Lung Cancer Research Results and Study Updates
See Advances in Lung Cancer Research for an overview of recent findings and progress, plus ongoing projects supported by NCI.
In a study of people with advanced lung cancer, palliative care delivered via telehealth was just as effective at improving patients’ quality of life and other measures of well-being as care delivered in person.
Lorlatinib (Lorbrena) is superior to crizotinib (Xalkori) as an initial treatment for people with ALK-positive advanced non-small cell lung cancer, according to new clinical trial results. Treatment with lorlatinib also helped prevent new brain metastases.
The immunotherapy drug durvalumab (Imfinzi) can help people with early-stage small cell lung cancer live longer, results from a large clinical trial show. Three years after starting treatment, nearly 60% of people who received the drug were still alive.
FDA has approved alectinib (Alecensa) as adjuvant therapy for people with lung cancer who have ALK-positive tumors. In a clinical trial, alectinib helped people live longer after surgery without their cancer returning than chemotherapy.
The results of the clinical trial that led to FDA’s 2023 approval of repotrectinib (Augtyro) for lung cancers with ROS1 fusions have been published. The drug shrank tumors in 80% of people receiving the drug as an initial treatment.
A collection of material about the ALCHEMIST lung cancer trials that will examine tumor tissue from patients with certain types of early-stage, completely resected non-small cell lung cancer for gene mutations in the EGFR and ALK genes, and assign patients with these gene mutations to treatment trials testing post-surgical use of drugs targeted against these mutations.
Tarlatamab, a new type of targeted immunotherapy, shrank small cell lung cancer (SCLC) tumors in more than 30% of participants in an early-stage clinical trial. Participants had SCLC that had progressed after previous treatments with other drugs.
For people with lung cancer and medullary thyroid cancer whose tumors have changes in the RET gene, selpercatinib improved progression-free survival compared with other common treatments, according to new clinical trial results.
In the ADAURA clinical trial, people with early-stage lung cancer treated with osimertinib (Tagrisso) after surgery lived longer than people treated with a placebo after surgery. Despite some criticisms about its design, the trial is expected to change patient care.
For certain people with early-stage non-small cell lung cancer, sublobar surgery to remove only a piece of the affected lung lobe is as effective as surgery to remove the whole lobe, new research shows.
Pragmatica-Lung is a clinical trial for people with non-small cell lung cancer that has spread beyond the lungs (stage 4 cancer). The trial will help confirm if the combination of pembrolizumab and ramucirumab helps people with advanced lung cancer live longer.
On August 11, the Food and Drug Administration (FDA) gave accelerated approval to trastuzumab deruxtecan (Enhertu) for adults with non-small cell lung cancer (NSCLC) that has a specific mutation in the HER2 gene. Around 3% of people with NSCLC have this kind of HER2 mutation.
Giving people with early-stage lung cancer the immunotherapy drug nivolumab (Opdivo) and chemotherapy before surgery can substantially delay the progression or return of their cancer, a large clinical trial found.
Atezolizumab (Tecentriq) is now the first immunotherapy approved by FDA for use as an additional, or adjuvant, treatment for some patients with non-small cell lung cancer. The approval was based on results of a clinical trial called IMpower010.
Quitting smoking after a diagnosis of early-stage lung cancer may help people live longer, a new study finds. The study, which included more than 500 patients, also found that quitting smoking delayed the cancer from returning or getting worse.
NCI scientists and their international collaborators have found that the majority of lung cancers in never smokers arise when mutations caused by natural processes in the body accumulate. They also identified three subtypes of lung cancer these individuals.
FDA has approved the first KRAS-blocking drug, sotorasib (Lumakras). The approval, which covers the use of sotorasib to treat some patients with advanced lung cancer, sets the stage for other KRAS inhibitors already in development, researchers said.
Combining the chemotherapy drug topotecan and the investigational drug berzosertib shrank tumors in some patients with small cell lung cancer, results from an NCI-supported phase 1 clinical trial show. Two phase 2 trials of the combination are planned.
Mortality rates from the most common lung cancer, non-small cell lung cancer (NSCLC), have fallen sharply in the United States in recent years, due primarily to recent advances in treatment, an NCI study shows.
In a study of more than 50,000 veterans with lung cancer, those with mental illness who received mental health treatment—including for substance use—lived substantially longer than those who didn’t participate in such programs.
FDA has granted accelerated approval for selpercatinib (Retevmo) to treat certain patients with thyroid cancer or non-small cell lung cancer whose tumors have RET gene alterations. The drug, which works by blocking the activity of RET proteins, was approved based on the results of the LIBRETTO-001 trial.
Osimertinib (Tagrisso) improves survival in people with non-small cell lung cancer with EGFR mutations, updated clinical trial results show. People treated with osimertinib lived longer than those treated with earlier-generation EGFR-targeted drugs.
A large clinical trial showed that adding the immunotherapy drug durvalumab (Imfinzi) to standard chemotherapy can prolong survival in some people with previously untreated advanced small cell lung cancer.
The investigational drug selpercatinib may benefit patients with lung cancer whose tumors have alterations in the RET gene, including fusions with other genes, according to results from a small clinical trial.
FDA has approved entrectinib (Rozlytrek) for the treatment of children and adults with tumors bearing an NTRK gene fusion. The approval also covers adults with non-small cell lung cancer harboring a ROS1 gene fusion.
Clinical recommendations on who should be screened for lung cancer may need to be reviewed when it comes to African Americans who smoke, findings from a new study suggest.
Use of a multipronged approach within hospitals, including community centers, not only eliminated treatment disparities among black and white patients with early-stage lung cancer, it also improved treatment rates for all patients, results from a new study show.
In everyday medical care, there may be more complications from invasive diagnostic procedures performed after lung cancer screening than has been reported in large studies.
The Lung Cancer Master Protocol, or Lung-MAP, is a precision medicine research study for people with advanced non-small cell lung cancer that has continued to grow after treatment. Patients are assigned to different study drug combinations based on the results of genomic profiling of their tumors.
On December 6, 2018, the Food and Drug Administration (FDA) approved atezolizumab (Tecentriq) in combination with a standard three-drug regimen as an initial treatment for advanced lung cancer that does not have EGFR or ALK mutations.
A new study has identified a potential biomarker of early-stage non–small cell lung cancer (NSCLC). The biomarker, the study’s leaders said, could help diagnose precancerous lung growths and early-stage lung cancers noninvasively and distinguish them from noncancerous growths.

An official website of the United States government
The .gov means it's official. Federal government websites often end in .gov or .mil. Before sharing sensitive information, make sure you're on a federal government site.
The site is secure. The https:// ensures that you are connecting to the official website and that any information you provide is encrypted and transmitted securely.
- Publications
- Account settings
- Browse Titles
NCBI Bookshelf. A service of the National Library of Medicine, National Institutes of Health.
Wild CP, Weiderpass E, Stewart BW, editors. World Cancer Report: Cancer research for cancer prevention. Lyon (FR): International Agency for Research on Cancer; 2020.

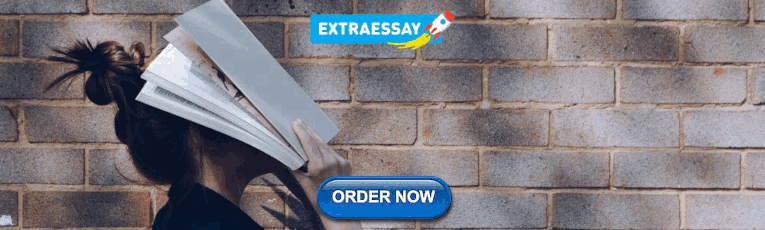
World Cancer Report: Cancer research for cancer prevention.
5.1 lung cancer.
Rayjean J. Hung , Adi F. Gazdar , and Joanna Didkowska , reviewer. Mattias Johansson , reviewer.
Lung cancer continues to be the most common cancer type and the leading cause of cancer death worldwide.
Relative to the hazards of smoking tobacco cigarettes, the hazards presented by e-cigarettes and by cannabis smoking are largely unknown.
The role of lung diseases, including chronic obstructive pulmonary disease and emphysema, in lung cancer is now clearer.
Several lung cancer susceptibility loci have been identified in the past decade, and more continue to be discovered through large-scale collaborations.
Comprehensive molecular profiling of adenocarcinoma, squamous cell carcinoma, and small cell carcinoma has been carried out. Some molecular changes provide druggable targets.
Lung cancer in never-smokers is a specific disease entity.
Lung cancer screening by low-dose computed tomography in high-risk populations represents an opportunity for mortality reduction, but its efficiency will be improved by individual risk prediction.
Fundamentals.
There are four main histological types of lung cancer: adenocarcinoma, squamous cell carcinoma, small cell carcinoma, and large cell carcinoma, each with different morphological features, molecular characterization, and etiology; the most common types are adenocarcinoma and squamous cell carcinoma [ 1 ].
- Epidemiology
Lung cancer continues to be the leading cause of cancer death worldwide, accounting for about 18% of all cancer deaths [ 2 ]. The highest incidence rates of lung cancer are observed in parts of North America, in East Asia, and in parts of central and eastern Europe ( Fig. 5.1.1 ) [ 2 ]. Incidence rates in men have declined during the past four decades in most countries, whereas incidence rates in women continue to rise, with a few exceptions ( Fig. 5.1.2 ) [ 3 ]. Because lung cancer survival is low globally, in general the trends in mortality rates over time correspond to the trends in incidence rates.
Fig. 5.1.1
Global distribution of estimated age-standardized (World) incidence rates (ASR) per 100 000 person-years for lung cancer (A) in men and (B) in women, 2018.
Fig. 5.1.2
Age-standardized (World) incidence rates per 100 000 person-years by calendar year in selected countries for lung cancer (left) in men and (right) in women, circa 1978–2012.
Carcinogens
The major cause of lung cancer is tobacco smoking (see Chapter 2.1), which is responsible for 80–85% of lung cancer cases worldwide; tobacco smoke contains more than 7000 chemicals and at least 69 carcinogens, including polycyclic aromatic hydrocarbons, tobacco-specific nitrosamines, and benzene [ 4 , 5 ].
Tobacco smoking is known to have a stronger effect on squamous cell carcinoma and small cell lung carcinoma (SCLC) than on adenocarcinoma [ 6 ]. In addition, the effect of smoking on risk of squamous cell carcinoma and SCLC increases with increased smoking duration and decreases rapidly after smoking cessation. The effect of smoking on risk of adenocarcinoma decreases less rapidly after smoking cessation; this partly explains the increasing percentage of adenocarcinoma in countries that are in a late stage of the tobacco epidemic. Another contributor to the increase in lung adenocarcinoma in smokers is the introduction of filtered and low-tar or low-nicotine cigarettes [ 7 ].
Apart from tobacco smoking, about 29 agents have been recognized to cause lung cancer, with varying degrees of risk and prevalences of exposure. These include asbestos, silica, several heavy metals, and radon (see Chapter 2.10). In addition, indoor air pollution from household combustion of solid fuel and cooking fumes in poorly ventilated homes was established as a lung carcinogen, predominantly on the basis of studies in female never-smokers in Asia (see Chapter 4.3). More recently, outdoor air pollution, particulate matter in outdoor air pollution, and one specific pollutant – diesel engine exhaust – have each been classified by the IARC Monographs as carcinogenic to humans (Group 1), on the basis of consistency in large pooled analyses and prospective cohort studies (see Chapter 2.9). These agents can have increasing importance as causes of lung cancer, especially in never-smokers. The established lung cancer carcinogens are included in the list of IARC Monographs classifications [ 8 ] (see “Known causes of human cancer by organ site”, p. 45).
The prevalence of tobacco smoking has declined in most high-income countries during the past few decades [ 9 ]. Recently, alternative smoking products have become popular. In addition, the use of cannabis has been legalized in some countries. Therefore, recent research efforts related to putative lung cancer risk factors have focused on electronic nicotine delivery systems (also called e-cigarettes) and cannabis smoking.
To date, studies on e-cigarettes have been based predominantly on cell culture or animal studies, which have demonstrated that e-cigarettes have pulmonary toxicity, although to a much smaller extent than tobacco smoking [ 10 ]. Therefore, e-cigarettes are considered by some to be an effective tool for harm reduction. However, because very limited data are available in humans, much effort will be required to fully monitor the effect of e-cigarettes on lung cancer risk and nicotine addiction, given the increasing popularity of e-cigarettes as an alternative to tobacco cigarettes, particularly among young people [ 10 , 11 ].
Cannabis has been legalized in Canada, in 28 states of the USA for medicinal use, and in several European countries. Cannabis smoke has some of the same carcinogenic constituents as tobacco smoke, such as selected polycyclic aromatic hydrocarbons [ 12 ]. Therefore, several studies have been conducted to evaluate its potential association with risk of lung cancer [ 13 , 14 ]. However, most studies are limited by either potential underreporting or sparse data among heavy cannabis users, and therefore the possibility of an association in heavy users cannot be excluded [ 14 ].
Previous lung disease
In addition to the known lung carcinogens, previous lung diseases were shown to be associated with risk of lung cancer. In particular, it is well established that chronic obstructive pulmonary disease is associated with risk of lung cancer [ 15 ]; this association can be explained at least partly by shared etiology, such as tobacco smoking and chronic inflammation [ 16 ]. The International Lung Cancer Consortium conducted a series of pooled analyses based on 17 studies with a total of 24 607 lung cancer cases and 81 829 controls. Although a history of chronic obstructive pulmonary disease was shown to be associated with lung cancer risk, only emphysema was associated with risk of lung cancer in never-smokers, and this association persisted even when considering a history of emphysema 5–10 years before the diagnosis of lung cancer [ 17 ]. A similar association was found for pneumonia, based on a pooled analysis of 12 studies [ 17 ].
Genetic susceptibility
Although tobacco smoking is the main risk factor for lung cancer, only about 15% of smokers eventually develop lung cancer [ 18 ]. A genetic component of lung cancer etiology is recognized on the basis of familial studies, and the analyses either accounted for smoking or focused on never-smokers [ 18 ]. The familial relative risk of lung cancer is consistently estimated to be about 2-fold across several large cancer registries [ 19 ], and the heritability of lung cancer has been estimated as 18% [ 20 ]. Having a first-degree relative with lung cancer increases the risk of lung cancer by 1.25–1.5-fold in never-smokers [ 21 ].
High-penetrance genetic syndromes, such as Li–Fraumeni syndrome and hereditary retinoblastoma, are associated with increased risk of lung cancer [ 18 ]. In addition, high-penetrance germline mutations in EGFR and HER2 in predominantly never-smokers have recently been described [ 22 , 23 ]. However, these high-penetrance mutations only account for perhaps 1% of lung cancer cases.
In the past decade, genome-wide association studies (GWAS) (see Chapter 3.2) have identified several lung cancer susceptibility loci, including CHRNA3/5 , TERT-CLPTM1L , the HLA/MHC region, RAD52 , BRCA2 , and CHEK2 , with extensive validations [ 24 , 25 ]. A list of major lung cancer susceptibility loci for European descendants was reported in the most recent and largest GWAS analysis to date [ 25 ]. The loci identified so far accounted for about 12% of the familial relative risk of lung cancer.
Several large-scale GWAS analyses conducted in Asian populations have identified multiple Asian-specific lung cancer susceptibility loci, such as ROS1 , along with several loci in common with those found in European descendants, such as TERT-CLPTM1L. A detailed list of lung cancer susceptibility loci in both European and Asian populations is included in a recent review [ 26 ]. Data on the African American population are currently limited to a single study, which confirmed the association of the CHRNA5 and TERT-CLPTM1 loci with lung cancer [ 27 ].
- Somatic characteristics of histological types
Comprehensive genomic characterizations were conducted by the Cancer Genome Atlas Research Network for lung adenocarcinoma and squamous cell carcinoma [ 28 , 29 ]. Both tumour types showed a very high average tumour mutation burden of about 8–9 somatic mutations per megabase. In adenocarcinoma, mutations in KRAS were mutually exclusive with those in EGFR . EGFR mutations were the main mutations in adenocarcinoma in never-smokers ( Fig. 5.1.3B ), and KRAS mutations were the predominant mutations in adenocarcinomas arising in patients in Europe and North America, of which about 85% were ever-smokers (Fig. 1.5.3A). TP53 mutations occurred in 46% of adenocarcinomas [ 29 ] and in almost all squamous cell carcinomas, along with a variety of activating mutations, although none at very high frequencies [ 28 ]. Biallelic inactivation of TP53 and RB1 appears to be a universal feature of SCLC [ 30 ]. All three of these types of lung tumours have marked genomic complexity, including rearrangements and copy number variations.
Fig. 5.1.3
Mutation spectra by histological type of lung cancer, showing the percentage of samples with a mutation detected by automated analysis. “Unknown” refers to potentially druggable mutations and excludes tumour recessive genes including (more...)
The mutation spectra shown in Fig. 5.1.3 are markedly different by histological type, suggesting that they may arise via very different molecular pathways. In addition, spatial and temporal intratumour heterogeneity in the processes of genomic instability is an active new area of research, with potential value as a prognostic predictor. The morphological and molecular features of the main histological subtypes are described below.
Adenocarcinoma
Adenocarcinomas have more morphological heterogeneity than other types of lung cancer; a uniform terminology was recently proposed and has been widely accepted [ 31 , 32 ]. The new subtypes, along with their major morphological features and the presence of frequent gene mutations, are summarized in Table 5.1.1 and illustrated in Fig. 5.1.4 . However, most adenocarcinomas are composed of more than one subtype, and the tumours are classified by the most common subtype present [ 31 ].
Table 5.1.1
Lung adenocarcinoma subtypes, growth patterns, and frequent gene mutations.
Fig. 5.1.4
Morphological features of adenocarcinoma subtypes: (A) adenocarcinoma in situ, (B) acinar, (C) solid with mucin, (D) papillary, (E) micropapillary, and (F) mucinous.
The adenocarcinoma in situ subtype is characterized by lepidic (scale-like) growth along existing alveolar walls without underlying tissue invasion. The papillary subtype has fibrovascular cores, which distinguish it from the micropapillary subtype. The acinar subtype is frequent and has gland formation as its hallmark feature. These three subtypes have frequent EGFR mutations. The solid with mucin subtype is poorly differentiated and is associated with KRAS mutations or translocations in ALK , ROS , RET , and NTRK . The recently recognized micropapillary subtype lacks fibrovascular cores and may contain ALK or HER2 mutations. Mucinous carcinomas, although not an official subtype, are relatively rare and have frequent KRAS mutations.
Squamous cell carcinoma
Squamous cell carcinoma has three subtypes: keratinizing, non-keratinizing, and basaloid. The morphological difference between the keratinizing subtype and the non-keratinizing subtype, which is less well differentiated, is the presence or absence of visible keratin on histological examination. No other molecular features have been described that separate these two common subtypes. The basaloid subtype has cells that are morphologically similar to those found in the basal layer of the large airways and that have a specific mRNA expression profile [ 33 ]. The mutation spectrum for squamous cell carcinoma is shown in Fig. 5.1.3C .
Small cell lung carcinoma
SCLCs are aggressive carcinomas that originate from neuroendocrine cells in the bronchial epithelium. Only two SCLC subtypes are recognized: pure SCLCs and combined SCLCs. Combined SCLCs have a non-SCLC (NSCLC) component that consists of at least 10% of the tumour [ 34 ].
Epigenetics of lung cancer
The epigenetic landscape of lung cancer commences early during pathogenesis and consists of two major components: methylation and histone modifications (see Chapter 3.8) [ 35 ].
Global hypomethylation is a common feature of cancer. Smoking-related hypomethylation measured in pre-diagnostic blood samples was shown to be associated with increased risk of lung cancer, and the most consistently replicated change was in the AHRR gene [ 36 ]. DNA hypermethylation, mainly in the promoter region, is a major mechanism for the silencing of tumour suppressor genes, although it may also occur in the body of the gene, where it may result in gene activation. Several hundred genes are methylated in lung cancers, and the best studied and most frequently methylated genes are listed in Table 5.1.2 . Methylation results in inactivation of one allele, and the other allele is usually deleted.
Table 5.1.2
Frequent abnormalities of genes involved in the epigenetic regulation of lung cancers.
