
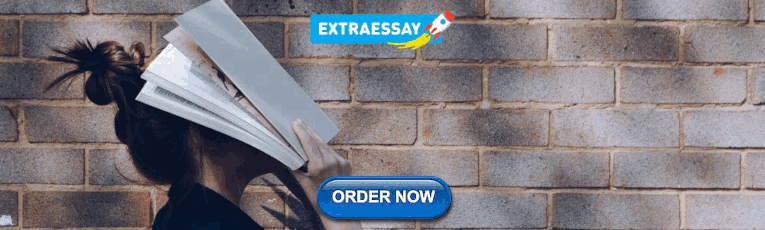
What Is the Theme of a Research Paper?
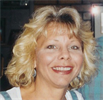
How to Write a Motif Paper
The term "theme" is a small word, but it can intimidate students when they see it on an assignment or test. To overcome the fear and develop confidence, especially with regard to research papers, understand what the word means and see the parallels with any work, including poems, essays, plays, novels and movies.
“Theme” Defined
A theme is a major and sometimes recurring idea, subject or topic that appears in a written work. A dominant theme usually reveals what the work is really about and can be helpful in forming insights and analysis. A theme can consist of one word, two words or more. For example, your teacher might ask you to explore the straightforward ideas of “anger” or “selfishness” or more complex themes of “emotional intelligence” or “conflicted emotions.” Either way, careful reading of the work is vital so that you can marshal examples of where the theme was apparent.
Examples in Research
Themes in research papers might require a little digging, but they are there. Sometimes they are easier to spot when several research papers on the same subject are compared or contrasted, for this is when such subtext emerges. For example, three research papers on the subject of avid TV viewing by teenagers might contain different themes, such as simpler ideas including “passivity” or "grades" or a more complex theme, such as “effects on familial relationships.”
Seize the Opportunity
Once you've identified the theme of a research paper or papers, seize the opportunity and analyze it. Say that you like the idea of exploring how avid TV viewing -- more than four hours per day -- affects teens' grades. Further, suppose that researchers are in general agreement about the correlation but cast a wide net in terms of how they define “passivity.” You might set up a thematic segue for a research paper by saying, “Researchers continue to debate how to define passivity in teens and reach across the spectrum to include the number of hours per day they spend in solitude, the number of people they count as close friends and their lack of interest in hobbies and extracurricular activities.” Then you would take each of these ideas and expound in greater detail.
Related Articles
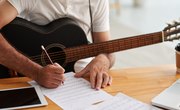
What Is the Theme for a Ballad?
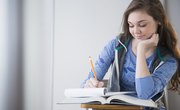
What Is a Theme Statement?
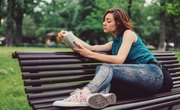
What Are Literal & Inferential Questions?
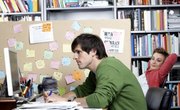
Tips on Writing an Explication Paper on a Short Story
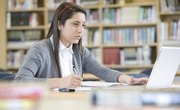
How to Write a Comparative Analytical Essay
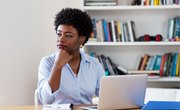
What Does Abstract Thinking Mean?
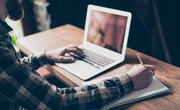
The Functions of Conjunctions in English Argumentative Writing
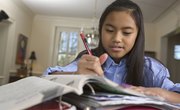
What Is a Descriptive Paragraph?
- The Scott, Foresman Handbook for Writers; Maxine Hairston and John Ruszkiewicz.
- The New St. Martin’s Handbook; Andrea Lunsford and Robert Connors.
- Purdue University: Online Writing Lab: Writing in Literature: Writing the Prompt Paper
- Queens College: Research Papers
With education, health care and small business marketing as her core interests, M.T. Wroblewski has penned pieces for Woman's Day, Family Circle, Ladies Home Journal and many newspapers and magazines. She holds a master's degree in journalism from Northern Illinois University.

How To Write A Research Paper
Step-By-Step Tutorial With Examples + FREE Template
By: Derek Jansen (MBA) | Expert Reviewer: Dr Eunice Rautenbach | March 2024
For many students, crafting a strong research paper from scratch can feel like a daunting task – and rightly so! In this post, we’ll unpack what a research paper is, what it needs to do , and how to write one – in three easy steps. 🙂
Overview: Writing A Research Paper
What (exactly) is a research paper.
- How to write a research paper
- Stage 1 : Topic & literature search
- Stage 2 : Structure & outline
- Stage 3 : Iterative writing
- Key takeaways
Let’s start by asking the most important question, “ What is a research paper? ”.
Simply put, a research paper is a scholarly written work where the writer (that’s you!) answers a specific question (this is called a research question ) through evidence-based arguments . Evidence-based is the keyword here. In other words, a research paper is different from an essay or other writing assignments that draw from the writer’s personal opinions or experiences. With a research paper, it’s all about building your arguments based on evidence (we’ll talk more about that evidence a little later).
Now, it’s worth noting that there are many different types of research papers , including analytical papers (the type I just described), argumentative papers, and interpretative papers. Here, we’ll focus on analytical papers , as these are some of the most common – but if you’re keen to learn about other types of research papers, be sure to check out the rest of the blog .
With that basic foundation laid, let’s get down to business and look at how to write a research paper .
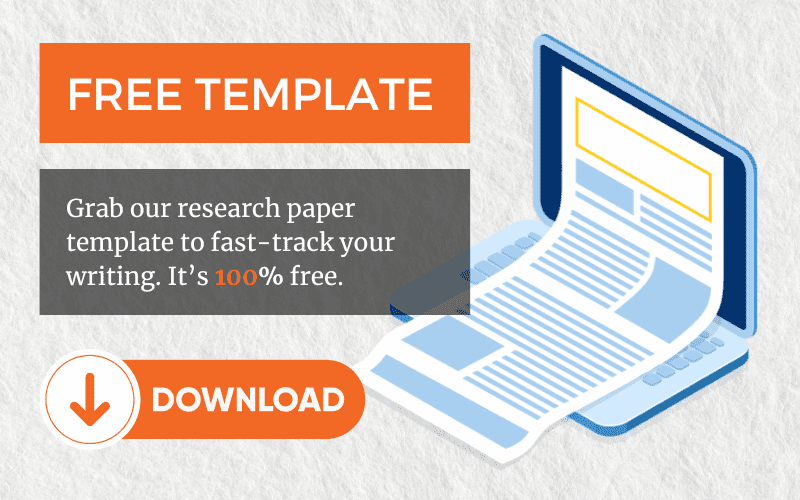
Overview: The 3-Stage Process
While there are, of course, many potential approaches you can take to write a research paper, there are typically three stages to the writing process. So, in this tutorial, we’ll present a straightforward three-step process that we use when working with students at Grad Coach.
These three steps are:
- Finding a research topic and reviewing the existing literature
- Developing a provisional structure and outline for your paper, and
- Writing up your initial draft and then refining it iteratively
Let’s dig into each of these.
Need a helping hand?
Step 1: Find a topic and review the literature
As we mentioned earlier, in a research paper, you, as the researcher, will try to answer a question . More specifically, that’s called a research question , and it sets the direction of your entire paper. What’s important to understand though is that you’ll need to answer that research question with the help of high-quality sources – for example, journal articles, government reports, case studies, and so on. We’ll circle back to this in a minute.
The first stage of the research process is deciding on what your research question will be and then reviewing the existing literature (in other words, past studies and papers) to see what they say about that specific research question. In some cases, your professor may provide you with a predetermined research question (or set of questions). However, in many cases, you’ll need to find your own research question within a certain topic area.
Finding a strong research question hinges on identifying a meaningful research gap – in other words, an area that’s lacking in existing research. There’s a lot to unpack here, so if you wanna learn more, check out the plain-language explainer video below.
Once you’ve figured out which question (or questions) you’ll attempt to answer in your research paper, you’ll need to do a deep dive into the existing literature – this is called a “ literature search ”. Again, there are many ways to go about this, but your most likely starting point will be Google Scholar .
