adaptability. Overall, this is a relatively simple XAI method that could be used also by practitioners to go beyond claims on correlation between variables of interest in their models.
7 Conclusions
We had the opportunity to analyze a unique cross-national survey of employee turnover intention, covering 30 European countries. The analytical methodologies adopted followed three perspectives. The first perspective is from the human resource predictive analytics, and it consisted of the comparison of state-of-the-art machine learning predictive models. Logistic Regression (LR) and LightGBM (LGBM) resulted the top performing models. The second perspective is from the eXplainable AI (XAI), consisting in the ranking of the determinants (themes and items) of turnover intention by resorting to feature importance of the predictive models. Moreover, a novel composition of feature importance rankings from repeated cross-validation was devised, consisting of critical difference diagrams. The output of the analysis showed that the themes Sustainable Employability , Employership , and Attendance Stability are within the top-five determinants for both LR and LGBM. From the XAI strand of research, we also adopted partial dependency plots, but with a stronger conclusion than correlation/importance. The third perspective, in fact, is a novel causal approach in support of policy interventions which is rooted in causal structural models. The output confirms those from the second perspective, where highly ranked themes showed PDPs with higher variability than lower ranked themes. The value added from the third perspective here is that we quantify the magnitude and direction for the causal claim \(T \rightarrow Y\) .
Three limitations of the conclusions of our analysis should be highlighted. The first one is concerned with comparison with related work. Due to the specific set of questions and the target respondents of the GEEI survey, it is difficult to compare our results with related works that use other survey data, which cover a different set of questions and/or respondents. The second limitation of our results consists of a weighting of datasets, to overcome selection bias, which is limited to country-specific workforce. Either the dataset under analysis should be representative of the workforce, or a more granular weighting should be used to account for country, gender, industry, and any other contextual feature. The final and third limitation of our results concern the causal claims. Our analysis is based on a specific and by far non-unique causal view of the problem of turnover intention where, for example, variables such as Gender and Education level that belong to the same group node I are considered independent. The interventions carried out to test the causal claim are reliant on the specified causal graph, which limits our results within Fig. 10 .
To conclude, we believe that further interdisciplinary research like this paper can be beneficial for tackling employee turnover. One possible extension would be to collect country’s national statistics to avoid selection bias in survey data or, alternatively, to align the weights of the data to a finer granularity level. Another extension would be to carry out the causal claim tests using a causal graph derived entirely from the data using causal discovery algorithms. In fact, an interesting combination of these two extensions would be to use methods for causal discovery that can account for shifts in the distribution of the data (see, e.g., [ 41 ] and [ 44 ]). All of these we consider for future work.
Consider, for example, the recent wave of workers quitting their jobs during the pandemic due to burn-out. See “Quitting Your Job Never Looked So Fun” and “Why The 2021 ‘Turnover Tsunami’ Is Happening And What Business Leaders Can Do To Prepare” .
https://www.effectory.com
https://www.kaggle.com/pavansubhasht/ibm-hr-analytics-attrition-dataset
https://www.kaggle.com/c/sm/overview
Two items belonging to a same theme are highly correlated (convergence), whilst two items from different themes are almost uncorrelated (discrimination). See https://en.wikipedia.org/wiki/Construct_validity
As a consequence of construct validity, each item belongs to one and only one theme.
We also experimented with a dataset with both themes and items scores, whose predictive performances were close to the items dataset. This is not surprising, since a theme’s score is an aggregation over a subset of items.
https://scikit-learn.org/
https://xgboost.readthedocs.io/
https://lightgbm.readthedocs.io/
https://github.com/dreamquark-ai/tabnet
https://optuna.org/
Tests performed on a PC with Intel 8 cores-16 threads i7-6900K at 3.7 GHz, 128 Gb RAM, and Windows Server 2016 OS. Python version 3.8.5.
Notice that the implementations of DT and LR are single-threaded, while the ones of RF, XGB, LGBM, and TABNET are multi-threaded.
https://ec.europa.eu/eurostat/web/lfs/data/database
https://databank.worldbank.org/reports.aspx?source=2 &series=SL.TLF.CACT.NE.ZS
Given that we focus only on European countries, the attribute Continent is fixed and thus controlled for. We can exclude it from G .
For example, under the causal graph \({\mathcal {G}}\) , \(I \rightarrow W\) implies the causal relationships \(Age \rightarrow Industry\) , \(Gender \rightarrow Industry\) , \(Age \rightarrow Work \; Status\) , \(Gender \rightarrow Work \; Status\) , but not \(Age \rightarrow Gender\) nor \(Gender \rightarrow Age\) .
We recognize that this is a strong assumption, but the alternative would be to drop all themes except T and fit b on that subset of the data, which would have considerable risks of overestimating the effect of T on Y .
To use the proper causal terminology, all themes have the same parents (the incoming edges from the variables in I , G , and W ) and the same child ( Y ). No given theme is the parent or child of any other theme in \(\tau \) .
The clustered map adopts a hierarchical clustering. Therefore, groups can be identified at different levels of granularity.
This under the assumption that the model that is generating the predicted outcomes approximates the “true” relationship between the feature of interest and the outcome variable. This is way [ 63 ] emphasizes the importance of having a good performing model for applying this approach.
To be more precise, ( 2 ) is equivalent to the expectation over ( 1 ), which would allow us to rewrite ( 1 ) in terms of expectations rather than in terms of probabilities and thus formally derive the equivalence between the two.
Here, again, under the assumption that b approximates the “true” where \(b(T) \rightarrow {\hat{Y}}\) contains relevant information concerning \(T \rightarrow Y\) .
Under this assumption, we can apply a simple average.
For example, by estimating the (joint) probability of being a German worker who is also female and has a college degree.
It is common to use the PDP on the training dataset [ 43 , 63 ] and since we are not interested here in testing performance, we use the entire dataset for fitting the model.
Akiba, T., Sano, S., Yanase, T., Ohta, T., Koyama, M.: Optuna: A next-generation hyperparameter optimization framework. In: KDD, pp. 2623–2631. ACM (2019)
Alduayj, S.S., Rajpoot, K.: Predicting employee attrition using machine learning. In: IIT, pp. 93–98. IEEE (2018)
Allen, D.G., Hancock, J.I., Vardaman, J.M., Mckee, D.N.: Analytical mindsets in turnover research. J. Org Behav 35 (S1), S61–S86 (2014)
Article Google Scholar
Allen, D.G., Shanock, L.R.: Perceived organizational support and embeddedness as key mechanisms connecting socialization tactics to commitment and turnover among new employees. J. Org. Behav. 34 (3), 350–369 (2013)
Angrist, J.D., Pischke, J.S.: Mostly Harmless Econometrics. Princeton University Press (2008)
Arik, S.Ö., Pfister, T.: Tabnet: Attentive interpretable tabular learning. In: AAAI, pp. 6679–6687. AAAI Press (2021)
Borisov, V., Leemann, T., Seßler, K., Haug, J., Pawelczyk, M., Kasneci, G.: Deep neural networks and tabular data: A survey. CoRR abs/2110.01889 (2021)
Boyd, K., Eng, K.H., Jr., C.D.P.: Area under the precision-recall curve: Point estimates and confidence intervals. In: ECML/PKDD (3), LNCS , vol. 8190, pp. 451–466. Springer (2013)
Branco, P., Torgo, L., Ribeiro, R.P.: A survey of predictive modeling on imbalanced domains. ACM Comput. Surv. 49 (2), 31:1-50 (2016)
Breiman, L.: Random forests. Mach. Learn. 45 (1), 5–32 (2001)
Breiman, L., Friedman, J.H., Olshen, R.A., Stone, C.J.: Classification and Regression Trees. Wadsworth (1984)
Chen, T., Guestrin, C.: Xgboost: A scalable tree boosting system. In: KDD, pp. 785–794. ACM (2016)
Cohen, G., Blake, R.S., Goodman, D.: Does turnover intention matter? Evaluating the usefulness of turnover intention rate as a predictor of actual turnover rate. Rev. Pub. Person. Adm. 36 (3), 240–263 (2016)
Commission, E.: Joint employment report 2021. https://ec.europa.eu/social/BlobServlet?docId=23156 &langId=en (2021)
Davis, J., Goadrich, M.: The relationship between precision-recall and ROC curves. In: ICML, ACM International Conference Proceeding Series , vol. 148, pp. 233–240. ACM (2006)
Delgado, M.F., Cernadas, E., Barro, S., Amorim, D.G.: Do we need hundreds of classifiers to solve real world classification problems? J. Mach. Learn. Res. 15 (1), 3133–3181 (2014)
MathSciNet MATH Google Scholar
Demsar, J.: Statistical comparisons of classifiers over multiple data sets. J. Mach. Learn. Res. 7 , 1–30 (2006)
DeVellis, R.F.: Scale development: Theory and applications. Sage (2016)
Dietterich, T.: Approximate statistical tests for comparing supervised classification learning algorithms. Neural Comput. 10 , 31895–1923 (1998)
Fallucchi, F., Coladangelo, M., Giuliano, R., Luca, E.W.D.: Predicting employee attrition using machine learning techniques. Computer 9 (4), 86 (2020)
Firth, L., Mellor, D.J., Moore, K.A., Loquet, C.: How can managers reduce employee intention to quit? J. Manag. Psychol. pp. 170–187 (2004)
Friedman, J.H.: Greedy function approximation: A gradient boosting machine. Ann. Stat. 29 (5), 1189–1232 (2001)
Article MathSciNet Google Scholar
Friedman, J.H.: Stochastic gradient boosting. Comput. Stat. Data Anal. 38 (4), 367–378 (2002)
Gabrani, G., Kwatra, A.: Machine learning based predictive model for risk assessment of employee attrition. In: ICCSA (4), Lecture Notes in Computer Science , vol. 10963, pp. 189–201. Springer (2018)
Goodman, A., Mensch, J.M., Jay, M., French, K.E., Mitchell, M.F., Fritz, S.L.: Retention and attrition factors for female certified athletic trainers in the national collegiate athletic association division I football bowl subdivision setting. J. Athl. Train. 45 (3), 287–298 (2010)
Griffeth, R., Hom, P.: Retaining Valued Employees. Sage (2001)
Griffeth, R.W., Hom, P.W., Gaertner, S.: A meta-analysis of antecedents and correlates of employee turnover: Update, moderator tests, and research implications for the next millennium. J. Manag. 26 (3), 463–488 (2000)
Google Scholar
Guidotti, R., Monreale, A., Ruggieri, S., Turini, F., Giannotti, F., Pedreschi, D.: A survey of methods for explaining black box models. ACM Comput. Surv. 51 (5), 93:1–93 (2019)
Hassan, S.: The importance of role clarification in workgroups: Effects on perceived role clarity, work satisfaction, and turnover rates. Public Adm. Rev. 73 (5), 716–725 (2013)
Heneman, H.G., Judge, T.A., Kammeyer-Mueller, J.: Staffing organizations, 9 edn. McGraw-Hill Higher Education (2018)
Hoffman, M., Tadelis, S.: People management skills, employee attrition, and manager rewards: An empirical analysis. J. Polit. Econ. 129 (1), 243–285 (2021)
Hollander, M., Wolfe, D.A., Chicken, E.: Nonparametric Statistical Methods, 3 edn. Wiley (2014)
Holtom, B.C., Mitchell, T.R., Lee, T.W., Eberly, M.B.: Turnover and retention research: a glance at the past, a closer review of the present, and a venture into the future. Acad. Manag. Ann. 2 (1), 231–274 (2008)
Hom, P., Lee, T., Shaw, J., Hausknecht, J.: One hundred years of employee turnover theory and research. J. Appl. Psychol. 102 , 530 (2017)
Hosmer, D.W., Lemeshow, S.: Applied Logistic Regression, 2 edn. Wiley (2000)
Jain, N., Tomar, A., Jana, P.K.: A novel scheme for employee churn problem using multi-attribute decision making approach and machine learning. J. Intell. Inf. Syst. 56 (2), 279–302 (2021)
Ke, G., Meng, Q., Finley, T., Wang, T., Chen, W., Ma, W., Ye, Q., Liu, T.: LightGBM: A highly efficient gradient boosting decision tree. In: NIPS, pp. 3146–3154 (2017)
Keilwagen, J., Grosse, I., Grau, J.: Area under precision-recall curves for weighted and unweighted data. PLoS ONE 9 (3), 1–13 (2014)
Kim, J.H.: Estimating classification error rate: repeated cross-validation, repeated hold-out and bootstrap. Comput. Stat. Data Anal. 53 (11), 3735–3745 (2009)
Kohavi, R.: A study of cross-validation and bootstrap for accuracy estimation and model selection. In: IJCAI, pp. 1137–1145. Morgan Kaufmann (1995)
Magliacane, S., van Ommen, T., Claassen, T., Bongers, S., Versteeg, P., Mooij, J.M.: Causal transfer learning. CoRR abs/1707.06422 (2017)
Mitchell, T.R., Holtom, B.C., Lee, T.W., Sablynski, C.J., Erez, M.: Why people stay: using job embeddedness to predict voluntary turnover. Acad. Manag. J. 44 (6), 1102–1121 (2001)
Molnar, C.: Interpretable Machine Learning (2019). https://christophm.github.io/interpretable-ml-book/
Mooij, J.M., Magliacane, S., Claassen, T.: Joint causal inference from multiple contexts. J. Mach. Learn. Res. 21 , 99:1–99:108 (2020)
Ngo-Henha, P.E.: A review of existing turnover intention theories. Int J Econ. Manag. Eng. 11 , 2760–2767 (2017)
Nijjer, S., Raj, S.: Predictive analytics in human resource management: a hands-on approach. Routledge India (2020)
Pearl, J.: Causality, 2 edn. Cambridge University Press (2009)
Price, J.L.: Reflections on the determinants of voluntary turnover. Int. J. Manpower 22 (7), 600–624 (2001)
Sahakyan, M., Aung, Z., Rahwan, T.: Explainable artificial intelligence for tabular data: A survey. IEEE Access 9 , 135392–135422 (2021)
Salzberger, T., Koller, M.: The direction of the response scale matters - accounting for the unit of measurement. Eur. J. Mark. 53 (5), 871–891 (2019)
Sato, T., Rehmsmeier, M.: Precision-recall plot is more informative than the ROC plot when evaluating binary classifiers on imbalanced datasets. PLoS ONE 10 (3), e0118432 (2015)
Schuurmans, J., Frasincar, F., Cambria, E.: Intent classification for dialogue utterances. IEEE Intell. Syst. 35 (1), 82–88 (2020)
Seidl, T.: Nearest neighbor classification. In: Encyclopedia of Database Systems, pp. 1885–1890. Springer (2009)
Shu, K., Mukherjee, S., Zheng, G., Awadallah, A.H., Shokouhi, M., Dumais, S.T.: Learning with weak supervision for email intent detection. In: SIGIR, pp. 1051–1060. ACM (2020)
Simmons, R.G., Browning, B., Zhang, Y., Sadekar, V.: Learning to predict driver route and destination intent. In: ITSC, pp. 127–132. IEEE (2006)
Sousa-Poza, A., Henneberger, F.: Analyzing job mobility with job turnover intentions: an international comparative study. J. Econ. Issues 38 (1), 113–137 (2004)
Tanova, C., Holtom, B.C.: Using job embeddedness factors to explain voluntary turnover in four European countries. Int. J. Human Res. Manag. 19 , 1553–1568 (2008)
Wang, S., Hu, L., Wang, Y., Sheng, Q.Z., Orgun, M.A., Cao, L.: Intention nets: Psychology-inspired user choice behavior modeling for next-basket prediction. In: AAAI, pp. 6259–6266. AAAI Press (2020)
Wang, S., Hu, L., Wang, Y., Sheng, Q.Z., Orgun, M.A., Cao, L.: Intention2basket: A neural intention-driven approach for dynamic next-basket planning. In: IJCAI, pp. 2333–2339. ijcai.org (2020)
William Lee, T., Burch, T.C., Mitchell, T.R.: The story of why we stay: A review of job embeddedness. Annu. Rev. Organ. Psych. Organ. Behav. 1 (1), 199–216 (2014)
Wunder, R.S., Dougherty, T.W., Welsh, M.A.: A casual model of role stress and employee turnover. In: Academy of Management Proceedings, vol. 1982, pp. 297–301 (1982)
Wynen, J., Dooren, W.V., Mattijs, J., Deschamps, C.: Linking turnover to organizational performance: the role of process conformance. Public Manag. Rev. 21 (5), 669–685 (2019)
Zhao, Q., Hastie, T.: Causal interpretations of black-box models. J. Bus. Econ. Stat. 39 (1), 272–281 (2021)
Download references
Acknowledgements
The work of J. M. Alvarez and S. Ruggieri has received funding from the European Union’s Horizon 2020 research and innovation programme under Marie Sklodowska-Curie Actions (grant agreement number 860630) for the project “NoBIAS - Artificial Intelligence without Bias”. This work reflects only the authors’ views and the European Research Executive Agency (REA) is not responsible for any use that may be made of the information it contains.
Open access funding provided by Università di Pisa within the CRUI-CARE Agreement.
Author information
Authors and affiliations.
Effectory Global, Amsterdam, Netherlands
Matilde Lazzari
Scuola Normale Superiore, Pisa, Italy
Jose M. Alvarez
University of Pisa, Pisa, Italy
Jose M. Alvarez & Salvatore Ruggieri
You can also search for this author in PubMed Google Scholar
Corresponding author
Correspondence to Salvatore Ruggieri .
Ethics declarations
Conflict of interest.
M. Lazzari declares that she is an employee of Effectory Global. J. M. Alvarez and S. Ruggieri declare that they have no conflict of interest.
Additional information
Publisher's note.
Springer Nature remains neutral with regard to jurisdictional claims in published maps and institutional affiliations.
Rights and permissions
Open Access This article is licensed under a Creative Commons Attribution 4.0 International License, which permits use, sharing, adaptation, distribution and reproduction in any medium or format, as long as you give appropriate credit to the original author(s) and the source, provide a link to the Creative Commons licence, and indicate if changes were made. The images or other third party material in this article are included in the article’s Creative Commons licence, unless indicated otherwise in a credit line to the material. If material is not included in the article’s Creative Commons licence and your intended use is not permitted by statutory regulation or exceeds the permitted use, you will need to obtain permission directly from the copyright holder. To view a copy of this licence, visit http://creativecommons.org/licenses/by/4.0/ .
Reprints and permissions
About this article
Lazzari, M., Alvarez, J.M. & Ruggieri, S. Predicting and explaining employee turnover intention. Int J Data Sci Anal 14 , 279–292 (2022). https://doi.org/10.1007/s41060-022-00329-w
Download citation
Received : 11 July 2021
Accepted : 15 April 2022
Published : 23 May 2022
Issue Date : September 2022
DOI : https://doi.org/10.1007/s41060-022-00329-w
Share this article
Anyone you share the following link with will be able to read this content:
Sorry, a shareable link is not currently available for this article.
Provided by the Springer Nature SharedIt content-sharing initiative
- Employee turnover
- Predictive models
- EXplainable AI (XAI)
- Structural causal models
- Find a journal
- Publish with us
- Track your research
Academia.edu no longer supports Internet Explorer.
To browse Academia.edu and the wider internet faster and more securely, please take a few seconds to upgrade your browser .
Enter the email address you signed up with and we'll email you a reset link.
- We're Hiring!
- Help Center
RESEARCH PROPOSAL (moffat Pihelo) HIGH TURNOVER (3)
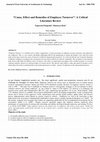
- We're Hiring!
- Help Center
- Find new research papers in:
- Health Sciences
- Earth Sciences
- Cognitive Science
- Mathematics
- Computer Science
- Academia ©2024
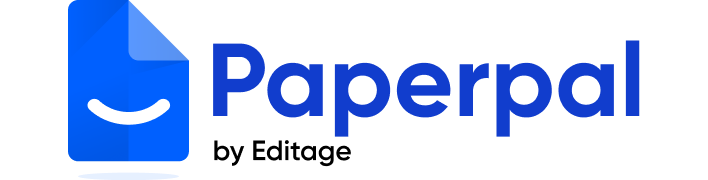
How to Write a Research Proposal: (with Examples & Templates)
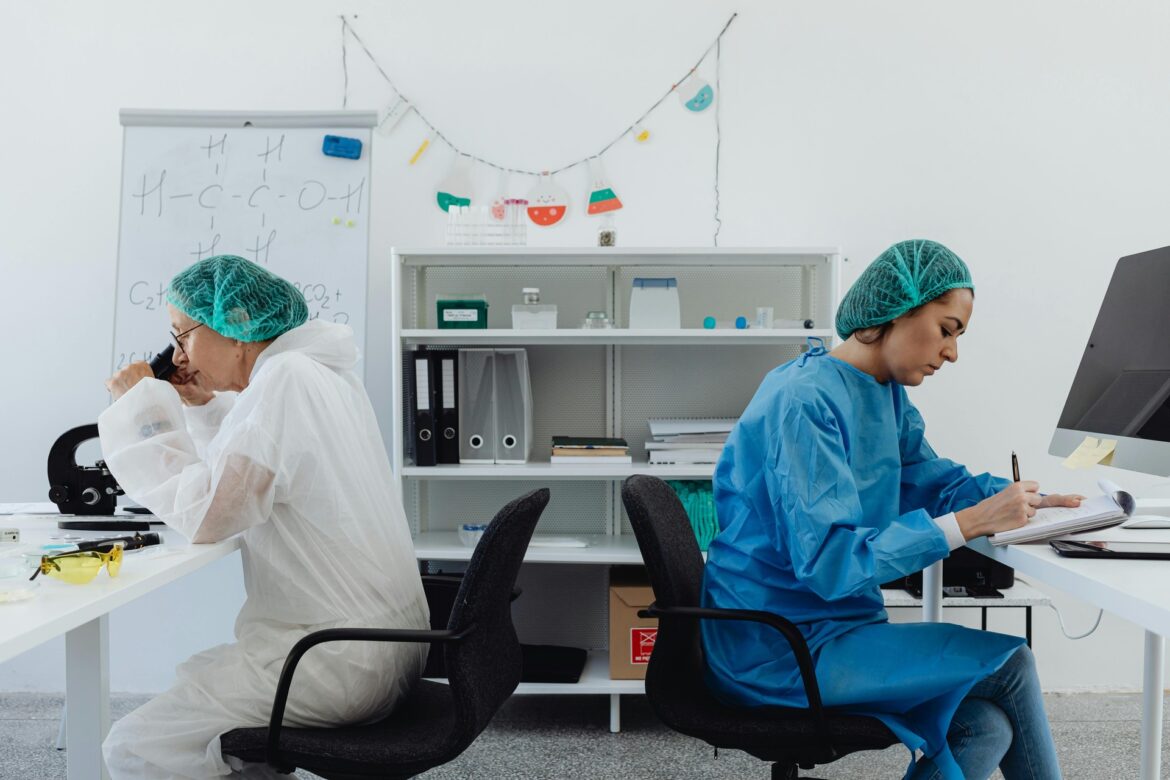
Table of Contents
Before conducting a study, a research proposal should be created that outlines researchers’ plans and methodology and is submitted to the concerned evaluating organization or person. Creating a research proposal is an important step to ensure that researchers are on track and are moving forward as intended. A research proposal can be defined as a detailed plan or blueprint for the proposed research that you intend to undertake. It provides readers with a snapshot of your project by describing what you will investigate, why it is needed, and how you will conduct the research.