In addition to methylation, many covalent modifications can occur on the N-terminal tail that protrudes from each of the four histone proteins. Histone modifications target many key tumour suppressor genes. The major histone changes that characterize NSCLC are listed in Table 5.1.2 .
Although most epigenetic studies of lung cancer focus on NSCLC, the epigenetics of SCLC has both similarities and differences with NSCLC. In particular, EZH2 , a master regulator of transcription that affects DNA methylation via upregulation of DNA methyltransferases, is upregulated in many cancer types, including SCLC, where it plays a major role in tumour progression and is associated with poor prognosis. These findings have led to widespread efforts to therapeutically target EZH2 . The genetic and epigenetic somatic alterations of lung cancer have recently been reviewed [ 37 ].
- Lung cancer in never-smokers
Lung cancer in never-smokers is a specific disease entity, because there are significant differences in etiology and clinical characteristics between lung cancer in never-smokers versus ever-smokers. For example, adenocarcinomas are more prevalent in never-smoker patients with lung cancer [ 38 ]. In addition, lung cancers in never-smokers have different somatic characteristics ( Fig. 5.1.3 ). Most notably, never-smoker patients with lung cancer have a lower prevalence of KRAS mutations and a higher prevalence of EGFR mutations and show longer survival after treatment with EGFR inhibitors than do ever-smokers. Overall, there are extensive differences between smokers and never-smokers with regard to the tumour mutation landscape, burden, and affected genes; TP53 is the most extensively documented gene [ 39 ]. Other features that distinguish lung cancer in never-smokers and ever-smokers, such as methylation patterns, have also been reported [ 39 ].
- Prevention and mortality reduction
Currently, the best hopes for reducing lung cancer mortality are preventing smoking through effective tobacco control and promoting successful smoking cessation in current smokers. However, in populations where the prevalence of smoking is low, an increasing proportion of lung cancer occurs in never-smokers and former smokers.
The National Lung Screening Trial in the USA reported that the low-dose computed tomography (LDCT) screening reduced the lung cancer mortality by 20% in former and current smokers who were eligible to be screened, based on age (age 55 years to 74 or 80 years) and history of tobacco smoking (at least 30 pack-years of smoking, or have smoked within the past 15 years). This presented an appealing complementary strategy for reducing lung cancer mortality through detection of early-stage lung cancer, which is still potentially curable by surgical resection [ 40 ].
As a result, many public health agencies and medical institutions are now considering implementing LDCT lung cancer screening at the population level, and the United States Preventive Services Task Force has issued the Grade B recommendation for LDCT screening. Since 2015, several major health insurance programmes in the USA, including Medicare, have started to approve LDCT screening for insurance coverage.
Currently, in the USA most of the screening recommendations provided by health agencies are derived from the National Lung Screening Trial eligibility criteria based on age and history of tobacco smoking, and a recent National Comprehensive Cancer Network Category 2 recommendation also included family history and non-tobacco risk factors to improve the screening criteria [ 41 ]. However, studies have shown that applying individual risk probability-based screening criteria could prevent more lung cancer deaths and reduce the number needed to screen to prevent one lung cancer death [ 42 ]. Although substantial efforts have been made to establish lung cancer risk prediction models based on personal health and exposure history [ 43 ], lung cancer researchers are now working towards integrating individual molecular profiles to improve risk prediction.
The development of biomarkers for early detection of lung cancer is an active research area, which encompasses a wide range of biomarker research, including markers and metabolites that could be found in the various biological fluids, particularly circulating blood, urine, or sputum. The main types of circulating biomarkers are protein-based markers, metabolites, autoantibodies from humoral immune response, epigenetic markers, and circulating tumour DNA.
Although most of the biomarkers have failed to be replicated in independent studies, several promising biomarkers have been established across multiple prospective cohort studies. For example, plasma level of pro-surfactant protein B was shown to be an independent predictor of lung cancer risk based on a pan-Canadian screening programme and the Carotene and Retinol Efficacy Trial, after adjusting for demographic factors and lung cancer risk factors [ 44 ]. It has become clear that a panel of multiple biomarkers, rather than any single marker, would be needed to improve risk prediction [ 45 ]. A succinct review of various reported biomarker panels was recently published [ 46 ].
In terms of epigenetic markers, in addition to methylation and histone modification as mentioned above, microRNAs and long non-coding RNAs are also potential epigenomic biomarkers. In particular, several previous studies have shown a promising predictive performance of multi-microRNA panels [ 47 ], although the sample sizes tend to be limited and external validation in independent studies is still required.
In addition to blood-based biomarkers, another type of biomarker for early detection of lung cancer focuses on the gene expression profile of the airway epithelium, based on the theory of field of injury and field cancerization [ 48 , 49 ]. Finally, given the known association between chronic obstructive pulmonary disease and risk of lung cancer, previous studies have evaluated the added predictive performance of lung function [ 50 , 51 ].
Biomarker research for early detection of lung cancer can help to better identify individuals who are at high risk of lung cancer and should be recommended for LDCT screening. To yield an optimal predictive performance for early detection of lung cancer, one can consider multiple layers of data, including epidemiological and clinical information and an individual’s molecular profiles; this aligns with the concept of precision medicine ( Fig. 5.1.6 ) [ 52 ].
Fig. 5.1.6
A concept schema of biomarker integration for precision medicine.
It is anticipated that biomarkers may also help to differentiate malignant nodules from benign ones. The challenge is to establish a panel that would be applicable in the clinical setting and remain cost-effective for the health-care system.
Nodule malignancy
For individuals who undergo LDCT screening, about 15–20% of chest scans detect non-calcified pulmonary nodules. However, the National Lung Screening Trial reported that only 1 in 20 nodules detected by LDCT screening are actually lung cancers [ 40 ]. To address this issue, several clinical probability models were proposed to improve the assessment of nodules, and use of the Lung CT Screening Reporting and Data System (Lung-RADS) classification of the American College of Radiology was shown to substantially decrease the false-positive rate, with a moderate reduction in sensitivity [ 53 ]. However, currently there is still a wide range of clinical protocols for how patients with pulmonary nodules detected on LDCT screening are managed, and the diagnostic evaluation of suspicious abnormalities can range from watchful waiting and monitoring to needle biopsy and pulmonary resection.
In response to the need to differentiate between benign and malignant nodules, radiomics has emerged as a field of study. Radiomics is the analysis of high-dimensional imaging data, focusing on the extraction of quantitative variables from radiographic features for subsequent agnostic data mining [ 54 ]. This field has shown promise to better differentiate nodules with malignant potential. However, there is no standardized process of feature extraction, the analytical methods vary greatly across studies, and proper validation is still required for robust reproducibility. Therefore, it is currently considered premature to implement radiomics as part of the routine diagnostic process.
- Prognosis and targeted treatment
Lung cancer survival remains dismal, with 5-year survival rates of only 10–20% in most parts of the world [ 55 , 56 ]. The stage at diagnosis is a major determinant of lung cancer prognosis; 5-year survival rates range from 50–70% for diagnosis at stage I to 1–5% for diagnosis at stage IV, because surgical resection at an early stage is still the most effective treatment [ 55 ]. However, fewer than 20% of patients are diagnosed at stage I, and most are diagnosed at stage IIIB or IV [ 55 ]; hence, early detection is important.
The clinical outcome varies by histological type. SCLC is the most aggressive type, and combined SCLC may have a worse prognosis, perhaps because the NSCLC component is resistant to cytotoxic therapies. Adenocarcinoma in situ usually has an excellent prognosis if it is completely resected, even if small foci of invasion are present (microinvasive carcinomas).
Despite the growing number of mutations that continue to be identified, only a few somatic mutations can be used for targeted therapy, such as EGFR mutation, ROS1 fusion, and ALK translocation; more recently, immunotherapy agents have been developed that target programmed cell death 1 (PD-1) protein. A range of other targeted and immunotherapy trials are currently in progress, with the hope of improving treatment response based on the principle of precision medicine. A complete review has been provided by the International Association for the Study of Lung Cancer [ 57 ].
In summary, lung cancer comprises very different types and subtypes, which affect the strategies for prevention, early detection, diagnosis, and clinical management.
Fig. 5.1.5
A man undergoing lung cancer screening at the University of Connecticut Health Center, USA.
Some rights reserved. This work is available under the Creative Commons Attribution-NonCommercial-NoDerivs 3.0 IGO licence (CC BY-NC-ND 3.0 IGO; https://creativecommons.org/licenses/by-nc-nd/3.0/igo/ ). Under the terms of this licence, you may copy and redistribute the work for non-commercial purposes, provided the work is appropriately cited, as indicated below. In any use of this work, there should be no suggestion that WHO endorses any specific organization, products or services. The use of the WHO logo is not permitted.
- Cite this Page Hung RJ, Gazdar AF, Didkowska J, et al. Lung cancer: Continues to be the leading cause of cancer death. In: Wild CP, Weiderpass E, Stewart BW, editors. World Cancer Report: Cancer research for cancer prevention. Lyon (FR): International Agency for Research on Cancer; 2020. 5.1.
- PDF version of this title (32M)
In this Page
Other titles in this collection.
- IARC World Cancer Reports
Related information
- PMC PubMed Central citations
- PubMed Links to PubMed
Recent Activity
- Lung cancer - World Cancer Report Lung cancer - World Cancer Report
Your browsing activity is empty.
Activity recording is turned off.
Turn recording back on
Connect with NLM
National Library of Medicine 8600 Rockville Pike Bethesda, MD 20894
Web Policies FOIA HHS Vulnerability Disclosure
Help Accessibility Careers
An official website of the United States government
Official websites use .gov A .gov website belongs to an official government organization in the United States.
Secure .gov websites use HTTPS A lock ( Lock Locked padlock icon ) or https:// means you've safely connected to the .gov website. Share sensitive information only on official, secure websites.
- Publications
- Account settings
- Advanced Search
- Journal List
An annual review of the remarkable advances in lung cancer clinical research in 2019
Hengrui liang, zisheng chen, wenhua liang, jianxing he.
- Author information
- Article notes
- Copyright and License information
Contributions: (I) Conception and design: B Cheng, J He, W Liang; (II) Administrative support: J He, W Liang; (III) Provision of study materials or patients: S Xiong, C Li; (IV) Collection and assembly of data: All authors; (V) Data analysis and interpretation: All authors; (VI) Manuscript writing: All authors; (VII) Final approval of manuscript: All authors.
These authors contributed equally to this work.
Correspondence to: Jianxing He, MD; Wenhua Liang, MD. Department of Thoracic Surgery and Oncology, the First Affiliated Hospital of Guangzhou Medical University, State Key Laboratory of Respiratory Disease, National Clinical Research Center for Respiratory Disease, 151, Yanjiang Road, Guangzhou 510120, China. Email: [email protected] ; [email protected] .
Corresponding author.
Received 2020 Jan 21; Accepted 2020 Feb 27; Issue date 2020 Mar.
Open Access Statement: This is an Open Access article distributed in accordance with the Creative Commons Attribution-NonCommercial-NoDerivs 4.0 International License (CC BY-NC-ND 4.0), which permits the non-commercial replication and distribution of the article with the strict proviso that no changes or edits are made and the original work is properly cited (including links to both the formal publication through the relevant DOI and the license). See: https://creativecommons.org/licenses/by-nc-nd/4.0 .
In recent years, lung cancer has become the most common cancer and the leading cause of deaths attributed to cancer. In China, lung cancer is the third leading cause of death after strokes and ischemic heart disease. This review aimed to provide an up-to-date summary of studies in regard to lung cancer in 2019 and to present the remarkable progress seen in lung cancer clinical research. A systematic search of PubMed and Web of Science for research published in 2019 was conducted using the search terms “lung cancer”, “early stage”, “advanced”, “diagnosis”, “treatment”, or any combination of these terms. We selected 56 studies that we considered to be significant and have presented their major findings. In summary, survival among lung cancer patients was significantly improved compared with historical controls, turning lung cancer from an incurable disease into a chronic disease. In addition, treating lung cancer has become increasingly comprehensive, diversified, and individualized. Exploring the accurate biomarkers in immunotherapy and the mechanism of drug resistance in targeted therapy constitutes the greatest challenge at this stage.
Keywords: Lung cancer, clinical trial, chemotherapy, immunotherapy, targeted therapy
Introduction
The diagnosis and treatment of lung cancer became a hot spot of research due to the disease’s increased morbidity and mortality in recent years. Therefore, more and more novel diagnosis methods for early-stage lung cancer and plenty of clinical trials have been conducted. Over past 12 years, China has seen a significant increase in the 5-year survival rates of various cancers, among which the 5-year survival rate of lung cancer has increased by an average of 1.3% every year and has achieved 19.7% so far ( 1 ). In terms of different patient characteristics, a series of treatment modes have been adopted, such as combination therapy, sensitization of drugs, and targeted therapy on various driver mutations. The aim of this review is to provide an up-to-date summary of lung cancer studies in 2019 and to present the remarkable progress made in lung cancer clinical research.
A systemic search of PubMed and Web of Science was conducted to find studies or conference abstracts published in English in the period between January 1, 2019 and December 31, 2019. The search terms included “lung cancer”, “early stage”, “advanced”, “diagnosis”, “treatment”, or any combination of these terms. The articles published in The New England Journal of Medicine ( NEJM ), The Lancet , JAMA , BMJ , and some other journals in the oncology field, as well as the abstracts reported in the conferences of the American Society of Clinical Oncology (ASCO), the World Conference on Lung Cancer (WCLC), and the European Society for Medical Oncology (ESMO) were included. Additionally, we reviewed in detail the survival of patients, the efficacy of drugs and drug-related adverse events in each enrolled study.
Early-stage lung cancer
The trends and risk of morbidity in lung cancer, lung cancer has become the third leading cause of death in china.
In June 2019, The Lancet published China’s burden of disease data online, covering a period of nearly 30 years ( 2 ). The data, which was reported by Professor Xiaofeng Liang from the Chinese Center for Disease Control and Prevention, revealed lung cancer to have become the third leading cause of death in China after strokes and ischemic heart disease (IHD). Between 1990 to 2017, increases of 28.2% and 12.6%, respectively, were seen in age-standardized mortality and years of life lost (YLLs) in lung cancer, leading to a change in ranking from 13th in 1990 to third in 2017.
This trend and current situation suggest that the prevention and control of lung cancer needs to be carried out with reference to the management mode of common diseases such as diabetes and hypertension, including the popular science and screening activities for lung cancer which are increasingly valued in China. How to balance the goals of early diagnosis and concerns regarding the health economy will be an important challenge.
Dietary fiber and yogurt consumption were associated with reduced risk of lung cancer
In October 2019, JAMA Oncology published a meta-analysis of data from ten prospective cohort studies performed by Xiao-Ou Shu’s team at Vanderbilt University ( 3 ), which involved a total of 1.44 million people and 8.6 years of follow-up. The results showed that dietary fiber and yogurt consumption were associated with a lower risk of lung cancer. The two groups with the highest intake of fiber and yogurt had a lower risk (17% and 19% lower, respectively) of lung cancer than the group with the lowest intake. For individuals who reported high yogurt consumption along with the highest fiber intake, the risk of lung cancer was reduced by 33% compared with those who did not consume yogurt and had the lowest fiber intake. In addition, young people may benefit more from the consumption of dietary fiber and yogurt.
Early screening and diagnosis of lung cancer
Genetic risk of lung cancer in chinese and its measurement.
In July 2019, a large multicenter study of the Chinese population conducted by Professor Hongbing Shen and his team from Nanjing Medical University was published in The Lancet Respiratory Medicine ( 4 ). This study systematically identified 19 susceptibility loci to be significantly associated with risk of non-small cell lung cancer (NSCLC) in the Chinese population through a cohort involving nearly 55,000 people and built a polygenic risk score (PRS) for Chinese people based on it. Meanwhile, this research, which was verified by an independent prospective cohort of about 100,000 individuals from the China Kadoorie Biobank (CKB), indicated that the incidence of lung cancer in the highest risk group was nearly double that of the lowest risk group. Moreover, PRS was an independent, effective predictive indicator of risk beyond age and smoking, which could provide an important reference for the screening and accurate prevention of lung cancer for high-risk individuals.
Liquid biopsy (LBx) is expected to be used as an early diagnosis tool for lung cancer
The preliminary results of Circulating Cell-free Genome Atlas (CCGA) Study were released at the conference of the ASCO and the ESMO ( 5 , 6 ). By combining three prototype assays [whole-genome bisulfite (WGBS), whole-genome sequencing (WGS), and targeted (507 gene) sequencing], signals from 12 cancers including lung cancer can be detected at an early stage. The overall detection rate (sensitivity) of this method for all stages and types of cancers was 55%, and the tissue of origin (TOO) localization could also be identified with high accuracy. The detection results of early-stage cancer (stage I–III) were favorable, with a sensitivity of 32%, 76%, and 85% for stages I, II, and III, respectively. This is consistent with the preliminary results relating to lung cancer of the CCGA study announced by ASCO annual meeting last year, which showed a diagnostic sensitivity of 38–51% for stage I lung cancer.
In addition, Professor Jianxing He and his team from Guangzhou Institute of Respiratory Health developed a non-invasive diagnostic tool for early-stage lung cancer using high-throughput targeted DNA methylation sequencing of circulating tumor DNA (ctDNA), which had a sensitivity of more than 70% for stage I lung cancer ( 7 ). Moreover, this assay was being validated through two national multicenter studies ( NCT03181490 and NCT03651986 ) in current stage, including the “Thunder Project” which involves more than 10,000 cases.
The treatment of stage I–III lung cancer
Immunotherapy joins the “neoadjuvant family”.
Three important neoadjuvant immunotherapy studies were presented at the ASCO annual meeting in June 2019: LCMC3 ( 8 ), NEOSTAR ( 9 ), and NADIM ( 10 ).
The LCMC3 study assessed the efficacy and safety of atezolizumab used as neoadjuvant therapy for patients with stages IB to selected IIIB resectable NSCLC. The interim analysis showed that 7% of patients achieved partial response (PR), 18% achieved major pathological response (MPR), and 5% achieved pathological complete response (pCR). Pathological response was also observed in some patients with positive epidermal growth factor receptor (EGFR)/ALK mutations. Neither PD-L1 expression nor tumor mutational burden (TMB) level was correlated with pathological response. This regimen was tolerated.
NEOSTAR confirmed that nivolumab (NV) plus ipilimumab showed better efficacy as a neoadjuvant therapy for resectable NSCLC patients than NV monotherapy. Patients received neoadjuvant therapy for three cycles before surgery and underwent surgery within three to six weeks after treatment. The results revealed that the MPR rate was 17% in the monotherapy group vs. 33% in the combination group, and the pCR rate was 9% vs. 21% in the monotherapy and combination groups, respectively. For imaging evaluation, the objective response rate (ORR) was 22% and 19% in the monotherapy group and the combination group, respectively, and the ORR was positively correlated with MPR (P<0.001). Subgroup analysis presented that the higher expression of PD-L1, the benefit of imaging, and the pathological response of patients would be greater. Additionally, this combination could increase the lymphocyte infiltration of the tumor and enhance cell diversity and reactivity-related function. No unacceptable toxicity or increased perioperative morbidity or mortality was found.
NADIM was the first multicenter study on conventional chemotherapy (CT) combined with immuno-oncology agent (IO) in a neoadjuvant setting for stage IIIA patients. Participants received three cycles of NV + paclitaxel + carboplatin as preoperative treatment, followed by adjuvant NV treatment for 1 year after the surgery. The chemotherapy combined with immunology was well tolerated, and 41 patients ultimately underwent surgery, with R0 resection in all cases. Downstaging was seen in 90% of cases after neoadjuvant CT+IO, the MPR rate was 83%, and the pCR rate was 71%. According to RECIST (Response Evaluation Criteria in Solid Tumors), the imaging PR rate was 71%, and the CR rate was 7%. No patients withdrew from the study before surgery due to progressive disease (PD) or safety problems.
Neoadjuvant immunotherapy, especially combined with chemotherapy, achieved a surprising pathological response rate, which will largely change the treatment model of early- and middle-stage lung cancer. However, a series of questions relating to, for example, response evaluation criteria, surgery time, and postoperative treatment choices also surfaced and need to be answered.