If you’re new to Google Scholar, think of it as Google for the academic world. You can start by simply entering a few different keywords that are relevant to your research question and it will then present a host of articles for you to review. What you want to pay close attention to here is the number of citations for each paper – the more citations a paper has, the more credible it is (generally speaking – there are some exceptions, of course).
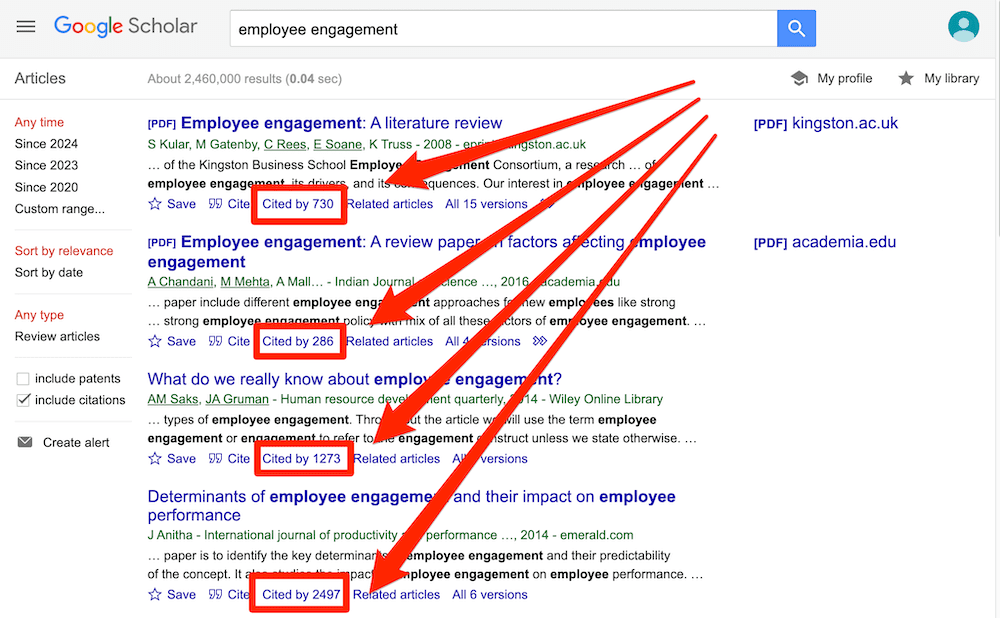
Ideally, what you’re looking for are well-cited papers that are highly relevant to your topic. That said, keep in mind that citations are a cumulative metric , so older papers will often have more citations than newer papers – just because they’ve been around for longer. So, don’t fixate on this metric in isolation – relevance and recency are also very important.
Beyond Google Scholar, you’ll also definitely want to check out academic databases and aggregators such as Science Direct, PubMed, JStor and so on. These will often overlap with the results that you find in Google Scholar, but they can also reveal some hidden gems – so, be sure to check them out.
Once you’ve worked your way through all the literature, you’ll want to catalogue all this information in some sort of spreadsheet so that you can easily recall who said what, when and within what context. If you’d like, we’ve got a free literature spreadsheet that helps you do exactly that.
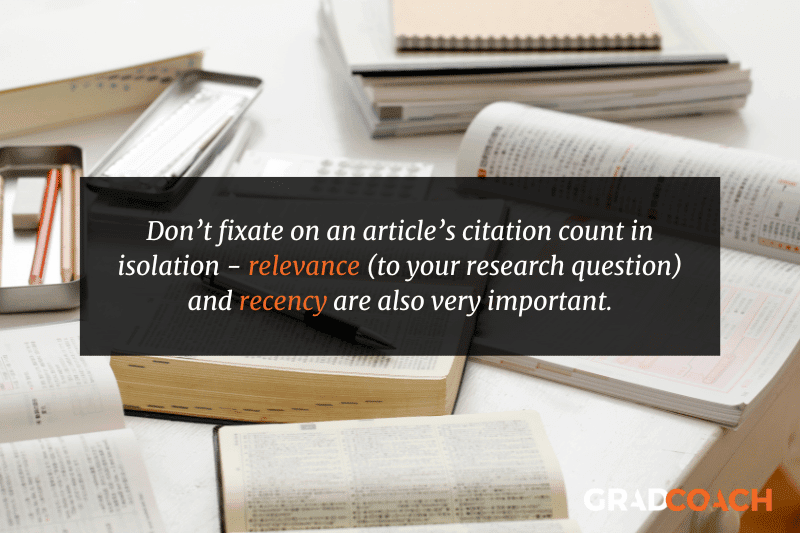
Step 2: Develop a structure and outline
With your research question pinned down and your literature digested and catalogued, it’s time to move on to planning your actual research paper .
It might sound obvious, but it’s really important to have some sort of rough outline in place before you start writing your paper. So often, we see students eagerly rushing into the writing phase, only to land up with a disjointed research paper that rambles on in multiple
Now, the secret here is to not get caught up in the fine details . Realistically, all you need at this stage is a bullet-point list that describes (in broad strokes) what you’ll discuss and in what order. It’s also useful to remember that you’re not glued to this outline – in all likelihood, you’ll chop and change some sections once you start writing, and that’s perfectly okay. What’s important is that you have some sort of roadmap in place from the start.
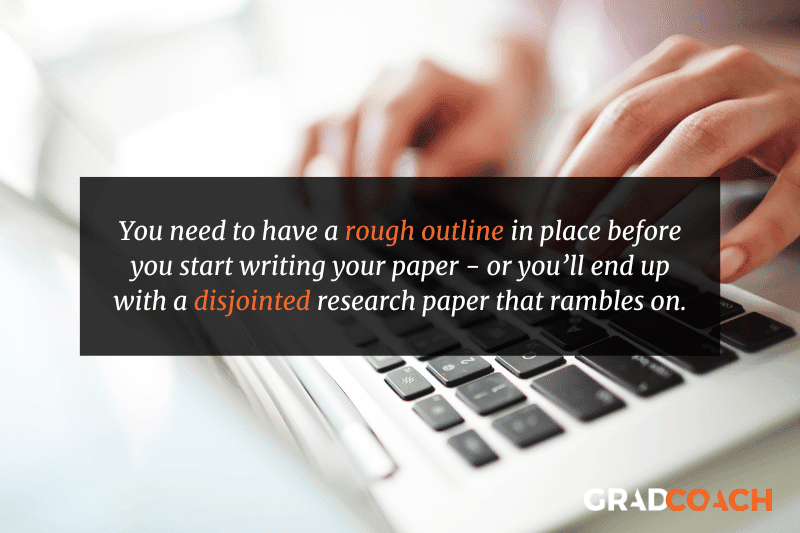
At this stage you might be wondering, “ But how should I structure my research paper? ”. Well, there’s no one-size-fits-all solution here, but in general, a research paper will consist of a few relatively standardised components:
- Introduction
- Literature review
- Methodology
Let’s take a look at each of these.
First up is the introduction section . As the name suggests, the purpose of the introduction is to set the scene for your research paper. There are usually (at least) four ingredients that go into this section – these are the background to the topic, the research problem and resultant research question , and the justification or rationale. If you’re interested, the video below unpacks the introduction section in more detail.
The next section of your research paper will typically be your literature review . Remember all that literature you worked through earlier? Well, this is where you’ll present your interpretation of all that content . You’ll do this by writing about recent trends, developments, and arguments within the literature – but more specifically, those that are relevant to your research question . The literature review can oftentimes seem a little daunting, even to seasoned researchers, so be sure to check out our extensive collection of literature review content here .
With the introduction and lit review out of the way, the next section of your paper is the research methodology . In a nutshell, the methodology section should describe to your reader what you did (beyond just reviewing the existing literature) to answer your research question. For example, what data did you collect, how did you collect that data, how did you analyse that data and so on? For each choice, you’ll also need to justify why you chose to do it that way, and what the strengths and weaknesses of your approach were.
Now, it’s worth mentioning that for some research papers, this aspect of the project may be a lot simpler . For example, you may only need to draw on secondary sources (in other words, existing data sets). In some cases, you may just be asked to draw your conclusions from the literature search itself (in other words, there may be no data analysis at all). But, if you are required to collect and analyse data, you’ll need to pay a lot of attention to the methodology section. The video below provides an example of what the methodology section might look like.