Your research proposal should aim to explain to the readers why your research is relevant and original, that you understand the context and current scenario in the field, have the appropriate resources to conduct the research, and that the research is feasible given the usual constraints.
This article will describe in detail the purpose and typical structure of a research proposal , along with examples and templates to help you ace this step in your research journey.
What is a Research Proposal ?
A research proposal¹ ,² can be defined as a formal report that describes your proposed research, its objectives, methodology, implications, and other important details. Research proposals are the framework of your research and are used to obtain approvals or grants to conduct the study from various committees or organizations. Consequently, research proposals should convince readers of your study’s credibility, accuracy, achievability, practicality, and reproducibility.
With research proposals , researchers usually aim to persuade the readers, funding agencies, educational institutions, and supervisors to approve the proposal. To achieve this, the report should be well structured with the objectives written in clear, understandable language devoid of jargon. A well-organized research proposal conveys to the readers or evaluators that the writer has thought out the research plan meticulously and has the resources to ensure timely completion.
Purpose of Research Proposals
A research proposal is a sales pitch and therefore should be detailed enough to convince your readers, who could be supervisors, ethics committees, universities, etc., that what you’re proposing has merit and is feasible . Research proposals can help students discuss their dissertation with their faculty or fulfill course requirements and also help researchers obtain funding. A well-structured proposal instills confidence among readers about your ability to conduct and complete the study as proposed.
Research proposals can be written for several reasons:³
- To describe the importance of research in the specific topic
- Address any potential challenges you may encounter
- Showcase knowledge in the field and your ability to conduct a study
- Apply for a role at a research institute
- Convince a research supervisor or university that your research can satisfy the requirements of a degree program
- Highlight the importance of your research to organizations that may sponsor your project
- Identify implications of your project and how it can benefit the audience
What Goes in a Research Proposal?
Research proposals should aim to answer the three basic questions—what, why, and how.
The What question should be answered by describing the specific subject being researched. It should typically include the objectives, the cohort details, and the location or setting.
The Why question should be answered by describing the existing scenario of the subject, listing unanswered questions, identifying gaps in the existing research, and describing how your study can address these gaps, along with the implications and significance.
The How question should be answered by describing the proposed research methodology, data analysis tools expected to be used, and other details to describe your proposed methodology.
Research Proposal Example
Here is a research proposal sample template (with examples) from the University of Rochester Medical Center. 4 The sections in all research proposals are essentially the same although different terminology and other specific sections may be used depending on the subject.
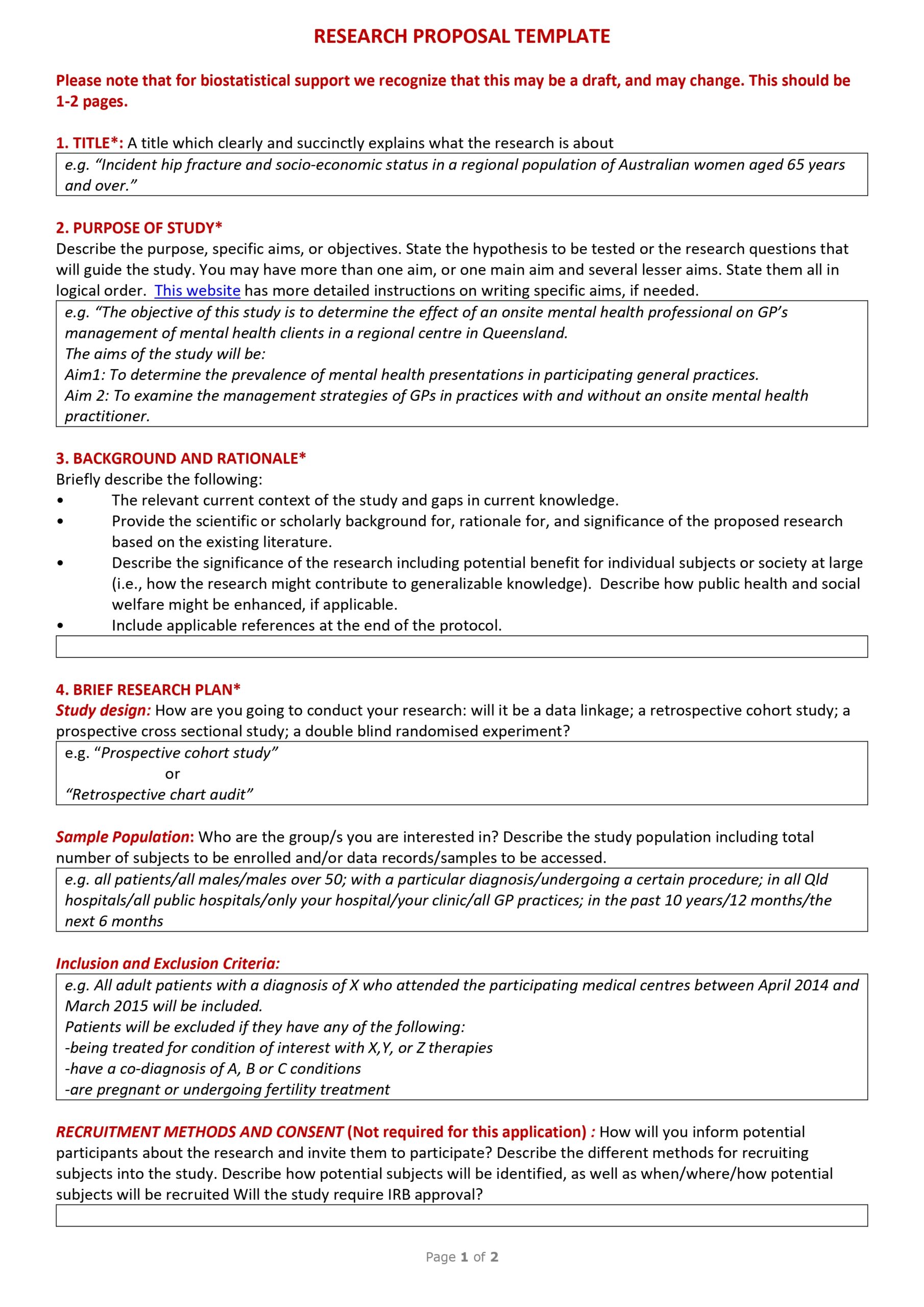
Structure of a Research Proposal
If you want to know how to make a research proposal impactful, include the following components:¹
1. Introduction
This section provides a background of the study, including the research topic, what is already known about it and the gaps, and the significance of the proposed research.
2. Literature review
This section contains descriptions of all the previous relevant studies pertaining to the research topic. Every study cited should be described in a few sentences, starting with the general studies to the more specific ones. This section builds on the understanding gained by readers in the Introduction section and supports it by citing relevant prior literature, indicating to readers that you have thoroughly researched your subject.
3. Objectives
Once the background and gaps in the research topic have been established, authors must now state the aims of the research clearly. Hypotheses should be mentioned here. This section further helps readers understand what your study’s specific goals are.
4. Research design and methodology
Here, authors should clearly describe the methods they intend to use to achieve their proposed objectives. Important components of this section include the population and sample size, data collection and analysis methods and duration, statistical analysis software, measures to avoid bias (randomization, blinding), etc.
5. Ethical considerations
This refers to the protection of participants’ rights, such as the right to privacy, right to confidentiality, etc. Researchers need to obtain informed consent and institutional review approval by the required authorities and mention this clearly for transparency.
6. Budget/funding
Researchers should prepare their budget and include all expected expenditures. An additional allowance for contingencies such as delays should also be factored in.
7. Appendices
This section typically includes information that supports the research proposal and may include informed consent forms, questionnaires, participant information, measurement tools, etc.
8. Citations
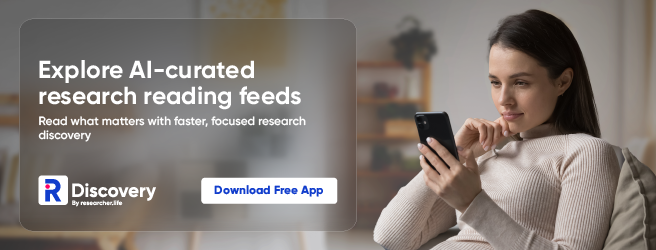
Important Tips for Writing a Research Proposal
Writing a research proposal begins much before the actual task of writing. Planning the research proposal structure and content is an important stage, which if done efficiently, can help you seamlessly transition into the writing stage. 3,5
The Planning Stage
- Manage your time efficiently. Plan to have the draft version ready at least two weeks before your deadline and the final version at least two to three days before the deadline.
- What is the primary objective of your research?
- Will your research address any existing gap?
- What is the impact of your proposed research?
- Do people outside your field find your research applicable in other areas?
- If your research is unsuccessful, would there still be other useful research outcomes?
The Writing Stage
- Create an outline with main section headings that are typically used.
- Focus only on writing and getting your points across without worrying about the format of the research proposal , grammar, punctuation, etc. These can be fixed during the subsequent passes. Add details to each section heading you created in the beginning.
- Ensure your sentences are concise and use plain language. A research proposal usually contains about 2,000 to 4,000 words or four to seven pages.
- Don’t use too many technical terms and abbreviations assuming that the readers would know them. Define the abbreviations and technical terms.
- Ensure that the entire content is readable. Avoid using long paragraphs because they affect the continuity in reading. Break them into shorter paragraphs and introduce some white space for readability.
- Focus on only the major research issues and cite sources accordingly. Don’t include generic information or their sources in the literature review.
- Proofread your final document to ensure there are no grammatical errors so readers can enjoy a seamless, uninterrupted read.
- Use academic, scholarly language because it brings formality into a document.
- Ensure that your title is created using the keywords in the document and is neither too long and specific nor too short and general.
- Cite all sources appropriately to avoid plagiarism.
- Make sure that you follow guidelines, if provided. This includes rules as simple as using a specific font or a hyphen or en dash between numerical ranges.
- Ensure that you’ve answered all questions requested by the evaluating authority.
Key Takeaways
Here’s a summary of the main points about research proposals discussed in the previous sections:
- A research proposal is a document that outlines the details of a proposed study and is created by researchers to submit to evaluators who could be research institutions, universities, faculty, etc.
- Research proposals are usually about 2,000-4,000 words long, but this depends on the evaluating authority’s guidelines.
- A good research proposal ensures that you’ve done your background research and assessed the feasibility of the research.
- Research proposals have the following main sections—introduction, literature review, objectives, methodology, ethical considerations, and budget.
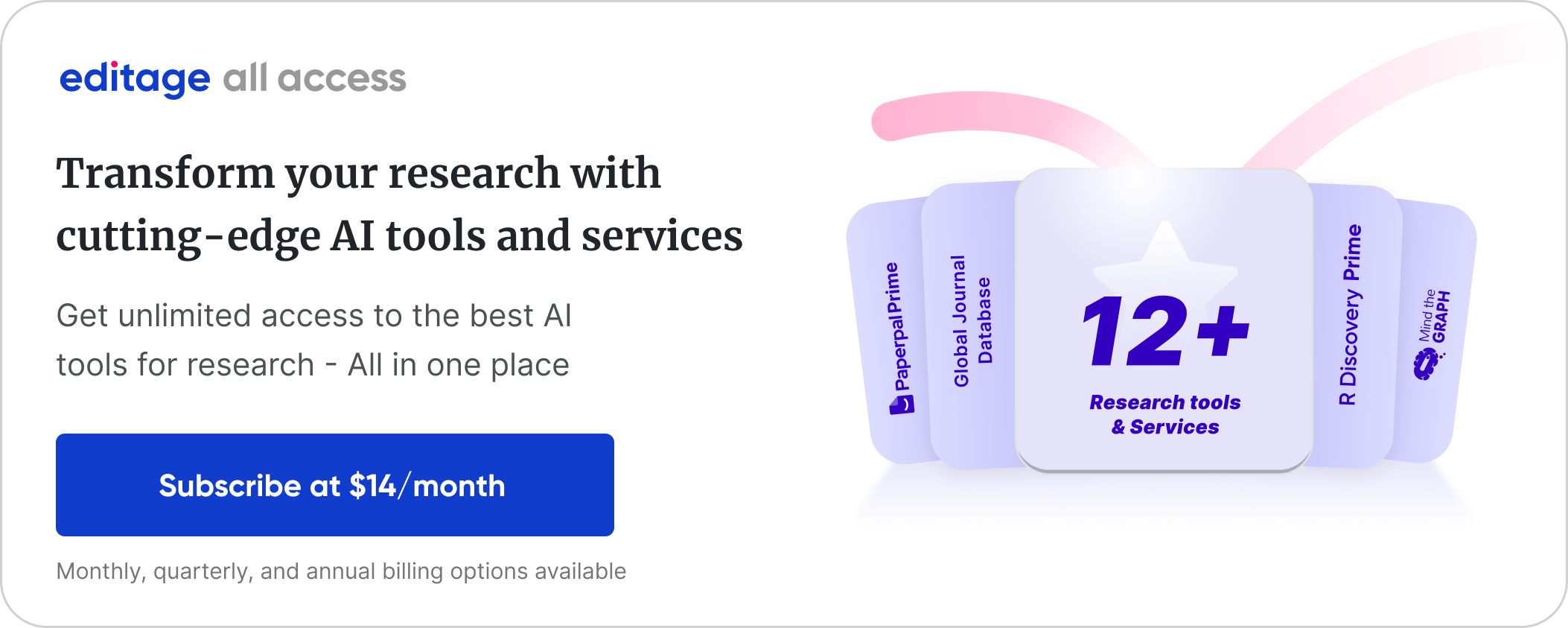
Frequently Asked Questions
Q1. How is a research proposal evaluated?
A1. In general, most evaluators, including universities, broadly use the following criteria to evaluate research proposals . 6
- Significance —Does the research address any important subject or issue, which may or may not be specific to the evaluator or university?
- Content and design —Is the proposed methodology appropriate to answer the research question? Are the objectives clear and well aligned with the proposed methodology?
- Sample size and selection —Is the target population or cohort size clearly mentioned? Is the sampling process used to select participants randomized, appropriate, and free of bias?
- Timing —Are the proposed data collection dates mentioned clearly? Is the project feasible given the specified resources and timeline?
- Data management and dissemination —Who will have access to the data? What is the plan for data analysis?
Q2. What is the difference between the Introduction and Literature Review sections in a research proposal ?
A2. The Introduction or Background section in a research proposal sets the context of the study by describing the current scenario of the subject and identifying the gaps and need for the research. A Literature Review, on the other hand, provides references to all prior relevant literature to help corroborate the gaps identified and the research need.
Q3. How long should a research proposal be?
A3. Research proposal lengths vary with the evaluating authority like universities or committees and also the subject. Here’s a table that lists the typical research proposal lengths for a few universities.
Arts programs | 1,000-1,500 | |
University of Birmingham | Law School programs | 2,500 |
PhD | 2,500 | |
2,000 | ||
Research degrees | 2,000-3,500 |
Q4. What are the common mistakes to avoid in a research proposal ?
A4. Here are a few common mistakes that you must avoid while writing a research proposal . 7
- No clear objectives: Objectives should be clear, specific, and measurable for the easy understanding among readers.
- Incomplete or unconvincing background research: Background research usually includes a review of the current scenario of the particular industry and also a review of the previous literature on the subject. This helps readers understand your reasons for undertaking this research because you identified gaps in the existing research.
- Overlooking project feasibility: The project scope and estimates should be realistic considering the resources and time available.
- Neglecting the impact and significance of the study: In a research proposal , readers and evaluators look for the implications or significance of your research and how it contributes to the existing research. This information should always be included.
- Unstructured format of a research proposal : A well-structured document gives confidence to evaluators that you have read the guidelines carefully and are well organized in your approach, consequently affirming that you will be able to undertake the research as mentioned in your proposal.
- Ineffective writing style: The language used should be formal and grammatically correct. If required, editors could be consulted, including AI-based tools such as Paperpal , to refine the research proposal structure and language.
Thus, a research proposal is an essential document that can help you promote your research and secure funds and grants for conducting your research. Consequently, it should be well written in clear language and include all essential details to convince the evaluators of your ability to conduct the research as proposed.
This article has described all the important components of a research proposal and has also provided tips to improve your writing style. We hope all these tips will help you write a well-structured research proposal to ensure receipt of grants or any other purpose.
References
- Sudheesh K, Duggappa DR, Nethra SS. How to write a research proposal? Indian J Anaesth. 2016;60(9):631-634. Accessed July 15, 2024. https://www.ncbi.nlm.nih.gov/pmc/articles/PMC5037942/
- Writing research proposals. Harvard College Office of Undergraduate Research and Fellowships. Harvard University. Accessed July 14, 2024. https://uraf.harvard.edu/apply-opportunities/app-components/essays/research-proposals
- What is a research proposal? Plus how to write one. Indeed website. Accessed July 17, 2024. https://www.indeed.com/career-advice/career-development/research-proposal
- Research proposal template. University of Rochester Medical Center. Accessed July 16, 2024. https://www.urmc.rochester.edu/MediaLibraries/URMCMedia/pediatrics/research/documents/Research-proposal-Template.pdf
- Tips for successful proposal writing. Johns Hopkins University. Accessed July 17, 2024. https://research.jhu.edu/wp-content/uploads/2018/09/Tips-for-Successful-Proposal-Writing.pdf
- Formal review of research proposals. Cornell University. Accessed July 18, 2024. https://irp.dpb.cornell.edu/surveys/survey-assessment-review-group/research-proposals
- 7 Mistakes you must avoid in your research proposal. Aveksana (via LinkedIn). Accessed July 17, 2024. https://www.linkedin.com/pulse/7-mistakes-you-must-avoid-your-research-proposal-aveksana-cmtwf/
Paperpal is a comprehensive AI writing toolkit that helps students and researchers achieve 2x the writing in half the time. It leverages 21+ years of STM experience and insights from millions of research articles to provide in-depth academic writing, language editing, and submission readiness support to help you write better, faster.
Get accurate academic translations, rewriting support, grammar checks, vocabulary suggestions, and generative AI assistance that delivers human precision at machine speed. Try for free or upgrade to Paperpal Prime starting at US$19 a month to access premium features, including consistency, plagiarism, and 30+ submission readiness checks to help you succeed.
Experience the future of academic writing – Sign up to Paperpal and start writing for free!
Related Reads:
- How to Paraphrase Research Papers Effectively
- How to Cite Social Media Sources in Academic Writing?
- What is the Importance of a Concept Paper and How to Write It
APA format: Basic Guide for Researchers
The future of academia: how ai tools are changing the way we do research, you may also like, the ai revolution: authors’ role in upholding academic..., the future of academia: how ai tools are..., how to choose a dissertation topic, how to write a phd research proposal, how to write an academic paragraph (step-by-step guide), five things authors need to know when using..., 7 best referencing tools and citation management software..., maintaining academic integrity with paperpal’s generative ai writing..., research funding basics: what should a grant proposal....
- Open access
- Published: 01 August 2024
The relationship of perceived nurse manager competence with job satisfaction and turnover intention among clinical nurses: an analytical cross-sectional study
- Alireza Mirzaei ORCID: orcid.org/0000-0003-4242-2108 1 , 2 ,
- Reza Imashi ORCID: orcid.org/0000-0002-7204-3603 3 ,
- Roghayeh Yaghoobi Saghezchi ORCID: orcid.org/0009-0005-3119-2193 3 ,
- Mohammad Javad Jafari ORCID: orcid.org/0009-0007-5888-2666 1 &
- Reza Nemati-Vakilabad ORCID: orcid.org/0000-0002-8016-8625 4 , 5
BMC Nursing volume 23 , Article number: 528 ( 2024 ) Cite this article
Metrics details
Nursing managers have the potential to significantly impact the outcomes of nurses, patients, and organizations. Their competencies for effective performance are crucial. The global nursing workforce is facing a severe shortage of nurses. Dissatisfaction with management is one of the most important reasons for nurses’ inclination to leave their jobs. Therefore, this study examines the relationship between nursing managers’ competencies, job satisfaction and intention to leave among clinical nurses.
An analytical cross-sectional study was conducted on 354 nurses in five hospitals affiliated with Ardabil University of Medical Sciences from May to August 2023. This research used web-based software to design demographic information forms, nurse manager competency scale, turnover intention, and single-item job satisfaction questionnaires. The significance level for the study was set at p < 0.05.
This study revealed that participants had an average score of 3.06 out of 5 in perceived nurse manager competence. Only one-fourth of the participants (25.7%) were satisfied with their current job, and less than half (46.9%) intended to leave. Employee support and development, change and resource management, supervision and quality monitoring, and personal mastery positively influenced nurses’ job satisfaction. These competencies hurt employees’ intention to leave their jobs.
Conclusions
The research findings indicate that nursing managers in Iran have lower competency than in previous studies. Developing and strengthening these competencies is very important, as it significantly improves job satisfaction and reduces nurses’ tendency to leave their jobs. Additionally, the results show that nursing managers who excel in supporting and developing staff, resource management, quality supervision, and personal mastery positively impact nurses’ job satisfaction. However, these competencies can also help reduce employees’ intention to leave their jobs. Retaining and maintaining nurses in the healthcare field is of utmost importance.