Treatment strategy options for early-stage lung cancer
Stereotactic body radiotherapy (SBRT) was widely used to treat inoperable stage-I NSCLC. The Lancet Oncol ogy published the phase 3 randomised controlled trial (RCT) “TROG 09.02 CHISEL”, the results of which indicated that SBRT compared to standard radiotherapy could reduce the local recurrence rate (14% vs. 31%, respectively), which supports the use of SBRT in treating early-stage NSCLC ( 11 ).
In relation to adjuvant treatment options for stage-I NSCLC patients, as defined by the eighth edition TNM staging system, Jianxing He and Wenhua Liang’s research group from Guangzhou Institute of Respiratory Health established a risk prediction model based on data from a large sample size, which was published on Annals of Surgical Oncology ( 12 ). This research found that 21.7% of stage IB patients (7.5% of all stage I) were categorized into the high-risk of recurrence group, and verified that these patients can benefit from chemotherapy. Furthermore, in regard to the optimal treatment for stage IIIA-N2 NSCLC, the same team published a network meta-analysis based on 18 RCTs in The Annals of Thoracic Surgery ( 13 ), finding that neoadjuvant chemotherapy followed by surgery and adjuvant chemotherapy or radiotherapy holds the greatest potential to become the optimal treatment with the best efficacy and safety. This paper was included in the recommended literature on the F1000Prime platform.
The treatment of locally advanced NSCLC
Immunology consolidation therapy after concurrent chemoradiotherapy.
The PACIFIC study is a milestone to rewrite the therapeutic method of locally advanced NSCLC, the results of which were published in NEJM for twice in 2018 and confirmed that treatment with PD-L1 antibody durvalumab after concurrent chemoradiotherapy for unresectable stage III NSCLC can significantly prolong progression free survival (PFS) and overall survival (OS) compared with the placebo group. The PACIFIC study presented the 3-year updated OS data at the 2019 ASCO annual meeting ( 14 ). The median duration of follow-up was 33.3 months, and the median OS (mOS) of the treatment group was significantly longer than that of the placebo group [not reached (NR) vs. 29.1 months]. The 3-year OS rate in these two groups was 57% vs. 43.5%, respectively. Compared with the placebo group, patients in the treatment group received subsequent therapies for a longer period of time, with the first subsequent treatment continuing for twice as long (21.2 vs. 10.4 months), and the second subsequent treatment lasting for 30.2 vs. 17.8 months.
Furthermore, the PACIFIC study updated the information of patient-reported outcomes (PROs) in The Lancet Oncology ( 15 ), proving that with the consolidation therapy of durvalumab, OS could be greatly improved in patients with unresectable stage III NSCLC and patients’ quality of life was not compromised.
Immunotherapy plus concurrent chemoradiotherapy
The current concerned point is whether immunotherapy can be used concurrently with chemoradiotherapy. The treatment of atezolizumab combined with concurrent chemoradiotherapy had favorable safety for locally advanced NSCLC patients, as reported at ASCO annual meeting 2019, suggesting that concurrent chemoradiotherapy plus immunotherapy does not increase toxicity compared with chemoradiotherapy followed by immunotherapy. At present, the PACIFIC2 study is also under way, and the impact on efficacy due to bringing immunotherapy forward to the same stage as chemoradiotherapy is anticipated.
Whether patients with locally advanced NSCLC need prophylactic cranial irradiation (PCI)
An update of a prospective, randomized, multicenter phase 3 study of locally advanced NSCLC patients (RTOG 0214 trial) was published in JAMA Oncology ( 16 ). In patients without disease progression after local therapy, despite PCI therapy significantly decreasing the incidence of brain metastasis (BM) and remarkably improving disease-free survival (DFS) compared with the observation group, there was no significant difference in terms of OS (the 5- and 10-year rates were 24.7% and 17.6% vs. 26.0% and 13.3%, respectively).
Advanced lung cancer
First-line immunotherapy for advanced lung cancer, io monotherapy.
(I) KEYNOTE-024
Keynote-024 updated 3-year OS data at the WCLC conference 2019 and showed that the superior efficacy of PD-1 antibody monotherapy compared to CT. There was a significant difference in the 3-year OS rate between the treatment group and the control group (43.7% vs. 24.9%, respectively) ( 17 ).
(II) KEYNOTE-042
This important phase 3 study was formally published in The Lancet , and its results confirmed that for locally advanced or metastatic NSCLC patients without sensitizing EGFR/ALK alterations and with PD-L1 positive [tumor proportion score (TPS) ≥1%], first-line pembrolizumab treatment could obviously extend the OS compared to chemotherapy (16.7 vs. 12.1 months; HR, 0.81; 95% CI, 0.71–0.93; P=0.0018) and was safer ( 18 ).
(III) IMpower 110
The interim OS analysis announced on the ESMO congress demonstrated that, compared to standard chemotherapy, atezolizumab significantly improved the mOS (20.2 vs. 13.1 months, respectively; HR, 0.59; 95% CI, 0.40–0.89; P=0.0106) of untreated stage IV patients with PD-L1 high expression (TC3/IC3-WT). Median PFS was 8.1 vs. 5.0 months (HR, 0.63; 95% CI, 0.45–0.88; P=0.007) for atezolizumab and standard chemotherapy, respectively. ORR and duration of response (DoR) had notable benefits with atezolizumab ( 19 ).
Throughout the current research data for IO monotherapy, the level of patients’ PD-L1 expression has been the key to selecting IO monotherapy. Existing evidence demonstrates that IO monotherapy should be preferentially considered for patients with PD-L1 high expression (≥50%), whereas a combination of immuno- and chemo-therapy should be considered for patients with PD-L1 expression <50%.
The long-term survival outcome of PD-1 monoclonal antibody treatment
The Lancet Oncology published the long-term survival outcome of NV as a second-line treatment for advanced NSCLC patients ( 20 ). A pooled analysis from the Checkmate 017 and 057 studies showed the 4-year OS rate with NV was 14% for all patients, and 19% for those with positive PD-L1 expression (at least 1%), which was far higher than the 5% of docetaxel chemotherapy. This confirmed that PD-1 antibody immunotherapy can achieve a clinical cure for some patients.
The ASCO annual meeting presented the long-term outcomes of the KEYNOTE-001 trial ( 21 ). With pembrolizumab monotherapy treatment, the estimated 5-year OS rates were 23.2% for treatment-naive advanced NSCLC patients and 15.5% for patients who had been previously treated.
IO plus chemotherapy
(I) KEYNOTE-189 ( 22 )
At the 2019 ASCO annual meeting, the KEYNOTE-189 study updated the OS results after follow-up time was extended and, for the first time reported the results of PFS2 (progression after the next line of therapy). For nonsquamous NSCLC, persistent OS and PFS benefit was still observed in the combination arm of pembrolizumab and chemotherapy. For the combination and control arms, mOS was 22.0 vs. 10.7 months, respectively (HR, 0.56; 95% CI, 0.45–0.70; P<0.00001), and median PFS (mPFS) was 9.0 vs. 4.9 months, respectively (HR, 0.48; 95% CI, 0.40–0.58; P<0.00001). In the intention-to-treat (ITT) population, PFS2 in the combination arm was significantly higher than in chemotherapy alone arm. In these two groups, the mPFS2 was 17.0 vs. 9.0 months, respectively (HR, 0.49; 95% CI, 0.40–0.59; P<0.00001). The benefit of all endpoints was independent of the PD-L1 TPS level of patients.
(II) KEYNOTE-407 ( 23 )
The study’s updated outcome at the ESMO congress 2019 indicated that for squamous NSCLC, first-line treatment with pembrolizumab plus chemotherapy significantly prolonged PFS2. In the chemotherapy alone group, 49.1% of the patients received the subsequent treatment of PD-1/PD-L1 antibody, and the combination of pembrolizumab and chemotherapy still brought continuous OS benefit to patients. In the ITT population, mOS attained 17.1 months (range, 14.4 to 19.9 months), compared to 11.6 months (range, 10.1 to 13.7 months) in the chemotherapy alone group, and the mortality risk was reduced by 29% with combination treatment (HR, 0.71; 5% CI, 0.58–0.88). In comparison with chemotherapy alone, combination treatment also significantly improved the PFS of patients. Regardless of the level of PD-L1 expression of patients, pembrolizumab plus chemotherapy continued to demonstrate improved end points including OS, PFS, ORR, and PFS2 as the first-line treatment, compared with chemotherapy alone for advanced squamous NSCLC, with a manageable safety profile.
(III) IMpower130
The results published in The Lancet Oncology showed a significant and clinically meaningful improvement in OS and PFS with atezolizumab plus chemotherapy versus chemotherapy alone as first-line treatment for patients with stage IV nonsquamous NSCLC and no ALK or EGFR mutations ( 24 ), with acceptable safety and tolerability. This was the first PD-L1 inhibitor to be successfully used as first-line treatment for advanced NSCLC.
These study results further consolidated the status of pembrolizumab as well as atezolizumab combined with chemotherapy as the first-line standard treatments for advanced NSCLC patients with negative driver genes, and suggested once again that the earlier the use of immunotherapy, the more effective it was, and its use as a first-line treatment combined with chemotherapy was superior to its use as a second-line salvage therapy.
The sensitization of first-line immunotherapy
Io plus radiotherapy.
In 2019, JAMA Oncology published two consecutive studies related to the treatment of oligometastatic NSCLC with immunotherapy plus radiotherapy.
A single-arm phase 2 study ( 25 ) demonstrated that after the completion of locally ablative therapy (LAT) such as surgery or stereotactic radiotherapy for oligometastatic NSCLC (≤4 metastatic sites), the mPFS of patients subsequently treated with pembrolizumab was 19.1 months, significantly longer than the historical median of 6.6 months (P=0.005), with no new safety signals and no reduction in quality of life.
Another important randomized controlled trial, the PembroRT study ( 26 ), was designed to evaluate the tolerability and efficacy of SBRT prior to pembrolizumab treatment on a single metastatic site. The ORR of the control arm and the experimental arm was 18% (n=40) and 36% (n=36), respectively, and the mPFS was 1.9 and 6.6 months, respectively (HR, 0.71; 95% CI, 0.42–1.18; P=0.19). Due to reasons including experimental design, the PembroRT study did not meet its prespecified end point, but it clearly showed the powerful sensitization effect of radiotherapy, which is worthy of further examination.
At present, all of the first-line studies are aiming to compare whether immunotherapy should be added on the basis of chemotherapy. Therefore, it is still controversial whether to add chemotherapy on the basis of immunotherapy for a population which is suitable for immunotherapy, especially for those with PD-L1 expression >50%. Through an indirect comparison of 11 first-line RCTs, Jianxing He and Wenhua Liang’s research group from Guangzhou Institute of Respiratory Health found that adding chemotherapy on the basis of immunotherapy could further significantly improve PFS/OS in the overall population and in the high PD-L1 expression population ( 27 ). This provides evidence for treatment selection, whereas the optimal sensitization model still needs to be optimized and confirmed.
PD-1 monoclonal antibody plus CTLA-4 monoclonal antibody
In September 2019, NEJM once again published the results from the CheckMate 227 study ( 28 ), confirming that for patients with NSCLC, nivolumab plus ipilimumab resulted in a longer OS duration than platinum-containing chemotherapy, providing a “chemo-free” first-line treatment regimen for these patients. The researchers enrolled more than 1,700 untreated advanced NSCLC patients who were EGFR and ALK mutation negative.
First, the patients were stratified according to their expression of PD-L1, and divided into the PD-L1 positive group (PD-L1 expression ≥1%) and the PD-L1 negative group (PD-L1 expression <1%). Each group was further divided into three subgroups: the PD-L1 positive group was divided into the dual immunotherapy (nivolumab plus ipilimumab), single immunotherapy (nivolumab alone group), and chemotherapy groups (standard chemotherapy regimen). The grouping of the PD-L1 negative group was similar to that of the PD-L1 positive group, except the single immunotherapy group was combined with chemotherapy in PD-L1 negative group, while the other two subgroups were the same as the in the PD-L1 positive group.
There were two primary end points: (I) patients with a high TMB (TMB ≥10 mutations per megabase): PFS with dual immunotherapy versus with chemotherapy; and (II) PD-L1 positive patients (PD-L1 expression level of 1% or more): OS with dual immunotherapy versus with chemotherapy. NEJM reported the PFS in patients with a high TMB in 2018, with the dual immunotherapy group being obviously superior to the chemotherapy group. A second primary end point was simultaneously reported by ESMO congress and NEJM in 2019, showing that the OS of PD-L1 positive patients was significantly longer with dual immunotherapy (NV plus ipilimumab) than with chemotherapy. Furthermore, regardless of the level of PD-L1 expression and TMB, the mOS of the dual immunotherapy group was longer than that of the chemotherapy group, especially in the PD-L1 negative group (17.2 vs. 12.2 months; HR, 0.62). Additionally, the median DoR (mDoR) was also significantly longer in the dual immunotherapy group than in the chemotherapy group (23.2 vs. 6.2 months).
IO plus antiangiogenic agents
Following the Impower150 study’s report in 2018 of the efficacy of chemotherapy + atezolizumab + bevacizumab, a multicenter, phase Ib study that aimed to assess the preliminary antitumor activity of the inhibitor of vascular endothelial growth factor receptor (VEGFR) ramucirumab plus pembrolizumab in multiple types of cancer (including lung cancer) patients was published in The Lancet Oncology in 2019 ( 29 ). Patients with NSCLC attained ORR of 30% and a disease control rate (DCR) of 86%, which suggests this is a regimen worthy of further exploration.
The first-line treatment for EGFR-mutated advanced NSCLC
The third-generation tyrosine kinase inhibitor of the egfr (egfr-tki) consolidated its status in first-line treatment.
NEJM once again published the FLAURA study, the foremost research into targeted therapy of lung cancer ( 30 ). This trial was designed to compare the efficacy of third-generation EGFR-TKI osimertinib as the first-line treatment with first-generation EGFR-TKI. The analysis of the FLAURA study, which was reported for the first time in 2017, showed that the PFS of the osimertinib group was significantly longer than that of the control group, and the mPFS was 18.9 and 10.2 months (HR, 0.46; P<0.001), respectively.
As announced in NEJM and at the ESMO congress 2019, the mOS of the osimertinib group and the comparator group was 38.6 and 31.8 months, respectively (HR, 0.8; P=0.046), achieving a reduction of 20% in disease mortality risk in the osimertinib group (HR, 0.80; 95% CI, 0.64–1.00; P=0.046). The 12-, 24-, and 36-month OS rates in the osimertinib group were all better than those in the first-generation EGFR-TKI group, and osimertinib also extended the time to first and second subsequent therapy. An OS benefit with osimertinib was observed across most of the predefined subgroups, apart from the Asian subgroup (HR, 1.00; 95% CI, 0.75–1.32).
In addition, Journal of Clinical Oncology published the results of the BLOOM study, which investigated osimertinib treatment in patients with EGFR-mutated NSCLC and leptomeningeal metastases (LMs) ( 31 ). The LM ORR and DoR were 62% (95% CI, 45–78%) and 15.2 months (95% CI, 7.5–17.5 months), respectively. The FLAURA study established the treatment mode of putting third-generation TKI as a priority option in first-line treatment, but its results in Asian populations remain controversial.
First-generation EGFR-TKI plus chemotherapy
A phase 3 randomized controlled trial NEJ005 was released at the 2019 ASCO annual meeting, with the purpose of comparing gefitinib plus chemotherapy with gefitinib alone as the first-line treatment ( 32 ). In this trial, estimated mPFS was significantly longer with gefitinib plus chemotherapy than gefitinib alone (16 vs. 8 months; HR, 0.5; 95% CI, 0.39–0.65; P<0.001). Moreover, patients treated with gefitinib plus chemotherapy had longer mOS than those treated with gefitinib alone (NR vs. 18 months; HR, 0.45; 95% CI, 0.31–0.66; P<0.001). In terms of adverse events, the incidence of adverse events ≥ grade 3 was 51% for the gefitinib plus chemotherapy arm and 25% in the gefitinib group, with significant difference between the arms (P<0.001). The NEJ009 study presented its results at the ASCO annual meeting 2018, and these two studies were published successively in the Journal of Clinical Oncology .
At the WCLC conference 2019, Professor Baohui Han and his team from Shanghai Chest Hospital affiliated to Shanghai Jiao Tong University verbally reported the efficacy and safety of first-generation EGFR-TKI gefitinib combined with chemotherapy as the first-line treatment for advanced EGFR-mutated NSCLC ( 33 ). Updated data of the clinical trial showed that the mOS in the combinational group was obviously extended to 37.9 months (95% CI:17.3–58.6). Multiple studies have confirmed that first-generation EGFR-TKI combined with chemotherapy shows significant survival benefit. However, the comparison of first-generation EGFR-TKI plus chemotherapy with third-generation EGFR-TKI, and the mechanism of first-line treatment resistance are yet to be fully explored.
First-generation EGFR-TKI plus antiangiogenic agents
The ARTEMIS study is a Chinese multicenter, randomized, controlled phase 3 clinical trial aiming to investigate the efficacy and safety of erlotinib with or without bevacizumab as the first-line treatment for EGFR-mutated NSCLC patients. The study was verbally presented by Professor Qing Zhou from Guangdong Provincial People’s Hospital at ESMO congress 2019 ( 34 ). The mPFS evaluated by the Independent Review Committee (IRC) was 18 months in the combinational group and 11.3 months in the erlotinib group (HR, 0.55; P<0.001), and PFS benefited significantly in the combinational group. In addition, the analysis of drug resistance showed a similar proportion of T790M mutation between these two groups (P=0.732).
The RELAY study was a worldwide, randomized, double-blind, and placebo-controlled study which evaluated ramucirumab plus erlotinib versus placebo plus erlotinib as first-line treatment for advanced NSCLC patients ( 35 ). There was a significantly statistical and clinical improvement of PFS in the treatment group compared to the placebo group (mPFS, 19.4 vs. 12.4 months; HR, 0.59; 95% CI, 0.46–0.79; P<0.0001), and the frequencies of EGFR-T790M mutation were similar between the groups. The related results were published in the The Lancet Oncology .
The combination of first-generation EGFR-TKI with antiangiogenic agents can delay the occurrence of drug resistance, but the long-term survival benefit has not yet been confirmed, and, at present, there is not enough evidence which demonstrates that this treatment model can change the biological activity of tumor.
Comprehensive comparison of first-line treatment regimens
Regarding the numerous options currently available for first-line treatment for EGFR mutations, Jianxing He and Wenhua Liang’s research group from Guangzhou Medical University conducted a network meta-analysis involving a total of 4,628 patients from 18 RCTs ( 36 ). This meta-analysis found that gefitinib plus chemotherapy and third-generation EGFR-TKI osimertinib ranked highest and equal in efficacy among all regimens. Furthermore, the meta-analysis indicated that these two regimens were the optimal therapy for the two major mutation types of EGFR L858R and exon 19 deletion, respectively.
This study was the first study related to the treatment of lung cancer to be published in the BMJ in China, providing evidence for the optimization and individualized options of first-line regimens for patients with EGFR mutations.
Third-generation EGFR-TKI showed efficacy on uncommon mutations
A multicenter, open-label, single-arm, phase II trial published in the Journal of Clinical Oncology demonstrated that patients with NSCLC harboring EGFR mutations (other than the exon 19 deletion, L858R and T790M mutations, and exon 20 insertion) achieved an ORR of 50% (95% CI, 33–67%, 18/36) after receiving osimertinib treatment ( 37 ). The mPFS was 8.2 months (95% CI, 5.9–10.5 months) and the mDoR was 11.2 months (95% CI, 7.7–14.7 months), with manageable toxicity.