By this stage of your paper, you will have explained what your research question is, what the existing literature has to say about that question, and how you analysed additional data to try to answer your question. So, the natural next step is to present your analysis of that data . This section is usually called the “results” or “analysis” section and this is where you’ll showcase your findings.
Depending on your school’s requirements, you may need to present and interpret the data in one section – or you might split the presentation and the interpretation into two sections. In the latter case, your “results” section will just describe the data, and the “discussion” is where you’ll interpret that data and explicitly link your analysis back to your research question. If you’re not sure which approach to take, check in with your professor or take a look at past papers to see what the norms are for your programme.
Alright – once you’ve presented and discussed your results, it’s time to wrap it up . This usually takes the form of the “ conclusion ” section. In the conclusion, you’ll need to highlight the key takeaways from your study and close the loop by explicitly answering your research question. Again, the exact requirements here will vary depending on your programme (and you may not even need a conclusion section at all) – so be sure to check with your professor if you’re unsure.
Step 3: Write and refine
Finally, it’s time to get writing. All too often though, students hit a brick wall right about here… So, how do you avoid this happening to you?
Well, there’s a lot to be said when it comes to writing a research paper (or any sort of academic piece), but we’ll share three practical tips to help you get started.
First and foremost , it’s essential to approach your writing as an iterative process. In other words, you need to start with a really messy first draft and then polish it over multiple rounds of editing. Don’t waste your time trying to write a perfect research paper in one go. Instead, take the pressure off yourself by adopting an iterative approach.
Secondly , it’s important to always lean towards critical writing , rather than descriptive writing. What does this mean? Well, at the simplest level, descriptive writing focuses on the “ what ”, while critical writing digs into the “ so what ” – in other words, the implications. If you’re not familiar with these two types of writing, don’t worry! You can find a plain-language explanation here.
Last but not least, you’ll need to get your referencing right. Specifically, you’ll need to provide credible, correctly formatted citations for the statements you make. We see students making referencing mistakes all the time and it costs them dearly. The good news is that you can easily avoid this by using a simple reference manager . If you don’t have one, check out our video about Mendeley, an easy (and free) reference management tool that you can start using today.
Recap: Key Takeaways
We’ve covered a lot of ground here. To recap, the three steps to writing a high-quality research paper are:
- To choose a research question and review the literature
- To plan your paper structure and draft an outline
- To take an iterative approach to writing, focusing on critical writing and strong referencing
Remember, this is just a b ig-picture overview of the research paper development process and there’s a lot more nuance to unpack. So, be sure to grab a copy of our free research paper template to learn more about how to write a research paper.
You Might Also Like:
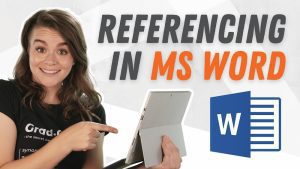
Submit a Comment Cancel reply
Your email address will not be published. Required fields are marked *
Save my name, email, and website in this browser for the next time I comment.
- Print Friendly
Have a language expert improve your writing
Run a free plagiarism check in 10 minutes, automatically generate references for free.
- Knowledge Base
- Methodology
- How to Do Thematic Analysis | Guide & Examples
How to Do Thematic Analysis | Guide & Examples
Published on 5 May 2022 by Jack Caulfield .
Thematic analysis is a method of analysing qualitative data . It is usually applied to a set of texts, such as an interview or transcripts . The researcher closely examines the data to identify common themes, topics, ideas and patterns of meaning that come up repeatedly.
There are various approaches to conducting thematic analysis, but the most common form follows a six-step process:
- Familiarisation
- Generating themes
- Reviewing themes
- Defining and naming themes
This process was originally developed for psychology research by Virginia Braun and Victoria Clarke . However, thematic analysis is a flexible method that can be adapted to many different kinds of research.
Table of contents
When to use thematic analysis, different approaches to thematic analysis, step 1: familiarisation, step 2: coding, step 3: generating themes, step 4: reviewing themes, step 5: defining and naming themes, step 6: writing up.
Thematic analysis is a good approach to research where you’re trying to find out something about people’s views, opinions, knowledge, experiences, or values from a set of qualitative data – for example, interview transcripts , social media profiles, or survey responses .
Some types of research questions you might use thematic analysis to answer:
- How do patients perceive doctors in a hospital setting?
- What are young women’s experiences on dating sites?
- What are non-experts’ ideas and opinions about climate change?
- How is gender constructed in secondary school history teaching?
To answer any of these questions, you would collect data from a group of relevant participants and then analyse it. Thematic analysis allows you a lot of flexibility in interpreting the data, and allows you to approach large datasets more easily by sorting them into broad themes.
However, it also involves the risk of missing nuances in the data. Thematic analysis is often quite subjective and relies on the researcher’s judgement, so you have to reflect carefully on your own choices and interpretations.
Pay close attention to the data to ensure that you’re not picking up on things that are not there – or obscuring things that are.
Prevent plagiarism, run a free check.
Once you’ve decided to use thematic analysis, there are different approaches to consider.
There’s the distinction between inductive and deductive approaches:
- An inductive approach involves allowing the data to determine your themes.
- A deductive approach involves coming to the data with some preconceived themes you expect to find reflected there, based on theory or existing knowledge.
There’s also the distinction between a semantic and a latent approach:
- A semantic approach involves analysing the explicit content of the data.
- A latent approach involves reading into the subtext and assumptions underlying the data.
After you’ve decided thematic analysis is the right method for analysing your data, and you’ve thought about the approach you’re going to take, you can follow the six steps developed by Braun and Clarke .
The first step is to get to know our data. It’s important to get a thorough overview of all the data we collected before we start analysing individual items.
This might involve transcribing audio , reading through the text and taking initial notes, and generally looking through the data to get familiar with it.
Next up, we need to code the data. Coding means highlighting sections of our text – usually phrases or sentences – and coming up with shorthand labels or ‘codes’ to describe their content.
Let’s take a short example text. Say we’re researching perceptions of climate change among conservative voters aged 50 and up, and we have collected data through a series of interviews. An extract from one interview looks like this:
In this extract, we’ve highlighted various phrases in different colours corresponding to different codes. Each code describes the idea or feeling expressed in that part of the text.
At this stage, we want to be thorough: we go through the transcript of every interview and highlight everything that jumps out as relevant or potentially interesting. As well as highlighting all the phrases and sentences that match these codes, we can keep adding new codes as we go through the text.
After we’ve been through the text, we collate together all the data into groups identified by code. These codes allow us to gain a condensed overview of the main points and common meanings that recur throughout the data.
Next, we look over the codes we’ve created, identify patterns among them, and start coming up with themes.
Themes are generally broader than codes. Most of the time, you’ll combine several codes into a single theme. In our example, we might start combining codes into themes like this:
At this stage, we might decide that some of our codes are too vague or not relevant enough (for example, because they don’t appear very often in the data), so they can be discarded.
Other codes might become themes in their own right. In our example, we decided that the code ‘uncertainty’ made sense as a theme, with some other codes incorporated into it.
Again, what we decide will vary according to what we’re trying to find out. We want to create potential themes that tell us something helpful about the data for our purposes.
Now we have to make sure that our themes are useful and accurate representations of the data. Here, we return to the dataset and compare our themes against it. Are we missing anything? Are these themes really present in the data? What can we change to make our themes work better?
If we encounter problems with our themes, we might split them up, combine them, discard them, or create new ones: whatever makes them more useful and accurate.
For example, we might decide upon looking through the data that ‘changing terminology’ fits better under the ‘uncertainty’ theme than under ‘distrust of experts’, since the data labelled with this code involves confusion, not necessarily distrust.
Now that you have a final list of themes, it’s time to name and define each of them.
Defining themes involves formulating exactly what we mean by each theme and figuring out how it helps us understand the data.
Naming themes involves coming up with a succinct and easily understandable name for each theme.