Peer Review reports
Introduction
Nurse managers play a critical role in overseeing work units and directly supporting nursing staff to tackle various healthcare challenges [ 1 , 2 ]. Their leadership styles can profoundly influence nurse, patient, and organizational outcomes, with positive and negative implications [ 2 ]. A systematic review by Akbiyik et al. highlighted that management approaches focused on building relationships can improve patient outcomes and higher care quality [ 3 ]. Furthermore, previous research has established a strong link between leadership styles and management practices, vital for employee satisfaction and retention [ 4 , 5 ]. Effective perceived nurse manager competence—the specific skills, behaviors, and characteristics necessary for successful performance—are essential for fostering a productive work environment. These competencies encompass various elements, including communication, decision-making, and emotional intelligence, collectively enhance patient care quality [ 6 ]. Cummings et al. conducted a systematic review that revealed significant associations between different leadership styles and key nurse outcomes, such as productivity, job satisfaction, and employee retention; by understanding and enhancing the competencies of nurse managers, this study aims to address the pressing clinical and social issues related to workforce stability and patient care quality in healthcare settings [ 7 ]. In addition, the results of Shojaei et al.‘s study in northwest Iran (Tabriz) showed a significant relationship between nursing managers’ leadership and nurses’ job satisfaction, even though nursing managers considered their leadership and management styles collaborative and consultative. However, the opinion of the nurses working in the therapeutic centers was not like this, and they described their leadership and management style as autocratic [ 8 ].
The current situation of the global nursing workforce is characterized by a critical shortage of nurses, an increasing number of nurses retiring in the next few years, and an increasing proportion of nurses leaving and planning to leave their organizations in the next few years [ 9 , 10 ]. Evidence supports the relationship between the quality of the practice environment and nurse outcomes; management support is one of the dimensions underlying the structure of the practice environment [ 11 , 12 ]. The nurses’ work environment, which encompasses organizational and social elements, serves as the backdrop for the patient care provided by nurses. The work environment is regarded as a crucial factor for the success of any healthcare organization that aims to maintain good standing and compete effectively in achieving organizational and employee goals [ 13 ]. Researchers examined the impact of the work environment on nurses’ turnover intention. They found that “dissatisfaction with management” was the top reason for nurses’ intention to quit their jobs and emphasized the need to explore the competencies of nurse managers further and how they impact the performance of nurses in clinical settings [ 6 , 14 ]. Additionally, high turnover rates among new nurse graduates are a significant concern in healthcare [ 15 ]. This is due to the challenging transition from nursing school to clinical practice, lack of support, and emotional strain [ 16 ].
The global nursing workforce faces a critical shortage, exacerbated by an increasing number of retirements and a rising proportion of nurses planning to leave their organizations in the coming years [ 8 , 9 ]. Research has demonstrated a strong relationship between the quality of the practice environment and nurse outcomes, with management support being a key dimension of this environment [ 10 , 11 ]. A positive work environment, which includes both organizational and social elements, is essential for effective patient care and the overall success of healthcare organizations [ 12 ].
Dissatisfaction with management has emerged as the leading reason for nurses’ intentions to quit, highlighting the urgent need to explore the competencies of nurse managers and their impact on nursing performance in clinical settings [ 6 , 13 ]. Effective perceived nurse manager competence —such as communication, leadership, and emotional intelligence—is crucial for fostering a supportive work environment that can mitigate turnover intentions among nursing staff. Additionally, high turnover rates among newly graduated nurses pose a significant challenge, often stemming from difficulties in transitioning from academic settings to clinical practice, insufficient support, and emotional strain [ 14 , 15 ]. Addressing these issues through enhanced perceived nurse manager competence may lead to improved retention and better patient care outcomes.
The current body of research presents compelling evidence that nurse managers with strong management skills can greatly empower their nursing staff. This empowerment is associated with various positive outcomes, such as increased job satisfaction [ 17 , 18 ], heightened professional commitment [ 19 ], greater work participation [ 20 ], improved safety performance [ 21 ], and the encouragement of innovative work behaviors [ 22 ]. However, there hasn’t been sufficient exploration of how Dissatisfaction with management has emerged as the leading reason for nurses’ intentions to quit, highlighting the urgent need to explore the competencies of nurse managers and their impact on nursing performance in clinical settings [ 6 , 13 ]. Effective perceived nurse manager competence—such as communication, leadership, and emotional intelligence—are crucial for fostering a supportive work environment that can mitigate turnover intentions among nursing staff. Additionally, high turnover rates among newly graduated nurses pose a significant challenge, often stemming from difficulties in transitioning from academic settings to clinical practice, insufficient support, and emotional strain [ 14 , 15 ]. Addressing these issues through enhanced perceived nurse manager competence may lead to improved retention and better patient care outcomes.
impact the work outcomes of clinical nurses in the specific context of Iran. This knowledge gap is especially significant considering the challenges currently facing the nursing workforce in Iran. With a critical shortage of nurses, an aging nursing population, and a growing number of nurses expressing intentions to leave their organizations and the profession, it is more important than ever to understand the factors contributing to job satisfaction and turnover intention. Existing literature indicates that factors such as management support, workplace culture, and professional development opportunities play crucial roles in influencing nurses’ job satisfaction and retention rates.
Our study explores how perceived nurse manager competence relate to job satisfaction and turnover intention among clinical nurses in Iran. By addressing this gap in the literature, we seek to underscore the necessity of effective nurse management in promoting workforce stability and enhancing patient care outcomes within the Iranian healthcare system. This research has the potential to significantly improve nurses’ working conditions and the quality of patient care in Iran.
An analytical cross-sectional study was conducted in Ardabil, northwest of Iran, following the Strengthening the Reporting of Observational Studies in Epidemiology (STROBE) guidelines [ 23 ].
Setting and sample
Data was collected from May to August 2023 using a simple random sampling technique. The study involved clinical nurses from five educational-therapeutic hospitals affiliated with Ardabil University of Medical Sciences. The inclusion and exclusion criteria were carefully considered, ensuring a comprehensive and well-planned study. Inclusion criteria included a willingness to participate, a minimum of six months of work experience, and no history of hearing disorders, neurological conditions, or psychiatric diseases. Additionally, participants must not hold chief or head nurse positions, as the dynamics and relationships of staff in leadership roles may differ significantly from those of general staff nurses, potentially influencing the study’s outcomes. Exclusion criteria included individuals with leadership responsibilities that could affect their perspectives on job satisfaction and turnover intention. Sample size calculation was done by the formula: n = 1.96 2 × p × (1-p)/ d 2 , where p = 0.5 and d = 0.05 indicated the acceptable margin of error (5.0%); this formula required 382 participants. Considering a 10% non-response rate, the final sample size was determined to be 420 participants. The sample attrition rate in this study was 15.7%, where 66 incomplete questionnaires were removed due to data deficiency. Finally, data from 354 samples were analyzed (Fig. 1 ).
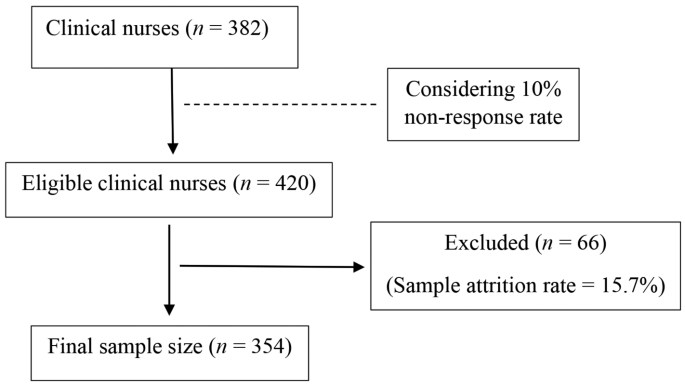
Flow chart of the study sample selection
Data collection
The researchers used a demographic information form and the Nurse Manager Competency Scale to collect data. Additionally, two single-item measures were used to assess job satisfaction and turnover intention. The questionnaires were designed using web-based software and sent to clinical nurses through email and virtual messengers (WhatsApp, Telegram, e.g.). The nurses then completed the questionnaires through a self-administered form.
Demographic information form
The study collected demographic information from clinical nurses using a structured form that included six closed-ended questions: age, working experience, gender, education level, marital status, and unit.
All the participants involved full-time clinical nurses whose employment status could affect their commitment to professional development and innovative practices. Participants had diverse educational levels, with many holding advanced degrees such as BSN or MSN, which could indicate a greater familiarity with innovative practices and a proactive approach to professional development. Their varied working experiences may influence their perspectives on innovation in clinical settings, with more experienced nurses likely bringing different insights than newly qualified ones. Additionally, participants were assigned to various clinical units (e.g., intensive care, emergency, pediatrics), potentially shaping their experiences and exposure to innovative practices based on the unit’s culture and support for new ideas. These factors highlight the possible influence of demographic characteristics on the attitudes and engagement of clinical nurses in their professional roles.
Nurse manager competency scale
The Nurse Manager Competency Scale used in the study was developed by Choi et al. [ 6 ]. This scale includes 60 items that capture five domains of perceived nurse manager competence, which are: (1) Staff advocacy and development (12 items), which evaluated the competencies of advocating for the interests of staff and nurturing their potential for development; (2) Team communication and collaboration (15 items), which evaluated the competencies necessary to create united teams by promoting effective communication and cooperation among team members; (3) Change and resource management (10 items), which evaluated the competencies involved in effectively managing and adapting to changes, as well as optimizing resource allocation; (4) Quality monitoring and pursuance (11 items), which evaluated the competencies for safeguarding the quality of care and cultivating a quality culture in the work unit; (5) Personal mastery (12 items), which evaluated the competencies related to self-knowledge, internal attributes, purpose, and vision. Participants rated their nurse managers’ competencies using a 5-point Likert scale from 1 (strongly disagree) to 5 (strongly agree). Average scores were calculated for each subscale and overall scale. Higher scores indicate a more positive perceived nurse manager competence and vice versa. The instrument had a scale-level content validity index (S-CVI) of 0.94, indicating high relevance and clarity. Internal consistency coefficients were calculated using Cronbach’s alpha to assess the extent to which the items measure the same construct. An internal consistency coefficient of 0.96 was obtained for the overall scale, indicating that the instrument is reliable.
Validation of the nurse manager competency scale
In the study, the Nurse Manager Competency Scale was translated using the Forward-Backward method, and its psychometric properties were tested among 180 clinical nurses. Content validity was evaluated using a qualitative approach based on the opinions of a panel of experts, including ten nursing faculty members. The experts stated that the questionnaire was suitable regarding wording, item allocation, and scaling. Necessary corrections were made based on brief grammatical suggestions at this stage. Confirmatory factor analysis (CFA) was used to measure and determine construct validity. The sample size at this stage was estimated based on the ratio of participants to items 3:1 [ 24 ]. Maximum likelihood estimation (MLE) was used to estimate the parameters. The fit indices of the model were evaluated using the following parameters: χ 2 /df ≤ 3, Root Mean Square Error of Approximation (RMSEA) ≤ 0.08 [ 25 ], Goodness of Fit Index (GFI) ≥ 0.90, Comparative Fit Index (CFI) ≥ 0.90, Tucker-Lewis Index (TLI) ≥ 0.90, Normal Fit Index (NFI) ≥ 0.90 [ 26 ].
The factor loading of all items was more than 0.3 ( p < 0.001), and CFA results for the 5-factor model showed that all latent factors have acceptable correlation coefficients with related items. Additionally, all relationships between dimensions and their items were significant, and no heterogeneity existed. The goodness of fit indices in confirmatory factor analysis showed acceptable values: χ 2 /df = 2.112, RMSEA = 0.061, GFI = 0.953, CFI = 0.947, TLI = 0.969, and NFI = 0.917. These results suggest that the desired model and its constituent concepts are acceptable and confirmed by five dimensions in clinical nurses.
In the study, the internal consistency of the Nurse Manager Competency Scale was evaluated using Cronbach’s alpha coefficient (α). A value greater than 0.7 was considered acceptable [ 27 ], and the overall Cronbach’s alpha was 0.98. The five dimensions of the scale varied between 0.92 and 0.98. To assess the test-retest reliability of the questionnaire, data were collected from 30 clinical nurses over a two-week period, and the Intraclass Correlation Coefficient (ICC) was calculated. In this study, the minimum ICC value was considered to be 0.75 [ 28 ], and the ICC over two weeks was 0.87. These results suggest that the Nurse Manager Competency Scale is a reliable and valid tool for assessing the perceived nurse manager competence.
Job satisfaction and turnover intention
The study asked participants to rate their job satisfaction and intention to leave their current workplace using a 5-point Likert scale. The scale ranged from 1 (most dissatisfied/least intended to leave) to 5 (most satisfied/most intended to leave). A higher score on the job satisfaction measure indicated a higher level of job satisfaction, and vice versa. It was postulated that job satisfaction positively relates to perceived nurse manager competence. On the other hand, a higher score on the turnover intention measure indicated a higher level of intention to leave the current workplace, and vice versa. It was postulated that turnover intention is negatively related to the nurse manager competencies.
Data analysis
The researchers used IBM SPSS Statistics for Windows, version 26.0 (IBM Corp., Armonk, NY, USA) to analyze the data in the study. They used descriptive statistics (frequency, percentage, mean, and standard deviation) to summarize demographic and work-related data, perceived nurse manager competencies, and nurse outcome variables (job satisfaction and turnover intention). Pearson’s correlation coefficient was used to investigate the relationship between perceived nurse manager competence, job satisfaction, and turnover intention. Before performing the statistical tests, the normality of the data distribution was checked using the Kolmogorov-Smirnov one-sample test [ 29 ]. Multiple linear regression analysis were conducted to examine the predictors of job satisfaction and turnover intention while the demographic characteristics of the participants were controlled. Before using multiple linear regression, we checked for multicollinearity using the variance inflation factor (VIF) and tolerance. Generally, if the tolerance value is less than 0.1 and the VIF value is greater than five, it indicates an issue with multicollinearity. None of the variables in this study exhibited significant multicollinearity based on these measures [ 30 ]. Confirmatory factor analysis was performed using AMOS software, version 24.0 (IBM Corp., Armonk, NY, USA). The significance level for statistical analysis was p < 0.05.
Ethical considerations
The Research Ethics Committee of Ardabil University of Medical Sciences has approved this study proposal with the ethics code IR.ARUMS.REC.1402.063. Before participating, participants were informed about the study’s objectives and methods, and written informed consent was obtained from them. We want to highlight that the Declaration of Helsinki, an internationally recognized set of ethical principles, guided all procedures. Participation in this study was voluntary, and participants were told that they could withdraw from the study at any stage. We implemented strict data management protocols to protect personal information, including email addresses. Email addresses were collected solely for survey communication and stored securely. Access to this information was limited to authorized personnel only, and all data was anonymized to prevent identification of participants. Ethical considerations, such as confidentiality, anonymity, and information privacy, were strictly observed throughout the study.
In total, 354 nurses participated in this study, of whom more than half were male ( n = 186, 52.5%). The participants’ mean (SD) age was 31.36 (4.03) years. Almost half of the participants were single ( n = 179, 50.6%), and most had a bachelor’s degree ( n = 301, 85.0%). The demographic characteristics of the participants in this study are summarized in Table 1 .
The mean scores of perceived nurse manager competence, job satisfaction, and turnover intention are presented in Table 2 . The participants’ average (SD) perceived nurse manager competence score was 3.06 (1.24) out of 5. Among the five dimensions, Change and resource management scored the highest at 3.09 (1.32), and Personal mastery scored the lowest at 3.02 (1.23). Additionally, the mean (SD) job satisfaction was 3.05 (1.57) out of 5, and turnover intention was 2.82 (1.44) out of 5. Approximately one-fourth of the participants ( n = 91, 25.7%) were satisfied with their current job, while less than half were dissatisfied with their current job ( n = 170, 48.0%) and intended to leave their current job ( n = 165, 46.9%).
A Pearson correlation coefficient was calculated to examine the relationship between The mean scores of perceived nurse manager competence, job satisfaction, and turnover intention are presented in Table 2 . The participants’ average (SD) perceived nurse manager competence score was 3.06 (1.24) out of 5. Among the five dimensions, Change and resource management scored the highest at 3.09 (1.32), and Personal mastery scored the lowest at 3.02 (1.23). Additionally, the mean (SD) job satisfaction was 3.05 (1.57) out of 5, and turnover intention was 2.82 (1.44) out of 5. Approximately one-fourth of the participants ( n = 91, 25.7%) were satisfied with their current job, while less than half were dissatisfied with their current job ( n = 170, 48.0%) and intended to leave their current job ( n = 165, 46.9%).
, job satisfaction, and turnover intention. The results indicated a significant positive relationship between job satisfaction and perceived nurse manager competence ( r = 0.933, p < 0.001) and its dimensions. Additionally, there was a significant negative correlation between turnover intention and nurse manager competencies ( r = -0.901, p < 0.001) and its dimensions. As a result, the research findings led to the confirmation of the research hypothesis. It is worth mentioning that the power of the evaluated relationships was above 0.6, indicating a strong relationship between perceived nurse manager competence, job satisfaction, and turnover intention. The correlation between perceived nurse manager competence, job satisfaction, and turnover intention is shown in the correlation matrix ( Table 3 ) .
We used multiple linear regression to explain the impact of perceived nurse manager competence on job satisfaction and turnover intention. The results showed that job satisfaction is determined by all dimensions of perceived nurse manager competence, except for “Team communication and collaboration” (F = 217.940, p < 0.001), and turnover intention is determined by all dimensions of perceived nurse manager competence, except for “Team communication and collaboration” (F = 140.037, p < 0.001). Regression coefficients (B) showed that job satisfaction increases by 0.014 to 0.036 units for one-unit increase in each dimension of nursing managers’ competencies. In contrast, the intention to leave the service decreases by 0.007 to 0.022 units. These results showed that nursing managers’ competencies play an important role in increasing job satisfaction and reducing nurses’ intention to leave the service. The dimensions of perceived nurse manager competence predicted 87.3% and 81.3% of the variance in job satisfaction and turnover intention, respectively (Table 4 ).
The role of nursing in providing high-quality patient care is crucial. It’s essential to understand the factors that affect job satisfaction and turnover intention among nurses, especially in areas facing staff shortages. Nursing manager competencies are vital for coordinating medical activities and meeting patient needs [ 31 ]. A study examined the relationship between nursing manager competencies, job satisfaction, and nurse turnover in the Ardabil region of Iran. The study found that nurse managers had an average competency score of 3.06 out of 5, indicating a significant potential for improvement. Only 25.7% of nurses reported job satisfaction, while 46.9% expressed intentions to leave their current positions. The study revealed that all perceived nurse manager competence, except “team communication and collaboration,” influence job satisfaction. Additionally, turnover intention is affected by all competencies except “team communication and collaboration.” The competencies predicted 87.3% of the variance in job satisfaction and 81.3% in turnover intention. The study highlighted the potential for enhancing nursing managers’ competencies to improve job satisfaction and reduce turnover, ultimately benefiting patient care and organizational stability.
The findings of our study revealed that the average competency score of nurse managers among participants was 3.06 out of 5. In comparison, Choi’s study showed an overall perceived nurse manager competence level of 3.15 out of 5 [ 6 ]. In our research, Change and resource management received the highest score (3.09), while Personal mastery received the lowest score (3.02). In Choi et al.‘s study, “Quality Monitoring and Pursuance” received the highest score with an average of 3.20, while “Team Communication and Collaboration” scored the lowest with an average of 3.05 [ 6 ]. Therefore, our study suggests that perceived nurse manager competence is relatively lower than Choi’s study’s findings. It is important to allocate more effort to develop and enhance these competencies. Additionally, change and resource management are crucial in developing the abilities of nurse managers. This emphasizes the substantial importance of implementing effective change and resource management strategies to improve the skills and capabilities of nurse managers in the ever-changing healthcare environment.
In our research, about 25.7% of the participants expressed satisfaction with their current job. In a study by Choi et al., more than a third of the respondents stated they were satisfied with their current job. It’s worth noting that Choi et al.‘s study was conducted during the COVID-19 pandemic [ 6 ]. According to the study by Smith et al., the majority of nurses reported average (1.38%) or high (9.27%) job satisfaction [ 32 ]. The results of the systematic review study by Dilig-Ruiz et al. indicated that the average job satisfaction score for nurses across all studies was 56%, showing fluctuations over time [ 33 ]. Previous research also suggests that job satisfaction has decreased in developing countries [ 34 , 35 ]. Due to the impact of different job aspects on nurses’ job satisfaction and the variation of these dimensions in other countries, cities, and hospitals, differences in job satisfaction scores are also expected and explainable. These differences may be attributed to dissimilar working conditions and professional status in research environments.
Based on our study results, less than half of the participants were considering leaving their current job (46.9%). Choi’s study findings showed that one-third of respondents are thinking about leaving their current workplace. The prevalence of combined turnover intention rates for Asia, North America, and Europe were 20%, 15%, and 7% [ 36 ]. In Maaitah’s study, nurses had a strong inclination to leave. This may be due to multiple factors affecting the work environment and workload, contributing to high turnover intentions [ 37 ]. The most common reasons for job turnover are poor management, low wages, and insufficient staff [ 38 ]. The lack of necessary competencies for nursing managers may reduce job satisfaction and increase turnover intentions among nursing staff, potentially leading to decreased quality of care and patient satisfaction and threatening patient health outcomes [ 39 ].
In our research, we discovered that nursing managers possessing high competencies in “change and resource management” have a positive impact on nursing job satisfaction and turnover intention. This is achieved through effective change management, optimal resource allocation, budget management, prioritizing employee needs, and enhancing quality improvement initiatives. In today’s healthcare systems, challenges such as bed shortages, staff shortages, and equipment shortages are common. To tackle these challenges, nursing managers have worked together to create and implement new care systems and protocols. These are specifically designed to deal with the issues that nursing team encounter. These systems improve patient care overall and ensure that patients and healthcare professionals are well taken care of [ 40 ]. Nursing managers have a crucial role in ensuring high-quality patient care by embracing new tools, staying updated on healthcare advancements, and addressing patients’ evolving needs while optimizing the organizational framework [ 31 ]. Recent studies have reported that the daily work of nursing managers often involves organizing, planning work, and managing resources [ 2 , 41 ]. It should be noted that the decision of the nurse manager to allocate resources to nursing processes, along with staff training and orientation, may reduce resources for nursing at the bedside and, therefore, may impact nursing staff [ 2 ]. Organizational factors such as staff adequacy can increase nurse job satisfaction [ 7 ]. The effective interventions identified in the Niskala study were the first step in developing human resource strategies for healthcare organizations. These findings suggest that external factors (such as pay and rewards) are less effective than internal factors (such as spiritual intelligence, professional identity, and awareness) in maintaining job satisfaction [ 42 ]. Improving management decisions and increasing human resources in crisis conditions can impact nurses’ performance and job satisfaction [ 43 ]. Nurse managers can increase job satisfaction by demonstrating practical change and resource management competencies, ensuring adequate staffing, and making sound management decisions.