Anaplastic lymphoma kinase-positive (ALK-positive) advanced NSCLC
The ALESIA study, led by Professor Caicun Zhou from Shanghai Pulmonary Hospital Affiliated to Tongji University School of Medicine, was published in The Lancet Respiratory Medicine ( 38 ). This trial aimed to compare the first-line treatment of alectinib and crizotinib head-to-head in Asian patients with advanced ALK-positive NSCLC. The mPFS was NR in the alectinib group compared with 11.1 months in the crizotinib group. (HR, 0.22; 95% CI, 0.13–0.38; P<0.0001). OS data are currently immature for both groups (HR, 0.28; 95% CI, 0.12–0.68; P=0.0027), but the results showed that the prognosis of both groups of patients with or without central nervous system (CNS) metastatic lesions was superior in the alectinib group, and alectinib can significantly delay the progression of CNS (HR, 0.14; 95% CI, 0.06–0.30). Additionally, the incidence of grade 3–5 adverse events in the alectinib group was lower than that in the crizotinib group.
A phase 2 clinical trial led by Professor Li Zhang/Yunpeng Yang from Sun Yat-sen University Cancer Center which assessed the efficacy and safety of ensartinib in ALK-positive NSCLC patients who underwent crizotinib treatment failure was published in The Lancet Respiratory Medicine ( 39 ). A total of 156 patients, 62% of whom had BMs, were included in the final analysis. Data showed that 52% of patients attained a response after treatment, the DCR reached 93%, and the mPFS was 9.6 months. Among the patients with BMs, the intracranial ORR was 70%, and the DCR of intracranial lesions reached 98%. Moreover, ensartinib had significant efficacy with varieties of ALK-resistant mutations, including those of other second-generation ALK inhibitors.
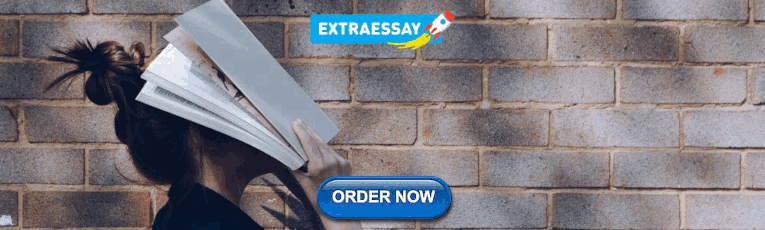
ROS1 fusion-positive advanced NSCLC
Entrectinib.
An integrated analysis of three phase 1–2 clinical trials published in The Lancet Oncology showed that entrectinib was effective and well tolerated for advanced ROS1-fusion positive NSCLC ( 40 ). The outcomes from trials ALKA-372-001, STARTRK-1, and STARTRK-2 indicated that the ORR of 53 ROS1-fusion positive NSCLC patients treated with entrectinib was 77%, the mPFS was 19 months, and the mDoR was 24.6 months.
The Lancet Oncology published the results of a phase 1–2 clinical trial of lorlatinib in advanced ROS1-positive NSCLC which included a total of 69 ROS1-positive NSCLC patients ( 41 ). For 21 TKI-naive patients who had not received ROS1 inhibitors treatment before, the ORR and DCR were 62% and 90%, respectively, and the mPFS and mDoR were 21 and 25.3 months, respectively. Among the 11 patients with CNS metastases, the overall ORR was 45%, the intracranial response rate was 64%, and the median intracranial DoR was not reached. For the 40 patients previously treated with crizotinib as their only TKI, the ORR and DCR were 35%, and 75%, respectively, and the mPFS and mDoR were 8.5 and 13.8 months, respectively. Among the 24 patients with CNS metastases, the ORR rate was 25%, the intracranial response rate was 50%, and the median intracranial DoR was not reached.
Advanced NSCLC with KRAS mutation
A study evaluating the efficacy of AMG 510 in patients with advanced KRAS G12C mutant solid tumors was reported at ASCO annual meeting 2019 ( 42 ). Tumor response was assessable in 29 patients, including 10 NSCLC and 19 colorectal cancer (CRC) patients. All of these patients had previously received ≥2-line treatment regimens. The results indicated that the ORR and DCR of the patients overall were 17.24% and 79.31%, respectively, and the response lasted for a long duration. In particular, in the ten NSCLC patients, the ORR was 50% and the DCR reached 100%.
Advanced NSCLC with other mutations
A study evaluating the efficacy and safety of a novel MET (MET exon 14 skipping mutations) inhibitor tepotinib in advanced NSCLC was presented at the 2019 ASCO annual meeting ( 43 ). This single-arm, phase 2 clinical trial was planned to enroll more than 120 patients, who would receive tepotinib 500 mg once a day. At the time the data was released, 85 patients were enrolled, including 55 identified by LBx and 52 by tumor biopsy (TBx). A total of 35 and 41 patients in these two groups were evaluable for efficacy (≥2 post-baseline assessments or discontinuation for any reason), respectively. The ORR of the two groups assessed by IRC was 51.4% and 41.5%, respectively, and the mDoR was 9.8 and 12.4 months, respectively.
A phase 1 clinical trial was reported at the 2019 ASCO annual meeting ( 44 ). It included patients with advanced EGFR-mutated NSCLC who had previously experienced disease progression after receiving the treatment of EGFR-TKI (including first-/second-generation EGFR-TKI and osimertinib) and were subsequently treated with U3-1402. The outcome showed that out of 13 evaluable patients, all but 1 had a decrease in sum of longest diameter of lesions (median best change −29%, range, +10% to −67%), and the DCR reached 100%. In addition, U3-1402 was also effective on different pathways which were activated secondary to EGFR-TKI resistance, including EGFR C797S, HER2, and CDK4.
An integrated analysis of phase 1–2 clinical trials was published in The Lancet Oncology and showed that entrectinib was effective and well tolerated in advanced NTRK fusion-positive NSCLC patients ( 45 ). Among 54 NTRK fusion-positive NSCLC patients, 31 (57%; 95% CI, 43.2–70.8%) patients had an objective response, with an mPFS of 11.2 months and mDoR of 10.4 months.
The 2019 ASCO annual meeting reported the efficacy and safety of TAK-788 (an inhibitor of EGFR/HER2) in advanced NSCLC patients with EGFR exon 20 insertions ( 46 ). In a phase 1/2 multicenter clinical study, patients who met the inclusion criteria were first subjected to dose escalation and then an expansion cohort. A total of 101 patients were enrolled in the study, 76% of whom had previously received at least two therapies, and 53% of whom had BMs. The treatment dose was 5–180 mg once a day, and the recommended phase 2 dose (RP2D) was 160 mg once a day. Twenty-eight patients received this dose (during dose escalation or in the expansion cohort). The ORR and DCR of 26 patients in the extended cohort were 54% (95% CI, 33–73%) and 89% (95% CI, 70–98%), respectively.
BLU-667 is a highly potent and selective RET inhibitor, and the data of its efficacy and safety was released at the 2019 ASCO annual meeting ( 47 ). A total of 79 patients (21 of dose escalation and 58 of dose expansion) were enrolled and treated with BLU-667, and 39% of these patients had baseline BMs. Among the 57 patients who received at least one follow-up disease assessment, the ORR was 56% (95% CI, 42–69%), the DCR was 91%, and 6 patients had a DoR of more than 6 months. Among 30 patients treated at the RP2D, the ORR was 60%, and responses occurred regardless of prior treatment or RET fusion genotypes. Only 3% of patients discontinued because of the adverse event of drug.
Small-cell lung cancer (SCLC)
The CASPIAN study was a randomized, open-label, worldwide, multicenter phase 3 clinical trial that aimed to explore the efficacy of anti-PD-L1 antibody durvalumab, with or without anti-CTLA-4 antibody tremelimumab, in combination with etoposide plus either cisplatin or carboplatin (platinum-etoposide) in treatment-naive patients with extensive-stage SCLC (ES-SCLC). The results, which were published in The Lancet ( 48 ), demonstrated that the mOS was significantly longer in the durvalumab plus platinum-etoposide group compared to the platinum-etoposide alone group (13.0 vs. 10.3 months; HR, 0.73; 95% CI, 0.59–0.91; P=0.0047).
To date, there are two PD-L1 monoclonal antibodies (atezolizumab and durvalumab) which have obtained positive results in first-line combination treatment of SCLC, establishing the special status of PD-L1 monoclonal antibodies in the treatment of SCLC.
Related research on PD-1 antibodies independently developed in China for lung cancer
The chinese-developed pd-1 antibody and related research have entered onto the international stage, camrelizumab.
SHR-1210-303 was an open, randomized, multicenter phase 3 clinical trial presented at the WCLC conference 2019 ( 49 ), which included patients with advanced non-squamous NSCLC with negative EGFR or ALK and was designed to explore the efficacy of adding camrelizumab (200 mg) on the basis of chemotherapy. The mPFS was 11.3 months (95% CI, 9.5–NR) in the camrelizumab plus chemotherapy arm and 8.3 months (95% CI, 6.0–9.7) in the chemotherapy arm (HR, 0.61; 95% CI, 0.46–0.80; P=0.0002). For other secondary endpoints including ORR, DCR, DoR, and OS, related data of the camrelizumab plus chemotherapy arm were also superior to the chemotherapy arm.
Another study reported at the 2019 ASCO annual meeting showed that for nonsquamous NSCLC patients treated with camrelizumab plus apatinib as second-line and above treatment, the ORR and DCR in 91 evaluable patients were 29.7% and 81.3%, respectively, providing a “chemo-free” option for patients who received second-line treatment ( 50 ).
At the WCLC conference 2019, Professor Baohui Han from Shanghai Chest Hospital affiliated to Shanghai Jiao Tong University reported the relevant data of PD-1 monoclonal antibody sintilimab combined with anlotinib in the first-line treatment of advanced NSCLC ( 51 ). Treatment-naive, stage IIIB/IV NSCLC patients were eligible, and patients with EGFR, ALK or ROS1 mutations were excluded. A total of 22 patients were enrolled in this study, and the results showed that the ORR was 68.2%, the DCR was 100%. At the data cutoff, 81.8% of the patients were still receiving treatment; the proportion of patients with PFS of at least 6 months was 93.8%.
At the WCLC conference 2019, Professors Jie He and Shugeng Gao from Cancer Hospital, Chinese Academy of Medical Sciences released the research results of sintilimab in resectable NSCLC as a neoadjuvant therapy ( 52 ). The MPR reached 40.5% and the pCR reached 16.2%, with manageable safety. This study also designed three postoperative adjuvant treatment cohorts (chemotherapy, PD-1 antibody, and chemotherapy plus PD-1 antibody) synchronously, which are expected to answer the question of postoperative treatment options.
Jianxing He and Wenhua Liang’s research group from Guangzhou Institute of Respiratory Health launched a clinical study (CCTC-1901) in relation to sintilimab treatment in early-stage multiple primary lung cancer (MPLC) with ground-glass density ( 53 ). This is a single-center, single-arm, prospective Simon’s two-stage design phase II clinical trial, aimed at assessing the efficacy of sintilimab in very early-stage (“stage 0”) lung cancer.
Toripalimab
At the WCLC 2019 conference, Professor Caicun Zhou from Shanghai Pulmonary Hospital presented the latest results of a study on toripalimab plus chemotherapy in advanced NSCLC patients with EGFR activating mutations for whom prior EGFR-TKI therapies have failed ( 54 ). A total of 40 patients are included in this study and the ORR reached 32.5% at the 12th week. As of July 25, 2019, the ORR was 50.0%, the DCR was 87.5%, PR had occurred in 20 patients, 15 patients (including 1 unconfirmed PR) performed SD, the mDoR was 7.0 months, and the mPFS was 7.0 months.
Biomarkers for the drug efficacy
In a study published in JAMA ( 55 ), Flatiron Health and Foundation Medicine (FMI) confirmed the associations between clinical and genomic characteristics of NSCLC patients through the data of real-world study and demonstrated that targeted therapy could prolong the OS of patients with driver mutations compared to without targeted therapy (18.6 vs. 11.4 months; P<0.001), and that patients with TMB-high (TMB-H, ≥20 mutations/Mb) attained longer OS after receiving IO therapy compared to the patients with TMB-low/intermediate (TMB-L/I, <20 mutations/Mb) (16.8 vs. 8.5 months).
An analysis of the FDA database was published in JAMA Oncology ( 56 ), indicating that a lung immune prognostic index (LIPI) derived from neutrophils/(leukocytes minus neutrophils) ratio (dNLR) and lactate dehydrogenase (LDH) was significantly correlated with the PFS and OS of immune checkpoint inhibitor (ICI) therapy, targeted therapy, and chemotherapy in advanced NSCLC patients.
Professor Jie Wang and Zhijie Wang from Cancer Hospital, Chinese Academy of Medical Sciences evaluated the feasibility of blood-based TMB (bTMB) as a predictor of efficacy in advanced NSCLC treated with immunotherapy in the Chinese population ( 57 ). The results showed that the response rate and response duration of advanced NSCLC patients treated with anti-PD-1/PD-L1 monoclonal antibody could be predicted by adopting a NCC-GP150 panel based on 150 genes to estimate bTMB based on ctDNA.
Conclusions
With the incidence of lung cancer increasing, various centers worldwide have gradually started to value early screening and diagnosis of lung cancer. The survival of lung cancer patients has significantly improved compared with historical controls, turning lung cancer from an incurable disease into a chronic disease and suggesting that lung cancer prevention and control need to be carried out with reference to the management mode of common diseases, including popular science and screening activities. Systemic therapy, especially immunotherapy in stage I–III lung cancer, reached a new milestone. Treatment of lung cancer tended to be comprehensive, diversified, and individualized. It is of great importance to explore a biomarker that can accurately predict the efficacy of immunotherapy in the treatment of lung cancer patients. In addition, the mechanism of drug resistance in targeted therapy and how to overcome it is deserving of further investigation in the future.
Acknowledgments
Funding: None.
Ethical Statement: The authors are accountable for all aspects of the work in ensuring that questions related to the accuracy or integrity of any part of the work are appropriately investigated and resolved.
Conflicts of Interest: JH serves as the unpaid Executive Editor-in-Chief and WL serves as the unpaid editorial board member of Journal of Thoracic Disease . The other authors have no conflicts of interest to declare.
- 1. Zeng H, Chen W, Zheng R, et al. Changing cancer survival in China during 2003-15: a pooled analysis of 17 population-based cancer registries. Lancet Glob Health 2018;6:e555-67. 10.1016/S2214-109X(18)30127-X [ DOI ] [ PubMed ] [ Google Scholar ]
- 2. Zhou M, Wang H, Zeng X, et al. Mortality, morbidity, and risk factors in China and its provinces, 1990-2017: a systematic analysis for the Global Burden of Disease Study 2017. Lancet 2019;394:1145-58. 10.1016/S0140-6736(19)30427-1 [ DOI ] [ PMC free article ] [ PubMed ] [ Google Scholar ]
- 3. Yang JJ, Yu D, Xiang YB, et al. Association of Dietary Fiber and Yogurt Consumption With Lung Cancer Risk: A Pooled Analysis. JAMA Oncol 2019. [Epub ahead of print]. [ DOI ] [ PMC free article ] [ PubMed ] [ Google Scholar ]
- 4. Dai J, Lv J, Zhu M, et al. Identification of risk loci and a polygenic risk score for lung cancer: a large-scale prospective cohort study in Chinese populations. Lancet Respir Med 2019;7:881-91. 10.1016/S2213-2600(19)30144-4 [ DOI ] [ PMC free article ] [ PubMed ] [ Google Scholar ]
- 5. Cohn AL, Seiden M, Kurtzman KN, et al. The Circulating Cell-free Genome Atlas (CCGA) Study: Follow-up (F/U) on non-cancer participants with cancer-like cell-free DNA signals. J Clin Oncol 2019;37:abstr 5574.
- 6. Oxnard GR, Klein EA, Seiden MV, et al. Simultaneous multi-cancer detection and tissue of origin (TOO) localization using targeted bisulfite sequencing of plasma cell-free DNA (cfDNA). Ann Oncol 2019;30:912. 10.1093/annonc/mdz394.074 [ DOI ] [ Google Scholar ]
- 7. Liang W, Zhao Y, Huang W, et al. Non-invasive diagnosis of early-stage lung cancer using high-throughput targeted DNA methylation sequencing of circulating tumor DNA (ctDNA). Theranostics 2019;9:2056-70. 10.7150/thno.28119 [ DOI ] [ PMC free article ] [ PubMed ] [ Google Scholar ]
- 8. Kwiatkowski DJ, Rusch VW, Chaft JE, et al. Neoadjuvant atezolizumab in resectable non-small cell lung cancer (NSCLC): Interim analysis and biomarker data from a multicenter study (LCMC3). J Clin Oncol 2019;37:abstr 8503.
- 9. Cascone T, William WN, Weissferdt A, et al. Neoadjuvant nivolumab (N) or nivolumab plus ipilimumab (NI) for resectable non-small cell lung cancer (NSCLC): Clinical and correlative results from the NEOSTAR study. J Clin Oncol 2019;37:abstr 8504.
- 10. Provencio M, Nadal E, Insa A, et al. Neoadjuvant chemo-immunotherapy for the treatment of stage IIIA resectable non-small-cell lung cancer (NSCLC): A phase II multicenter exploratory study—Final data of patients who underwent surgical assessment. J Clin Oncol 2019;37:abstr 8509.
- 11. Ball D, Mai GT, Vinod S, et al. Stereotactic ablative radiotherapy versus standard radiotherapy in stage 1 non-small-cell lung cancer (TROG 09.02 CHISEL): a phase 3, open-label, randomised controlled trial. Lancet Oncol 2019;20:494-503. 10.1016/S1470-2045(18)30896-9 [ DOI ] [ PubMed ] [ Google Scholar ]
- 12. Zeng Y, Mayne N, Yang CJ, et al. A Nomogram for Predicting Cancer-Specific Survival of TNM 8th Edition Stage I Non-small-cell Lung Cancer. Ann Surg Oncol 2019;26:2053-62. [ DOI ] [ PubMed ] [ Google Scholar ]
- 13. Zhao Y, Wang W, Liang H, et al. The Optimal Treatment for Stage IIIA-N2 Non-Small Cell Lung Cancer: A Network Meta-Analysis. Ann Thorac Surg 2019;107:1866-75. 10.1016/j.athoracsur.2018.11.024 [ DOI ] [ PubMed ] [ Google Scholar ]
- 14. Gray JE, Villegas AE, Daniel DB, et al. Three-year overall survival update from the PACIFIC trial. J Clin Oncol 2019;37:abstr 8526.
- 15. Hui R, Özgüroğlu M, Villegas A, et al. Patient-reported outcomes with durvalumab after chemoradiotherapy in stage III, unresectable non-small-cell lung cancer (PACIFIC): a randomised, controlled, phase 3 study. Lancet Oncol 2019;20:1670-80. 10.1016/S1470-2045(19)30519-4 [ DOI ] [ PubMed ] [ Google Scholar ]
- 16. Sun A, Hu C, Wong SJ, et al. Prophylactic Cranial Irradiation vs Observation in Patients With Locally Advanced Non-Small Cell Lung Cancer: A Long-term Update of the NRG Oncology/RTOG 0214 Phase 3 Randomized Clinical Trial. JAMA Oncol 2019;5:847-55. 10.1001/jamaoncol.2018.7220 [ DOI ] [ PMC free article ] [ PubMed ] [ Google Scholar ]
- 17. Reck M, Rodriguez-Abreu D, Robinson AG, et al. KEYNOTE-024 3-Year Survival Update: Pembrolizumab vs Platinum-Based Chemotherapy for Advanced Non-Small-Cell Lung Cancer. J Thorac Oncol 2019;14:S243. 10.1016/j.jtho.2019.08.483 [ DOI ] [ PubMed ] [ Google Scholar ]
- 18. Mok TSK, Wu YL, Kudaba I, et al. Pembrolizumab versus chemotherapy for previously untreated, PD-L1-expressing, locally advanced or metastatic non-small-cell lung cancer (KEYNOTE-042): a randomised, open-label, controlled, phase 3 trial. Lancet 2019;393:1819-30. 10.1016/S0140-6736(18)32409-7 [ DOI ] [ PubMed ] [ Google Scholar ]
- 19. Spigel D, de Marinis F, Giaccone G, et al. IMpower110: Interim overall survival (OS) analysis of a phase III study of atezolizumab (atezo) vs platinum-based chemotherapy (chemo) as first-line (1L) treatment (tx) in PD-L1-selected NSCLC. Ann Oncol 2019;30:915. 10.1093/annonc/mdz293 [ DOI ] [ Google Scholar ]
- 20. Antonia SJ, Borghaei H, Ramalingam SS, et al. Four-year survival with nivolumab in patients with previously treated advanced non-small-cell lung cancer: a pooled analysis. Lancet Oncol 2019;20:1395-408. 10.1016/S1470-2045(19)30407-3 [ DOI ] [ PMC free article ] [ PubMed ] [ Google Scholar ]
- 21. Garon EB, Hellmann MD, Costa EC, et al. Five-year long-term overall survival for patients with advanced NSCLC treated with pembrolizumab: Results from KEYNOTE-001. J Clin Oncol 2019;37:LBA9015. 10.1200/JCO.2019.37.18_suppl.LBA9015 [ DOI ] [ PMC free article ] [ PubMed ] [ Google Scholar ]
- 22. Gadgeel SM, Garassino MC, Esteban E, et al. KEYNOTE-189: Updated OS and progression after the next line of therapy (PFS2) with pembrolizumab (pembro) plus chemo with pemetrexed and platinum vs placebo plus chemo for metastatic nonsquamous NSCLC. J Clin Oncol 2019;37:abstr 9013.