For example, we might look at ‘distrust of experts’ and determine exactly who we mean by ‘experts’ in this theme. We might decide that a better name for the theme is ‘distrust of authority’ or ‘conspiracy thinking’.
Finally, we’ll write up our analysis of the data. Like all academic texts, writing up a thematic analysis requires an introduction to establish our research question, aims, and approach.
We should also include a methodology section, describing how we collected the data (e.g., through semi-structured interviews or open-ended survey questions ) and explaining how we conducted the thematic analysis itself.
The results or findings section usually addresses each theme in turn. We describe how often the themes come up and what they mean, including examples from the data as evidence. Finally, our conclusion explains the main takeaways and shows how the analysis has answered our research question.
In our example, we might argue that conspiracy thinking about climate change is widespread among older conservative voters, point out the uncertainty with which many voters view the issue, and discuss the role of misinformation in respondents’ perceptions.
Cite this Scribbr article
If you want to cite this source, you can copy and paste the citation or click the ‘Cite this Scribbr article’ button to automatically add the citation to our free Reference Generator.
Caulfield, J. (2022, May 05). How to Do Thematic Analysis | Guide & Examples. Scribbr. Retrieved 6 May 2024, from https://www.scribbr.co.uk/research-methods/thematic-analysis-explained/
Is this article helpful?
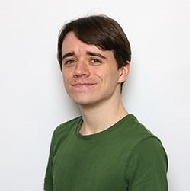
Jack Caulfield
Other students also liked, qualitative vs quantitative research | examples & methods, inductive reasoning | types, examples, explanation, what is deductive reasoning | explanation & examples.
- How it works
Thematic Analysis – A Guide with Examples
Published by Alvin Nicolas at August 16th, 2021 , Revised On August 29, 2023
Thematic analysis is one of the most important types of analysis used for qualitative data . When researchers have to analyse audio or video transcripts, they give preference to thematic analysis. A researcher needs to look keenly at the content to identify the context and the message conveyed by the speaker.
Moreover, with the help of this analysis, data can be simplified.
Importance of Thematic Analysis
Thematic analysis has so many unique and dynamic features, some of which are given below:
Thematic analysis is used because:
- It is flexible.
- It is best for complex data sets.
- It is applied to qualitative data sets.
- It takes less complexity compared to other theories of analysis.
Intellectuals and researchers give preference to thematic analysis due to its effectiveness in the research.
How to Conduct a Thematic Analysis?
While doing any research , if your data and procedure are clear, it will be easier for your reader to understand how you concluded the results . This will add much clarity to your research.
Understand the Data
This is the first step of your thematic analysis. At this stage, you have to understand the data set. You need to read the entire data instead of reading the small portion. If you do not have the data in the textual form, you have to transcribe it.
Example: If you are visiting an adult dating website, you have to make a data corpus. You should read and re-read the data and consider several profiles. It will give you an idea of how adults represent themselves on dating sites. You may get the following results:
I am a tall, single(widowed), easy-going, honest, good listener with a good sense of humor. Being a handyperson, I keep busy working around the house, and I also like to follow my favourite hockey team on TV or spoil my two granddaughters when I get the chance!! Enjoy most music except Rap! I keep fit by jogging, walking, and bicycling (at least three times a week). I have travelled to many places and RVD the South-West U.S., but I would now like to find that special travel partner to do more travel to warm and interesting countries. I now feel it’s time to meet a nice, kind, honest woman who has some of the same interests as I do; to share the happy times, quiet times, and adventures together
I enjoy photography, lapidary & seeking collectibles in the form of classic movies & 33 1/3, 45 & 78 RPM recordings from the 1920s, ’30s & ’40s. I am retired & looking forward to travelling to Canada, the USA, the UK & Europe, China. I am unique since I do not judge a book by its cover. I accept people for who they are. I will not demand or request perfection from anyone until I am perfect, so I guess that means everyone is safe. My musical tastes range from Classical, big band era, early jazz, classic ’50s & 60’s rock & roll & country since its inception.
Development of Initial Coding:
At this stage, you have to do coding. It’s the essential step of your research . Here you have two options for coding. Either you can do the coding manually or take the help of any tool. A software named the NOVIC is considered the best tool for doing automatic coding.
For manual coding, you can follow the steps given below:
- Please write down the data in a proper format so that it can be easier to proceed.
- Use a highlighter to highlight all the essential points from data.
- Make as many points as possible.
- Take notes very carefully at this stage.
- Apply themes as much possible.
- Now check out the themes of the same pattern or concept.
- Turn all the same themes into the single one.
Example: For better understanding, the previously explained example of Step 1 is continued here. You can observe the coded profiles below:
Make Themes
At this stage, you have to make the themes. These themes should be categorised based on the codes. All the codes which have previously been generated should be turned into themes. Moreover, with the help of the codes, some themes and sub-themes can also be created. This process is usually done with the help of visuals so that a reader can take an in-depth look at first glance itself.
Extracted Data Review
Now you have to take an in-depth look at all the awarded themes again. You have to check whether all the given themes are organised properly or not. It would help if you were careful and focused because you have to note down the symmetry here. If you find that all the themes are not coherent, you can revise them. You can also reshape the data so that there will be symmetry between the themes and dataset here.
For better understanding, a mind-mapping example is given here:
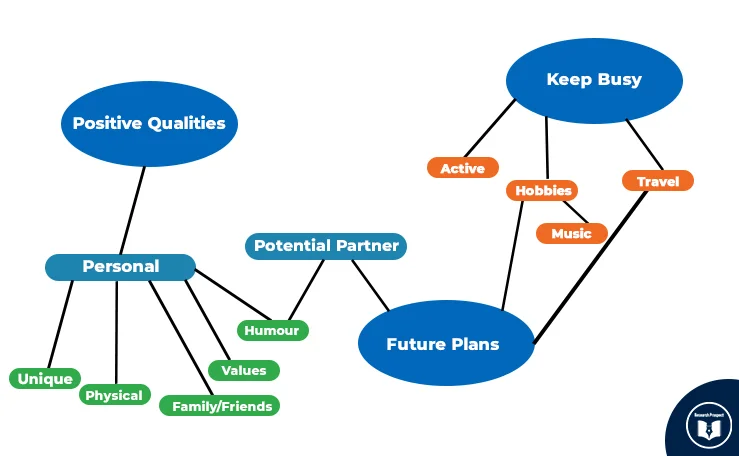
Reviewing all the Themes Again
You need to review the themes after coding them. At this stage, you are allowed to play with your themes in a more detailed manner. You have to convert the bigger themes into smaller themes here. If you want to combine some similar themes into a single theme, then you can do it. This step involves two steps for better fragmentation.
You need to observe the coded data separately so that you can have a precise view. If you find that the themes which are given are following the dataset, it’s okay. Otherwise, you may have to rearrange the data again to coherence in the coded data.
Corpus Data
Here you have to take into consideration all the corpus data again. It would help if you found how themes are arranged here. It would help if you used the visuals to check out the relationship between them. Suppose all the things are not done accordingly, so you should check out the previous steps for a refined process. Otherwise, you can move to the next step. However, make sure that all the themes are satisfactory and you are not confused.
When all the two steps are completed, you need to make a more précised mind map. An example following the previous cases has been given below:
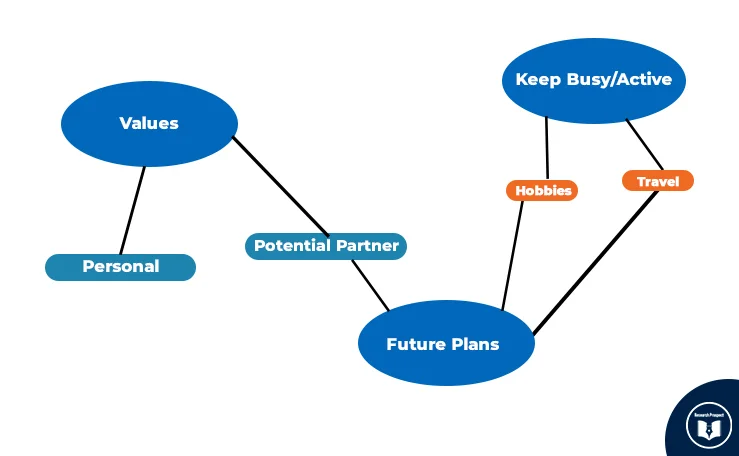
Define all the Themes here
Now you have to define all the themes which you have given to your data set. You can recheck them carefully if you feel that some of them can fit into one concept, you can keep them, and eliminate the other irrelevant themes. Because it should be precise and clear, there should not be any ambiguity. Now you have to think about the main idea and check out that all the given themes are parallel to your main idea or not. This can change the concept for you.