Our findings indicate that the quality monitoring and pursuit competencies of nurse managers positively impact nurses’ job satisfaction and intention to leave their jobs. Competent nurse managers play a crucial role in upholding high care standards, fostering a supportive environment, and acknowledging the efforts of staff to reduce turnover. Quality management also helps lighten nurses’ workloads and optimize resource allocation, ultimately leading to increased satisfaction. Effective management is essential for ensuring that patients receive care that is effective, efficient, and of high quality [ 31 ]. Leading quality improvement in the complex daily environment of a healthcare organization poses a challenge for nursing managers [ 44 ]. The day-to-day responsibilities of nursing managers involve caring for patients and overseeing the quality of care, which can be viewed as entirely logical [ 45 ]. Nursing managers can influence the quality of care by ensuring adequate staff [ 45 , 46 ]. Nursing management fosters commitment, coherence, consistency, and direction in service provision. Strategic leadership aligns the skills of nursing staff with healthcare goals, empowers nurses to deliver high-quality care, and ensures adherence to quality and safety standards [ 31 ]. To enhance the quality of care and cultivate a culture of quality in the nursing sector, nursing managers should strive to encourage staff to improve efficiency and comprehensiveness in achieving nursing goals and objectives. By implementing these approaches, nursing managers can elevate the quality of care and establish a quality culture, leading to further development and achievements in the nursing unit.
The results show that supportive nursing managers significantly impact job satisfaction and retention among nurses. They create a positive work environment, offer professional development, communicate effectively, and promote work-life balance as a priority, which reduces turnover rates and boosts morale. This support leads to increased job satisfaction and lower intention to leave among nurses [ 6 ]. Therefore, managerial support is crucial in predicting job satisfaction and turnover intention [ 47 ]. Smokrović et al. found that of all the factors in the practice environment scales of the nursing work index (PES-NWI), only nurse manager ability, leadership, and nurse support reliably predict nurse job satisfaction. The nursing management structure refers to nurses’ abilities, leadership, and support, as well as the ability of nurse leaders to manage and lead nursing managers effectively, support other nurses, and involve them in decision-making [ 48 ]. Nursing managers play a crucial role in creating a healthy work environment for nurses by fostering a positive culture and ensuring effective communication, high-quality nursing care, and teamwork. They are responsible for providing guidance and support to nursing staff, promoting a safe and supportive space that values open communication, collaboration, and mutual respect [ 49 , 50 , 51 ]. Previous research has shown that managerial support can help reduce employees’ intention to leave their jobs [ 52 ]. Tuckett et al. study introduced nurses’ rights and administrative support as essential predictors of intention to leave their jobs. It stated that a lack of managerial support can impact turnover intention among healthcare staff [ 53 ]. Nursing managers play a crucial role in providing vital support to their staff. By ensuring the job satisfaction of their team, they significantly increase the likelihood of retaining talented professionals in the nursing profession [ 54 ].
Our study highlights the paramount importance of personal mastery in nursing managers, a skill that is a strong predictor of job satisfaction and turnover intention. Managers with high personal mastery are adept at managing stress and challenges, thereby fostering a stable work environment that bolsters job satisfaction among their staff. Effective communication and conflict resolution are crucial to building trust and positive relationships within the team, thereby increasing job satisfaction and reducing turnover intention. It is imperative for nursing managers to create a supportive workplace and communicate effectively to motivate their team, thereby leading to higher job satisfaction and reduced intention to leave the job [ 55 ]. The journey to becoming a nurse manager begins with developing an awareness of the role, knowledge, and fundamental theories of healthcare management. The index serves as a roadmap for the managerial position, guiding future nurse managers in their next management role [ 39 ]. Previous studies have underscored the importance of nurse managers in upholding the quality of care, including their ability to demonstrate personal mastery, address potential issues, and build trust, calmness, and control [ 56 , 57 ]. Nurse managers play a crucial role in improving nursing services by demonstrating their structural capacity through results management [ 58 ]. Furthermore, nurse managers should be proficient in critical thinking, team management, conflict resolution, and collaborative decision-making to enhance pre-action nursing thinking [ 59 ].
Limitations
Our research is dedicated to exploring the significant role of nursing managers’ competencies in creating a positive work environment for nurses in Iran. This focus is crucial as it directly affects this group’s job satisfaction and turnover intentions. However, it’s essential to recognize the limitations of the study. For example, our research’s geographical scope may restrict our findings’ applicability to specific segments of the population. Additionally, while innovative, using WhatsApp for data collection may limit the representation of certain nurses, especially those who are less tech-savvy. Furthermore, the cross-sectional nature of the study design makes it challenging to establish a causal relationship between nursing managers’ competencies and job satisfaction or turnover intentions. Due to the specific characteristics of the participating hospitals, there is also a potential for selection bias, which may influence the nurses’ responses and perceptions. This bias could be due to [specific reasons for potential bias in the participating hospitals]. These limitations underscore the need for further research and development in this area.
Furthermore, relying on the same individuals to evaluate nursing managers’ competencies, job satisfaction, and turnover intentions may lead to bias. Participants’ subjective views, influenced by their experiences, could distort the results. While using nurses’ assessments to gauge nurse managers’ competencies provides direct perceptions, it may need to capture their objective competencies fully. Future research would benefit from a multi-method approach, such as performance evaluations or peer assessments, to enhance the validity of the findings. Moreover, additional research is needed to explore other variables affecting perceived competency levels, job satisfaction, and turnover. Variables such as leadership styles, organizational culture, career development opportunities, work-life balance strategies, employee recognition programs, job security measures, and competitive salary and benefits packages warrant further investigation to gain insights into the complex dynamics of nursing management and job satisfaction.
The research findings indicate that nursing managers in Iran have lower competencies than in previous studies. It is essential to strengthen these competencies as they significantly improve job satisfaction and reduce nurse turnover intentions. Effective leadership, mainly, plays a crucial role in this process. Managers who effectively support staff, manage resources, and demonstrate strong leadership not only positively impact nurses’ job satisfaction and retention but also have the power to inspire and motivate their teams. To enhance nurse job satisfaction and reduce turnover rates, healthcare organizations should conduct unit-level assessments to identify areas for improvement. Promoting professional development, recognizing staff efforts, and creating supportive work environments are essential. In conclusion, enhancing nursing managers’ competencies and prioritizing job satisfaction is crucial for retaining a qualified nursing workforce and improving healthcare quality. These findings provide valuable insights into effective nursing management practices.
Data availability
The datasets used and analyzed during the current study are available from the corresponding author upon reasonable request.
Abbreviations
Content Validity Ratio
Content Validity Index
Root Mean Square Error of Approximation
Confirmatory Factor Analysis
Goodness of Fit Index
Comparative Fit Index
Tucker-Lewis Index
Incremental Fit Index
Intraclass Correlation Coefficient
Roche MA, Duffield C, Dimitrelis S, Frew B. Leadership skills for nursing unit managers to decrease intention to leave. Nursing: Res Reviews 2015:57–64.
Nurmeksela A, Mikkonen S, Kinnunen J, Kvist T. Relationships between nurse managers’ work activities, nurses’ job satisfaction, patient satisfaction, and medication errors at the unit level: a correlational study. BMC Health Serv Res. 2021;21:1–13.
Article Google Scholar
Akbiyik A, Korhan EA, Kiray S, Kirsan M. The effect of nurses ‘Leadership Behavior on the quality of nursing care and patient outcomes. Creat Nurs. 2020;26(1):8–18.
Ngabonzima A, Asingizwe D, Kouveliotis K. Influence of nurse and midwife managerial leadership styles on job satisfaction, intention to stay, and services provision in selected hospitals of Rwanda. BMC Nurs. 2020;19:1–11.
Schwendimann R, Dhaini S, Ausserhofer D, Engberg S, Zúñiga F. Factors associated with high job satisfaction among care workers in Swiss nursing homes–a cross sectional survey study. BMC Nurs. 2016;15:1–10.
Choi P-P, Lee W-M, Wong S-S, Tiu M-H. Competencies of nurse managers as predictors of staff nurses’ job satisfaction and turnover intention. Int J Environ Res Public Health. 2022;19(18):11461.
Article PubMed PubMed Central Google Scholar
Cummings GG, Tate K, Lee S, Wong CA, Paananen T, Micaroni SP, Chatterjee GE. Leadership styles and outcome patterns for the nursing workforce and work environment: a systematic review. Int J Nurs Stud. 2018;85:19–60.
Article PubMed Google Scholar
Akhtary Shojaei E, Nazari A, Vahidi R. Leadership styles of managers and job satisfaction among nurses in Tabriz hospitals. Hakim Res J. 2005;7(4):20–4.
Google Scholar
Labrague LJ, Gloe D, McEnroe DM, Konstantinos K, Colet P. Factors influencing turnover intention among registered nurses in Samar Philippines. Appl Nurs Res. 2018;39:200–6.
Labrague L, De Los Santos J, Falguera C, Nwafor C, Galabay J, Rosales R, Firmo C. Predictors of nurses’ turnover intention at one and five years’ time. Int Nurs Rev. 2020;67(2):191–8.
Article CAS PubMed Google Scholar
Al Sabei SD, Al-Rawajfah O, AbuAlRub R, Labrague LJ, Burney IA. Nurses’ job burnout and its association with work environment, empowerment and psychological stress during COVID‐19 pandemic. Int J Nurs Pract. 2022;28(5):e13077.
Gurková E, Mikšová Z, Šáteková L. Missed nursing care in hospital environments during the COVID-19 pandemic. Int Nurs Rev. 2022;69(2):175–84.
AL-Ghwary AA, AL-Oweidat IA, Al-Qudimat AR, Abu Shosha GM, Khalifeh AH, ALBashtawy M. The impact of work environment on structural empowerment among nurses in Governmental hospitals. Nurs Rep. 2024;14(1):482–93.
Taylor-Clark TM, Swiger PA, Anusiewicz CV, Loan LA, Olds DM, Breckenridge-Sproat ST, Raju D, Patrician PA. Identifying potentially preventable reasons nurses intend to leave a job. JONA: J Nurs Adm. 2022;52(2):73–80.
Article PubMed Central Google Scholar
Mohamed Z, Al-Hmaimat N. The effectiveness of nurse residency programs on new graduate nurses’ retention: systematic review. Heliyon. 2024;10(5):e26272.
Kaihlanen A-M, Elovainio M, Haavisto E, Salminen L, Sinervo T. Final clinical practicum, transition experience and turnover intentions among newly graduated nurses: a cross sectional study. Nurse Educ Today. 2020;84:104245.
Boamah SA, Laschinger HKS, Wong C, Clarke S. Effect of transformational leadership on job satisfaction and patient safety outcomes. Nurs Outlook. 2018;66(2):180–9.
Ofei AMA, Poku CA, Paarima Y, Barnes T, Kwashie AA. Toxic leadership behaviour of nurse managers and turnover intentions: the mediating role of job satisfaction. BMC Nurs. 2023;22(1):374.
Brewer CS, Kovner CT, Djukic M, Fatehi F, Greene W, Chacko TP, Yang Y. Impact of transformational leadership on nurse work outcomes. J Adv Nurs. 2016;72(11):2879–93.
García-Sierra R, Fernández‐Castro J. Relationships between leadership, structural empowerment, and engagement in nurses. J Adv Nurs. 2018;74(12):2809–19.
Lievens I, Vlerick P. Transformational leadership and safety performance among nurses: the mediating role of knowledge-related job characteristics. J Adv Nurs. 2014;70(3):651–61.
Masood M, Afsar B. Transformational leadership and innovative work behavior among nursing staff. Nurs Inq. 2017;24(4):e12188.
Von Elm E, Altman DG, Egger M, Pocock SJ, Gøtzsche PC, Vandenbroucke JP, Initiative S. The strengthening the reporting of Observational studies in Epidemiology (STROBE) Statement: guidelines for reporting observational studies. Int J Surg. 2014;12(12):1495–9.
Anderson JC, Gerbing DW. The effect of sampling error on convergence, improper solutions, and goodness-of-fit indices for maximum likelihood confirmatory factor analysis. Psychometrika. 1984;49:155–73.
Byrne BM. Structural equation modeling with Mplus: basic concepts, applications, and programming. routledge; 2013.
Floyd FJ, Widaman KF. Factor analysis in the development and refinement of clinical assessment instruments. Psychol Assess. 1995;7(3):286.
DeVellis RF, Thorpe CT. Scale development: theory and applications. Sage; 2021.
Koo TK, Li MY. A guideline of selecting and reporting intraclass correlation coefficients for reliability research. J Chiropr Med. 2016;15(2):155–63.
Das KR, Imon A. A brief review of tests for normality. Am J Theoretical Appl Stat. 2016;5(1):5–12.
Draper NR, Smith H. Applied regression analysis. Volume 326. Wiley; 1998.
Ofei AMA, Barnes T, Paarima Y. Strategic Leadership in nursing. Nursing: New insights for Clinical Care. edn.: IntechOpen; 2022.
Smith S, Lapkin S, Halcomb E, Sim J. Job satisfaction among small rural hospital nurses: a cross-sectional study. J Nurs Scholarsh. 2023;55(1):378–87.
Dilig-Ruiz A, MacDonald I, Demery Varin M, Vandyk A, Graham ID, Squires JE. Job satisfaction among critical care nurses: a systematic review. Int J Nurs Stud. 2018;88:123–34.
Akbari M, Bagheri A, Fathollahi A, Darvish M. Job satisfaction among nurses in Iran: does gender matter? J Multidisciplinary Healthc 2020:71–8.
Huaman N, Morales-García WC, Castillo-Blanco R, Saintila J, Huancahuire-Vega S, Morales-García SB, Calizaya-Milla YE, Palacios-Fonseca A. An explanatory model of work-family conflict and resilience as predictors of job satisfaction in nurses: the mediating role of work engagement and communication skills. J Prim care Community Health. 2023;14:21501319231151380.
Wu F, Lao Y, Feng Y, Zhu J, Zhang Y, Li L. Worldwide prevalence and associated factors of nursing staff turnover: a systematic review and meta-analysis. Nurs Open. 2024;11(1):e2097.
Maaitah AM. The role of leadership style on turnover intention. Int Rev Manage Mark. 2018;8(5):24.
Magbity J, Ofei A, Wilson D. Leadership styles of nurse managers and turnover intention. Hosp Top. 2020;98(2):45–50.
Xu X, Zhang Y, Zhou P, Lin Y, Pan W. A modified Delphi study on establishing a curriculum content structure for the leadership and management competency cultivation for future nurse managers in China. Heliyon 2022, 8(12).
Yu X, Li M, Du M, Wang Y, Liu Y, Wang H. Exploring factors that affect nurse staffing: a descriptive qualitative study from nurse managers’ perspective. BMC Nurs. 2024;23(1):80.
Warshawsky N, Cramer E. Describing nurse manager role preparation and competency: findings from a national study. JONA: J Nurs Adm. 2019;49(5):249–55.
Niskala J, Kanste O, Tomietto M, Miettunen J, Tuomikoski AM, Kyngäs H, Mikkonen K. Interventions to improve nurses’ job satisfaction: a systematic review and meta-analysis. J Adv Nurs. 2020;76(7):1498–508.
Fereidouni A, Teymoori E, Maleki Z, Ghanavati M, Vizeshfar F. Relationships between Job satisfaction of operating Room nurses and Hospital’s Compliance with Protective guidelines during the Covid-19 pandemic: a cross-sectional study, Iran. J Perianesthesia Nurs. 2023;38(1):51–7.
Sjølie BM, Hartviksen TA, Bondas T. Navigation to prioritizing the patient–first-line nurse managers’ experiences of participating in a quality improvement collaborative. BMC Health Serv Res. 2020;20:1–13.
Van Bogaert P, Peremans L, Van Heusden D, Verspuy M, Kureckova V, Van de Cruys Z, Franck E. Predictors of burnout, work engagement and nurse reported job outcomes and quality of care: a mixed method study. BMC Nurs. 2017;16:1–14.
Arakelian E, Rudolfsson G. Managerial challenges faced by Swedish nurse managers in perioperative settings–a qualitative study. BMC Nurs. 2021;20(1):117.
Smokrović E, Gusar I, Hnatešen D, Bačkov K, Bajan A, Gvozdanović Z, Placento H, Žvanut B. Influence of work motivation, work environment and job satisfaction on turnover intention of Croatian nurses: a qualitative study. Southeast Eur Med Journal: SEEMEDJ. 2019;3(2):33–44.
Smokrović E, Kizivat T, Bajan A, Šolić K, Gvozdanović Z, Farčić N, Žvanut B. A conceptual model of nurses’ turnover intention. Int J Environ Res Public Health. 2022;19(13):8205.
Eltaybani S, Yamamoto-Mitani N, Ninomiya A, Igarashi A. The association between nurses’ burnout and objective care quality indicators: a cross-sectional survey in long-term care wards. BMC Nurs. 2021;20:1–10.
Ljungbeck B, Sjögren Forss K. Advanced nurse practitioners in municipal healthcare as a way to meet the growing healthcare needs of the frail elderly: a qualitative interview study with managers, doctors and specialist nurses. BMC Nurs. 2017;16:1–9.
Lucas P, Jesus É, Almeida S, Araújo B. Relationship of the nursing practice environment with the quality of care and patients’ safety in primary health care. BMC Nurs. 2023;22(1):413.
Ahlstedt C, Eriksson Lindvall C, Holmström IK, Muntlin Å. Flourishing at work: nurses’ motivation through daily communication–An ethnographic approach. Nurs Health Sci. 2020;22(4):1169–76.
Tuckett A, Winters-Chang P, Bogossian F, Wood M. Why nurses are leaving the profession… lack of support from managers’: what nurses from an e‐cohort study said. Int J Nurs Pract. 2015;21(4):359–66.
Andrews MC, Woolum A, Mesmer-Magnus J, Viswesvaran C, Deshpande S. Reducing turnover intentions among first-year nurses: the importance of work centrality and coworker support. Health Serv Manage Res 2023:09514848231165891.
Asamani JA, Naab F, Ofei AMA. Leadership styles in nursing management: implications for staff outcomes. J Health Sci. 2016;6(1):23–36.
Zaghini F, Fiorini J, Piredda M, Fida R, Sili A. The relationship between nurse managers’ leadership style and patients’ perception of the quality of the care provided by nurses: Cross sectional survey. Int J Nurs Stud. 2020;101:103446.
Van Bogaert P, Peremans L, Franck E, Timmermans O, Havens DS. Nurse managers’ perceptions and experiences regarding staff nurse empowerment: a qualitative study. Front Psychol. 2015;6:151183.
Aqtash S, Alnusair H, Brownie S, Alnjadat R, Fonbuena M, Perinchery S. Evaluation of the impact of an education program on self-reported leadership and management competence among nurse managers. SAGE Open Nurs. 2022;8:23779608221106450.
PubMed PubMed Central Google Scholar
González García A, Pinto-Carral A, Sanz Villorejo J, Marqués-Sánchez P. Nurse manager core competencies: a proposal in the Spanish health system. Int J Environ Res Public Health. 2020;17(9):3173.
Download references
Acknowledgements
This study has been approved by the student research committee of Ardabil University of Medical Sciences (IR.ARUMS.REC.1402.063). The authors would like to thank all the nurses and the officials of the hospitals affiliated with the Ardabil University of Medical Sciences and all those who helped us in this study.
This research received no specific grant from funding agencies in the public, commercial or not-for-profit sectors.
Author information
Authors and affiliations.
Students Research Committee, School of Nursing and Midwifery, Ardabil University of Medical Sciences, Ardabil, Iran
Alireza Mirzaei & Mohammad Javad Jafari
Department of Emergency Nursing, School of Nursing and Midwifery, Ardabil University of Medical Sciences, Ardabil, Iran
Alireza Mirzaei
Department of Emergency Medicine, School of Medicine, Ardabil University of Medical Sciences, Ardabil, Iran
Reza Imashi & Roghayeh Yaghoobi Saghezchi
Department of Medical-Surgical Nursing, School of Nursing and Midwifery, Ardabil University of Medical Sciences, Ardabil, Iran
Reza Nemati-Vakilabad
Student Research Committee, School of Nursing and Midwifery, Guilan University of Medical Sciences, Rasht, Iran
You can also search for this author in PubMed Google Scholar
Contributions
All the authors were involved in designing the study. MJJ carried out the data collection and data entry, AM performed the statistical analyses and interpretations, RI and RYS wrote the revised version, which was reviewed and edited, and RNV wrote the final report and manuscript. All the authors read and approved the final manuscript.
Corresponding author
Correspondence to Reza Nemati-Vakilabad .
Ethics declarations
Ethics approval and consent to participate.
The Ethics Committee of Ardabil University of Medical Sciences has approved this study proposal with ethics code IR.ARUMS.REC.1402.063. Before participation, participants were informed about the study’s objectives and methods, and written informed consent was obtained from them. All procedures were performed following the Deceleration of Helsinki. Participation in this study was voluntary, and participants were informed that they could withdraw from the study at any stage. Ethical considerations, such as confidentiality, anonymity, and information privacy, were strictly observed during the study.
Consent for publication
Not applicable.
Competing interests
The authors declare no competing interests.
Additional information
Publisher’s note.
Springer Nature remains neutral with regard to jurisdictional claims in published maps and institutional affiliations.