- 23. Paz-Ares L, Vicente D, Tafreshi A, et al. LBA82 - Pembrolizumab (pembro) + chemotherapy (chemo) in metastatic squamous NSCLC: Final analysis and progression after the next line of therapy (PFS2) in KEYNOTE-407. Ann Oncol 2019;30:v918-9. 10.1093/annonc/mdz394.080 [ DOI ] [ Google Scholar ]
- 24. West H, McCleod M, Hussein M, et al. Atezolizumab in combination with carboplatin plus nab-paclitaxel chemotherapy compared with chemotherapy alone as first-line treatment for metastatic non-squamous non-small-cell lung cancer (IMpower130): a multicentre, randomised, open-label, phase 3 trial. Lancet Oncol 2019;20:924-37. 10.1016/S1470-2045(19)30167-6 [ DOI ] [ PubMed ] [ Google Scholar ]
- 25. Bauml JM, Mick R, Ciunci C, et al. Pembrolizumab After Completion of Locally Ablative Therapy for Oligometastatic Non-Small Cell Lung Cancer: A Phase 2 Trial. JAMA Oncol 2019. [Epub ahead of print]. 10.1001/jamaoncol.2019.1449 [ DOI ] [ PMC free article ] [ PubMed ] [ Google Scholar ]
- 26. Theelen WSME, Peulen HMU, Lalezari F, et al. Effect of Pembrolizumab After Stereotactic Body Radiotherapy vs Pembrolizumab Alone on Tumor Response in Patients With Advanced Non-Small Cell Lung Cancer: Results of the PEMBRO-RT Phase 2 Randomized Clinical Trial. JAMA Oncol 2019. [Epub ahead of print]. [ DOI ] [ PMC free article ] [ PubMed ]
- 27. Liang H, Liu Z, Cai X, et al. PD-(L)1 inhibitors vs. chemotherapy vs. their combination in front-line treatment for NSCLC: An indirect comparison. Int J Cancer 2019;145:3011-21. 10.1002/ijc.32366 [ DOI ] [ PubMed ] [ Google Scholar ]
- 28. Hellmann MD, Paz-Ares L, Bernabe Caro R, et al. Nivolumab plus Ipilimumab in Advanced Non-Small-Cell Lung Cancer. N Engl J Med 2019;381:2020-31. 10.1056/NEJMoa1910231 [ DOI ] [ PubMed ] [ Google Scholar ]
- 29. Herbst RS, Arkenau HT, Santana-Davila R, et al. Ramucirumab plus pembrolizumab in patients with previously treated advanced non-small-cell lung cancer, gastro-oesophageal cancer, or urothelial carcinomas (JVDF): a multicohort, non-randomised, open-label, phase 1a/b trial. Lancet Oncol 2019;20:1109-23. 10.1016/S1470-2045(19)30458-9 [ DOI ] [ PubMed ] [ Google Scholar ]
- 30. Ramalingam SS, Vansteenkiste J, Planchard D, et al. Overall Survival with Osimertinib in Untreated, EGFR-Mutated Advanced NSCLC. N Engl J Med 2020;382:41-50. 10.1056/NEJMoa1913662 [ DOI ] [ PubMed ] [ Google Scholar ]
- 31. Yang JCH, Kim SW, Kim DW, et al. Osimertinib in Patients With Epidermal Growth Factor Receptor Mutation-Positive Non-Small-Cell Lung Cancer and Leptomeningeal Metastases: The BLOOM Study. J Clin Oncol 2020;38:538-47. 10.1200/JCO.19.00457 [ DOI ] [ PMC free article ] [ PubMed ] [ Google Scholar ]
- 32. Noronha V, Joshi A, Patil VM, et al. Phase III randomized trial comparing gefitinib to gefitinib with pemetrexed-carboplatin chemotherapy in patients with advanced untreated EGFR mutant non-small cell lung cancer (gef vs gef+C). J Clin Oncol 2019;37:abstr 9001.
- 33. Zhang W, Xu J, Lou Y, et al. Chemotherapy Plus EGFR-TKI as First-Line Treatment Provides Better Survival for EGFR Mutation NSCLC Patients: Update Data for NCT02148380 . J Thorac Oncol 2019;14:S236. 10.1016/j.jtho.2019.08.470 [ DOI ] [ Google Scholar ]
- 34. Zhou Q, Wu YL, Cheng Y, et al. 1480O - CTONG 1509: Phase III study of bevacizumab with or without erlotinib in untreated Chinese patients with advanced EGFR-mutated NSCLC. Ann Oncol 2019;30:v603. 10.1093/annonc/mdz260.002 [ DOI ] [ Google Scholar ]
- 35. Nakagawa K, Garon EB, Seto T, et al. Ramucirumab plus erlotinib in patients with untreated, EGFR-mutated, advanced non-small-cell lung cancer (RELAY): a randomised, double-blind, placebo-controlled, phase 3 trial. Lancet Oncol 2019;20:1655-69. 10.1016/S1470-2045(19)30634-5 [ DOI ] [ PubMed ] [ Google Scholar ]
- 36. Zhao Y, Liu J, Cai X, et al. Efficacy and safety of first line treatments for patients with advanced epidermal growth factor receptor mutated, non-small cell lung cancer: systematic review and network meta-analysis. BMJ 2019;367:l5460. 10.1136/bmj.l5460 [ DOI ] [ PMC free article ] [ PubMed ] [ Google Scholar ]
- 37. Cho JH, Lim SH, An HJ, et al. Osimertinib for Patients With Non-Small-Cell Lung Cancer Harboring Uncommon EGFR Mutations: A Multicenter, Open-Label, Phase II Trial (KCSG-LU15-09). J Clin Oncol 2020;38:488-95. 10.1200/JCO.19.00931 [ DOI ] [ PMC free article ] [ PubMed ] [ Google Scholar ]
- 38. Zhou C, Kim SW, Reungwetwattana T, et al. Alectinib versus crizotinib in untreated Asian patients with anaplastic lymphoma kinase-positive non-small-cell lung cancer (ALESIA): a randomised phase 3 study. Lancet Respir Med 2019;7:437-46. 10.1016/S2213-2600(19)30053-0 [ DOI ] [ PubMed ] [ Google Scholar ]
- 39. Yang Y, Zhou J, Zhou J, et al. Efficacy, safety, and biomarker analysis of ensartinib in crizotinib-resistant, ALK-positive non-small-cell lung cancer: a multicentre, phase 2 trial. Lancet Respir Med 2020;8:45-53. 10.1016/S2213-2600(19)30252-8 [ DOI ] [ PubMed ] [ Google Scholar ]
- 40. Drilon A, Siena S, Dziadziuszko R, et al. Entrectinib in ROS1 fusion-positive non-small-cell lung cancer: integrated analysis of three phase 1-2 trials. Lancet Oncol 2020;21:261-70. 10.1016/S1470-2045(19)30690-4 [ DOI ] [ PMC free article ] [ PubMed ] [ Google Scholar ]
- 41. Shaw AT, Solomon BJ, Chiari R, et al. Lorlatinib in advanced ROS1-positive non-small-cell lung cancer: a multicentre, open-label, single-arm, phase 1-2 trial. Lancet Oncol 2019;20:1691-701. 10.1016/S1470-2045(19)30655-2 [ DOI ] [ PubMed ] [ Google Scholar ]
- 42. Fakih M, O'Neil B, Price TJ, et al. Phase 1 study evaluating the safety, tolerability, pharmacokinetics (PK), and efficacy of AMG 510, a novel small molecule KRASG12C inhibitor, in advanced solid tumors. J Clin Oncol 2019;37:abstr 3003.
- 43. Paik PK, Veillon R, Cortot AB, et al. Phase II study of tepotinib in NSCLC patients with METex14 mutations. J Clin Oncol 2019;37:abstr 9005.
- 44. Janne PA, Yu HA, Johnson ML, et al. Safety and preliminary antitumor activity of U3-1402: A HER3-targeted antibody drug conjugate in EGFR TKI-resistant, EGFRm NSCLC. J Clin Oncol 2019;37:abstr 9010.
- 45. Doebele RC, Drilon A, Paz-Ares L, et al. Entrectinib in patients with advanced or metastatic NTRK fusion-positive solid tumours: integrated analysis of three phase 1-2 trials. Lancet Oncol 2020;21:271-82. 10.1016/S1470-2045(19)30691-6 [ DOI ] [ PMC free article ] [ PubMed ] [ Google Scholar ]
- 46. Janne PA, Neal JW, Camidge DR, et al. Antitumor activity of TAK-788 in NSCLC with EGFR exon 20 insertions. J Clin Oncol 2019;37:abstr 9007.
- 47. Gainor JF, Lee DH, Curigliano G, et al. Clinical activity and tolerability of BLU-667, a highly potent and selective RET inhibitor, in patients (pts) with advanced RET-fusion+ non-small cell lung cancer (NSCLC). J Clin Oncol 2019;37:abstr 9008.
- 48. Paz-Ares L, Dvorkin M, Chen Y, et al. Durvalumab plus platinum-etoposide versus platinum-etoposide in first-line treatment of extensive-stage small-cell lung cancer (CASPIAN): a randomised, controlled, open-label, phase 3 trial. Lancet 2019;394:1929-39. 10.1016/S0140-6736(19)32222-6 [ DOI ] [ PubMed ] [ Google Scholar ]
- 49. Zhou C, Chen G, Huang Y, et al. OA04.03 A Randomized Phase 3 Study of Camrelizumab plus Chemotherapy as 1st Line Therapy for Advanced/Metastatic Non-Squamous Non-Small Cell Lung Cancer. J Thorac Oncol 2019;14:S215-6. 10.1016/j.jtho.2019.08.425 [ DOI ] [ Google Scholar ]
- 50. Zhou C, Gao G, Wang YN, et al. Efficacy of PD-1 monoclonal antibody SHR-1210 plus apatinib in patients with advanced nonsquamous NSCLC with wild‐type EGFR and ALK. J Clin Oncol 2019;37:abstr 9112.
- 51. Han B, Chu T, Zhong R, et al. JCSE01.11 Efficacy and Safety of Sintilimab with Anlotinib as First-Line Therapy for Advanced Non-Small Cell Lung Cancer (NSCLC). J Thorac Oncol 2019;14:S129. 10.1016/j.jtho.2019.08.269 [ DOI ] [ Google Scholar ]
- 52. Li N, Ying J, Tao X, et al. Efficacy and Safety of Neoadjuvant PD-1 Blockade with Sintilimab in Resectable Non-Small Cell Lung Cancer. J Thorac Oncol 2019;14:S627-8. 10.1016/j.jtho.2019.08.1322 [ DOI ] [ Google Scholar ]
- 53. Editorial Office . The first attempt worldwide to test PD-1 antibody on multiple "Stage 0" lung cancer. Transl Lung Cancer Res 2019;8:556-7. 10.21037/tlcr.2019.08.01 [ DOI ] [ PMC free article ] [ PubMed ] [ Google Scholar ]
- 54. Zhang J, Zhou C, Zhao Y, et al. MA11.06 A PII Study of Toripalimab, a PD-1 mAb, in Combination with Chemotherapy in EGFR+ Advanced NSCLC Patients Failed to Prior EGFR TKI Therapies. J Thorac Oncol 2019;14:S292. 10.1016/j.jtho.2019.08.587 [ DOI ] [ Google Scholar ]
- 55. Singal G, Miller PG, Agarwala V, et al. Association of Patient Characteristics and Tumor Genomics With Clinical Outcomes Among Patients With Non-Small Cell Lung Cancer Using a Clinicogenomic Database. JAMA 2019;321:1391-9. 10.1001/jama.2019.3241 [ DOI ] [ PMC free article ] [ PubMed ] [ Google Scholar ]
- 56. Mezquita L, Auclin E, Ferrara R, et al. Association of the Lung Immune Prognostic Index With Immune Checkpoint Inhibitor Outcomes in Patients With Advanced Non-Small Cell Lung Cancer. JAMA Oncol 2018;4:351-7. 10.1001/jamaoncol.2017.4771 [ DOI ] [ PMC free article ] [ PubMed ] [ Google Scholar ]
- 57. Wang Z, Duan J, Wang G, et al. Allele Frequency-Adjusted Blood-Based Tumor Mutational Burden as a Predictor of Overall Survival for Patients With NSCLC Treated With PD-(L)1 Inhibitors. J Thorac Oncol 2019. [Epub ahead of print]. 10.1016/j.jtho.2019.12.001 [ DOI ] [ PubMed ] [ Google Scholar ]
- View on publisher site
- PDF (316.9 KB)
- Collections
Similar articles
Cited by other articles, links to ncbi databases.
- Download .nbib .nbib
- Format: AMA APA MLA NLM
Add to Collections
Lung cancer
Affiliations.
- 1 Peter MacCallum Cancer Centre, Melbourne, VIC, Australia; Sir Peter MacCallum Department of Oncology, University of Melbourne, VIC, Australia.
- 2 Department of Medicine, Massachusetts General Hospital, Boston, MA, USA.
- 3 Department of Medicine, Massachusetts General Hospital, Boston, MA, USA. Electronic address: [email protected].
- PMID: 34273294
- DOI: 10.1016/S0140-6736(21)00312-3
Lung cancer is one of the most frequently diagnosed cancers and the leading cause of cancer-related deaths worldwide with an estimated 2 million new cases and 1·76 million deaths per year. Substantial improvements in our understanding of disease biology, application of predictive biomarkers, and refinements in treatment have led to remarkable progress in the past two decades and transformed outcomes for many patients. This seminar provides an overview of advances in the screening, diagnosis, and treatment of non-small-cell lung cancer and small-cell lung cancer, with a particular focus on targeted therapies and immune checkpoint inhibitors.
Copyright © 2021 Elsevier Ltd. All rights reserved.
Publication types
- Research Support, Non-U.S. Gov't
- Carcinoma, Non-Small-Cell Lung / diagnosis*
- Carcinoma, Non-Small-Cell Lung / therapy*
- Lung Neoplasms / diagnosis*
- Lung Neoplasms / therapy*
- Small Cell Lung Carcinoma / diagnosis*
- Small Cell Lung Carcinoma / therapy*
MINI REVIEW article
Lung cancer: understanding its molecular pathology and the 2015 who classification.
- Division of Pathology, The Cancer Institute, Japanese Foundation for Cancer Research, Tokyo, Japan
Lung cancer is the leading cause of cancer-related death worldwide due to late diagnoses and limited treatment interventions. Recently, comprehensive molecular profiles of lung cancer have been identified. These novel characteristics have enhanced the understanding of the molecular pathology of lung cancer. The identification of driver genetic alterations and potential molecular targets has resulted in molecular-targeted therapies for an increasing number of lung cancer patients. Thus, the histopathological classification of lung cancer was modified in accordance with the increased understanding of molecular profiles. This review focuses on recent developments in the molecular profiling of lung cancer and provides perspectives on updated diagnostic concepts in the new 2015 WHO classification. The WHO classification will require additional revisions to allow for reliable, clinically meaningful tumor diagnoses as we gain a better understanding of the molecular characteristics of lung cancer.
Introduction
Lung cancer is the leading cause of cancer-related deaths worldwide in both men and women ( 1 ). It is categorized into two main histological groups: small cell lung carcinoma (SCLC, 15% of all lung cancers) and non-SCLC (NSCLC, 85% of all lung cancers). NSCLCs are generally subcategorized into adenocarcinoma, squamous cell carcinoma (SqCC), and large cell carcinoma. Accumulating evidence suggests that lung cancer represents a group of histologically and molecularly heterogeneous diseases even within the same histological subtype ( 2 – 26 ).
The histopathological classification of lung cancer has recently been revised and published as the 2015 WHO classification ( 2 ). Several major revisions were made in this classification to reflect recent discoveries related to the molecular pathology of lung cancer.
Human comprehensive molecular characterization projects have resulted in the identification of novel molecular characteristics of lung cancer and the different subtypes at levels of DNA alteration, DNA methylation, mRNA expression, microRNA expression, and protein expression. This review introduces and briefly summarizes recent studies on the molecular pathology of lung cancer with a focus on the association between molecular profiles and morphology ( 2 ).
The 2015 Who Classification
The WHO classification was updated based on newly identified molecular profiles and targetable genetic alterations in lung cancer. For lung adenocarcinoma, the 2011 International Association for the Study of Lung Cancer, American Thoracic Society, and European Respiratory Society classification ( 27 ) was mostly adopted in the 2015 WHO classification. Table 1 shows the WHO classification of lung tumors (epithelial tumors) ( 2 ). The major revisions to the WHO classification are described below.
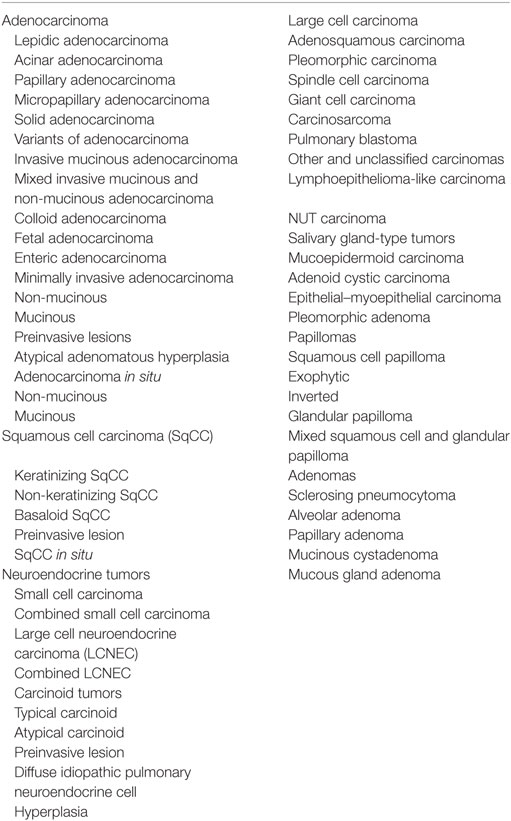
Table 1 . WHO classification of tumors of the lung (epithelial tumors) ( 2 ).
Definition of Adenocarcinoma and SqCC
Pathologists are required to categorize lung cancer into adenocarcinoma and SqCC due to the targetable driver genetic alterations identified in lung adenocarcinoma and inappropriate drugs for SqCCs due to side effects in patients with SqCC. Before the 2015 WHO classification, adenocarcinoma was defined as carcinoma with an acinar/tubular structure or mucin production, whereas SqCC was defined as carcinoma with keratinization or intercellular bridges. If poorly differentiated carcinoma lacking light microscopic evidence of glandular differentiation (Figure 1 A) is proven by immunohistochemistry to express “adenocarcinoma markers,” such as TTF-1 (Figure 1 B) and/or Napsin A (Figure 1 C), it is diagnosed as a solid adenocarcinoma. If poorly differentiated carcinoma lacking light microscopic evidence of squamous differentiation (Figure 1 D) is proven by immunohistochemistry to express “SqCC markers,” ( 28 ) such as p40 (Figure 1 E), CK5/6 (Figure 1 F), and p63, it is diagnosed as non-keratinizing SqCC. Because of this classification, the proportion of large cell carcinoma has been markedly reduced.