The given names should be so that it can give any reader a clear idea about your findings. However, it should not oppose your thematic analysis; rather, everything should be organised accurately.
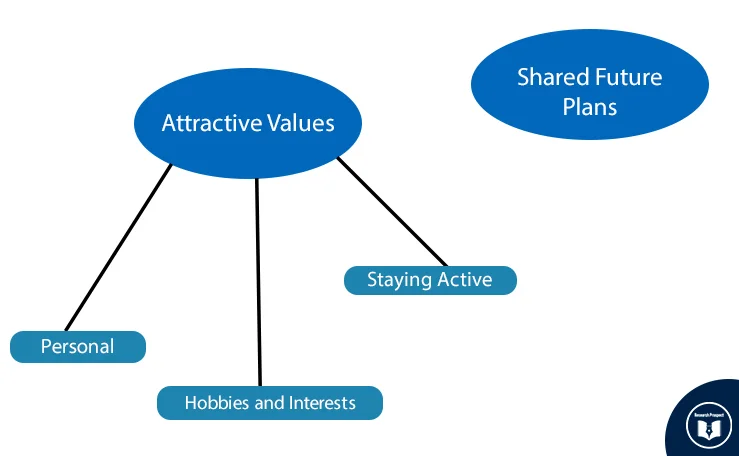
Does your Research Methodology Have the Following?
- Great Research/Sources
- Perfect Language
- Accurate Sources
If not, we can help. Our panel of experts makes sure to keep the 3 pillars of Research Methodology strong.
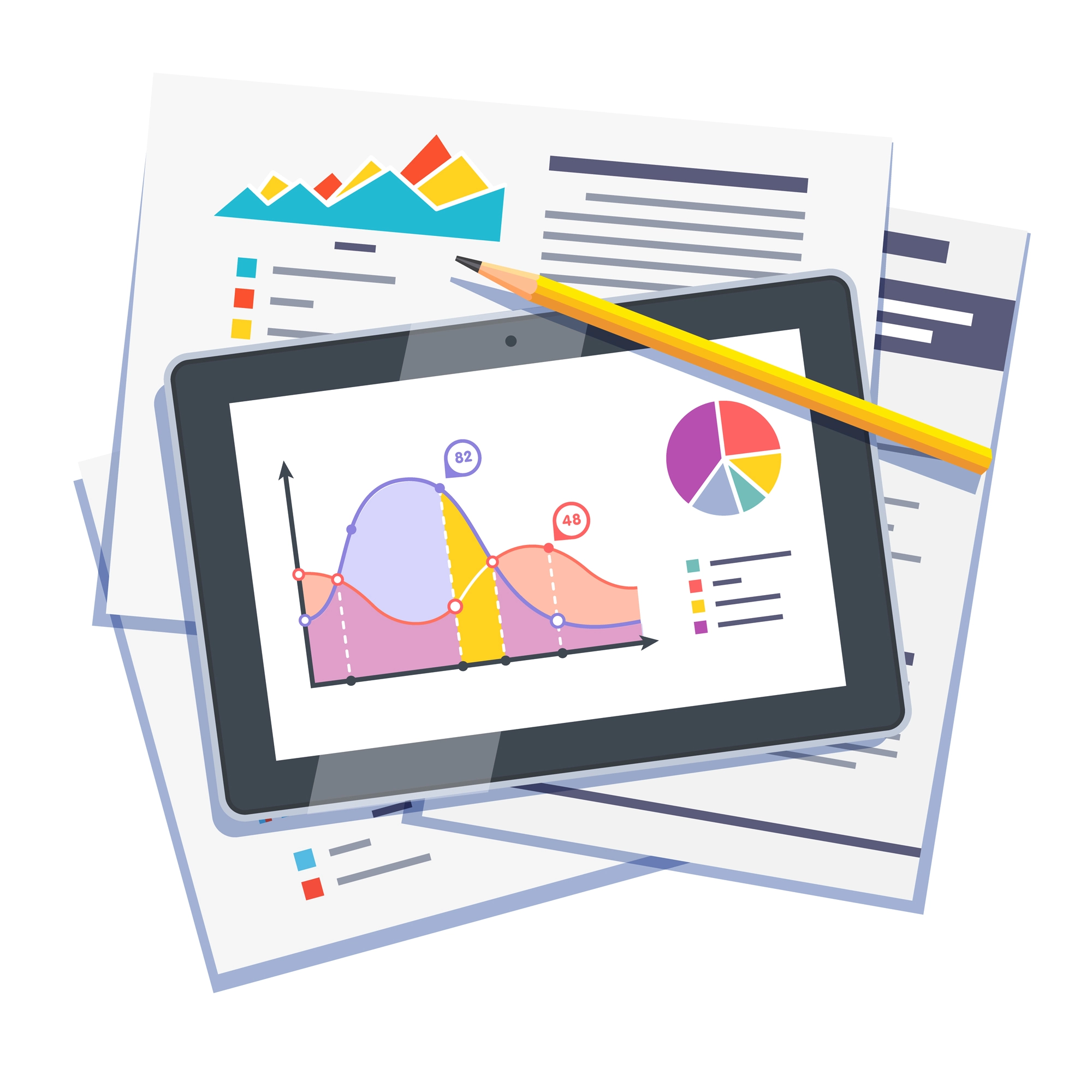
Also, read about discourse analysis , content analysis and survey conducting . we have provided comprehensive guides.
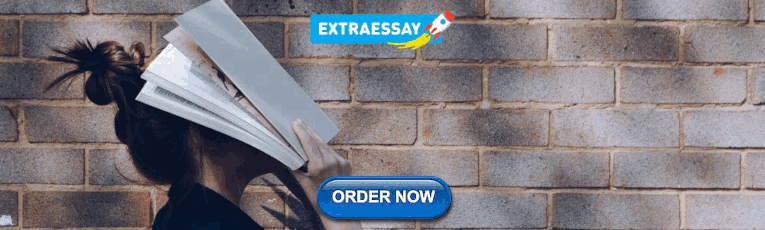
Make a Report
You need to make the final report of all the findings you have done at this stage. You should include the dataset, findings, and every aspect of your analysis in it.
While making the final report , do not forget to consider your audience. For instance, you are writing for the Newsletter, Journal, Public awareness, etc., your report should be according to your audience. It should be concise and have some logic; it should not be repetitive. You can use the references of other relevant sources as evidence to support your discussion.
Frequently Asked Questions
What is meant by thematic analysis.
Thematic Analysis is a qualitative research method that involves identifying, analyzing, and interpreting recurring themes or patterns in data. It aims to uncover underlying meanings, ideas, and concepts within the dataset, providing insights into participants’ perspectives and experiences.
You May Also Like
What are the different types of research you can use in your dissertation? Here are some guidelines to help you choose a research strategy that would make your research more credible.
Content analysis is used to identify specific words, patterns, concepts, themes, phrases, or sentences within the content in the recorded communication.
Sampling methods are used to to draw valid conclusions about a large community, organization or group of people, but they are based on evidence and reasoning.
USEFUL LINKS
LEARNING RESOURCES
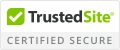
COMPANY DETAILS
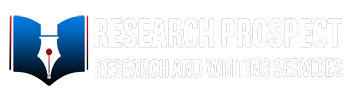
- How It Works
Themes, Theories, and Models
Affiliation.
- 1 1 Portland State University, Portland, Oregon, USA.