Rights and permissions
Open Access This article is licensed under a Creative Commons Attribution-NonCommercial-NoDerivatives 4.0 International License, which permits any non-commercial use, sharing, distribution and reproduction in any medium or format, as long as you give appropriate credit to the original author(s) and the source, provide a link to the Creative Commons licence, and indicate if you modified the licensed material. You do not have permission under this licence to share adapted material derived from this article or parts of it.The images or other third party material in this article are included in the article’s Creative Commons licence, unless indicated otherwise in a credit line to the material. If material is not included in the article’s Creative Commons licence and your intended use is not permitted by statutory regulation or exceeds the permitted use, you will need to obtain permission directly from the copyright holder.To view a copy of this licence, visit http://creativecommons.org/licenses/by-nc-nd/4.0/ .
Reprints and permissions
About this article
Cite this article.
Mirzaei, A., Imashi, R., Saghezchi, R.Y. et al. The relationship of perceived nurse manager competence with job satisfaction and turnover intention among clinical nurses: an analytical cross-sectional study. BMC Nurs 23 , 528 (2024). https://doi.org/10.1186/s12912-024-02203-5
Download citation
Received : 22 March 2024
Accepted : 25 July 2024
Published : 01 August 2024
DOI : https://doi.org/10.1186/s12912-024-02203-5
Share this article
Anyone you share the following link with will be able to read this content:
Sorry, a shareable link is not currently available for this article.
Provided by the Springer Nature SharedIt content-sharing initiative
- Job satisfaction
- Nursing management
- Turnover intention
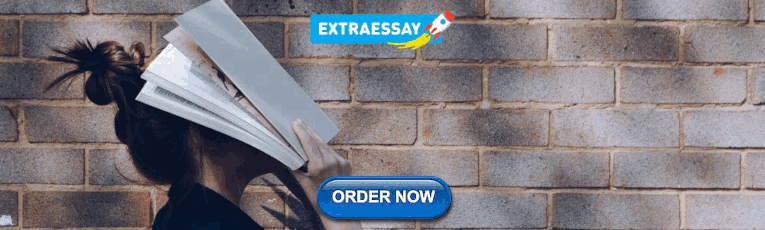
BMC Nursing
ISSN: 1472-6955
- General enquiries: [email protected]

CAREER & HIRING ADVICE
19 employee retention statistics that will surprise you.
- Ryan Bradshaw
- March 26, 2024

Employee retention is a big issue in the workforce. It’s not just about keeping your employees happy , it’s about retaining your talent and ensuring you keep the best people with you as long as possible.
One-third of new employees quit after about six (6) months , according to statistics. This is a significant number and one that needs to be addressed with some urgency if employers want to fix this problem in their company.
Each month in the US, 3 to 4.5 million employees quit their job according to the Job Openings and Labor Turnover Survey (JOLTS) . One survey found 94% would stay at their current employer if they invested in their long term learning.
Statistics show that employee turnover has been rising in recent years. Employers are not only losing valuable talent but also having to deal with hiring, training, and replacing lost workers.
What is employee retention?
An organization’s ability to keep its employees refers to employee retention . The retention rate of employees can be expressed by a simple statistic. Employee retention , however, is frequently regarded as the effort employers make to keep their employees.
Why employee retention is important
Low employee retention can be particularly costly and detrimental to businesses in industries where specialised skills are essential, such as information technology, hospitality, and manufacturing .
Think about it when an employee leaves a company, it takes a considerable chunk of time to deal with their departure. In fact, entry-level employees typically cost 50% of their salary to replace .
Employee retention also helps boost morale, reduces costs, maintains a good customer experience, and reduces overall costs.
The best way to retain employees is by making them feel valued and providing them the opportunity for growth within your organization. When an employee does not feel valued at work, 76% look for another job opportunity
A workplace survey report found that 94% of surveyed employees responded that if a company invested in helping them learn, they would stay longer.
This can be done through training programs or mentoring opportunities that will help develop skillsets in new areas.
It’s important to make sure you are offering these types of opportunities so they feel like they have something to look forward to. If not, then there may come a point where they leave because they don’t see any future for themselves at your business.
There are various types of staff turnover . Voluntary turnover happens when an individual leaves their job for personal reasons. On the other hand, involuntary turnover occurs when a company decides for an individual to leave employment.
Retirement turnover signifies the conclusion of an individual’s working life, while transfer turnover involves changing employment by moving to another department within the same organization. Each type of turnover reflects distinct factors influencing the employment transition.
It costs an employer an average of 33% of an employee’s yearly salary for their exit.
The cost of replacing employees is high. So, before making rigorous decisions employers should screen and assess with the help of the right tools on their current roles and responsibilities .
On the other hand, the process of finding the best talent typically involves advertising job postings, recruitment agencies , screening, interviews, and hiring.
This adds up to spending a lot of time, money, and energy to replace workers that could have been retained with a good employee retention strategy.
Statistics on employee retention
In the 2021 Bureau of Labor Statistics report, the overall turnover rate is 57.3 % , but that number drops to 25% when considering only voluntary turnover, 29% when considering involuntary turnover, and just 3% when looking at only high-performers.
One report suggests that a turnover rate of close to 19% can be expected in many industries. SHRM estimates that the average cost-per-hire for a new employee is $4129.
Economic News Releases from the Bureau of Labor Statistics report that employees earning wages and salaries averaged 4.6 years with their current employer.
A person changes careers on average when they are 39 years old. Over the next five years, 87% of respondents surveyed by Kronos consider improved employee retention a high or critical priority. An analysis of 34,000 responses to the Work Institute’s 2017 Retention Report found that 75% of the reasons for employee turnover can be prevented.
The most common reason employees leave their jobs is because they are not being challenged at work. This includes feeling under-appreciated and bored with what you do every day. If this sounds familiar, it may help if you think about how an employee’s job fits into a bigger picture or strategy.
You could also consider allowing employees to change roles within your company so they have more opportunty for growth , the chance to learn new skills and grow professionally.
There is a 16% decrease in retention rates for employees who aren’t comfortable giving upward feedback.
This was an interesting statistic found in the Tinypulse retention report . This makes it clear that managers and employees must communicate clearly in order to understand the needs and make improvements.
Regularly communicating employee thoughts to management should be strongly encouraged. It shows your employees that they are valued if you keep lines of communication open.
Regular and honest communication enhances employee retention by showing employees that their contribution is valued. As a leader, it also helps you recognize when adjustments may be needed in your employee retention strategy .

A good onboarding program leads to 69% of employees staying at least 3 years.
A survey conducted by CareerBuilder and Silkroad Technology (9%) represents the number of employees who left their company because of poor onboarding experiences, and 37% percent said their manager was not part of their onboarding experience.
Losing 1 in 10 people to a poor onboarding experience is something a good company should be able to fix quickly.
The most common reason for leaving an employer? Poor management practices. The second-most cited reason: lack of career development opportunities. And third on that list: bad culture fit.
If you’re looking at your own employee retention numbers , it might help to know what those three things are. If they aren’t addressed, then you can expect more turnover — which means less productivity and higher costs.
What is the national average employee retention rate?
As a general rule, employee retention rates of 90 percent or higher are considered good and a company should aim for a turnover rate of 10% or less.
The following factors can affect an employer’s ability to retain employees:
• Lack of training and development opportunities for new hires
• Poor communication between management and staff members
• Unclear job descriptions that do not clearly define responsibilities
• Inadequate staffing levels to cover the workload.
• A lack of recognition by managers when their work is done well
• An inability to provide feedback on performance issues
• The need for more flexible working hours
• High turnover costs associated with hiring and firing workers
How much is employee retention worth?
It costs 33% of a worker’s annual salary to replace them if they leave, according to Employee Benefit News .
The Work Institute’s Retention Report shows that the replacement cost is $16,500 per person for an employee earning a median salary of $50,000 a year.
Employee retention rate by industry
BLS statistics show the following numbers in 2019. As you can Government related jobs had the lowest amount of separations/turnovers compared to the highest turnover in the entertainment and hospitality industries.
Top 5 best retention rates in 2019 by industry
Government | 18.6% |
Finance and insurance | 24.6% |
Manufacturing / durable goods | 28.6% |
Educational services | 29.8% |
Wholesale trade | 29.6% |
Top 5 worst retention rates in 2019 by industry
Arts, entertainment, and recreation | 79.5% |
Leisure and hospitality | 79.0% |
Accommodation and food services | 78.9% |
Construction | 65.0% |
Professional and business services | 63.5% |
What is the difference between retention rate and turnover rate?
Retention rate is the percentage of a company’s customers that remain with them for a set period of time. Turnover rate is measuring the percentage of employees that leave in a given period.
The two are not directly related, but they can be used to calculate how long it will take your business to reach its target market size.

Creating an employee retention strategy
The following metrics should be monitored as part of your employee retention strategy:
- The average turnover is related to promotions or transfers.
- The average tenure of your employees.
- Tracking positions filled vs positions that are open
- When measuring the turnover rate break them down into three categories: Voluntary, Involuntary, and employees noted as high-performing.
Monitoring your turnover and retention rates using SHRM and AHP retention rate formulas over time will help you learn how well you’re doing.
You should also take into context the average retention rates for your industry. Industries such as food service have problems with restaurant staffing issues . Food service and retail have higher turnover than other sectors.
But how do you create an effective retention strategy and have more loyal employees? Developing an employee retention strategy is critical for organizations, as high turnover generates significant recruitment, hiring, and training expenses. Retaining skilled employees enhances overall productivity by leveraging their familiarity with company processes and expectations.
Additionally, it preserves valuable institutional knowledge. A stable, engaged workforce fosters a positive environment, supporting a strong company culture and positively impacting customer satisfaction. This stability is a competitive advantage, attracting top talent and enhancing the organization’s reputation for employee satisfaction.
Effective retention is crucial for successful succession planning and cultivating potential leaders. Long-term employees contribute to a sense of ownership and commitment, fostering an innovative environment. Investing in professional development further encourages growth and advancement, contributing to the organization’s long-term success.
No matter how much data you collect, you won’t improve retention and turnover unless you do something. While tracking employee turnover and retention is the first step, the real work begins when you assess these rates in relation to your industry and identify what needs to be done to improve your retention strategies.
The most common reason for high attrition rates is that employees are not being given enough opportunities to grow professionally or personally within their organization. This can happen if managers don’t provide training on new skills or give people chances to learn from mistakes they make while working with clients.
If this happens often enough, it will lead to a loss of talent. The best way to prevent this problem is by providing ongoing professional development programs so that staff members have access to learning resources as needed.
- Business Advice
- Employer Advice
- Job Seeker Advice
Related Posts
How ai is transforming the job market: what you need to know.
- August 2, 2024
Protecting Enterprise Resources: Essential Digital Solutions for Modern Businesses
Streamlining the transaction process: key services you should be making use of.
- August 1, 2024
Strategies for Efficient and Secure File Sharing in the Supply Chain Sector
- July 31, 2024
Employee Retention through Continuous Technical Skills Development
- July 30, 2024
YOUR NEXT ENGINEERING OR IT JOB SEARCH STARTS HERE.
How do you hire for engineering and it.
1955 Lake Park DR SE STE 350 Smyrna, GA 30080
Dallas, TX 75212
Our Dallas TX recruiting experts understand the challenges unique to companies in dozens of industries and of all sizes.
Houston, TX 77040
Our Houston TX recruiting experts understand the challenges unique to companies in dozens of industries and of all sizes.
Austin, TX 78717
Our Austin / San Antiono TX recruiting experts understand the challenges unique to companies in dozens of industries and of all sizes.
Tampa, FL 33612
Our Tampa FL recruiting experts understand the challenges unique to companies in dozens of industries and of all sizes.
Raleigh, NC 27612
Our Raleigh NC recruiting experts understand the challenges unique to companies in dozens of industries and of all sizes.
Columbia, SC 29203
Our Greenville SC recruiting experts understand the challenges unique to companies in dozens of industries and of all sizes.
Chicago, IL 60714
Our Chicago IL recruiting experts understand the challenges unique to companies in dozens of industries and of all sizes.
- Engineering Staffing
- CAD Design / Drafting
- Information Technology
- Renewable Energy Recruiters
- Software Engineering Recruiters
- Controls Engineering Recruiters
- Supply Chain Recruiting
- Wireless & Telecom Recruiting
- Mechanical Engineering Recruiters
- Electrical Engineering Recruiters
- Civil Engineering Recruiters
- Chemical Engineering Recruiters
Information
- Author Services
Initiatives
You are accessing a machine-readable page. In order to be human-readable, please install an RSS reader.
All articles published by MDPI are made immediately available worldwide under an open access license. No special permission is required to reuse all or part of the article published by MDPI, including figures and tables. For articles published under an open access Creative Common CC BY license, any part of the article may be reused without permission provided that the original article is clearly cited. For more information, please refer to https://www.mdpi.com/openaccess .
Feature papers represent the most advanced research with significant potential for high impact in the field. A Feature Paper should be a substantial original Article that involves several techniques or approaches, provides an outlook for future research directions and describes possible research applications.
Feature papers are submitted upon individual invitation or recommendation by the scientific editors and must receive positive feedback from the reviewers.
Editor’s Choice articles are based on recommendations by the scientific editors of MDPI journals from around the world. Editors select a small number of articles recently published in the journal that they believe will be particularly interesting to readers, or important in the respective research area. The aim is to provide a snapshot of some of the most exciting work published in the various research areas of the journal.
Original Submission Date Received: .
- Active Journals
- Find a Journal
- Proceedings Series
- For Authors
- For Reviewers
- For Editors
- For Librarians
- For Publishers
- For Societies
- For Conference Organizers
- Open Access Policy
- Institutional Open Access Program
- Special Issues Guidelines
- Editorial Process
- Research and Publication Ethics
- Article Processing Charges
- Testimonials
- Preprints.org
- SciProfiles
- Encyclopedia
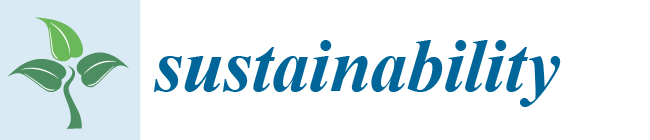
Article Menu
- Subscribe SciFeed
- Recommended Articles
- Google Scholar
- on Google Scholar
- Table of Contents
Find support for a specific problem in the support section of our website.
Please let us know what you think of our products and services.
Visit our dedicated information section to learn more about MDPI.
JSmol Viewer
Managing neurodiversity in workplaces: a review and future research agenda for sustainable human resource management.
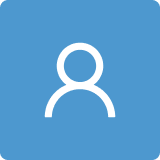
1. Introduction
2. literature review, 2.1. neurodiversity—origins of the concept and statistics, 2.2. neurodiversity in the labour market, 2.3. managing neurodiversity in workplaces—organizational barriers and needs, 3. materials and methods.
- What are the research directions and gaps in the topic of managing neurodiversity in workplaces mentioned in the literature?
- What are future research directions in the field of managing neurodiversity in workplaces with reference to Methodology, Theory, and Contexts?
5. Discussion and Conclusions
Author contributions, data availability statement, conflicts of interest.
No. | References | Research Objective | Research Topic/Contexts | Methodology |
---|---|---|---|---|
1 | [ ] | To emphasize the need for CEO adaptability and commitment in the ever-evolving landscape of workplace diversity | CEO leadership and its contribution to diversity management | - |
2 | [ ] | To present a multistage theoretical framework for managing neurodiversity | Addressing the gap between recognition and lack of theoretical frameworks and empirical research on workplace neurodiversity | - |
3 | [ ] | To understand the lived experiences of dealing with crisis in a remote working environment (based on 11 semi-structured interviews with 9 neurodivergent employees and 2 business professionals) | Lack of research on the impact of the COVID-19 crisis on neurominorities | Qualitative study |
4 | [ ] | To review existing research with respect to how autistic individuals fare for the four facets of emotional intelligence | Emotional intelligence in autistic individuals | Descriptive study |
5 | [ ] | To compare the experiences of neurodivergent adults undergoing workplace coaching before and during the pandemic | Disability coaching in a pandemic | Quantitative study—sample of 409 coachees from the UK |
6 | [ ] | To demystify the themes and assumptions affecting neurodivergent coachee experiences at work | Neurodiversity coaching | - |
7 | [ ] | To explore NDVs’ views about how the recruitment process and HR practices impact their employment relationship | Employment experience of the neurodiverse and the military veterans | Quantitative study (232 people with a medically diagnosed condition surveyed and 21 semi-structured interviews conducted) |
8 | [ ] | To seek to reframe the pathologized assumption of ADHD in career research and broader society to date. | Paradoxical career strengths and successes of ADHD adults | Qualitative positive-focused exploration |
9 | [ ] | To identify additional understudied invisible diversity dimensions | Invisible diversity dimensions in the hospitality industry | Prevailing methodologies in hospitality management |
10 | [ ] | To analyze power-laden tensions in traditional and novel brandings of neurodiversity | Neurodiversity branding | Critical discourse analysis |
11 | [ ] | To compare two families with disabled and neurodiverse children disproportionately affected by austerity cuts | Access to Special Educational Needs and Disability (SEND) support services in London, England | Qualitative study |
12 | [ ] | To examine successful management for autistic employees without applying a priori leadership constructs traditionally found in the literature | Effective management of autistic employees | - |
13 | [ ] | To promote effective and inclusive qualitative research that ensures that the specific needs of neurominorities are taken into account throughout the entire research pathway | How to conduct qualitative research with neurominorities | Qualitative research |
14 | [ ] | To explore an open, creative workplace developing neurodivergent talent | Neurodivergent individuals perceived as bringing new talent and innovation to the workplace | - |
15 | [ ] | To explore how interactions between clinicians, parents, and children lead to the reformulation of autism diagnosis from disorder to valuable social and cognitive differences | How clinician–patient interaction and patient age shape the process and meaning of autism diagnosis | A conversation analytic approach to data analysis/analysis of video recordings |
16 | [ ] | To examine the opportunities to create optimal conditions for individuals with autism in the workplace | How to redesign the work environment to enhance the well-being of individuals with autism | Qualitative research (21 in-depth interviews) |
17 | [ ] | To examine employee (manager and coworker) attitudes toward autism employment programs | Employee engagement and commitment to two Australian autism employment programs | Survey |
18 | [ ] | To examine the ability of corporate law firms to recognize the strengths and develop a type of employment both well suited to autistic strengths and able to make accommodations | Insight into the career paths of autistic professionals in large private practice law firms | - |
19 | [ ] | To explore the current inclusive human resource practices being adopted by neurodiversity champion companies | Enabling neurodiversity in the workplace via inclusive human resource practices | In-company interviews |
20 | [ ] | To generate a more nuanced understanding of the impact of accommodation on people with schizophrenia | Design considerations for residents with schizophrenia | Qualitative research approach |
21 | [ ] | To analyze the links between the neurodiversity of the workforce and digital transformation at the individual, organizational, and industry levels | Neurodiversity of the workforce and digital transformation: the case of inclusion of autistic workers at the workplace | Phenomenology (qualitative approach) |
22 | [ ] | To examine the disparate views on the neurodiverse workforce: 1/the benefits 2/the difficulty in finding and maintaining employment and to build a reconciling framework | How internal and external factors influence autistic employee outcomes and firm performance | - |
23 | [ ] | To examine neurodiversity as a cognitive strength from which leadership derives | Reconceptualizing leadership from a neurodiverse perspective | Critical disability theory |
24 | [ ] | To explore how autism influences the workplace | Autism in the workplace | Constant comparative method |
25 | [ ] | To describe how to design environmental conditions (acoustics, lighting, temperature, indoor air quality) that enhance performance by supporting basic physiological needs in the workplace (including designing spaces for neurodiversity) | Humanizing the office | - |
26 | [ ] | To explore the relationship between the above-average human capital of highly functioning neurodivergent employees, their subjective well-being in the workplace, and performance outcomes | The well-being of neurodivergent human capital in the workplace | AMO (ability, motivation, and opportunity) framework |
27 | [ ] | To identify the source of stereotype threat and the neurodivergent response to it | Stereotype threat anticipation in neurodivergent human capital | Analysis of brochures, learning sets, and posters |
28 | [ ] | To examine family relationships within families with neurodiverse kids further complicated by the COVID-19 pandemic | COVID-19 affecting alternative learners and their families | - |
29 | [ ] | To examine how and why parents with children with autism perform emotional work | Emotion work of parenting children with autism in Hong Kong | Qualitative data (analysis) |
30 | [ ] | To explore the school experience of high-functioning autistic teenagers regarding their academic and social inclusion | High-functioning autistic students speaking about their experience of inclusion in mainstream secondary schools (in France and Quebec) | A pragmatic, content analysis-driven approach |
31 | [ ] | To examine how DXC Technology (IT company) managed to develop disability-inclusive recruitment and selection systems along with work designs and environments that are disability friendly | DXC Technology is looking for neurodiverse talent (adults with high-functioning autism) | - |
32 | [ ] | To present a model of the unique challenges that workers with ASD face in the modern workplace | Overcoming barriers and integrating a neurodiverse workforce | A literature review on ASD juxtaposed to evidence-based social psychology and management theories |
33 | [ ] | To analyze how the introduction of autism into a home and the availability of intervention options change the structure and meaning of a home and reflect parental acceptance of a child’s autistic traits | Parental experiences of autism in Kerala, India, and Atlanta, GA USA | Observations |
34 | [ ] | This article examines challenges for human resource management posed by workplace adaptations for individuals with LD (learning disabilities) | Neurodiversity and human resource management | - |
- Honeybourne, V. The Neurodiverse Workplace: An Employer’s Guide to Managing and Working with Neurodivergent Employees, Clients and Customers ; Jessica Kingsley Publishers: London, UK, 2019. [ Google Scholar ]
- Hyland, S.; Connolly, J. Companies Leading in Disability Inclusion Have Outperformed Peers. Accenture Research Finds. Available online: https://newsroom.accenture.com/news/2018/companies-leading-in-disability-inclusion-have-outperformed-peers-accenture-research-finds (accessed on 4 July 2024).