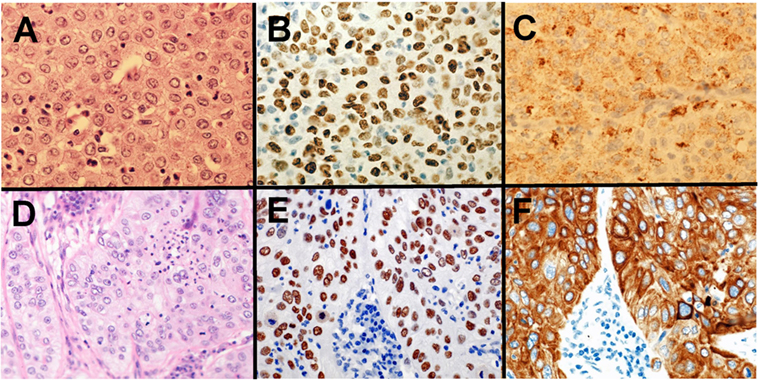
Figure 1 . Solid adenocarcinoma (A–C) and non-keratinizing squamous cell carcinoma (SqCC) (D–F) . Solid adenocarcinoma [ (A) HE staining] is immunohistochemically positive for TTF-1 (B) and Napsin A (C) . Non-keratinizing SqCC [ (D) HE staining] is immunohistochemically positive for p40 (E) and CK5/6 (F) .
Adenocarcinoma
Classification according to the extent of invasiveness.
The 2015 WHO classification divides adenocarcinomas into adenocarcinoma in situ (AIS, preinvasive lesion), minimally invasive adenocarcinoma (MIA), or (overt) invasive adenocarcinoma based on the extent of invasiveness. The disease-free survival rate of AIS and MIA when completely resected is 100% ( 29 ).
Adenocarcinoma in situ is defined as an adenocarcinoma comprising a lepidic pattern with a diameter of ≤3 cm. If the tumor diameter exceeds 3 cm, it is defined as “lepidic predominant adenocarcinoma, suspect AIS” because these tumors are rare and lack adequate characterization.
Minimally invasive adenocarcinoma is defined as an adenocarcinoma with a diameter of ≤3 cm and an invasion size of ≤5 mm. Even if the tumor size and invasion size comply with the definition of MIA, the presence of lymphovascular invasion, pleural invasion, or tumor necrosis can be an exclusion factor for an MIA diagnosis. If the tumor size exceeds 3 cm with an invasion size of ≤5 mm, it is defined as “lepidic predominant adenocarcinoma, suspect MIA” because these tumors are rare and lack adequate characterization.
The term “invasive adenocarcinoma, mixed subtype” for invasive adenocarcinoma is no longer used. Invasive adenocarcinoma is now classified using five predominant patterns: lepidic, papillary, acinar, micropapillary, and solid adenocarcinoma.
Variants of Invasive Adenocarcinoma
The term “mucinous bronchioloalveolar carcinoma (BAC)” is no longer used because most mucinous BACs included invasive components. Therefore, the term “invasive mucinous adenocarcinoma (IMA)” replaced mucinous BAC. IMA and mucinous AIS are accurately classified based on invasiveness. Besides IMA, variants of invasive adenocarcinoma comprise enteric, colloid, and fetal adenocarcinoma. Enteric adenocarcinoma is defined as adenocarcinoma with a predominant component that resembles adenocarcinoma arising in the colorectum and often shows CDX2 immunoreactivity ( 30 ).
Squamous Cell Carcinoma
In the 2015 WHO classification, SqCCs are classified into keratinizing SqCC, non-keratinizing SqCC, and basaloid SqCC. Before this classification, basaloid SqCC was categorized as a variant of large cell carcinoma. However, basaloid SqCC immunohistochemically shows “SqCC markers” (e.g., p40, CK5/6, and p63) and is therefore categorized as SqCC.
Neuroendocrine Tumors
In the 2015 WHO classification, a new category of “neuroendocrine tumors” was established. Invasive neuroendocrine tumors comprise three subtypes: SCLC, large cell neuroendocrine carcinoma (LCNEC), and carcinoid tumor (typical/atypical). Diffuse idiopathic pulmonary neuroendocrine cell hyperplasia is extremely rare and non-invasive; therefore, its clinical importance is low. On the other hand, the distinction between a high-grade neuroendocrine tumor (HGNET), comprising SCLC and LCNEC, and a carcinoid tumor is very important in both pathological and clinical practice. HGNET is one of the most aggressive subtypes and characterized by a history of heavy smoking in the patient, whereas carcinoid tumors usually carry a benign prognosis and frequently occur in patients with no history of smoking.
Comprehensive Molecular Profiling
With the emergence of high-throughput sequencing techniques, detailed molecular profiles of lung cancer have been identified. The Cancer Genome Atlas (TCGA) research network identified genomic and other molecular alterations among a number of different types of cancer, including lung cancer. In this section, the comprehensive molecular profiles of lung cancer, mainly determined by TCGA, are introduced.
The comprehensive molecular profiling of 230 lung adenocarcinoma by TCGA was published in 2014 ( 3 ). The authors reported high rates of somatic mutations (mean: 8.9 mutations per megabase) and identified 18 statistically significant genetic mutations: TP53 (46%), KRAS (33%), KEAP1 (17%), STK11 (17%), EGFR (14%), NF1 (11%), BRAF (10%), SETD2 (9%), RBM10 (8%), MGA (8%), MET (7%), ARID1A (7%), PIK3CA (7%), SMARCA4 (6%), RB1 (4%), CDKN2A (4%), U2AF1 (3%), and RIT1 (2%).
Furthermore, approximately 75% of the lung adenocarcinomas examined harbored genetic alterations that promote the RTK/RAS/RAF signaling pathway. Of all the cases, 62% showed driver genetic alterations that promote the RTK/RAS/RAF pathway. Among them, mutations in KRAS, EGFR , and BRAF comprised 32, 11, and 7.0%, respectively. Other genetic alterations that promote the RTK/RAS/RAF pathway included MET exon 14 skipping (4.3%), ERBB2 (or HER2 ) mutation (1.7%), ROS1 fusion (1.7%), ALK fusion (1.3%), MAP2K1 mutation (0.9%), RET fusion (0.9%), NRAS mutation (0.4%), and HRAS mutation (0.4%). An examination of the DNA copy number of the remaining 38% cases without driver genetic alterations that promote the RTK/RAS/RAF pathway revealed amplification of oncogenes in the RTK/RAS/RAF pathway: ERBB2 amplification (0.9%) and MET amplification (2.2%). The authors also identified new genetic alterations in this pathway: mutations in NF1 and RIT1 . NF1 is a tumor suppressor gene that regulates the RTK/RAS/RAF pathway, and the frequency of NF1 mutations was 8.3%. Similarly, RIT1 constitutes a part of the RTK/RAS/RAF pathway, and the frequency of RIT1 mutations was 2.2%. Consequently, 75% of lung adenocarcinomas have genetic alterations that promote the RTK/RAS/RAF pathway. This study on the comprehensive molecular characterization of lung adenocarcinoma widened the potential therapeutic targets of lung adenocarcinoma.
This study conducted mRNA profiling and provided new transcriptional subtypes: i.e., the terminal respiratory unit (TRU, formerly bronchioid), the proximal-inflammatory (PI, formerly squamoid), and the proximal-proliferative (PP, formerly magnoid) mRNA subtypes. The TRU subtype was enriched for EGFR mutation and kinase fusions. The PI subtype was characterized by solid morphology and co-mutation of NF1 and TP53 . The PP subtype was enriched for KRAS mutation and STK11 inactivation.
DNA methylation profiling divided lung adenocarcinomas into three subtypes: i.e., CpG island methylator phenotype (CIMP)-high, CIMP-intermediate, and CIMP-low subtypes. CIMP-high tumors often showed DNA hypermethylation of CDKN2A, GATA2, GATA5, HIC1, HOXA9, HOXD13, RASSF1, SFRP1, SOX17 , and WIF1 . The CIMP-high subtype was enriched for MYC overexpression as well as for DNA hypermethylation of genes in WNT pathway.
Protein profiling divided lung adenocarcinomas into six subtypes. The top 50 differentially expressed proteins among the 6 subtypes included Cyclin D1, Smad4, p-mTOR, Rad50, beta-Catenin, and HER2. The six subtypes partially overlapped with the mRNA three subtypes.
The comprehensive molecular profiling of 178 cases of SqCC by TCGA was published in 2012 ( 4 ). As can be expected from the history of heavy smoking in SqCC patients, SqCCs are characterized by complex genomic alterations. The authors indeed detected a mean of 360 exonic mutations, 165 genomic rearrangements, and 323 segments of copy number alteration per tumor. They identified 11 statistically significant genetic mutations: TP53, CDKN2A, PTEN, PIK3CA, KEAP1, MLL2, HLA-A, NFE2L2, NOTCH1, RB1 , and PDYN . The frequency of TP53 mutations was 90%. The authors identified novel loss-of-function mutations in the HLA-A class I major histocompatibility gene. They also conducted pathway analyses and identified pathways related to oxidative damage, including KEAP1 and NFE2L2 in 34% of cases, squamous cell differentiation pathway, including overexpression of SOX2 and TP63 in 44% of cases, PI3K/AKT pathway in 47% of cases, and inactivation of CDKN2A in 72% of SqCC cases. These results provided us multiple potential targets for the treatment of lung SqCC.
The mRNA profiling divided SqCCs into four subtypes: i.e., classical, basal, secretory, and primitive subtypes. The classical subtype was characterized by pronounced hypermethylation, chromosomal instability, and alterations in KEAP1, NFE2L2 , and PTEN . The basal subtype showed NF1 alterations. The primitive subtype was enriched for RB1 and PTEN alterations.
MicroRNA profiling divided SqCCs into four subtypes. These four subtypes roughly overlapped with the mRNA four subtypes. DNA methylation profiling also divided SqCCs into four subtypes (methylation clusters 1–4). Methylation cluster 4 showed little DNA hypermethylation and included most of the primitive mRNA subtype. Cluster 3 was predominantly made up of the classical mRNA subtype and enriched for NFE2L2 mutations. Cluster 3 and Cluster 2 showed the highest levels of DNA hypermethylation. Cluster 1 showed intermediate DNA hypermethylation levels.
Small Cell Lung Carcinoma
In 2012, comprehensive genomic analyses were reported by two groups. Rudin et al. identified SOX2 as a frequently amplified gene in SCLC ( 6 ). In vitro suppression of SOX2 blocked the proliferation of SOX2 -amplified SCLC cell lines. Furthermore, they identified a recurrent RLF–MYCL1 fusion in SCLC with RNA sequencing. In vitro silencing of MYCL1 in SCLC cell lines with an RLF–MYCL1 fusion decreased cell proliferation. On the other hand, Peifer et al. reported recurrent mutations in genes that encode histone modifiers, including CREBBP, EP300 , and MLL , suggesting histone modification as a major feature of SCLC ( 7 ).
In 2015, George et al. sequenced the genome of 110 SCLCs and found comprehensive genomic profiles ( 5 ). SCLC is characterized by highly complex genomic alterations, and C:G>A:T transversions were found in 28% of all mutations on average, which is a characteristic pattern of heavy smoking. Almost all examined SCLCs showed bi-allelic inactivation of TP53 and RB1 . Genomic alterations of tumor suppressor gene TP73 was observed in 13% of SCLCs. Among TP73 genomic alterations, genomic rearrangement of TP73Δex2/3 was identified. Because TP73Δex2/3 promotes carcinogenesis, TP73Δex2/3 -targeted strategy is a promising treatment for SCLC. The authors also observed inactivating mutations of NOTCH family genes ( 31 ), which suppressed neuroendocrine differentiation via the regulation of ASCL1 expression in 25% of SCLCs.
The mRNA profiling divided SCLCs into two groups ( 5 ). Most of SCLCs (83%) were categorized into group 2, which was characterized by higher expressions of CHGA, GRP, ASCL1 , and DLK1 . Group 1, comprising 17% of SCLCs, showed lower expressions of these four genes.
Histology and Genetic Profiles
Close associations exist between histology/morphology and genetic profiles. In this section, several of these associations are introduced.
Driver Genetic Alterations and Histology of Lung Adenocarcinoma
EGFR mutation is one of the most common driver mutations in lung adenocarcinoma, and EGFR -mutated adenocarcinoma is characterized by East-Asian ethnicity, female gender, and non/light-smoking history ( 32 ). Pathologically, EGFR -mutated lung adenocarcinomas typically show nuclear TTF-1 (NKX2-1) immunostaining and a hobnail cell type. Adenocarcinomas with a micropapillary pattern have a higher frequency of EGFR mutations than adenocarcinomas without this pattern ( 33 , 34 ).
Fusion genes were recently identified as oncogenic drivers. In lung adenocarcinoma, rearranged genes, including ALK, ROS1, RET, NTRK1 , and NRG1 , have been reported. ALK -rearranged adenocarcinoma comprises 4–5% of adenocarcinomas ( 35 ). ROS1 - and RET -rearranged adenocarcinoma each comprises approximately 1% ( 35 , 36 ). These rearranged adenocarcinomas show a good clinical response to molecular-targeted drugs. ALK -rearranged adenocarcinoma is characterized by a TTF-1 cell lineage, an acinar structure with mucin/signet-ring cell pattern, non-/light-smoking history, and young onset ( 37 – 39 ). ROS1 - and RET -rearranged adenocarcinomas have a similar histology to ALK -rearranged adenocarcinoma, such as mucinous cribriform pattern or solid signet-ring cell pattern ( 35 , 40 , 41 ).
NTRK1 fusion in lung adenocarcinoma was identified by Vaishnavi et al. ( 42 ) A NTRK1 -rearranged adenocarcinoma identified by Shim et al. belonged to IMA subtype ( 43 ). NTRK1 is a TRKA kinase; thus, TRKA kinase inhibitors have the potential to be used in the treatments of NTRK1 -rearranged adenocarcinomas. NRG1 -rearranged adenocarcinoma is also characterized by IMA ( 44 , 45 ). IMAs are frequently KRAS -mutated; therefore, Nakaoku et al. examined 34 IMA cases without KRAS mutations, partly by whole-transcriptome sequencing ( 45 ). They identified five oncogenic fusions: CD74–NRG1, SLC3A2–NRG1, EZR–ERBB4, TRIM24–BRAF , and KIAA1468–RET . These fusion genes were mutually exclusive from KRAS mutations. NRG1 fusions were present in 17.6% (6/34) of KRAS -wild-type IMAs. Because fusions of NRG1, ERBB4, BRAF , and RET are potential molecular targets, their clinical applications are promising.
Driver genetic alterations in lung adenocarcinomas differ between Caucasians and Asians, and between smokers and non-smokers. For example, KRAS mutations are frequently detected in lung adenocarcinomas in smokers, whereas genetic alterations in EGFR, ALK, ROS1 , and RET are frequently detected in lung adenocarcinomas in non-smokers. The frequencies of driver genetic alterations in Caucasians were determined in TCGA study ( 3 ), whereas lung adenocarcinoma in Asians is characterized by a high frequency of EGFR mutations (approximately 50%) and low frequency of KRAS mutations (approximately 10%).
MicroRNAs and Histological Subtypes of Lung Adenocarcinoma
MicroRNAs are small single-stranded non-coding RNAs (19–22 nucleotides in length) that play important regulatory roles, including lung carcinogenesis ( 46 – 50 ). Nadal et al. performed microRNA profiling of adenocarcinomas subclassified by the 2015 WHO classification ( 51 ). They demonstrated that different histological subtypes of lung adenocarcinoma have distinct microRNA expression profiles. Unsupervised hierarchical clustering divided adenocarcinomas into three major clusters, which correlated with the histological subtypes of the 2015 WHO classification. Cluster 1 included fewer acinar and solid adenocarcinomas, and nearly all the tumors in cluster 1 were categorized as lepidic adenocarcinomas or IMAs. In contrast, clusters 2 and 3 included more acinar and solid adenocarcinomas and fewer lepidic adenocarcinomas and IMAs. Solid adenocarcinoma was characterized by the overexpression of miR-27a, miR-212 , and miR-132 ( 51 ).
Enteric adenocarcinoma is one of the new variants of lung adenocarcinoma in the 2015 WHO classification. It is defined as an adenocarcinoma with a predominant component that shows enteric differentiation ( 30 ). Garajová et al. found that the microRNA signature of enteric adenocarcinomas shows similarities with NSCLCs and pancreatic adenocarcinomas, but not with colorectal adenocarcinomas. Enteric adenocarcinomas share oncogenic microRNAs ( miR-31*, miR-126*, miR-506, miR-508-3p , and miR-514 ) with pancreatic adenocarcinomas ( 52 ).
MicroRNAs and SCLC
Small cell lung carcinoma, which is categorized into neuroendocrine tumor, shows high expression of ASCL1, which is a transcription factor that promotes neuroendocrine differentiation. A study reported that miR-375 expression was promoted by ASCL1 in lung neuroendocrine carcinoma ( 53 ). This study suggested that miR-375 might reduce the YAP1-associated proliferative arrest by inhibiting YAP1. Another study examined microRNAs from 50 SCLC patients and 30 healthy individuals, and suggested that level of miR-92a-2 in plasma could be a potential non-invasive method for the SCLC diagnosis ( 54 ). Recent evidence suggests that miR-21 expression may be higher in HGNET (i.e., SCLC and LCNEC) than in typical/atypical carcinoid, and that high expression of miR-34a may be associated with atypical carcinoids ( 55 ).
Molecular Alterations and Their Therapeutic Relevance
Molecular alterations, which have been recently elucidated in lung cancer, are used in clinical practice or potentially useful therapeutic tools in the treatment of lung cancer.
In lung adenocarcinoma, fusions of ALK, RET , and ROS1 have been shown to be targetable genetic alterations ( 35 , 36 ). In IMA, new fusions of NTRK1, NRG1, ERBB4, BRAF , and RET were identified as targetable genetic alterations ( 43 , 45 ). The comprehensive molecular profiling in lung adenocarcinoma by TCGA research network has shown that 75% of lung adenocarcinomas have genetic alterations that promote RTK/RAS/RAF pathway; these genetic alterations included newly identified NF1 and RIT1 mutations, both of which are potentially targetable ( 3 ).
For lung SqCC, no effective targetable agents have been developed specifically. The comprehensive molecular profiling in lung SqCC by TCGA research network has identified a potential therapeutic target in the most lung SqCCs that they investigated ( 4 ). The alterations in targetable oncogenic pathways in lung SqCCs included RTK pathway (26%), RAS pathway (24%), and PI(3)K pathway (47%) ( 4 ). Targeting these pathways are potential ways of the treatment for lung SqCC.
Small cell lung carcinoma is the deadliest subtype of lung cancer, and no established molecular-targeted therapy for SCLC exists. For SCLC, recent studies discovered potentially targetable genetic alterations, including SOX2 amplification, RLF-MYCL1 fusion, and TP73Δex2/3 ( 5 , 6 ).
In the era that we have comprehensive genomic data of lung cancer, we should try to treat lung cancer thorough more efficacious targeted therapeutic interventions.
Conclusion and Future Directions
This review focuses on newly identified molecular pathology and the 2015 WHO classification of lung cancer, which was revised based on the better understanding of the molecular pathology of lung cancer and recent advancements in newly developed molecular-targeted drugs. However, in an era of precision medicine, these classification changes remain inadequate. In the near feature, the WHO classification will need to be further revised to allow for reliable, clinically meaningful tumor diagnoses that reflect our better understanding of the molecular characteristics of lung cancer.
Author Contributions
KI conceived the review, wrote the text, created the table and figure, and approved the final draft.
Conflict of Interest Statement
The author declares that the research was conducted in the absence of any commercial or financial relationships that could be construed as a potential conflict of interest.
This study was supported by JSPS KAKENHI Grant Number JP16K08679 and the Ministry of the Environment, Japan.