- PMID: 29313466
- DOI: 10.1177/1049732317750127
Although theory building is often described as the ultimate goal of qualitative research, an examination of articles in Qualitative Health Research ( QHR) shows that themes are actually the typical format for reporting results. In addition, articles that rely on themes often present low-level theories in the form of models that connect these themes. Because models have received less attention than either themes or theories, this article concentrates on summarizing four different kinds of models: hierarchies, timelines, processes, and cycles. In each of these cases, it presents both a general illustration of such a model and a realistic example from a published article in QHR. It concludes with a call for greater recognition of the role that models play in capturing the results of qualitative research.
Keywords: methodology; qualitative analysis; reflexivity; theory development.
- Health Services Research / methods*
- Models, Organizational
- Models, Theoretical*
- Qualitative Research
- Research Design
Techniques to Identify Themes in Qualitative Data
Gery W. Ryan RAND 1700 Main Street P.O. Box 2138 Santa Monica, CA 90407-2138 H. Russell Bernard Department of Anthropology 1350 Turlington Hall University of Florida Gaineville, FL 32611 Key Words: Theme Identification, Exploratory Analysis, Open Coding, Text Analysis, Qualitative Research Methods
Theme identification is one of the most fundamental tasks in qualitative research. It also one of the most mysterious. Explicit descriptions of theme discovery are rarely described in articles and reports and if so are often regulated to appendices or footnotes. Techniques are shared among small groups of social scientists and are often impeded by disciplinary or epistemological boundaries. During the proposal-writing phase of a project, investigators struggle to clearly explain and justify plans for discovering themes. These issues are particularly cogent when funding reviewers are unfamiliar with qualitative traditions. In this article we have outlined a dozen techniques that social scientists have used to discover themes in texts. The techniques are drawn from across epistemological and disciplinary boundaries. They range from quick word counts to laborious, in-depth, line-by-line scrutiny. Some methods work well for short answers to open-ended questions while others are more appropriate for rich, complex narratives. Novices and non-native speakers may find some techniques easier than others. No single technique is does it all. To us, these techniques are simply tools to help us do better research.
Authors’ Statement
Gery W. Ryan is an Associate Behavioral Scientist at RAND in Santa Monica, California. H. Russell Bernard is professor of anthropology at the University of Florida. The research on which this article is based is part of a National Science Foundation Grant, on "Methods for Conducting Systematic Text Analysis" (SRB-9811166). We wish to thank Stephen Borgatti for his helpful suggestions and two anonymous reviewers for their invaluable comments on earlier drafts of this paper.
Introduction
At the heart of qualitative data analysis is the task of discovering themes. By themes, we mean abstract, often fuzzy, constructs which investigators identify before, during, and after data collection. Where do these themes come from?
They come from reviewing the literature, of course. Richer literatures produce more themes. They come from the characteristics of the phenomena being studied. And they come from already-agreed-upon professional definitions, from local common-sense constructs, and from researchers’ values, theoretical orientation, and personal experience with the subject matter (Bulmer 1979; Strauss 1987; Maxwell 1996).
Mostly, though, researchers who consider themselves part of the qualitative tradition in social science induce themes from texts. This is what grounded theorists call open coding , and what classic content analysts call qualitative analysis (Berleson 1952) or latent coding (Shapiro and Markoff 1997). There are many variations on these methods. Unfortunately, however, they are (a) scattered across journals and books that are read by disparate groups of specialists; and (b) often entangled in the epistemological wars that have divided the social sciences. Our goal in this paper is to cross these boundaries and lay out a variety of theme-dredging methods so that all researchers who deal with texts can use them to solve common research problems.
We outline here a dozen helpful techniques for discovering themes in texts. These techniques are based on: (1) an analysis of words (word repetitions, key-indigenous terms, and key-words-in contexts); (2) a careful reading of larger blocks of texts (compare and contrast, social science queries, and searching for missing information); (3) an intentional analysis of linguistic features (metaphors, transitions, connectors); and (4) the physical manipulation of texts (unmarked texts, pawing, and cut and sort procedures).
The list is by no means exhaustive. Social scientists are an enterprising lot. Over the last century they have invented solutions to all kinds of problems for managing and analyzing texts, and they will continue to do so. These bursts of methodological creativity, however, are commonly described perfunctorily, or are relegated to footnotes, and get little notice by colleagues across disciplines. The dozen methods we describe here come from across the social sciences and have been used by positivists and interpretivists alike.
1. Word repetitions
We begin with word-based techniques. Word repetitions, key-indigenous terms, and key-words-in-contexts (KWIC) all draw on a simple observation—if you want to understand what people are talking about, look at the words they use.
Words that occur a lot are often seen as being salient in the minds of respondents. D'Andrade notes that "perhaps the simplest and most direct indication of schematic organization in naturalistic discourse is the repetition of associative linkages" (1991:294). He observes that "indeed, anyone who has listened to long stretches of talk, whether generated by a friend, spouse, workmate, informant, or patient, knows how frequently people circle through the same network of ideas" (1991:287).
Word repetitions can be analyzed formally and informally. In the informal mode, investigators simply read the text and note words or synonyms that people use a lot. For example, while conducting multiple in-depth interviews with Tony, a retired blue collar worker in Connecticut, Claudia Strauss (1992) found that Tony repeatedly referred to ideas associated with greed, money, businessmen, siblings, and "being different." These repetitions indicated to Strauss that these ideas were important, recurring themes in Tony’s life. Strauss displayed the relationships among these ideas by writing the concepts on a page of paper and connecting them with lines and explanations. Computer programs such as ATLAS.ti and Nud*ist let you do this kind of connect-the-dots exercise by computer. 1
A more formal analysis of word frequencies can be done by generating a list of all the unique words in a text and counting the number of times each occurs. Computers can easily generate word-frequency lists from texts and are a quick and easy way to look for themes. Ryan and Weisner (1996) asked fathers and mothers of adolescents: "Describe your children. In your own words, just tell us about them." Ryan and Weisner produced a list of all the unique words in the set of responses and the number of times each word was used by mothers and by fathers. Mothers were more likely than fathers to use words like friends, creative, time, and honest; fathers were more likely than mothers to use words like school, good, lack, student, enjoys, independent, and extremely. Ryan and Weisner used this information as clues for themes that they would use later in actually coding the texts.
2. Indigenous categories
Another way to find themes is to look for local terms that may sound unfamiliar or are used in unfamiliar ways. Patton (1990:306, 393-400) refers to these as "indigenous categories" and contrasts them with "analyst-constructed typologies." Grounded theorist refer to the process of identifying local terms as in vivo coding (Strauss 1987:28-32, Strauss and Corbin 1990:61-74) .
Understanding indigenous categories and how they are organized has long been a goal of cognitive anthropologists. The basic idea in this area of research is that experience and expertise are often marked by specialized vocabulary. For example, Spradley (1972) recorded conversations among tramps at informal gatherings, meals, card games, and bull sessions. As the men talked to each other about their experiences, there were many references to making a flop .
Spradley combed through his recorded material and notes looking for verbatim statements made by informants about his topic. On analyzing the statements, he found that most of the statements could fit into subcategories such as kinds of flops , ways to make flops , ways to make your own flop , kinds of people who bother you when you flop , ways to make a bed , and kinds of beds . Spradley then returned to his informants and sought additional information from them on each of the subcategories. For other classic examples of coding for indigenous categories see Becker’s (1993) description of medical students use of the word crock , and Agar’s (1973) description of drug addicts’ understandings of what it means to shoot up .
3. Key-words-in-context (KWIC)
Key-words-in-context (KWIC) are closely associated with indigenous categories. KWIC is based on a simple observation: if you want to understand a concept, then look at how it is used. In this technique, researchers identify key words and then systematically search the corpus of text to find all instances of the word or phrase. Each time they find a word, they make a copy of it and its immediate context. Themes get identified by physically sorting the examples into piles of similar meaning.