- Jefferies, J.G.; Ahmed, W. Marketing Neurodiversity for Well-Being. J. Consum. Mark. 2022 , 39 , 632–648. [ Google Scholar ] [ CrossRef ]
- Whelpley, C.E.; Perrault, E. Autism at Work: How Internal and External Factors Influence Employee Outcomes and Firm Performance. J. Gen. Manag. 2021 , 46 , 210–219. [ Google Scholar ] [ CrossRef ]
- Vogus, T.J.; Taylor, J.L. Flipping the Script: Bringing an Organizational Perspective to the Study of Autism at Work. Autism 2018 , 22 , 514–516. [ Google Scholar ] [ CrossRef ] [ PubMed ]
- Campos-García, I.; Alonso-Muñoz, S.; González-Sánchez, R.; Medina-Salgado, M.-S. Human Resource Management and Sustainability: Bridging the 2030 Agenda. Corp. Soc. Responsib. Environ. Manag. 2024 , 31 , 2033–2053. [ Google Scholar ] [ CrossRef ]
- Ehnert, I.; Harry, W.; Zink, K.J. (Eds.) Sustainability and Human Resource Management. Developing Sustainable Business Organizations ; Springer: Berlin/Heidelberg, Germany, 2014. [ Google Scholar ] [ CrossRef ]
- Sarkis, J.; Sundaram, A. An Examination of Sustainable Human Resource Management Practices in Developing Countries. J. Clean. Prod. 2016 , 114 , 664–673. [ Google Scholar ]
- Flamini, G.; Gnan, L. Sustainable HRM. In Organizing Sustainable Development ; Routledge: London, UK, 2023; pp. 172–186. [ Google Scholar ] [ CrossRef ]
- Singer, J. Odd People in: The Birth of Community Amongst People on the Autism Spectrum: A Personal Exploration of a New Social Movement Based on Neurological Diversity ; University of Technology: Sydney, Australia, 1998. [ Google Scholar ]
- Armstrong, T. The Power of Neurodiversity: Unleashing the Advantages of Your Differently Wired Brain ; Da Capo Press: Philadelphia, PA, USA, 2011. [ Google Scholar ]
- Rothstein, A. Review: Mental Disorder or Neurodiversity? New Atlantis 2012 , 36 , 99–115. [ Google Scholar ]
- Singer, J. Why Can’t You Be Normal for Once in Your Life? From a Problem with No Name to the Emergence of a New Category of Difference. In Disability Discourse ; Corker, M., French, S., Eds.; Open University Press: Buckingham, UK, 1999; pp. 59–67. [ Google Scholar ]
- Hendrickx, S. The Adolescent and Adult Neuro-Diversity Handbook: Asperger’s Syndrome, ADHD, Dyslexia, Dyspraxia, and Related Conditions ; Jessica Kingsley Publishers: London, UK, 2010. [ Google Scholar ]
- McGee, M. Neurodiversity. Contexts 2012 , 11 , 12–13. [ Google Scholar ] [ CrossRef ]
- Maenner, M.J.; Shaw, K.A.; Baio, J.; Washington, A.; Patrick, M.; DiRienzo, K.; Christensen, D.L.; Wiggins, D.L.; Pettygrove, S.; Andrews, J.G.; et al. Prevalence of Autism Spectrum Disorder Among Children Aged 8 Years—Autism and Developmental Disabilities Monitoring Network, 11 Sites, United States, 2016. CDC MMWR Surveill. Summ. 2020 , 69 , 1–16. [ Google Scholar ] [ CrossRef ] [ PubMed ]
- Polskiautyzm.pl. Available online: https://polskiautyzm.pl/ (accessed on 28 June 2024).
- Supreme Audit Office. Available online: https://www.nik.gov.pl/plik/id,23545.pdf (accessed on 28 June 2024).
- Wiater, M. Innowacje Społeczne w Obszarze Neuroróżnorodności. Eduk. Ekon. i Menedżerów 2022 , 64 , 37–49. Available online: https://econjournals.sgh.waw.pl/EEiM/article/view/2989 (accessed on 28 June 2024). [ CrossRef ]
- Whelpley, C.E.; Banks, G.C.; Bochantin, J.E.; Sandoval, R. Tensions on the Spectrum: An Inductive Investigation of Employee and Manager Experiences of Autism. J. Bus. Psychol. 2020 , 36 , 283–297. [ Google Scholar ] [ CrossRef ]
- Morath, E. America’s Hidden Workforce Returns; Disabled Workers Join Job Market at Highest Level in Years Amid Low Unemployment and Stricter Rules for Claiming Benefits. Wall Street Journal. Available online: https://www.wsj.com/articles/americas-hidden-workforce-returns-11548478801 (accessed on 28 June 2024).
- American Psychiatric Association. Diagnostic and Statistical Manual of Mental Disorders , 5th ed.; American Psychiatric Publishing: Arlington, VA, USA, 2013. [ Google Scholar ]
- Smith, M.D.; Belcher, R.G.; Juhrs, P.D. A Guide to Successful Employment for Individuals with Autism ; Brookes Publishing Company: Baltimore, MD, USA, 1997. [ Google Scholar ]
- Austin, R.D.; Pisano, G.P. Neurodiversity as a Competitive Advantage. Harv. Bus. Rev. 2017 , 95 , 96–103. [ Google Scholar ]
- Howlin, P.; Goode, S.; Hutton, J.; Rutter, M. Adult Outcome for Children with Autism. J. Child Psychol. Psychiatry 2004 , 45 , 212–229. [ Google Scholar ] [ CrossRef ] [ PubMed ]
- Krumm, N.; Turner, T.N.; Baker, C.; Vives, L.; Mohajeri, K.; Witherspoon, K.; Raja, A.; Coe, B.P.; Stessman, H.A.; He, Z.X.; et al. Excess of Rare, Inherited Truncating Mutations in Autism. Nat. Genet. 2015 , 47 , 582–588. [ Google Scholar ] [ CrossRef ] [ PubMed ]
- Gerhardt, P.F.; Lainer, I. Addressing the Needs of Adolescents and Adults with Autism: A Crisis on the Horizon. J. Contemp. Psychother. 2011 , 41 , 37–45. [ Google Scholar ] [ CrossRef ]
- Polish Economic Institute; JiM Foundation. Raport Praca Autyzm Jim Pik ; Polish Economic Institute: Warsaw, Poland, 2022; p. 4. Available online: https://jim.org/en/raport_praca_autyzm_jim_pik/ (accessed on 28 June 2024).
- Wiater, M. Wyzwania Związane z Zatrudnianiem Osób Neuroróżnorodnych w Polsce. HRM Zarz. Zasob. Ludzk. 2023 , 154 , 80–92. [ Google Scholar ]
- Fundacja Synapsis. Available online: https://synapsis.org.pl/ (accessed on 28 June 2024).
- Johnson, T.D.; Joshi, A. Dark Clouds or Silver Linings? A Stigma Threat Perspective on the Implications of an Autism Diagnosis for Workplace Well-Being. J. Appl. Psychol. 2016 , 101 , 430–449. [ Google Scholar ] [ CrossRef ] [ PubMed ]
- Lorenz, T.; Frischling, C.; Cuadros, R.; Heinitz, K. Autism and Overcoming Job Barriers: Comparing Job-Related Barriers and Possible Solutions In and Outside of Autism-Specific Employment. PLoS ONE 2016 , 11 , e0147040. [ Google Scholar ] [ CrossRef ] [ PubMed ]
- Neely, B.H.; Hunter, S.T. In a Discussion of Invisible Disabilities, Let Us not Lose Sight of Employees on the Autism Spectrum. Ind. Organ. Psychol. 2014 , 7 , 274–277. [ Google Scholar ] [ CrossRef ]
- Hill, E.L. Linking Clinical and Industrial Psychology: Autism Spectrum Disorder at Work. Ind. Organ. Psychol. 2014 , 7 , 152–155. [ Google Scholar ] [ CrossRef ]
- Richards, J.; Sang, K.; Marks, A.; Gill, S. “I’ve Found It Extremely Draining” Emotional Labour and the Lived Experience of Line Managing Neurodiversity. Pers. Rev. 2019 , 48 , 1903–1923. [ Google Scholar ] [ CrossRef ]
- Ryan, R.M.; Deci, E.L. Self-Determination Theory and the Facilitation of Intrinsic Motivation, Social Development, and Well-Being. Am. Psychol. 2000 , 55 , 68–78. [ Google Scholar ] [ CrossRef ] [ PubMed ]
- Berwick, K.R. Stress Among Student Affairs Administrators: The Relationship of Personal Characteristics and Organizational Variables to Work-Related Stress. J. Coll. Stud. Dev. 1992 , 33 , 11–19. [ Google Scholar ]
- Conley, V.M. Separation: An Internal Aspect of the Staffing Process. Coll. Stud. Aff. J. 2001 , 21 , 57–63. [ Google Scholar ]
- Tull, A. Synergistic Supervision, Job Satisfaction, and Intention to Turnover of New Professionals in Student Affairs. J. Coll. Stud. Dev. 2006 , 4 , 465–480. [ Google Scholar ] [ CrossRef ]
- Snyder, H. Literature Review as a Research Methodology: An Overview and Guidelines. J. Bus. Res. 2019 , 104 , 333–339. [ Google Scholar ] [ CrossRef ]
- Paul, J.; Criado, A.R. The Art of Writing Literature Review: What Do We Know and What Do We Need to Know? Int. Bus. Rev. 2020 , 29 , 1–7. [ Google Scholar ] [ CrossRef ]
- Rollnik-Sadowska, E. Labor Market in Sustainability Transitions: A Systematic Literature Review. Econ. Environ. 2023 , 87 , 4. [ Google Scholar ] [ CrossRef ]
- Ejdys, J.; Szpilko, D. European Green Deal–Research Directions. Syst. Lit. Review. Econ. Environ. 2022 , 81 , 8–38. [ Google Scholar ] [ CrossRef ]
- Barkun, Y.; Rollnik-Sadowska, E.; Glińska, E. The Concept of ‘Talent’ in the Labor Management Perspective-The Bibliometric Analysis of Literature. Int. J. Ind. Eng. Manag. 2020 , 12 , 104–115. [ Google Scholar ] [ CrossRef ]
- Kaššaj, M.; Peráček, T. Synergies and potential of Industry 4.0 and automated vehicles in smart city infrastructure. Appl. Sci. 2024 , 14 , 3575. [ Google Scholar ] [ CrossRef ]
- Krzeminska, A.; Austin, R.; Bruyére, S.; Hedley, D. The Advantages and Challenges of Neurodiversity Employment in Organizations. J. Manag. Organ. 2019 , 25 , 453–463. [ Google Scholar ] [ CrossRef ]
- Morrison, K.E.; DeBrabander, K.M.; Jones, D.R.; Ackerman, R.A.; Sasson, N.J. Social Cognition, Social Skill, and Social Motivation Minimally Predict Social Interaction Outcomes for Autistic and Non-Autistic Adults. Front. Psychol. 2020 , 11 , 1–22. [ Google Scholar ] [ CrossRef ] [ PubMed ]
- Stenn, T.; Lalor, A.R.; Coplan, J.; Osterholt, D.A. Managing neurodiversity inclusion in today’s entrepreneurial-styled workplace. In Managing for Social Justice. Harnessing Management Theory and Practice for Collective Good ; Poonamalle, L., Howard, A.D., Joy, S., Eds.; Springer International Publishing: Cham, Switzerland, 2023. [ Google Scholar ]
- Doyle, N.; McDowall, A. Diamond in the Rough? An ‘Empty Review’ of Research into ‘Neurodiversity’ and a Road Map for Developing the Inclusion Agenda. Equal. Divers. Incl. 2022 , 41 , 352–382. [ Google Scholar ] [ CrossRef ]
- Muhammad, R.; Omkar, D.; Duan, X. Leadership Strategies for Effective Diversity Management ; IGI Global: Hershey, PA, USA, 2023. [ Google Scholar ] [ CrossRef ]
- Khan, M.H.; Grabarski, M.K.; Ali, M.; Buckmaster, S. Insights into Creating and Managing an Inclusive Neurodiverse Workplace for Positive Outcomes: A Multistaged Theoretical Framework. Group Organ. Manag. 2023 , 48 , 1339–1386. [ Google Scholar ] [ CrossRef ]
- Szulc, J.M.; McGregor, F.-L.; Cakir, E. Neurodiversity and Remote Work in Times of Crisis: Lessons for HR. Pers. Rev. 2023 , 52 , 1677–1692. [ Google Scholar ] [ CrossRef ]
- Howes, S.S. Emotional Intelligence in Autistic Adults: A Review with Considerations for Employers. Sustainability 2023 , 15 , 7252. [ Google Scholar ] [ CrossRef ]
- Doyle, N.; Bradley, E. Disability Coaching in a Pandemic. J. Work. -Appl. Manag. 2023 , 15 , 135–147. [ Google Scholar ] [ CrossRef ]
- Doyle, N.; McDowall, A. Neurodiversity Coaching: A Psychological Approach to Supporting Neurodivergent Talent and Career Potential , 1st ed.; Routledge: London, UK, 2023. [ Google Scholar ] [ CrossRef ]
- Allen, R.A.; Dickmann, M.; Priscott, T.; White, G.R.T. Exploring Positive and Negative Intersectionality Effects: An Employment Study of Neurodiverse UK Military Veterans. Int. J. Hum. Resour. Manag. 2023 . [ Google Scholar ] [ CrossRef ]
- Crook, T.; McDowall, A. Paradoxical Career Strengths and Successes of ADHD Adults: An Evolving Narrative. J. Work. Manag. 2023 , 16 , 112–126. [ Google Scholar ] [ CrossRef ]
- Waterbury, C.J.; Smith, N. Invisible Diversity in the Hospitality Industry. In The Routledge Handbook of Diversity, Equity, and Inclusion Management in the Hospitality Industry ; Routledge: London, UK, 2023; pp. 128–139. [ Google Scholar ]
- Branton, S.E.; Villamil, A.M.; Reed, J.L. Branding Neurodiversity: A Critical Discourse Analysis of Communicative Capitalism and Change Empowerment Among Neurodiversity Workforce Intermediaries. J. Public Relat. Res. 2023 , 35 , 357–374. [ Google Scholar ] [ CrossRef ]
- Warnock, R. Relational Legacies and Relative Experiences: Austerity, Inequality, and Access to Special Educational Needs and Disability (SEND) Support in London, England. Geogr. J. 2023 , 1–12. [ Google Scholar ] [ CrossRef ]
- Whelpley, C.E.; Woznyj, H.M. Balancing the Teeter Totter: A Dialectical View of Managing Neurodiverse Employees. Hum. Perform. 2023 , 36 , 133–154. [ Google Scholar ] [ CrossRef ]
- Szulc, J.M. Towards More Inclusive Qualitative Research: The Practice of Interviewing Neurominorities. Labour Ind. 2023 , 33 , 179–187. [ Google Scholar ] [ CrossRef ]
- Turowetz, J.; Wiscons, L.Z.; Maynard, D.W. Disorder or Difference? How Clinician-Patient Interaction and Patient Age Shape the Process and Meaning of Autism Diagnosis. Sociol. Heal. Illn. 2023 , 46 , 171–188. [ Google Scholar ] [ CrossRef ] [ PubMed ]
- Tomczak, M.T. How Can the Work Environment Be Redesigned to Enhance the Well-Being of Individuals with Autism? Empl. Relat. 2022 , 44 , 1467–1484. [ Google Scholar ] [ CrossRef ]
- Spoor, J.R.; Flower, R.L.; Bury, S.M.; Hedley, D. Employee Engagement and Commitment to Two Australian Autism Employment Programs: Associations with Workload and Perceived Supervisor Support. Equal. Divers. Incl. 2022 , 41 , 508–524. [ Google Scholar ] [ CrossRef ]
- Hinder, A.M. Stellar Evolution: Career Paths of Autistic Professionals in Corporate Law Firms. In Emerald Studies in Workplace Neurodiversity ; Generation, A., Hurley-Hanson, A.E., Giannantonio, C.M., Eds.; Emerald Publishing Limited: Leeds, UK, 2022; pp. 65–82. [ Google Scholar ] [ CrossRef ]
- Molloy, A.; O‘Donoghue, A.; Fu, N. Enabling Neurodiversity in the Workplace via Inclusive Human Resource Practices. In Emerald Studies in Workplace Neurodiversity ; Generation, A., Hurley-Hanson, A.E., Giannantonio, C.M., Eds.; Emerald Publishing Limited: Leeds, UK, 2022; pp. 85–109. [ Google Scholar ] [ CrossRef ]
- Karol, E.; Smith, D. Design Considerations for Residents with Impeded Cognitive Functioning: Conversations with People with Schizophrenia. Sustainability 2021 , 13 , 7733. [ Google Scholar ] [ CrossRef ]
- Walkowiak, E. Neurodiversity of the Workforce and Digital Transformation: The Case of Inclusion of Autistic Workers at the Workplace. Technol. Forecast. Soc. Chang. 2021 , 168 , 120739. [ Google Scholar ] [ CrossRef ]
- Roberson, Q.; Quigley, N.R.; Vickers, K.; Bruck, I. Reconceptualizing Leadership from a Neurodiverse Perspective. Group Organ. Manag. 2021 , 46 , 399–423. [ Google Scholar ] [ CrossRef ]
- Oseland, N. Beyond the Workplace Zoo: Humanising the Office , 1st ed.; Routledge: London, UK, 2021. [ Google Scholar ] [ CrossRef ]
- Szulc, J.M.; Davies, J.; Tomczak, M.T.; McGregor, F.-L. AMO Perspectives on the Well-Being of Neurodivergent Human Capital. Empl. Relat. 2021 , 43 , 858–872. [ Google Scholar ] [ CrossRef ]
- Priscott, T.; Allen, R.A. Human Capital Neurodiversity: An Examination of Stereotype Threat Anticipation. Empl. Relat. 2021 , 43 , 1067–1082. [ Google Scholar ] [ CrossRef ]
- Saline, S. Thriving in the New Normal: How COVID-19 Has Affected Alternative Learners and Their Families and Implementing Effective, Creative Therapeutic Interventions. Smith Coll. Stud. Soc. Work. 2021 , 91 , 1–28. [ Google Scholar ] [ CrossRef ]
- Kwok, K.; Kwok, D.K. More than Comfort and Discomfort: Emotion Work of Parenting Children with Autism in Hong Kong. Child. Youth Serv. Rev. 2020 , 118 , 105456. [ Google Scholar ] [ CrossRef ]
- Aubineau, M.; Blicharska, T. High-Functioning Autistic Students Speak about Their Experience of Inclusion in Mainstream Secondary Schools. Sch. Ment. Health 2020 , 12 , 537–555. [ Google Scholar ] [ CrossRef ]
- Carrero, J.; Krzeminska, A.; Härtel, C.E.J. The DXC Technology Work Experience Program: Disability-Inclusive Recruitment and Selection in Action. J. Manag. Organ. 2019 , 25 , 535–542. [ Google Scholar ] [ CrossRef ]
- Patton, E. Autism, Attributions and Accommodations: Overcoming Barriers and Integrating a Neurodiverse Workforce. Pers. Rev. 2019 , 48 , 915–934. [ Google Scholar ] [ CrossRef ]
- Sarrett, J.C. Custodial Homes, Therapeutic Homes, and Parental Acceptance: Parental Experiences of Autism in Kerala, India, and Atlanta, GA USA. Cult. Med. Psychiatry 2015 , 39 , 254–276. [ Google Scholar ] [ CrossRef ] [ PubMed ]
- Sumner, K.E.; Brown, T.J. Neurodiversity and Human Resource Management: Employer Challenges for Applicants and Employees with Learning Disabilities. Psychol.-Manag. J. 2015 , 18 , 77–85. [ Google Scholar ] [ CrossRef ]
Click here to enlarge figure
Cluster Number | Cluster Name | Key Words |
---|---|---|
1 | Gender-Specific Mental Health and Quality of Life in Autism Spectrum Disorder | Article, autism spectrum disorder, female, human, male, mental health, quality of life, well-being |
2 | Inclusive Human Engineering and Neurodiversity in Disability Studies | Disability, human engineering, inclusion, neurodiversity |
3 | Psychological Perspectives on Autism and Employment in the Workplace | Autism, employment, psychology, workplace |
4 | Advanced Strategies in Human Resource Management: Recruitment and Selection | Human resource management, recruitment, selection |
The statements, opinions and data contained in all publications are solely those of the individual author(s) and contributor(s) and not of MDPI and/or the editor(s). MDPI and/or the editor(s) disclaim responsibility for any injury to people or property resulting from any ideas, methods, instructions or products referred to in the content. |
Share and Cite
Rollnik-Sadowska, E.; Grabińska, V. Managing Neurodiversity in Workplaces: A Review and Future Research Agenda for Sustainable Human Resource Management. Sustainability 2024 , 16 , 6594. https://doi.org/10.3390/su16156594
Rollnik-Sadowska E, Grabińska V. Managing Neurodiversity in Workplaces: A Review and Future Research Agenda for Sustainable Human Resource Management. Sustainability . 2024; 16(15):6594. https://doi.org/10.3390/su16156594
Rollnik-Sadowska, Ewa, and Violetta Grabińska. 2024. "Managing Neurodiversity in Workplaces: A Review and Future Research Agenda for Sustainable Human Resource Management" Sustainability 16, no. 15: 6594. https://doi.org/10.3390/su16156594
Article Metrics
Article access statistics, further information, mdpi initiatives, follow mdpi.
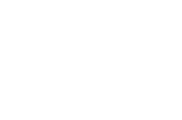
Subscribe to receive issue release notifications and newsletters from MDPI journals
Cookies on GOV.UK
We use some essential cookies to make this website work.
We’d like to set additional cookies to understand how you use GOV.UK, remember your settings and improve government services.
We also use cookies set by other sites to help us deliver content from their services.
You have accepted additional cookies. You can change your cookie settings at any time.
You have rejected additional cookies. You can change your cookie settings at any time.
- Government efficiency, transparency and accountability
Civil Service Pay Remit Guidance 2024 to 2025
- Cabinet Office
Published 29 July 2024
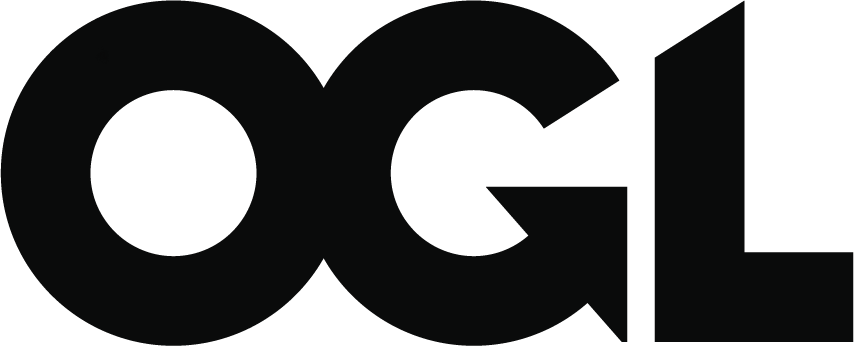
© Crown copyright 2024
This publication is licensed under the terms of the Open Government Licence v3.0 except where otherwise stated. To view this licence, visit nationalarchives.gov.uk/doc/open-government-licence/version/3 or write to the Information Policy Team, The National Archives, Kew, London TW9 4DU, or email: [email protected] .