1. Torre LA, Bray F, Siegel RL, Ferlay J, Lortet-Tieulent J, Jemal A. Global cancer statistics, 2012. CA Cancer J Clin (2015) 65(2):87–108. doi:10.3322/caac.21262
PubMed Abstract | CrossRef Full Text | Google Scholar
2. Travis WD, Brambilla E, Burke AP, Marx A, Nicholson AG. WHO Classification of Tumours of the Lung, Pleura, Thymus and Heart . 4th ed. Lyon: IARC (2015).
Google Scholar
3. The Cancer Genome Atlas Research Network. Comprehensive molecular profiling of lung adenocarcinoma. Nature (2014) 511(7511):543–50. doi:10.1038/nature13385
4. The Cancer Genome Atlas Research Network. Comprehensive genomic characterization of squamous cell lung cancers. Nature (2012) 489(7417):519–25. doi:10.1038/nature11404
CrossRef Full Text | Google Scholar
5. George J, Lim JS, Jang SJ, Cun Y, Ozretic L, Kong G, et al. Comprehensive genomic profiles of small cell lung cancer. Nature (2015) 524(7563):47–53. doi:10.1038/nature14664
6. Rudin CM, Durinck S, Stawiski EW, Poirier JT, Modrusan Z, Shames DS, et al. Comprehensive genomic analysis identifies SOX2 as a frequently amplified gene in small-cell lung cancer. Nat Genet (2012) 44(10):1111–6. doi:10.1038/ng.2405
7. Peifer M, Fernandez-Cuesta L, Sos ML, George J, Seidel D, Kasper LH, et al. Integrative genome analyses identify key somatic driver mutations of small-cell lung cancer. Nat Genet (2012) 44(10):1104–10. doi:10.1038/ng.2396
8. Beer DG, Kardia SL, Huang CC, Giordano TJ, Levin AM, Misek DE, et al. Gene-expression profiles predict survival of patients with lung adenocarcinoma. Nat Med (2002) 8(8):816–24. doi:10.1038/nm733
9. Paez JG, Janne PA, Lee JC, Tracy S, Greulich H, Gabriel S, et al. EGFR mutations in lung cancer: correlation with clinical response to gefitinib therapy. Science (2004) 304(5676):1497–500. doi:10.1126/science.1099314
10. Lynch TJ, Bell DW, Sordella R, Gurubhagavatula S, Okimoto RA, Brannigan BW, et al. Activating mutations in the epidermal growth factor receptor underlying responsiveness of non-small-cell lung cancer to gefitinib. N Engl J Med (2004) 350(21):2129–39. doi:10.1056/NEJMoa040938
11. Inamura K, Fujiwara T, Hoshida Y, Isagawa T, Jones MH, Virtanen C, et al. Two subclasses of lung squamous cell carcinoma with different gene expression profiles and prognosis identified by hierarchical clustering and non-negative matrix factorization. Oncogene (2005) 24(47):7105–13. doi:10.1038/sj.onc.1208858
12. Yanaihara N, Caplen N, Bowman E, Seike M, Kumamoto K, Yi M, et al. Unique microRNA molecular profiles in lung cancer diagnosis and prognosis. Cancer Cell (2006) 9(3):189–98. doi:10.1016/j.ccr.2006.01.025
13. Soda M, Choi YL, Enomoto M, Takada S, Yamashita Y, Ishikawa S, et al. Identification of the transforming EML4-ALK fusion gene in non-small-cell lung cancer. Nature (2007) 448(7153):561–6. doi:10.1038/nature05945
14. Inamura K, Togashi Y, Nomura K, Ninomiya H, Hiramatsu M, Satoh Y, et al. Let-7 microRNA expression is reduced in bronchioloalveolar carcinoma, a non-invasive carcinoma, and is not correlated with prognosis. Lung Cancer (2007) 58(3):392–6. doi:10.1016/j.lungcan.2007.07.013
15. Bansal P, Osman D, Gan GN, Simon GR, Boumber Y. Recent advances in immunotherapy in metastatic NSCLC. Front Oncol (2015) 6:239. doi:10.3389/fonc.2016.00239
16. Rizvi NA, Hellmann MD, Snyder A, Kvistborg P, Makarov V, Havel JJ, et al. Cancer immunology. Mutational landscape determines sensitivity to PD-1 blockade in non-small cell lung cancer. Science (2015) 348(6230):124–8. doi:10.1126/science.aaa1348
17. Inamura K, Yokouchi Y, Sakakibara R, Kobayashi M, Subat S, Ninomiya H, et al. Relationship of tumor PD-L1 expression with EGFR wild-type status and poor prognosis in lung adenocarcinoma. Jpn J Clin Oncol (2016) 46(10):935–41. doi:10.1093/jjco/hyw087
18. Mirzapoiazova T, Mambetsariev N, Lennon FE, Mambetsariev B, Berlind JE, Salgia R, et al. HABP2 is a novel regulator of hyaluronan-mediated human lung cancer progression. Front Oncol (2015) 5:164. doi:10.3389/fonc.2015.00164
19. Hwang DH, Sholl LM, Rojas-Rudilla V, Hall DL, Shivdasani P, Garcia EP, et al. KRAS and NKX2-1 mutations in invasive mucinous adenocarcinoma of the lung. J Thorac Oncol (2016) 11(4):496–503. doi:10.1016/j.jtho.2016.01.010
20. Polley E, Kunkel M, Evans D, Silvers T, Delosh R, Laudeman J, et al. Small cell lung cancer screen of oncology drugs, investigational agents, and gene and microRNA expression. J Natl Cancer Inst (2016) 108(10):djw122. doi:10.1093/jnci/djw122
21. Bansal P, Osman D, Gan GN, Simon GR, Boumber Y. Recent advances in targetable therapeutics in metastatic non-squamous NSCLC. Front Oncol (2016) 6:112. doi:10.3389/fonc.2016.00112
22. Inamura K, Yokouchi Y, Kobayashi M, Sakakibara R, Ninomiya H, Subat S, et al. Tumor B7-H3 (CD276) expression and smoking history in relation to lung adenocarcinoma prognosis. Lung Cancer (2017) 103:44–51. doi:10.1016/j.lungcan.2016.11.013
23. Kim H, Yang JM, Jin Y, Jheon S, Kim K, Lee CT, et al. MicroRNA expression profiles and clinicopathological implications in lung adenocarcinoma according to EGFR, KRAS, and ALK status. Oncotarget (2017) 8(5):8484–98. doi:10.18632/oncotarget.14298
24. Saruwatari K, Ikemura S, Sekihara K, Kuwata T, Fujii S, Umemura S, et al. Aggressive tumor microenvironment of solid predominant lung adenocarcinoma subtype harboring with epidermal growth factor receptor mutations. Lung Cancer (2016) 91:7–14. doi:10.1016/j.lungcan.2015.11.012
25. Inamura K, Yokouchi Y, Kobayashi M, Ninomiya H, Sakakibara R, Subat S, et al. Association of tumor TROP2 expression with prognosis varies among lung cancer subtypes. Oncotarget (2017) 8(17):28725–35. doi:10.18632/oncotarget.5647
26. Zhang Y, Li ZY, Hou XX, Wang X, Luo YH, Ying YP, et al. Clinical significance and effect of AEG-1 on the proliferation, invasion, and migration of NSCLC: a study based on immunohistochemistry, TCGA, bioinformatics, in vitro and in vivo verification. Oncotarget (2017) 8(10):16531–52. doi:10.18632/oncotarget.4972
27. Travis WD, Brambilla E, Noguchi M, Nicholson AG, Geisinger KR, Yatabe Y, et al. International Association for the Study of Lung Cancer/American Thoracic Society/European Respiratory Society: international multidisciplinary classification of lung adenocarcinoma. J Thorac Oncol (2011) 6(2):244–85. doi:10.1097/JTO.0b013e318206a221
28. Bishop JA, Teruya-Feldstein J, Westra WH, Pelosi G, Travis WD, Rekhtman N. p40 (DeltaNp63) is superior to p63 for the diagnosis of pulmonary squamous cell carcinoma. Mod Pathol (2012) 25(3):405–15. doi:10.1038/modpathol.2011.173
29. Noguchi M, Morikawa A, Kawasaki M, Matsuno Y, Yamada T, Hirohashi S, et al. Small adenocarcinoma of the lung. Histologic characteristics and prognosis. Cancer (1995) 75(12):2844–52. doi:10.1002/1097-0142(19950615)75:12<2844::AID-CNCR2820751209>3.0.CO;2-#
30. Inamura K, Satoh Y, Okumura S, Nakagawa K, Tsuchiya E, Fukayama M, et al. Pulmonary adenocarcinomas with enteric differentiation: histologic and immunohistochemical characteristics compared with metastatic colorectal cancers and usual pulmonary adenocarcinomas. Am J Surg Pathol (2005) 29(5):660–5. doi:10.1097/01.pas.0000160438.00652.8b
31. Crabtree JS, Singleton CS, Miele L. Notch signaling in neuroendocrine tumors. Front Oncol (2016) 6:94. doi:10.3389/fonc.2016.00094
32. Shigematsu H, Lin L, Takahashi T, Nomura M, Suzuki M, Wistuba II, et al. Clinical and biological features associated with epidermal growth factor receptor gene mutations in lung cancers. J Natl Cancer Inst (2005) 97(5):339–46. doi:10.1093/jnci/dji055
33. Ninomiya H, Hiramatsu M, Inamura K, Nomura K, Okui M, Miyoshi T, et al. Correlation between morphology and EGFR mutations in lung adenocarcinomas significance of the micropapillary pattern and the hobnail cell type. Lung Cancer (2009) 63(2):235–40. doi:10.1016/j.lungcan.2008.04.017
34. Inamura K, Ninomiya H, Ishikawa Y, Matsubara O. Is the epidermal growth factor receptor status in lung cancers reflected in clinicopathologic features? Arch Pathol Lab Med (2010) 134(1):66–72. doi:10.1043/2008-0586-RAR1.1
35. Takeuchi K, Soda M, Togashi Y, Suzuki R, Sakata S, Hatano S, et al. RET, ROS1 and ALK fusions in lung cancer. Nat Med (2012) 18(3):378–81. doi:10.1038/nm.2658
36. Kohno T, Ichikawa H, Totoki Y, Yasuda K, Hiramoto M, Nammo T, et al. KIF5B-RET fusions in lung adenocarcinoma. Nat Med (2012) 18(3):375–7. doi:10.1038/nm.2644
37. Inamura K, Takeuchi K, Togashi Y, Nomura K, Ninomiya H, Okui M, et al. EML4-ALK fusion is linked to histological characteristics in a subset of lung cancers. J Thorac Oncol (2008) 3(1):13–7. doi:10.1097/JTO.0b013e31815e8b60
38. Shaw AT, Yeap BY, Mino-Kenudson M, Digumarthy SR, Costa DB, Heist RS, et al. Clinical features and outcome of patients with non-small-cell lung cancer who harbor EML4-ALK. J Clin Oncol (2009) 27(26):4247–53. doi:10.1200/JCO.2009.22.6993
39. Inamura K, Takeuchi K, Togashi Y, Hatano S, Ninomiya H, Motoi N, et al. EML4-ALK lung cancers are characterized by rare other mutations, a TTF-1 cell lineage, an acinar histology, and young onset. Mod Pathol (2009) 22(4):508–15. doi:10.1038/modpathol.2009.2
40. Yoshida A, Kohno T, Tsuta K, Wakai S, Arai Y, Shimada Y, et al. ROS1-rearranged lung cancer: a clinicopathologic and molecular study of 15 surgical cases. Am J Surg Pathol (2013) 37(4):554–62. doi:10.1097/PAS.0b013e3182758fe6
41. Tsuta K, Kohno T, Yoshida A, Shimada Y, Asamura H, Furuta K, et al. RET-rearranged non-small-cell lung carcinoma: a clinicopathological and molecular analysis. Br J Cancer (2014) 110(6):1571–8. doi:10.1038/bjc.2014.36
42. Vaishnavi A, Capelletti M, Le AT, Kako S, Butaney M, Ercan D, et al. Oncogenic and drug-sensitive NTRK1 rearrangements in lung cancer. Nat Med (2013) 19(11):1469–72. doi:10.1038/nm.3352
43. Shim HS, Kenudson M, Zheng Z, Liebers M, Cha YJ, Hoang Ho Q, et al. Unique genetic and survival characteristics of invasive mucinous adenocarcinoma of the lung. J Thorac Oncol (2015) 10(8):1156–62. doi:10.1097/JTO.0000000000000579
44. Fernandez-Cuesta L, Plenker D, Osada H, Sun R, Menon R, Leenders F, et al. CD74-NRG1 fusions in lung adenocarcinoma. Cancer Discov (2014) 4(4):415–22. doi:10.1158/2159-8290.CD-13-0633
45. Nakaoku T, Tsuta K, Ichikawa H, Shiraishi K, Sakamoto H, Enari M, et al. Druggable oncogene fusions in invasive mucinous lung adenocarcinoma. Clin Cancer Res (2014) 20(12):3087–93. doi:10.1158/1078-0432.CCR-14-0107
46. Bartel DP. MicroRNAs: genomics, biogenesis, mechanism, and function. Cell (2004) 116(2):281–97. doi:10.1016/S0092-8674(04)00045-5
47. Lu J, Getz G, Miska EA, Alvarez-Saavedra E, Lamb J, Peck D, et al. MicroRNA expression profiles classify human cancers. Nature (2005) 435(7043):834–8. doi:10.1038/nature03702
48. Inamura K, Ishikawa Y. MicroRNA in lung cancer: novel biomarkers and potential tools for treatment. J Clin Med (2016) 5(3):36. doi:10.3390/jcm5030036
49. Kuninty PR, Schnittert J, Storm G, Prakash J. MicroRNA targeting to modulate tumor microenvironment. Front Oncol (2016) 6:3. doi:10.3389/fonc.2016.00003
50. Jahagirdar D, Purohit S, Jain A, Sharma NK. Export of microRNAs: a bridge between breast carcinoma and their neighboring cells. Front Oncol (2016) 6:147. doi:10.3389/fonc.2016.00147
51. Nadal E, Zhong J, Lin J, Reddy RM, Ramnath N, Orringer MB, et al. A microRNA cluster at 14q32 drives aggressive lung adenocarcinoma. Clin Cancer Res (2014) 20(12):3107–17. doi:10.1158/1078-0432.CCR-13-3348
52. Garajová I, Funel N, Fiorentino M, Agostini V, Ferracin M, Negrini M, et al. MicroRNA profiling of primary pulmonary enteric adenocarcinoma in members from the same family reveals some similarities to pancreatic adenocarcinoma – a step towards personalized therapy. Clin Epigenetics (2015) 7:129. doi:10.1186/s13148-015-0162-5
53. Nishikawa E, Osada H, Okazaki Y, Arima C, Tomida S, Tatematsu Y, et al. miR-375 is activated by ASH1 and inhibits YAP1 in a lineage-dependent manner in lung cancer. Cancer Res (2011) 71(19):6165–73. doi:10.1158/0008-5472.CAN-11-1020
54. Yu Y, Zuo J, Tan Q, Zar Thin K, Li P, Zhu M, et al. Plasma miR-92a-2 as a biomarker for small cell lung cancer. Cancer Biomark (2017) 18(3):319–27. doi:10.3233/CBM-160254
55. Demes M, Aszyk C, Bartsch H, Schirren J, Fisseler-Eckhoff A. Differential miRNA-expression as an adjunctive diagnostic tool in neuroendocrine tumors of the lung. Cancers (Basel) (2016) 8(4):38. doi:10.3390/cancers8040038
Keywords: adenocarcinoma, driver mutation, genetic alteration, histology, molecular pathology, lung cancer
Citation: Inamura K (2017) Lung Cancer: Understanding Its Molecular Pathology and the 2015 WHO Classification. Front. Oncol. 7:193. doi: 10.3389/fonc.2017.00193
Received: 29 March 2017; Accepted: 11 August 2017; Published: 28 August 2017
Reviewed by:
Copyright: © 2017 Inamura. This is an open-access article distributed under the terms of the Creative Commons Attribution License (CC BY) . The use, distribution or reproduction in other forums is permitted, provided the original author(s) or licensor are credited and that the original publication in this journal is cited, in accordance with accepted academic practice. No use, distribution or reproduction is permitted which does not comply with these terms.
*Correspondence: Kentaro Inamura, kentaro.inamura@jfcr.or.jp
Disclaimer: All claims expressed in this article are solely those of the authors and do not necessarily represent those of their affiliated organizations, or those of the publisher, the editors and the reviewers. Any product that may be evaluated in this article or claim that may be made by its manufacturer is not guaranteed or endorsed by the publisher.
Advertisement
Recent advances in lung cancer research: unravelling the future of treatment
- Review Article
- Published: 06 April 2024
- Volume 76 , pages 2129–2140, ( 2024 )
Cite this article
- Luca Bertolaccini ORCID: orcid.org/0000-0002-1153-3334 1 ,
- Monica Casiraghi 1 , 2 ,
- Clarissa Uslenghi 1 ,
- Sebastiano Maiorca 1 &
- Lorenzo Spaggiari 1 , 2
1053 Accesses
3 Citations
1 Altmetric
Explore all metrics
Lung cancer, a multifaceted disease, demands tailored therapeutic approaches due to its diverse subtypes and stages. This comprehensive review explores the intricate landscape of lung cancer research, delving into recent breakthroughs and their implications for diagnosis, therapy, and prevention. Genomic profiling and biomarker identification have ushered in the era of personalised medicine, enabling targeted therapies that minimise harm to healthy tissues while effectively combating cancer cells. The relationship between pulmonary tuberculosis and lung cancer is examined, shedding light on potential mechanisms linking these two conditions. Early detection methods, notably low-dose computed tomography scans, have significantly improved patient outcomes, emphasising the importance of timely interventions. There has been a growing interest in segmentectomy as a surgical intervention for early-stage lung cancer in recent years. Immunotherapy has emerged as a transformative approach, harnessing the body's immune system to recognise and eliminate cancer cells. Combining immunotherapy with traditional treatments, such as chemotherapy and targeted therapies, has shown enhanced efficacy, addressing the disease's heterogeneity and overcoming drug resistance. Precision medicine, guided by genomic profiling, has enabled the development of targeted therapies like tyrosine kinase inhibitors, offering personalised treatments tailored to individual patients. Challenges such as drug resistance and limited accessibility to advanced therapies persist, emphasising the need for collaborative efforts and innovative technologies like artificial intelligence. Despite challenges, ongoing interdisciplinary collaborations and technological advancements offer hope for a future where lung cancer is treatable and preventable, reducing the burden on patients and healthcare systems worldwide.
This is a preview of subscription content, log in via an institution to check access.
Access this article
Subscribe and save.
- Get 10 units per month
- Download Article/Chapter or eBook
- 1 Unit = 1 Article or 1 Chapter
- Cancel anytime
Price includes VAT (Russian Federation)
Instant access to the full article PDF.
Rent this article via DeepDyve
Institutional subscriptions
Similar content being viewed by others
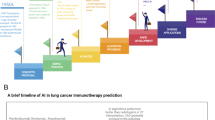
The artificial intelligence and machine learning in lung cancer immunotherapy
Application of clinical bioinformatics in lung cancer-specific biomarkers.
A Personalized Treatment for Lung Cancer: Molecular Pathways, Targeted Therapies, and Genomic Characterization
Data availability.
Not applicable.