The concept of deconstruction is an abstract and often incomprehensible term used by social scientists, literary critics and writers in the popular press. Jacques Derrida, who coined the term, refused to define it. To Derrida, the meaning of any text is inherently unstable and variable. Wiener (1997) was curious as to how the concept of deconstruction was used in the popular press. He used a text-based data set (such as Lexis/Nexis), to find instances of the word in popular publications. He found the term used in by everything from Entertainment Weekly to the American Banker . Wiener concludes that:
Most often writers use "deconstruction" as a fancy word for "analysis" or "explanation," or else as an upscale synonym for "destruction." But in some genres, like rock music writing, the term isn't negative at all; it has become a genuinely floating signifier, a verbal gesture that implies a kind of empty intellectual sophistication.
Word-based techniques are typically a fast and efficient ways to start looking for themes. We find that they are particularly useful at early stages of theme identification. These techniques are also easy for novice researchers to apply. Nothing, however, beats a careful scrutiny of the texts for finding themes that may be more subtle or that don’t get signified directly in the lexicon of the text. Scrutiny-based techniques are more time-intensive and require a lot of attention to details and nuances.
4. Compare and contrast
The compare and contrast approach is based on the idea that themes represent the ways in which texts are either similar or different from each other. Glazer and Strauss (1967:101_116) refer to this as the "constant comparison method." [For other good descriptions of the technique see Glazer (1978:56_72) and Strauss and Corbin (1990:84_95).] Typically, grounded theorists begin by conducting a careful line-by-line analysis. They read each line or sentence and ask themselves, "What is this about?" and "How does it differ from the preceding or following statements?" This kind of detailed work keeps the researcher focused on the data themselves rather than on theoretical flights of fancy (Charmaz 1990).
This approach is like interviewing the text and is remarkably similar to the ethnographic interviewing style that Spradley talks about using with his informants (1979:160_172). Researchers compare pairs of texts by asking "How is this text different from the preceding text?" and "What kinds of things are mentioned in both?" They ask hypothetical questions like "What if the informant who produced this text had been a woman instead of a man?" and "How similar is this text to my own experiences?" Bogdan and Biklen (1982:153) recommend reading through passages of text and asking "What does this remind me of?" Like a good journalist, investigators compare answers to questions across people, space, and time.
5. Social science queries
Besides identifying indigenous themes—themes that characterize the experience of informants—researchers are interested in understanding how textual data illuminate questions of importance to social science. Spradley (1979:199–201) suggested searching interviews for evidence of social conflict, cultural contradictions, informal methods of social control, things that people do in managing impersonal social relationships, methods by which people acquire and maintain achieved and ascribed status, and information about how people solve problems. Bogdan & Bilken (1982:156-162) suggested examining the setting and context, the perspectives of the informants, and informants’ ways of thinking about people, objects, processes, activities, events, and relationships. "Moving across substantive areas," says Charmaz, "fosters developing conceptual power, depth, and comprehensiveness" (1990:1163).
Strauss and Corbin (1990:158_175) urge investigators to be more sensitive to conditions, actions/interactions, and consequences of a phenomenon and to order these conditions and consequences into theories. To facilitate this, they offer a useful tool called the conditional matrix. The conditional matrix is a set of concentric circles, each level corresponding to a different unit of influence. At the center are actions and interactions. The inner rings represent individual and small group influences on these actions, and the outer rings represent international and national effects.
Querying the text as a social scientist is a powerful technique because investigators concentrate their efforts on searching for specific kinds of topics – any of which are likely to generate major social and cultural themes. By examining the data from a more theoretical perspective, however, researchers must be careful that they do not overfit the data – that is, find only that for which they are looking. There is a trade-off between bringing a lot of prior theorizing to the theme-identification effort and going at it fresh. Prior theorizing, as Charmaz says (1990), can inhibit the forming of fresh ideas and the making of surprising connections. Assiduous theory-avoidance brings the risk of not making the connection between data and important research questions. Novice researchers may be more comfortable with the tabula rasa approach. More seasoned researchers, who are more familiar with theory issues, may find the social science query approach more compatible with their interests.
6. Searching for missing information
The final scrutiny-based approach we describe works in reverse from typical theme identification techniques. Instead of identifying themes that emerge from the text, investigators search for themes that are missing in the text.
Much can be learned from a text by what is not mentioned. As early as 1959, propaganda analysts found that material not covered in political speeches were sometimes more predictive that material that was covered (George 1959). Sometimes silences indicate areas that people are unwilling or afraid to discuss. For instance, women with strong religious convictions may fail to mention abortion during discussions of birth control. In power-laden interviewers, silence may be tied to implicit or explicit domination (Gal 1991). In a study of birth planning in China, Greenhalgh (1994) surveyed 1,011ever-married women, gathered social and economic histories from 150 families. She conducted in-depth interviews with present and formal officials (known as cadres), and collected documentary evidence from local newspapers, journals and other sources. Greenhalgh notes that "Because I was largely constrained from asking direct questions about resistance, the informal record of field notes, interview transcripts, and questionnaire data contains few overt challenges to state policy (1994:9)." Greenhalgh concludes, however, that
I believe that in their conversations with us, both peasants and cadres made strategic use of silence to protest aspects of the policy they did not like. Cadres, for example were loathe to comment on birth-planning campaigns; peasant women were reluctant to talk about sterilization. These silences form one part of the unofficial record of birth planning in the villages. More explicit protests were registered in informal conversations. From these interactions emerged a sense of profound distress of villagers forced to choose between a resistance that was politically risky and a compliance that violated the norms of Chinese culture and of practical reason (1994:9).
Other times, absences may indicate primal assumptions made by respondents. Spradley (1987:314) noted that when people tell stories, they assume that their listeners share many assumptions about how the world works and so they leave out information that "everyone knows." He called this process abbreviating . Price (1987) takes this observation and builds on it. Thus, she looks for what is not said in order to identify underlying cultural assumptions. Price finds the missing pieces by trying to translate what people say in the stories into something that the general public would understand.
Of all the scrutiny-based techniques, searching for missing information is the most difficult. There are many reasons people do not mention topics. In addition to avoiding sensitive issues or assuming investigator already knows about the topic, people may not trust the interviewer, may not wish to speak when others are present, or may not understand the investigator’s questions. Distinguishing between when informants are unwilling to discuss topics and when they assume the investigator already knows about the topic requires a lot of familiarity with the subject matter.
In addition to word- and scrutiny-based techniques, researchers have used linguistic features such as metaphors, topical transitions, and keyword connectors to help identify themes.
7. Metaphors and analogies
Schema analysts suggest searching through text for metaphors, similes, and analogies (D’Andrade 1995, Quinn and Strauss 1997). The emphasis on metaphor owes much to the pioneering work by Lakoff and Johnson (1980) and the observation that people often represent their thoughts, behaviors, and experiences with analogies.
Naomi Quinn (1997) has analyzed hundreds of hours of interviews to discover concepts underlying American marriage and to show how these concepts are tied together. She began by looking at patterns of speech and at the repetition of key words and phrases, paying particular attention to informants' use of metaphors and the commonalities in their reasoning about marriage. Nan, one of her informants, says that "marriage is a manufactured product." This popular metaphor indicates that Nan sees marriages as something that has properties, like strength and staying power, and as something that requires work to produce. Some marriages are "put together well," while others "fall apart" like so many cars or toys or washing machines (Quinn 1987:174).
The object is to look for metaphors in rhetoric and deduce the schemas, or underlying principles, that might produce patterns in those metaphors. Quinn found that people talk about their surprise at the breakup of a marriage by saying that they thought the couple’s marriage was "like the Rock of Gibraltar" or that they thought the marriage had been "nailed in cement." People use these metaphors because they assume that their listeners know that cement and the Rock of Gibraltar are things that last forever.
But Quinn reasons that if schemas or scripts are what make it possible for people to fill in around the bare bones of a metaphor, then the metaphors must be surface phenomena and cannot themselves be the basis for shared understanding. Quinn found that the hundreds of metaphors in her corpus of texts fit into just eight linked classes that she calls: lastingness, sharedness, compatibility, mutual benefit, difficulty, effort, success (or failure), and risk of failure. For example, Quinn’s informants often compared marriages (their own and those of others) to manufactured and durable products ("it was put together pretty good") and to journeys ("we made it up as we went along; it was a sort of do-it-yourself project"). Quinn sees these metaphors, as well as references to marriage as "a lifetime proposition," as exemplars of the overall expectation of lastingness in marriage.