Where we have identified any third party copyright information you will need to obtain permission from the copyright holders concerned.
This publication is available at https://www.gov.uk/government/publications/civil-service-pay-remit-guidance-2024-to-2025/civil-service-pay-remit-guidance-2024-to-2025
1. Scope and Purpose of the Pay Remit Guidance
This guidance covers pay setting arrangements for civil servants throughout the Civil Service, including departments, non-ministerial departments and agencies, as well as for public sector workers in non-departmental public bodies [footnote 1] and other arm’s length bodies. [footnote 2]
- Where reference is made to departments it also includes those organisations which are not government departments but are in scope of the Pay Remit Guidance.
- Where reference is made to civil servants, it also includes references to other workers employed in organisations covered by this guidance.
The guidance provides a framework within which all departments will set pay for 2024/25 and for departmental pay strategies and pay reporting. This guidance does not apply to departments which are already in approved arrangements outside of the Pay Remit Guidance, including those for which multi-year deals extending into the 2024/25 pay year have been agreed. A glossary of terms used in the guidance can be found in Section 7.
2. Key factors determining the 2024/2025 pay remit
2.1 introduction , context and 2024/2025 headline figure.
This year, departments are able to make average pay awards up to 5%.
This 2024/25 pay remit year is the last of a three year spending settlement for departments. It is critical that departments consider which priorities are best addressed in the final year of the current Spending Review, and how these relate to and facilitate longer-term objectives and wider workforce priorities without limiting options under the next Spending Review. This year, departments have flexibility to target their pay awards in a way which best suits their needs. This will enable departments (and other organisations covered by its scope) to provide their workforces with a significant consolidated increase, whilst also providing flexibility to address pay anomalies or wider workforce issues, such as recruitment and retention challenges. Departments should have particular regard to such issues as addressing pay compression due to National Living Wage (NLW) increases.
Departments must ensure that pay awards are affordable within their current spending settlements. Departments should be aware of the need to balance other budgetary pressures with consideration of the wider economy and the Government’s macroeconomic framework.
The percentage increase will refer to overall average pay awards within the department. Individuals may receive a higher or lower award, as it is for departments to decide how to target their pay award based on their own workforce and business needs, and the criteria as set out above.
Increase in Remuneration Costs (IRC)
Organisations are reminded that all elements which increase paybill cost must be included in the calculation of a pay award, except employer National Insurance contributions and employer pension contributions. The following must be included:
- Re-valorisation of paybands
- any remaining historic progression increments
- introduction of new allowances
- increases to existing allowances
- cost of increases in the non-consolidated performance pot above its existing proportion of total paybill
- non-consolidated payments (except for payments related to performance from the non-consolidated performance pot)
- buy-out of allowances or non-pay entitlements
- incentive payments relating to the implementation of pay reforms
- cost associated with changes in non-pay benefits/rewards (e.g. increasing annual/sick/maternity/paternity/parental leave entitlements, introducing long service or non pay recognition awards)
- increases arising from pay restructuring (e.g. associated with Machinery of Government changes or repositioning staff within the pay range)
salary sacrifice schemes [footnote 3]
Exceptions to Increase in Remuneration Costs
- Buying and selling annual leave schemes - where increased costs associated with the scheme will be offset against additional productivity.
- Costs associated with employee benefits from cross-government benefits framework - costs associated with employee benefits from the cross-government benefits framework or a departmental benefits framework
- Carers leave - From 1 April 2024, the cost of introducing the legislative requirement of one week unpaid leave for care provision
As set out above, if a department makes any changes to elements of their paybill this forms part of their total paybill. If that presents an increase in remuneration costs (IRC) for the remit year above the controls set out in this guidance, i.e. 5%, then further approval is required from Cabinet Office and HM Treasury Ministers.
Departments should contact the Cabinet Office for advice if they are unsure whether any changes they are considering would present an IRC.
Cabinet Office and HM Treasury will consider requests from departments for pay flexibility proposals in very exceptional circumstances, and where they meet the requirements set out in Section 3. Departments are encouraged to discuss any potential business case with the Cabinet Office at the earliest opportunity and before starting detailed work on any pay flexibility business case, in the first instance at [email protected] . The deadline to submit a case for the 2024/25 remit year is no later than 31 October 2024.
Pay remits can only be reopened in exceptional cases once the relevant Secretary of State has approved them. For example, organisations may be able to make an award within remit whilst awaiting the outcome of a pay flexibility case. Organisations must seek approval from the Cabinet Office and HM Treasury prior to implementing an interim award.
2.2 National Minimum Wage & National Living Wage
From 1 April 2024, departments must ensure that they apply the legislative increase to the National Living Wage (NLW) and National Minimum Wage (NMW). The cost of raising individuals to the new rates can be met outside departments’ pay remit.
2.3 Holiday Pay
Employees (including specific arrangements for irregular hours and part-year workers) are entitled to holiday, paid at a rate which reflects their normal earnings, so that they do not see a reduction in earnings when on annual leave. This calculation may include regular overtime, including using the statutory 52 week reference point for the calculation, and organisations should ensure that they are fully compliant with their legal obligations.
Increases in remuneration made specifically to fulfil the legal obligation to include payments such as regularly worked overtime in statutory holiday calculations should be considered outside of the headline pay awards, providing that the increases are made only to the extent that they fulfil the legal requirements.
If a department wishes to clarify the position on Holiday Pay, and further advice is required, they should contact the Cabinet Office using the contact details in Section 6. Any element of such payments from departments that exceeds the required legal obligation should expect to seek explicit HM Treasury consent, before the payment is made, as per the processes detailed in Managing Public Money .
2.4 Carer’s Leave
The Carer’s Leave Act 2023, which came into effect on 6 April 2024 and entitles employees to take 1 week unpaid leave every 12 months as a non-pay benefit to help a dependent who needs long-term care. The cost of implementing unpaid carers leave can be met outside departments’ pay remit.
2.5 Performance-Related Pay Awards
Non-consolidated performance pay will continue to be managed, as in previous years, within each department’s non-consolidated performance pay ‘pot’ calculated as a fixed percentage of paybill. Public sector organisations are encouraged to target their funds to ensure there are enough staff to deliver vital public services. In exceptional circumstances and with the agreement of HM Treasury and the Cabinet Office, departments have the option of transferring money between their consolidated and non-consolidated pots as set out below in Section 3.2.
Requests to increase the size of the non-consolidated performance pay ‘pot, permanently or temporarily, will only be considered in exceptional circumstances. Consideration of this change will be dependent on factors including the current size of a department’s non-consolidated performance pay ‘pot’ and the proposed increase, as well as the justification for change. If an organisation’s non-consolidated ‘pot’ has been previously reduced to fund higher consolidated pay, further requests will not be considered.
2.6 Recyclable Savings
Employer National Insurance and pension contributions saved as a result of salary sacrifice schemes, where such schemes are permitted by HMT, are recyclable savings and can offset against the IRC of a 2024/25 pay award.
3. Pay Flexibility
The Government wants to ensure that it is attracting the best and brightest to work for the Civil Service, and rewarding hard working staff fairly. It is important that pay awards ensure sustainability of public finances, deliver value for money for the taxpayer and consider economic conditions. Departments should also consider how their long-term pay strategy delivers on workforce priorities and efficiencies. Since 2017/18, the Pay Remit Guidance has permitted departments to submit a pay flexibility business case to HM Treasury and Cabinet Office ministers to make awards for delegated grades above the headline figure in the Pay Remit Guidance, only where departments can demonstrate productivity gains and efficiencies in return for a high pay award.
This year, departments are able to make average pay awards of up to 5%. Departments have flexibility within this figure to provide their workforces with a substantive consolidated pay increase to address any recruitment and retention issues or pay anomalies.
As a result of this, the normal Pay Flexibility Process will be paused for 2024/25.
Requests for pay flexibility will only be considered in very exceptional circumstances, for example, where a department is experiencing acute recruitment and retention issues that create a risk to service delivery. These cases should be limited to one year as it is the last year of the 2021 Spending Review.
Departments considering a request for pay flexibility should contact the Pay and Reward team in Government People Group, [email protected] .
Where there are very exceptional circumstances and departments request pay flexibility, cases should be cost neutral in the medium-term, with details to be set out in a business case. This means that any additional costs should be offset by delivering savings, which may include tangible productivity and efficiency gains. Proposals will be considered where savings and efficiencies to the paybill which cover the IRC can be met within the business case lifecycle or in the medium-term.
Below is a summary of the types of business case that can be submitted:
- transformational pay and workforce reform, including pay restructuring as a result of Machinery of Government changes
- to address recruitment and retention issues (including adopting the Digital, Data, Technology and Cyber pay framework)
- transfer of funds from the non-consolidated pay pot to consolidated pay, or increasing the size of the non-consolidated pay pot in exceptional cases
3.1 Pay Flexibility Process
As outlined above, cases for pay flexibility will only be considered in very exceptional circumstances.
Where departments feel that they meet this threshold, business cases for pay flexibility are subject to approval by Cabinet Office ministers, with subsequent sign-off by the Chief Secretary to the Treasury. Departments are required to seek their own relevant minister’s approval before submitting their proposals using the pay flexibility pro forma.
Departments must also receive approval from their Permanent Secretary and Human Resources and Finance Directors.
The deadline for submitting pay flexibility proposals for 2024/25 is 31 October 2024.
Non-ministerial departments and agencies, as well as for public sector workers in non-departmental public bodies (NDPBs), and other arm’s length bodies can also request pay flexibility in very exceptional circumstances. Proposals should be submitted to the Cabinet Office through their relevant sponsor department’s Secretary of State.
Where departments consider that their circumstances are very exceptional and have submitted a case for pay flexibility, they should await the outcome of Cabinet Office and HM Treasury decisions before paying any award for their workforce. In exceptional cases, subject to approval from Cabinet Office and HM Treasury officials, departments may be able to make an interim award. Accounting Officers should ensure that any interim award made does not affect the affordability of the pay case or exceed the headline award permitted by this guidance and would not result in overpayments to individuals in the event that Cabinet Office and HM Treasury ministers reject the pay flexibility proposals.
Departments should have removed automatic progression pay based on time-served from their workforces and it should not be reintroduced. Any progression pay still in place in core departments or their ALBs not agreed through business case approvals will be in breach of government policy and Cabinet Office and HM Treasury must be notified immediately.
Going forward, departments should ensure that pay arrangements they put in place do not involve automatic time-served progression pay, or create an entitlement for employees to receive automatic incremental pay increases.
3.2 Types of Business Case
Recruitment and retention.
Where there are exceptional circumstances, departments may seek targeted pay flexibility to address specific problems associated with recruitment and retention in specific grades and/or professions within their department. These cases must include associated efficiencies and reforms to deliver sustainable savings going forward. Organisations must include robust evidence in support of pay proposals and consider the wider current economic and labour market context. Proposals must also be strictly targeted and involve prioritisation.
The degree of labour turnover and recruitment deemed to be problematic will vary by department and by grade and profession of staff. Departments will need to demonstrate (with robust relevant data) that recruitment and retention problems are exceptional and severely risk service delivery, and the degree to which any turnover problems are associated with pay rather than other wider organisational factors.
Where departments are citing staff motivation as a contributor to turnover, they must demonstrate whether these problems are associated with pay, for example, through evidence from surveys of staff and exit interviews. Departments should expect to be challenged on whether alternative measures are more appropriate to address the issues they have identified. Departments can also consider a business case to adopt the Digital & Data pay framework to address specific recruitment and retention challenges (see below).
Transfer of funds from the non-consolidated pot to consolidated pay
Departments and NDPBs are permitted, in exceptional circumstances, to reduce their non-consolidated performance related pay (PRP) pot permanently as a percentage of consolidated paybill to offset agreed increases in paybill costs applied to meet targeted recruitment or retention pressures, and to address pay anomalies.
The permitted reduction in the PRP pot is capped at an amount equivalent to 50% of the pot, or 0.5% of the baseline paybill if smaller, in order to ensure that departments maintain sufficient resources to continue to fund non-consolidated performance arrangements. PRP pots may not be reduced to provide additional funding for the consolidated paybill generally (for example to fund an across-the-board increase to staff), but must be targeted to address recruitment and retention pressures or pay anomalies.
Funding remaining within the ring-fenced PRP pot following an agreed reduction is expected to be applied to performance-related payments. To avoid this flexibility resulting in subsequent paybill increase, the reduction in the PRP pot will be permanent once the pot as a percentage of paybill has been reduced. Departments must be satisfied that their proposed reduction will not jeopardise the operation, development or effectiveness of their performance-related pay arrangements.
Requests to increase the size of the non-consolidated performance pay ‘pot’, permanently or temporarily, will be considered in exceptional circumstances. If an organisation’s non-consolidated PRP pot has been previously reduced to fund higher consolidated pay, further cases will not be considered.
Transformational
In previous years, departments have been able to submit proposals for transformational pay reform. Departments may seek to take forward transformational pay reform to address structural issues or support delivery of workforce reform. This may include pay restructuring which is necessary as a result of Machinery of Government changes.
In 2024/25, such proposals will only be considered in cases where there is evidence that such reforms are necessary to address acute recruitment and retention issues and to ensure the continued delivery of vital public services.
In these cases, proposals are expected to be offset by delivering sustainable savings, which may include tangible productivity and efficiency gains. Such proposals will only be considered where there is a clear case that reforms will generate transformation in departmental and public service delivery, and are expected to be offset by delivering sustainable savings, which may include tangible productivity and efficiency gains. This may include, for example, reduction in contingent labour, savings from reduced turnover, recyclables, changes to terms and conditions of employment, or other productivity and efficiency savings.
Departments should also consider delivery of Spending Review priorities when formulating a business case.
Departments must discuss options with the Cabinet Office before formally submitting a business case. The assessment of a business case will take into account the individual circumstances of the department.
Capability-based pay frameworks
Departments have previously been permitted to seek approval from Cabinet Office and HM Treasury to introduce arrangements that enable movement through pay bands based on achievement of higher workforce productivity e.g. growth of capability.
In 2024/25, work will be undertaken to analyse the impact of these arrangements where they have been implemented. Following this, further guidance will be given in future versions of the Pay Remit Guidance in relation to the implementation of capability-based pay frameworks as part of multi-year pay flexibility cases.
As such, departments that submit requests for pay flexibility in 2024/25 as a result of very exceptional circumstances should not submit requests for the introduction of capability-based pay frameworks in the 2024/25 remit year.
The pay framework for Digital & Data, and for Cyber staff
Departments will continue to be able to submit a business case to the Cabinet Office for approval to adopt the Digital & Data Pay Framework, without the need to demonstrate very exceptional circumstances. The Pay Framework enables departments to pay capability-based allowances to recruit and retain staff in hard to fill digital and data, and cyber roles.
Business cases to adopt the pay framework are funded outside the headline pay remit by recycling savings from reducing contingent labour use. Departments should discuss options with the pay team in the Central Digital & Data Office before submitting a business case. The cost of paying allowances under the framework does not count towards the calculation of Increase in Remuneration Cost.
Digital & Data Pay Framework business cases do not require HM Treasury ministerial approval where they align with the pre-agreed Digital and Data Pay Framework. Any case which seeks to adopt this Pay Framework requires departmental ministerial approval.
Departments looking to adopt the Digital & Data pay framework should first discuss their business case with the pay team in the Central Digital & Data Office - [email protected] .
3.3 Business Case Assessment
As set out above, requests for pay flexibility will only be considered in this remit year where departments can demonstrate that there are exceptional circumstances which make flexibility necessary to ensure the continued effective delivery of public services.
If departments believe that this threshold is met, the business case produced must demonstrate that they have considered the following headline principles throughout their proposals:
- Transformational and targeted
- Delivers efficiencies and productivity
- Evidence-based
These principles should be met and are applicable specifically to the following business cases:
Transformational workforce reform
- Transformational and targeted: proposals should deliver long-term focused transformational changes to departmental delivery and, therefore, significant improvements in productivity and/or resolve specific problems encountered by departments. Proposals must consider how a pay strategy may support delivery of Spending Review workforce priorities, deliver productivity and efficiency gains, savings including through changes to terms and conditions of employment, and other recyclables.
- Delivers efficiencies and productivity: Proposals are expected to be cost neutral in the medium-term and, as set out in the business case, should not place a cost pressure on the department’s budgets, and should deliver on Spending Review priorities. This means that any additional costs should be offset by delivering savings, which may include tangible productivity and efficiency gains. Proposals should be accompanied by a holistic workforce strategy including detail on how proposed reforms will enable workforce targets to be met, and are also expected to provide sustainable and measurable workforce productivity gains.
- Evidence-based: proposals should demonstrate robust evidence in the form of data to back up the proposals. For example, showing low levels of pay that are below relevant comparators, and how this has translated into significant recruitment and retention problems (including relevant statistics). Proposals should demonstrate robust evidence that the pay proposals will have a front line impact on business delivery, outcomes, and productivity. Proposals should also provide evidence that demonstrates expected efficiencies and savings resulting from the duration of the pay deal.
- Measurable: timelines and milestones must be provided to enable the tracking of the pay reform to ensure savings and impacts are being achieved.
- Coherence: proposals must take into account wider Civil Service context and departments may decide it is appropriate for them to look towards more consistent approaches and policies. In particular, business cases are encouraged to look at where historic divergence between departments has made reward systems more complex, less agile, less fair to employees and less efficient overall to the taxpayer.
- Equality: Compliance with age discrimination and equal pay legislation, and ensuring an equality impact assessment has been undertaken when developing proposals.
Addressing recruitment and retention issues
- Transformational and targeted: Proposals should address specific problems associated with recruitment and retention in specific grades and professions within their department. Proposals should also deliver sustainable savings going forward and clearly demonstrate associated efficiencies and reforms.
- Delivers efficiencies and productivity: proposals are expected to deliver savings and efficiencies to the paybill which cover the Increase in Remuneration Cost (IRC), and do not place a cost pressure on the department’s budgets. Proposals are also expected to provide sustainable workforce productivity gains.
- Evidence-based: proposals should demonstrate low levels of pay that are below relevant comparators and how this has translated into significant recruitment and retention problems (including relevant statistics) that severely risk service delivery, and the degree to which any turnover problems are associated with pay rather than other wider organisational factors. Proposals must also demonstrate clear evidence that the pay discrepancy will have a front line impact on business delivery, outcomes, and productivity.
- Coherence: proposals may take into account wider Civil Service context and departments may decide it is appropriate for them to look towards more consistent approaches and policies. In particular, business cases are encouraged to look at where historic divergence between departments has made reward systems more complex, less agile, less fair to employees and less efficient overall to the taxpayer.
Transfer of funds from the non-consolidated pay pot to consolidated pay
- Transformational and targeted: proposals should address specific problems associated with recruitment and retention in specific grades and professions within their department. Proposals should demonstrate that funds are being targeted to ensure there are enough staff to deliver vital public services.
- Delivers efficiencies and productivity: proposals are expected to deliver productivity and efficiency savings which cover the Increase in Remuneration Cost. Proposals are also expected to provide sustainable workforce productivity gains. Proposals should demonstrate that the proposed reduction of the non-consolidated pay pot will not jeopardise the operation, development or effectiveness of their performance-related pay arrangements.
- Evidence-based: proposals should demonstrate low levels of pay that are below relevant comparators and how this has translated into significant recruitment and retention problems (including relevant statistics) and robust evidence that the pay discrepancy will have a front line impact on business delivery, outcomes, and productivity. Proposals should provide evidence that demonstrates expected efficiencies and savings resulting from the duration of the pay discrepancy.
- Coherence: proposals may take into account wider Civil Service context and departments may decide it is appropriate for them to look towards more consistent approaches and policies. In particular, business cases are encouraged to look at where historic divergence between departments has made reward systems more complex, less agile, less fair to employees and less efficient overall to the taxpayer.
All business cases must also include a detailed plan for implementation, including departmental Trade Union engagement.
4. Pay Remit Process and Approval
4.1 responsibilities, cabinet office and hm treasury.
Cabinet Office has responsibility for the overall management of the Civil Service. It is responsible for the publication of the Civil Service Pay Remit Guidance and ensuring that it is sufficiently affordable and flexible for all relevant departments to apply within their budgets. It works with departments and agencies on their workforce and reward strategies to encourage them in implementing tailored reward strategies that are consistent with their workforce and business needs, ensuring that they are able to attract and retain talent to deliver the Government’s priorities and world class public services.
HM Treasury has overall responsibility for the Government’s public sector pay and pensions policy, and maintaining control over public spending including with regards to departmental spending. Departments who have previously been granted clearance can continue the paybill control system going forward. HM Treasury will continue to keep the use of paybill control under review and will not consider new proposals this remit year.
Departments
Departments have responsibility for implementing Civil Service pay policy for their workforce in a way that is consistent with the Civil Service pay guidance but also reflects the needs of their business and their labour market position. All pay remits must be approved by a Secretary of State or responsible minister, and each department, through its accounting officer, is responsible for the propriety of the pay award to staff, including their obligations as set out in Section 4.3.
Trade Unions
Departments are responsible for negotiating the annual pay remit with their recognised Trade Unions in line with the delegated pay framework. As a matter of course, departments are encouraged to work constructively with recognised Trade Unions on the development of their overall pay, reward and workforce strategies, including annual pay remits and the development of pay flexibility business cases. Once the annual pay remit has been agreed by the relevant Secretary of State, departments can enter formal negotiations with the Trade Unions. If departments require guidance or advice about what constitutes ‘formal negotiation’, they should contact the Cabinet Office.
4.2 The Remit Process
Departments, NDPBs and Agencies are required to submit a short business case to their relevant Secretary of State or appropriate Minister for approval. The only exceptions to this are Government Communications Headquarters, the Secret Intelligence Service and the Security Service where there is no Secretary of State with authority to determine pay. Their annual pay remits will continue to require HM Treasury ministerial approval.