Cheng B, Xiong S, Li C, Liang H, Zhao Y, Li J et al (2020) An annual review of the remarkable advances in lung cancer clinical research in 2019. J Thorac Dis 12(3):1056–1069
PubMed Central PubMed Google Scholar
Ibodeng GO, Uche IN, Mokua R, Galo M, Odigwe B, Galeas JN, Dasgupta S (2023) A snapshot of lung cancer: where are we now?-a narrative review. Ann Transl Med 11(6):261
CAS PubMed Central PubMed Google Scholar
Bertolaccini L, Casiraghi M, Petrella F, Rampinelli C, Tessitore A, Spaggiari L (2022) A methodological quality evaluation of the published guidelines and recommendations about the lung cancer screening. Eur J Cancer Prev 31(1):19–25
PubMed Google Scholar
Duma N, Santana-Davila R, Molina JR (2019) Non-small cell lung cancer: epidemiology, screening, diagnosis, and treatment. Mayo Clin Proc 94(8):1623–1640
CAS PubMed Google Scholar
Hwang SY, Kim JY, Lee HS, Lee S, Kim D, Kim S et al (2022) Pulmonary tuberculosis and risk of lung cancer: a systematic review and meta-analysis. J Clin Med 11(3):765
Yaegashi LB, Baldavira CM, Prieto TG, Machado-Rugolo J, Velosa APP, da Silveira LKR et al (2021) In situ overexpression of matricellular mechanical proteins demands functional immune signature and mitigates non-small cell lung cancer progression. Front Immunol 12:714230
Bourgot I, Primac I, Louis T, Noel A, Maquoi E (2020) Reciprocal interplay between fibrillar collagens and collagen-binding integrins: implications in cancer progression and metastasis. Front Oncol 10:1488
Horne ZD, Jack R, Gray ZT, Siegfried JM, Wilson DO, Yousem SA et al (2011) Increased levels of tumor-infiltrating lymphocytes are associated with improved recurrence-free survival in stage 1A non-small-cell lung cancer. J Surg Res 171(1):1–5
Jamal-Hanjani M, Wilson GA, McGranahan N, Birkbak NJ, Watkins TBK, Veeriah S et al (2017) Tracking the evolution of non-small-cell lung cancer. N Engl J Med 376(22):2109–2121
Oliver AL (2022) Lung cancer: epidemiology and screening. Surg Clin North Am 102(3):335–344
Ribas A, Wolchok JD (2018) Cancer immunotherapy using checkpoint blockade. Science 359(6382):1350–1355
Sahin U, Derhovanessian E, Miller M, Kloke BP, Simon P, Lower M et al (2017) Personalized RNA mutanome vaccines mobilize poly-specific therapeutic immunity against cancer. Nature 547(7662):222–226
Maude SL, Frey N, Shaw PA, Aplenc R, Barrett DM, Bunin NJ et al (2014) Chimeric antigen receptor T cells for sustained remissions in leukemia. N Engl J Med 371(16):1507–1517
Franzi S, Mattioni G, Rijavec E, Croci GA, Tosi D (2022) Neoadjuvant chemo-immunotherapy for locally advanced non-small-cell lung cancer: a review of the literature. J Clin Med 11(9):2629
Szeto CH, Shalata W, Yakobson A, Agbarya A (2021) Neoadjuvant and adjuvant immunotherapy in early-stage non-small-cell lung cancer, past, present, and future. J Clin Med 10(23):5614
Chai Y, Wu X, Bai H, Duan J (2022) Combined immunotherapy with chemotherapy versus bevacizumab with chemotherapy in first-line treatment of driver-gene-negative non-squamous non-small cell lung cancer: an updated systematic review and network meta-analysis. J Clin Med 11(6):1655
Schiller JH, Harrington D, Belani CP, Langer C, Sandler A, Krook J et al (2002) Comparison of four chemotherapy regimens for advanced non-small-cell lung cancer. N Engl J Med 346(2):92–98
Gandhi L, Rodriguez-Abreu D, Gadgeel S, Esteban E, Felip E, De Angelis F et al (2018) Pembrolizumab plus chemotherapy in metastatic non-small-cell lung cancer. N Engl J Med 378(22):2078–2092
Tsuboi M, Herbst RS, John T, Kato T, Majem M, Grohe C et al (2023) Overall Survival with Osimertinib in Resected EGFR-mutated NSCLC. N Engl J Med 389(2):137–147
Dohopolski M, Iyengar P (2021) Oligometastatic non-small cell lung cancer: a narrative review of stereotactic ablative radiotherapy. Ann Palliat Med 10(5):5944–5953
Yuan Z, Wang Y, Zhang J, Zheng J, Li W (2019) A meta-analysis of clinical outcomes after radiofrequency ablation and microwave ablation for lung cancer and pulmonary metastases. J Am Coll Radiol 16(3):302–314
Chen Y, Luo H, Liu R, Tan M, Wang Q, Wu X et al (2023) Efficacy and safety of particle therapy for inoperable stage II–III non-small cell lung cancer: a systematic review and meta-analysis. Radiat Oncol 18(1):86
Harada H, Suefuji H, Mori K, Ishikawa H, Nakamura M, Tokumaru S et al (2023) Proton and carbon ion radiotherapy for operable early-stage lung cancer: 3-year results of a prospective nationwide registry. Int J Radiation Oncol Biol Phys 117(2):23
Google Scholar
de Koning HJ, van der Aalst CM, de Jong PA, Scholten ET, Nackaerts K, Heuvelmans MA et al (2020) reduced lung-cancer mortality with volume CT screening in a randomized trial. N Engl J Med 382(6):503–513
Huo B, Manos D, Xu Z, Matheson K, Chun S, Fris J et al (2023) Screening criteria evaluation for expansion in pulmonary neoplasias (SCREEN). Semin Thorac Cardiovasc Surg 35(4):769–780
Passiglia F, Cinquini M, Bertolaccini L, Del Re M, Facchinetti F, Ferrara R et al (2021) Benefits and harms of lung cancer screening by chest computed tomography: a systematic review and meta-analysis. J Clin Oncol 39(23):2574–2585
Qi SA, Wu Q, Chen Z, Zhang W, Zhou Y, Mao K et al (2021) High-resolution metabolomic biomarkers for lung cancer diagnosis and prognosis. Sci Rep 11(1):11805
Madama D, Martins R, Pires AS, Botelho MF, Alves MG, Abrantes AM, Cordeiro CR (2021) Metabolomic profiling in lung cancer: a systematic review. Metabolites 11(9):630
Planchard D, Kim TM, Mazieres J, Quoix E, Riely G, Barlesi F et al (2016) Dabrafenib in patients with BRAF(V600E)-positive advanced non-small-cell lung cancer: a single-arm, multicentre, open-label, phase 2 trial. Lancet Oncol 17(5):642–650
Araujo DC, Veloso AA, Borges KBG, Carvalho MDG (2022) Prognosing the risk of COVID-19 death through a machine learning-based routine blood panel: a retrospective study in Brazil. Int J Med Inform 165:104835
Chiu HY, Chao HS, Chen YM (2022) Application of artificial intelligence in lung cancer. Cancers (Basel) 14(6):1370
Christie JR, Lang P, Zelko LM, Palma DA, Abdelrazek M, Mattonen SA (2021) Artificial intelligence in lung cancer: bridging the gap between computational power and clinical decision-making. Can Assoc Radiol J 72(1):86–97
Goncalves S, Fong PC, Blokhina M (2022) Artificial intelligence for early diagnosis of lung cancer through incidental nodule detection in low- and middle-income countries-acceleration during the COVID-19 pandemic but here to stay. Am J Cancer Res 12(1):1–16
Goldsmith I, Chesterfield-Thomas G, Toghill H (2021) Pre-treatment optimization with pulmonary rehabilitation in lung cancer: making the inoperable patients operable. EClinicalMedicine 31:100663
Shields MD, Chen K, Dutcher G, Patel I, Pellini B (2022) Making the rounds: exploring the role of circulating tumor DNA (ctDNA) in non-small cell lung cancer. Int J Mol Sci 23(16):9006
Abbosh C, Frankell AM, Harrison T, Kisistok J, Garnett A, Johnson L et al (2023) Tracking early lung cancer metastatic dissemination in TRACERx using ctDNA. Nature 616(7957):553–562
Zaman FY, Subramaniam A, Afroz A, Samoon Z, Gough D, Arulananda S, Alamgeer M (2023) Circulating tumour DNA (ctDNA) as a predictor of clinical outcome in non-small cell lung cancer undergoing targeted therapies: a systematic review and meta-analysis. Cancers (Basel) 15(9):2425
Jaffee EM, Dang CV, Agus DB, Alexander BM, Anderson KC, Ashworth A et al (2017) Future cancer research priorities in the USA: a lancet oncology commission. Lancet Oncol 18(11):e653–e706
Saji H, Okada M, Tsuboi M, Nakajima R, Suzuki K, Aokage K et al (2022) Segmentectomy versus lobectomy in small-sized peripheral non-small-cell lung cancer (JCOG0802/WJOG4607L): a multicentre, open-label, phase 3, randomised, controlled, non-inferiority trial. Lancet 399(10335):1607–1617
Nakada T, Noda Y, Kato D, Shibasaki T, Mori S, Asano H et al (2019) Risk factors and cancer recurrence associated with postoperative complications after thoracoscopic lobectomy for clinical stage I non-small cell lung cancer. Thorac Cancer 10(10):1945–1952
Bedetti B, Bertolaccini L, Rocco R, Schmidt J, Solli P, Scarci M (2017) Segmentectomy versus lobectomy for stage I non-small cell lung cancer: a systematic review and meta-analysis. J Thorac Dis 9(6):1615–1623
Bertolaccini L, Prisciandaro E, Bardoni C, Cara A, Diotti C, Girelli L, Spaggiari L (2022) Minimally invasive anatomical segmentectomy versus lobectomy in stage IA non-small cell lung cancer: a systematic review and meta-analysis. Cancers (Basel) 14(24):6157
Wang P, Fu YH, Qi HF, He P, Wang HF, Li C, Liu XC (2023) Evaluation of the efficacy and safety of robot-assisted and video assisted thoracic surgery for early non-small cell lung cancer: a meta-analysis. Technol Health Care 32(2):511–523
Casiraghi M, Galetta D, Borri A, Tessitore A, Romano R, Diotti C et al (2019) Ten years’ experience in robotic-assisted thoracic surgery for early stage lung cancer. Thorac Cardiovasc Surg 67(7):564–572
Wang P, Wang S, Liu Z, Sui X, Wang X, Li X et al (2022) Segmentectomy and wedge resection for elderly patients with stage I non-small cell lung cancer: a systematic review and meta-analysis. J Clin Med 11(2):294
Bertolaccini L, Cara A, Chiari M, Diotti C, Glick N, Mohamed S et al (2023) Real-world survival outcomes of wedge resection versus lobectomy for cT1a/b cN0 cM0 non-small cell lung cancer: a single center retrospective analysis. Front Oncol 13:1226429
Bertolaccini L, Spaggiari L (2023) Is it time to cross the pillars of evidence in favor of segmentectomies in early-stage non-small cell lung cancer? Cancers (Basel) 15(7):1993
Zaraca F, Kirschbaum A, Pipitone MD, Bertolaccini L, Group PS (2023) Prospective randomized study on the efficacy of three-dimensional reconstructions of bronchovascular structures on preoperative chest CT scan in patients who are candidates for pulmonary segmentectomy surgery: the patches (prospective randomized study efficacy of three-dimensional reconstructions segmentecomy) study protocol. Trials 24(1):594
Komarnicki P, Musialkiewicz J, Stanska A, Maciejewski A, Gut P, Mastorakos G, Ruchala M (2022) Circulating neuroendocrine tumor biomarkers: past, present and future. J Clin Med 11(19):5542
Coudray N, Ocampo PS, Sakellaropoulos T, Narula N, Snuderl M, Fenyo D et al (2018) Classification and mutation prediction from non-small cell lung cancer histopathology images using deep learning. Nat Med 24(10):1559–1567
Biesinger M, Eicken N, Varga A, Weber M, Brndiar M, Erd G et al (2022) Lymph but not blood vessel invasion is independent prognostic in lung cancer patients treated by VATS-lobectomy and might represent a future upstaging factor for early stages. Cancers 14(8):1893
Asamura H, Nishimura KK, Giroux DJ, Chansky K, Hoering A, Rusch V, et al (2023) IASLC Lung Cancer Staging Project The New Database to Inform Revisions in the Ninth Edition of the TNM Classification of Lung Cancer. J Thorac Oncol 18(5): 564–575
Hardenberg MC, Patel B, Matthews C, Califano R, Garcia Campelo R, Grohe C et al (2022) The value of disease-free survival (DFS) and osimertinib in adjuvant non-small-cell lung cancer (NSCLC): an international Delphi consensus report. ESMO Open 7(5):100572
Wu YL, Tsuboi M, He J, John T, Grohe C, Majem M et al (2020) Osimertinib in resected EGFR-mutated non-small-cell lung cancer. N Engl J Med 383(18):1711–1723
Xu H, Baidoo AAH, Su S, Ye J, Chen C, Xie Y et al (2019) A comparison of EGFR mutation status in tissue and plasma cell-free DNA detected by ADx-ARMS in advanced lung adenocarcinoma patients. Transl Lung Cancer Res 8(2):135–143
Zou PC, Wang L, Liu B, Zhang HZ, Liu HC (2011) EGFR-targeted therapies combined with chemotherapy for treating advanced non-small-cell lung cancer: a meta-analysis. Diagnostics 9:38
Solomon BJ, Bauer TM, Mok TSK, Liu G, Mazieres J, de Marinis F et al (2023) Efficacy and safety of first-line lorlatinib versus crizotinib in patients with advanced, ALK-positive non-small-cell lung cancer: updated analysis of data from the phase 3, randomised, open-label CROWN study. Lancet Respir Med 11(4):354–366
Hotta K, Hida T, Nokihara H, Morise M, Kim YH, Azuma K et al (2022) Final overall survival analysis from the phase III J-ALEX study of alectinib versus crizotinib in ALK inhibitor-naive Japanese patients with ALK-positive non-small-cell lung cancer. ESMO Open 7(4):100527
Shaw AT, Bauer TM, de Marinis F, Felip E, Goto Y, Liu G et al (2020) First-line lorlatinib or crizotinib in advanced ALK-positive lung cancer. N Engl J Med 383(21):2018–2029
Herbst RS, Baas P, Kim DW, Felip E, Perez-Gracia JL, Han JY et al (2016) Pembrolizumab versus docetaxel for previously treated, PD-L1-positive, advanced non-small-cell lung cancer (KEYNOTE-010): a randomised controlled trial. Lancet 387(10027):1540–1550
Isaacs J, Stinchcombe TE (2022) Neoadjuvant and adjuvant systemic therapy for early-stage non-small-cell lung cancer. Drugs 82(8):855–863
John AO, Ramnath N (2023) Neoadjuvant versus adjuvant systemic therapy for early-stage non-small cell lung cancer: the changing landscape due to immunotherapy. Oncologist 28(9):752–764
Wakelee H, Liberman M, Kato T, Tsuboi M, Lee SH, Gao S et al (2023) Perioperative pembrolizumab for early-stage non-small-cell lung cancer. N Engl J Med 389(6):491–503
Kogure Y, Hashimoto H, Oki M (2021) A randomized phase iii study of pembrolizumab versus pembrolizumab-carboplatin-pemetrexed for locally advanced or metastatic nonsquamous non-small-cell lung cancer with PD-L1 50% or more (LAPLACE-50): study protocol. Clin Lung Cancer 11:921–924
Download references
Acknowledgements
This work was partially supported by the Italian Ministry of Health with Ricerca Corrente and 5x1000 funds.
Ministero della Salute, 5 × 1000, Ricerca Corrente.
Author information
Authors and affiliations.
Department of Thoracic Surgery, IEO, European Institute of Oncology IRCCS, Via Ripamonti 435, 20141, Milan, Italy
Luca Bertolaccini, Monica Casiraghi, Clarissa Uslenghi, Sebastiano Maiorca & Lorenzo Spaggiari
Department of Oncology and Hemato-Oncology, University of Milan, Milan, Italy
Monica Casiraghi & Lorenzo Spaggiari
You can also search for this author in PubMed Google Scholar
Corresponding author
Correspondence to Luca Bertolaccini .
Additional information
Publisher's note.
Springer Nature remains neutral with regard to jurisdictional claims in published maps and institutional affiliations.
Rights and permissions
Springer Nature or its licensor (e.g. a society or other partner) holds exclusive rights to this article under a publishing agreement with the author(s) or other rightsholder(s); author self-archiving of the accepted manuscript version of this article is solely governed by the terms of such publishing agreement and applicable law.
Reprints and permissions
About this article
Bertolaccini, L., Casiraghi, M., Uslenghi, C. et al. Recent advances in lung cancer research: unravelling the future of treatment. Updates Surg 76 , 2129–2140 (2024). https://doi.org/10.1007/s13304-024-01841-3
Download citation
Received : 06 March 2024
Accepted : 24 March 2024
Published : 06 April 2024
Issue Date : October 2024
DOI : https://doi.org/10.1007/s13304-024-01841-3
Share this article
Anyone you share the following link with will be able to read this content:
Sorry, a shareable link is not currently available for this article.
Provided by the Springer Nature SharedIt content-sharing initiative
- Lung cancer
- Comprehensive review
- Find a journal
- Publish with us
- Track your research
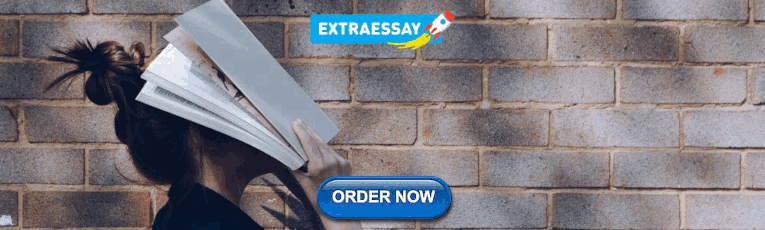
IMAGES
VIDEO
COMMENTS
Lung cancer is one of the most frequently diagnosed cancers and the leading cause of cancer-related deaths worldwide with an estimated 2 million new cases and 1·76 million deaths per year. Substantial improvements in our understanding of disease biology, application of predictive biomarkers, and refinements in treatment have led to remarkable progress in the past two decades and transformed ...
Posted: February 16, 2024. The results of the clinical trial that led to FDA’s 2023 approval of repotrectinib (Augtyro) for lung cancers with ROS1 fusions have been published. The drug shrank tumors in 80% of people receiving the drug as an initial treatment. The ALCHEMIST Lung Cancer Trials.
Introduction. Lung cancer or bronchogenic carcinoma refers to tumors originating in the lung parenchyma or within the bronchi. It is one of the leading causes of cancer-related deaths in the United States. Since 1987, lung cancer has been responsible for more deaths in women than breast cancer. It is estimated that there are 225,000 new cases ...
Lung cancer continues to be the leading cause of cancer death worldwide, accounting for about 18% of all cancer deaths [2]. The highest incidence rates of lung cancer are observed in parts of North America, in East Asia, and in parts of central and eastern Europe (Fig. 5.1.1) [2]. Incidence rates in men have declined during the past four ...
The aim of this review is to provide an up-to-date summary of lung cancer studies in 2019 and to present the remarkable progress made in lung cancer clinical research. Methods A systemic search of PubMed and Web of Science was conducted to find studies or conference abstracts published in English in the period between January 1, 2019 and ...
MeSH terms. Lung cancer is one of the most frequently diagnosed cancers and the leading cause of cancer-related deaths worldwide with an estimated 2 million new cases and 1·76 million deaths per year. Substantial improvements in our understanding of disease biology, application of predictive biomarkers, and ref ….
Introduction. Lung cancer is the leading cause of cancer-related deaths worldwide in both men and women ().It is categorized into two main histological groups: small cell lung carcinoma (SCLC, 15% of all lung cancers) and non-SCLC (NSCLC, 85% of all lung cancers).
Introduction. Lung cancer is one of the most prevalent and deadly diseases globally, necessitating continuous research and exploration of innovative treatment strategies. In recent years, lung cancer research has witnessed remarkable advancements, revolutionising the approaches to diagnosis, therapy, and prevention [1, 2]. This manuscript ...
Lung cancer persists as the main cause of cancer death in people globally, driven by the ongoing tobacco epidemic and the emergence of risk factors such as ionising radiation exposure and ambient pollution. Nearly 2 million people die of lung cancer each year, a far higher death rate than other common cancer types. However, developments over the past 10 years have dramatically improved the ...
1 Introduction. Lung cancer refers to carcinomas that originate from the respiratory epithelium, mainly in bronchi, bronchioles, and alveoli. Worldwide, it remains the leading cause of mortality in men and the second most common cause of death in women [1–3]. Lung cancer has a poor prognosis and is mostly detected at advanced stages.