No further approval from Cabinet Office or HM Treasury is necessary unless specific arrangements or requirements are already in place, or a department is looking to implement a pay award outside of the remit set by this guidance (such as a pay flexibility business case) at which point they should contact Cabinet Office and refer to the guidance in Section 3.
Pay flexibility business case to the Secretary of State
In very exceptional circumstances where departments have decided to submit proposals for pay flexibility, departments should ensure that clearance is sought from the relevant HR and Finance Directors, and Permanent Secretary prior to submission to the Secretary of State.
Secretaries of State should consider the proposals against the following criteria:
- Whether the circumstances within which the department finds itself are exceptional enough as to make flexibility necessary to ensure the continued effective delivery of public services.
- Departments need to demonstrate that their proposed pay remit is affordable within departmental budgets and through savings generated as a result of further flexibility in line with the guidance at Section 3.
- The requirements in Section 3 of this guidance must be met.
- information on how the business case is consistent with the department’s overall workforce, pay and reward strategy, and Spending Review priorities.
- information on the makeup of the department’s workforce, and the internal and external labour market in which they operate
- the relevant local labour market in which staff operate
- the recruitment and retention situation within the workforce
- Equality Act requirements to avoid discrimination, including the need for departments to meet their obligations under the Public Sector Equality Duty, and to record their findings on this
- the total reward of staff, including pensions and conditions of service
- any structural changes or reforms to pay arrangements
- information on how the department is meeting key long-term priorities
Departments should also work constructively with Trade Unions on the development of their overall pay and reward strategies, and may find it helpful to discuss proposals with Trade Unions as they are developed. Following approval from the relevant Secretary of State, departments should enter formal negotiations with their departmental Trade Unions. However, if during subsequent negotiations with Trade Unions there are any significant deviations from those proposals, then these must be reported to the Secretary of State and, as appropriate, Cabinet Office or HM Treasury before any final agreement is reached. Any department that is uncertain as to whether what they propose to agree constitutes a significant difference from that specified under the remit should contact the Cabinet Office for advice.
Pay settlement changes are expected to apply from the settlement date upon which the department’s pay remit year commences, and should not be deferred.
4.3 Legal Considerations for Departments
Departments are reminded of their obligation to comply with their Public Sector Equality Duty when considering pay awards for their staff and the requirements of the HR Functional Standard (including adherence to all legal and regulatory requirements).
Departments are also expected to apply this guidance alongside the HM Treasury guidance on public sector pay and terms .
Departments are encouraged to take legal advice on the drafting of any pay commitments to ensure that these are affordable and consistent with this guidance.
Ministerial approval of pay remits is given on the basis that a department does not enter into any legally binding agreements in Trade Union negotiations that effectively commit it to automatic costs in the future.
4.4 Senior Staff
Senior Civil Servants (SCS) and NDPB equivalents are not included within the scope of this Civil Service pay guidance. The pay of the SCS is covered by the Senior Salaries Review Body’s (SSRB) remit and is set centrally by the Cabinet Office. Pay for senior staff in NDPBs is not covered by the SSRB’s remit, nevertheless NDPBs have an important leadership role in following public sector pay policy, therefore, any annual pay increase or decision to award performance-related pay to such staff must be considered alongside and according to the same principles that apply to the SCS. Sponsor departments are responsible for enforcing this. The latest SCS Pay Practitioners Guide can be found on Gov.uk.
5. Pay Reporting to HMT
In return for the continued delegation of pay to Secretaries of State, departments are expected to provide data to HM Treasury on their forecast and outturn data for the pay round.
Departments are asked to submit this data using the Workforce and Pay Remit (WPR) application in OSCAR for each remit. Further guidance will be issued to departments on the WPR including the deadline for submission of data.
Failure by a department to provide appropriate data, or provide it in good time, may result in re-imposing the requirement for approval of remits for that department in future years or other action to encourage better compliance.
The information below summarises the data that departments are expected to report to HM Treasury for the 2024/25 remit year:
Each department should submit data covering the department itself, each non-ministerial department falling within the area of responsibility of their Secretary of State, each agency that they sponsor and each NDPB. Departments should not in general combine data relating to separate remits. Exceptionally, aggregate data may be entered for NDPBs with prior HM Treasury agreement.
The data required is outturn for 2022/23 and 2023/24, as well as a forecast for 2024/25. Data should be provided for the financial year, not the remit year. Outturn data should be consistent with published resource accounts (allowing for differences arising from the financial year basis of the latter) and forecasts, including any forecast changes to staff in post, should be consistent with the in-year expenditure forecasts that all central departments routinely provide to HM Treasury via the OSCAR system. Data must be provided for the delegated Civil Service grades and equivalents, and the Senior Civil Service and equivalent grades.
If there are significant anomalies in the data, for example because it represents less than a full year of activity, or there are large differences between successive forecast and outturn figures for a particular year, departments should provide a brief explanation, either in the space provided on OSCAR or in an email to the Workforce Pay and Pensions team at HM Treasury (see Section 6 for contact details).
HM Treasury will issue a commission directly to departments to formally begin the WPR process. This will include all relevant guidance and information.
6. Contacts
Any queries in relation to this guidance, or the remit process in general, should be emailed to the Cabinet Office in the first instance:
Email: [email protected]
Civil Service Reward and Employment Team Government People Group Cabinet Office, Zone 10/11 4th Floor 1 Horse Guards Road London SW1A 2HQ
For queries on WPR reporting: [email protected]
Workforce, Pay and Pensions Team HM Treasury, Zone 2 Red 1 Horse Guards Road London SW1A 2HQ
7. Glossary of Terms
7.1 total paybill (£ million).
Total paybill: Total paybill is the sum of direct wages and salaries, pension contributions and National Insurance Contributions. Includes all staff- related costs excluding the cost of staff not on the formal Civil Service payroll. This should include staff paid from programme budgets.
Direct wages and salaries: Direct wages and salaries are calculated from the sum of pay, allowances, non-consolidated performance (e.g. bonuses) and overtime entered in the OSCAR template. Direct wages and salaries include all the elements that go to employees on a current basis (as opposed to pension payments, for example, which are deferred).
Pension contributions: The total cost to the department of pension contributions.
Employer National Insurance Contributions: The total cost to the department of employer National Insurance Contributions.
Exit costs: These include any benefits to an individual, in the form of pay, pension or other remuneration, when leaving service (through either compulsory redundancy or other agreed departures) other than entitlements accrued in the normal course of employment up to and including the exit date.
7.2 Non-paybill staff costs (£ million)
Consultants/interim/agency staff costs not included in the paybill: Total cost of all staff who are not on the formal Civil Service payroll, including for example, consultants, interim and agency staff.
7.3 Non-consolidated performance pay pot (% of paybill)
Non-consolidated performance pay pot (% of paybill): The size of the non-consolidated performance pay pot expressed as a percentage of total pay bill. Note that this may differ from outturn or forecast expenditure on non-consolidated pay recorded as a component of salaries and wages.
7.4 Paybill per head (£)
Paybill per head: There are two measures to show the total paybill divided by the workforce size. Paybill per head (headcount) is total paybill divided by headcount. Paybill per head (FTE) is total paybill divided by full-time equivalent (FTE) workforce.
Average earnings per head: There are two measures to show direct wages and salaries i.e. excluding employer pensions and NICs) divided by the workforce size. Average earnings per head (headcount) is direct wages and salaries divided by headcount. Average earnings per head (FTE) is direct wages and salaries divided by FTE workforce.
7.5 Workforce size
Headcount and FTE must be calculated on the required basis for all years.
Headcount: The total Civil Service workforce on a headcount basis, calculated as the average for the remit year based on the size of the workforce at the end of each month.
Full time equivalent: The total Civil Service workforce on a full-time equivalent basis, calculated as the average for the remit year based on the size of the workforce at the end of each month.
Number of exit packages: The number of exit packages made within the year. This should relate to the ‘exit costs’ figure reported under paybill.
7.6 Wastage and vacancies (%)
Vacancy Rates: The number of unfilled posts (or forecast vacancies) in the final month of the remit year in question, expressed as a percentage of (headcount at the end of the month plus vacancies). This figure should reflect the number of posts that are either unfilled or filled by contract staff, which will be advertised under Civil Service fair and open competition rules. This figure should not include vacant posts that will be removed as part of organisational restructuring or planned workforce reductions.
Staff wastage: The proportion of employees leaving the department over the year (excluding redundancies but including exits due to all other factors including performance related exits and ill-health retirements) as a % of total workforce. Excludes those employees moving between jobs within the department.
7.7 Change in pay from previous year (%)
Basic award (%): The average % increase to the steps (for a step based pay system) or maxima/minima (for a non-step based pay system) of the pay ranges within an organisation.
Pay drift (%): The difference between average earnings growth % and basic award %.
Average earnings growth (%): The change in average earnings per head (FTE) from the previous year, as a % of average earnings per head in the previous year. It includes all changes in direct wages and salaries.
Percentage increase in remuneration cost (IRC) (%): The percentage change in the IRC. This is the difference between the projected remuneration cost and the baseline remuneration cost expressed as a percentage of the baseline remuneration cost. All departments should enter data for all three calculations of the IRC:
- Increase in remuneration cost
- Increase in remuneration cost excluding legally binding progression increments or progression buy out costs
- Increase in remuneration cost excluding progression increments or progression buy out costs, and PRP pot switches
For those departments to whom the below do not apply, the figures will be identical:
- departments that pay contractual progression increments or
- departments who have agreed a switch in funding from the non-consolidated performance pay pot to fund recruitment and retention pressures
Those departments that fall under either or both of the above are expected to report the effects of these factors on their IRC by entering outturn and forecast data for all three versions of the IRC.
7.8 National Living Wage (NLW)
Number of employees on the NLW: The number of eligible employees that are on (or are within 5 pence of) the NLW from April in the pay remit year. For example, as of April 2024, the rate of the National Living Wage is £11.44.
Paybill costs of those on the NLW: The total paybill costs incurred by all employees on the NLW within the pay remit year and the cost of increasing the wages of eligible employees to meet the legal entitlement of paying the NLW.
7.9 Baseline remuneration cost
The baseline remuneration cost is the cost to the department, for the remit year, of the expected staff complement, excluding the costs of progression or revalorisation or any other increases. Baseline remuneration cost does not include employer National Insurance and pension contributions.
7.10 Projected remuneration cost
The projected remuneration cost is calculated by adding the IRC arising from the remit proposals to the baseline remuneration cost.
Example: if the total costs of the remit proposals were £100,000 and the baseline costs were £2,000,000 the projected costs would be £2,100,000.
7.11 Increase in remuneration cost (IRC)
As stated in Section 2, the IRC includes all increases arising from the remit proposals, apart from employer National Insurance Contributions and pension contributions, and is net of any offsetting reductions in the costs.
The IRC calculation should assume that all increases are implemented from the settlement date. Departments cannot reduce the IRC by deferring the date of implementation of component increases.
7.12 Non-consolidated performance payments
Non-consolidated performance payments are awarded to staff based on performance either at an individual, team or organisational level. They are re-earnable and do not have associated future costs. Types of payment include:
- Performance related payments based on individual contributions to the department and assessed by the department’s performance management system
- Special bonus schemes for individual payments for special projects or outstanding pieces of work that are not covered by the normal performance management system
- Non-consolidated performance payments met from the performance pot should be excluded from the IRC calculation. However, non-consolidated payments not related to performance, as well as increases in the non-consolidated performance pot, must be included in the IRC calculation.
7.13 Calculating the performance pot
The organisation’s existing non-consolidated performance pot is a cash value derived from a percentage of the consolidated baseline paybill, and not a fixed cash amount.
Example: In 2023-24, an organisation has a consolidated paybill of £20 million and has built up a non-consolidated performance pot of 3%. The cash value of the non-consolidated pot is therefore 3% of £20 million, and so equals £600,000. In 2024/25, because of staff reductions, the consolidated baseline paybill is reduced to £19 million. While the non-consolidated pot as a proportion of consolidated paybill remains unchanged at 3%, the cash value is reduced to £570,000 (3% of £19 million).
7.14 Other non-consolidated payments
Non-consolidated payments other than those related to performance must be included in the IRC calculation.
7.15 Progression Pay
Progression pay systems are those under which pay to individuals in a specific grade or post increases periodically. Progression pay cost is the cost of moving someone through the pay range; for spine point or step-based systems, it relates to the costs of incremental steps. In some cases this is subject to demonstration of increased capability (to a particular standard), a satisfactory performance assessment and/or may be a legal entitlement. Progression may also be as a result of targeting a pay award. In milestone and reference-point based systems, progression means the cost of moving staff within the pay range.
7.16 Revalorisation
Revalorisation relates to the uprating of pay ranges, spine points or step-based systems and is the value by which set points are increased. The cost of any revalorisation must be included in the pay award, i.e. the IRC calculation.
7.17 Non-pay rewards and benefits
These include increases in annual leave entitlements, reduction in working hours, etc. The cost of such changes should be calculated and included in the IRC calculation.
7.18 Remit year
The period for which the approved pay remit applies. Remits apply for one year but the settlement dates, i.e. the date upon which the pay remit year commences, vary from one body to another.
7.19 Recyclable Savings
Recyclable savings are generated when staff leave the department and are replaced by entrants with a lower salary cost. The difference between the leaver’s salary costs and the entrant’s salary costs is the saving to the paybill.
Employer National Insurance and pension contributions saved as a result of salary sacrifice schemes are also recyclable savings and can be added back into the paybill.
Vacant posts do not generate recyclable savings, because until the post is filled the salary cost to the paybill cannot be determined.
- Throughout the guidance the term “department(s)” includes all organisations (ministerial and non-ministerial departments, agencies and NDPBs) that come within its scope, unless the context implies otherwise, and departments should seek clarity as necessary.
- Organisations should contact their parent or sponsor department to seek guidance from the Cabinet Office (contact details found in Section 6) if unsure whether they are in scope of the guidance.
- A letter from the Financial Secretary to Secretaries of State on 3 November 2009 clarified the rules on salary sacrifice schemes in the public sector. Departments should refer to this if needed.
Is this page useful?
- Yes this page is useful
- No this page is not useful
Help us improve GOV.UK
Don’t include personal or financial information like your National Insurance number or credit card details.
To help us improve GOV.UK, we’d like to know more about your visit today. Please fill in this survey (opens in a new tab) .
Determinants of Turnover Intention among Employees
- February 2015

- Universiti Teknologi MARA
- This person is not on ResearchGate, or hasn't claimed this research yet.
Abstract and Figures

Discover the world's research
- 25+ million members
- 160+ million publication pages
- 2.3+ billion citations
- Amrit Kumar Sharma Gaire
- MERVE KAPLAN

- Ibrahim Alkandari
- Farah Alsaeed
- Anwaar Al-Kandari

- حاكم جبوري الخفاجي
- ولاء محسن علي الحاتمي
- سامي غانم كاظم
- Pimlapas Pongsakornrungsilp

- Antony Lees

- D W N Sandunika

- Hazrina Ghazali and Nurul Izzah Anuar

- Ahmad Shobirin

- Allen C. Bluedorn
- PETER W. HOM
- LORIANN ROBERSON
- AIMEE D. ELLIS
- J. Nunnally
- I. Bernstein
- James M. White

- S.M. Abbasi
- K.W. Hollman
- Recruit researchers
- Join for free
- Login Email Tip: Most researchers use their institutional email address as their ResearchGate login Password Forgot password? Keep me logged in Log in or Continue with Google Welcome back! Please log in. Email · Hint Tip: Most researchers use their institutional email address as their ResearchGate login Password Forgot password? Keep me logged in Log in or Continue with Google No account? Sign up
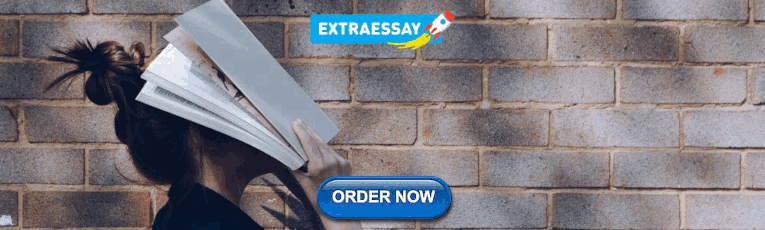
COMMENTS
several factors cause employee turnovers, such as c hanges in. management style, tension with other employees, and distrust. [44], [55], [56]. Besides, a lack of leadership management. strength ...
This study aims to examine the relationship model of job satisfaction, organizational commitment, and turnover intention. This research was conducted at the manufacturing company in Yogyakarta and Surakarta, with a sample of 206 employees. Testing four models of the relationship is done by using structural equation modeling with AMOS program.
Abstract. This qualitative proposal is intended to address the potential challenges that high employee turnover rates create in manufacturing organizations and the potential impact of using ...
INTRODUCTION. Voluntary employee turnover (hereafter turnover) is as old as employment itself, but as a subject of academic inquiry has existed for just over a century (Diemer, 1917; Fisher, 1917).Competition for skilled employees and episodic labour market shortages coupled with skills mismatches necessitate better understanding of turnover (WEF, 2020).
This study mainly investigates the effects o f job satisfaction on employee turnover as applied. to the chosen organization, the Oman Air. Main findings revealed that among the main factors ...
Female employees have comparatively higher-level job satisfaction [8]. This study implies that employees age 31 to 40 years have high employee turnover intention. The research findings are similar to Lu, Lu [8]; the older employees have high intentions to leave the company." Reviewer 1 Comment 24: Practical implications need further discussion.
Turnover has been and still is regarded as a critical issue in the management of an organization by many managers. As discussed by Grinza (Citation 2014), most managers appear to fear it; a Google search of 'employee turnover' mainly yields HR sites offering guidelines on how to retain employees and consequently reduce turnover. The main ...
Employee turnover is a common problem for many organizations. There have been a multitude of studies that explore turnover and an employee's intent to leave. A review of the relevant literature was conducted with specific focus on employee retention and the role that organizational culture plays on these factors.
Some scholars have proposed an attenuated negative relationship between employee turnover and organizational performance. From this perspective, when employee turnover is low, employees' average firm-specific human capital is high, and thus it takes a considerable amount of time for new hires to reach the average level of human capital. In con-
Turnover intention is an employee's reported willingness to leave her organization within a given period of time and is often used for studying actual employee turnover. Since employee turnover can have a detrimental impact on business and the labor market at large, it is important to understand the determinants of such a choice. We describe and analyze a unique European-wide survey on ...
Henry Ongori. Department of Management, University of Botswana, Botswana. E-mail: [email protected]. Accepted 22, May 2007. "Employee turnover" as a term is widely used in business circles. Although several studies have been conducted on this topic, most of the researchers focus on the causes of employee turnover but little has been done ...
turnover on organizational performance and analysed that employee turnover had a. mployeeturnover that included lack of employee retention strategies. low leve. motivation, lack of career development and poor working environment. Her study. recommended that the organizationa human resource management should employ.
Owing this issue, " Assessment of Factor of Employee's Turnover " was proposed for research with the objective of examination of factors causing staff resignation from MVi. In total 26 staff both women and men who resigned 2016 and 2017 was selected for interview. Quantitative data was used.
A turnover rate is the percentage of employees that a company must replace within a given time period. This proportion is a concern to most firms because employee turnover can be an expensive, especially for organizations under expansion, which typically has the highest turnover rates.
Employee turn over is the. rotation of workers around the labour market; between. firms, jobs and occupations; and between the sta tes of. employment and unem ployment Abassi et al. (2000). The ...
Hathaway (2013) stated that there was an increase in employee turnover in the United States of 200,000 employee turnovers a month from 2001 to 2011. Employee turnover in the retail industry costs each employer in the United States about $190,000 each year (Harrison & Gordan, 2014). Research on strategies for decreasing employee turnover
employee turnover (Bonenberger, Aikins, Akweongo, & Wyss, 2014). Further research might help employers understand different strategies to lessen employee turnover, specifically in the field of Child Protective Services (CPS). Background of the Problem Employee turnover is damaging to the sustainability of business organizations
Sayed Research Proposal Employee Turnover. research proposal. Module. Research Methodology and Proposal (BUSN11094) 133 Documents. Students shared 133 documents in this course. University University of the West of Scotland. Academic year: 2016/2017. Uploaded by: nazmul islam. University of the West of Scotland. 0 followers.
Before conducting a study, a research proposal should be created that outlines researchers' plans and methodology and is submitted to the concerned evaluating organization or person. Creating a research proposal is an important step to ensure that researchers are on track and are moving forward as intended. A research proposal can be defined as a detailed plan or blueprint for the proposed ...
This research goes farther than previous efforts in evaluating the scientific development of articles in employee turnover, including a closer look at the individuals and methods used in the process.
An analytical cross-sectional study was conducted on 354 nurses in five hospitals affiliated with Ardabil University of Medical Sciences from May to August 2023. This research used web-based software to design demographic information forms, nurse manager competency scale, turnover intention, and single-item job satisfaction questionnaires.
An analysis of 34,000 responses to the Work Institute's 2017 Retention Report found that 75% of the reasons for employee turnover can be prevented. The most common reason employees leave their jobs is because they are not being challenged at work. This includes feeling under-appreciated and bored with what you do every day.
This review article explores the scientific literature on managing neurodiversity in workplaces, aiming to identify emerging research directions, gaps, and methods used. A systematic literature review in the hybrid form was implemented, combining bibliometric analysis and structured review. A review of selected social sciences publications from the SCOPUS database was conducted. The literature ...
WASHINGTON, D.C., July 31, 2024 - U.S. Senators Katie Britt (R-Ala.) and Tim Kaine (D-Va.), a member of the Senate Health, Education, Labor and Pensions (HELP) Committee, introduced the Child Care Availability and Affordability Act and the Child Care Workforce Act—two pieces of legislation that together form the boldest bipartisan proposal to make child care […]
analysis, the research found employee ' s turnover has a significant cause on performance of the organiz ation. Table 2: Reliability Test and Average Mean I ndex Statistics.
7. Glossary of Terms 7.1 Total paybill (£ million). Total paybill: Total paybill is the sum of direct wages and salaries, pension contributions and National Insurance Contributions.
The possible negative impact of turnover to organizations might be due to high. cost of recruiting, training and getting new employees, productivit y loss d uring replacement. search and retaining ...