Other examples of the search for cultural schemas in texts include Holland’s (1985) study of the reasoning that Americans apply to interpersonal problems, Kempton’s (1987) study of ordinary Americans’ theories of home heat control, and Claudia Strauss’s (1997) study of what chemical plant workers and their neighbors think about the free enterprise system.
8. Transitions
Another linguistic approach is to look for naturally occurring shifts in thematic content. Linguistic forms of transition vary between oral and written texts. In written texts, new paragraphs are often used by authors to indicate either subtle or abrupt shifts in topics. In oral speech, pauses, change in tone, or particular phrases may indicate thematic transitions. Linguists who have worked with precisely recorded texts in Native American languages have noticed the recurrence of elements like "Now," "Then," "Now then," and "Now again." These often signal the separation of verses and "once such patterning has been discovered in cases with such markers, it can be discerned in cases without them" (Hymes 1977:439).
For example, Sherzer (1994) presents a detailed analysis of a two-hour performance by Chief Olopinikwa of a traditional San Blas Kuna chant. The chant was recorded in 1970. Like many linguistic anthropologists, Sherzer had taught an assistant, Alberto Campos, to use a phonetic transcription system. After the chant, Sherzer asked Campos, to transcribe and translate the tape. Campos put Kuna and Spanish on left- and right-facing pages (1994:907). By studying Campos’s translation against the original Kuna, Sherzer was able to pick out certain recurrent features. Campos left out the chanted utterances of the responding chief (usually something like "so it is"), which turned out to be markers for verse endings in the chant. Campos also left out so-called framing words and phrases (like "Thus" at the beginning of a verse and "it is said, so I pronounce" at the end of a verse). These contribute to the line and verse structure of the chant. Finally, "instead of transposing metaphors and other figurative and allusive language into Spanish" Campos "explains them in his translation" (Sherzer 1994:908). Researchers
In two-party and multiparty speech, transitions occur naturally. Conversation or discourse analysts closely examine linguistic features such as turn-taking and speaker interruptions to identify transitions in speech sequences. For a good overview, see Silverman (1993:114-143).
9. Connectors
A third linguistic approach is to look carefully at words and phrases that indicate relationships among things. For example, causal relationships are often indicated by such words and phrases as, because, since, and as a result. Words such as if or then , rather than, and instead of often signify conditional relationships. The phrase is a is often associated with taxonomic categories. Time-oriented relationships are expressed with words such as before, after, then, and next . Typically negative characteristics occur less often than positive characteristics. Simply searching for the words not , no , none , or the prefix non may be a quick way to identify themes. Investigator can discover themes by searching on such groups of word and looking to see what kinds of things the words connect.
What other kinds of relationships might be of interest to social scientists? Casagrande and Hale (1967) suggest looking for: attributes (e.g., X is Y), contingencies (e.g., if X, then Y), functions (e.g., X is a means of affecting Y), spatial orientations (e.g., X is close to Y), operational definitions (e.g., X is a tool for doing Y), examples (e.g., X is an instance of Y), comparisons (e.g., X resembles Y), class inclusions (X is a member of class Y), synonyms (e.g., X is equivalent to Y), antonyms (e.g., X is the negation of Y), provenience (e.g., X is the source of Y), and circularity (e.g., X is defined as X). [For lists of kinds of relationships that may be useful for identifying themes see Burton and Kirk (1980:271), Werner and Schoepfle (1987) and Lindsay and Norman (1972).]
Investigators often use the linguistic features described above unconsciously. Metaphors, transitions, and connectors are all part of a native speaker’s ability to grasp meaning in a text. By making these features more explicit, we sharpen our ability to find themes.
Finally, we turn to more tactile approaches for theme discovery. Each of the next three techniques requires some physical manipulation of the text itself.
10. Unmarked texts
One way to identify new themes is to examine any text that is not already associated with a theme (Ryan 1999). This technique requires multiple readings of a text. On the first reading, salient themes are clearly visible and can be quickly and readily marked with different colored pencils or highlighters. In the next stage, the search is for themes that remain unmarked. This tactic–marking obvious themes early and quickly—forces the search for new, and less obtrusive themes.
We highly recommend pawing through texts and marking them up with different colored highlighter pens. Sandelowski (1995a:373) observes that analysis of texts begins with proofreading the material and simply underlining key phrases "because they make some as yet inchoate sense." Bernard (2000) refers to this as the ocular scan method , otherwise known as eyeballing . In this method, you get a feel for the text by handling your data multiple times. [Bogdan and Biklen (1982:165) suggest reading over the text at least twice.] Researchers have been known to spread their texts out on the floor, tack bunches of them to a bulletin board, and sort them into different file folders. By living with the data, investigators can eventually perform the interocular percussion test—which is where you wait for patterns to hit you between the eyes.
This may not seem like a very scientific way to do things, but it is one of the best ways we know of to begin hunting for patterns in qualitative data. Once you have a feel for the themes and the relations among, then we see no reason to struggle bravely on without a computer. Of course, a computer is required from the onset if the project involves hundreds of interviews, or if it’s part of a multi-site, multi-investigator effort. Even then, there is no substitute for following hunches and intuitions in looking for themes to code in texts (Dey 1993).
12. Cutting and sorting
Cutting and sorting is a more formal way of pawing and a technique we both use quite a bit. It is particularly useful for identifying subthemes. The approach is based on a powerful trick most of us learned in kindergarten and requires paper and scissors. We first read through the text and identify quotes that seem somehow important. We cut out each quote (making sure to maintain some of the context in which it occurred) and paste the material on small index cards. On the back of each card, we then write down the quote’s reference—who said it and where it appeared in the text. Then we lay out the quotes randomly on a big table and sort them into piles of similar quotes. Then we name each pile. These are the themes. This can be done with tag and search software, but we find that nothing beats the ability to manually sort and group the cards.
There are many variations on this pile-sorting technique. The principle investigator on a large project might ask several team members to sort the quotes into named piles independently. This is likely to generate a longer list of possible themes than would be produced by a group discussion. In really large projects, pairs of coders could sort the quotes together and decide on the names for the piles. The pile-sorting exercise should be video- or audiotaped and investigators should pay close attention to discussions—between themselves and coders or between coders—about which quotes belong together and why. These conversations are about as close as we will ever get to witnessing the emergence of themes.
Barkin et al. (1999) interviewed clinicians, community leaders, and parents about what physicians could and did do to prevent violence among youth. These were long, complex interviews, so Barkin et al. broke the coding process into two steps. They started with three major themes that they developed from theory. The principle investigator went through the transcripts and cut out all the quotes that pertained to each of the major themes. Then four other coders independently sorted the quotes from each major theme into piles. Then, the pile sort data were analyzed with multidimensional scaling and cluster analysis to identify subthemes shared across coders. [See Patterson et al. (1993) for another example.]
Jehn and Doucet (1997) had short answers to open-ended questions. They found that several coders could easily sort these paragraph-length descriptions of inter and intra-ethnic conflict. Then, like Barkin et al., Jehn and Doucet then used multidimensional scaling and cluster analysis to identify subthemes of conflict.
Another advantage to the cutting and sorting technique is that the data can be used to systematically describe how such themes are distributed across informants. After the piles have been formed and themes have been named, simply turn over each quote and identify who mentioned each theme. (If the people sorting the quotes are unaware of who the quotes came from, this is an unbiased way of coding.)
The variety of methods available for coding texts raises some obvious questions:
(1) Which technique generates more themes?
Frankly, we don’t know. There are just too many factors that influence the number of themes that are generated, including the technique itself, who and how many people are looking for themes, and the kind and amount of texts being analyzed. If the goal is to generate as many themes as possible—which is often the case in initial exploratory phases of research—then more is better. This means using multiple techniques, investigators, and texts.
Nowhere is a multiple technique approach better exemplified than in the work of Jehn and Doucet (1996, 1997). Jehn and Doucet asked 76 U.S. managers who had worked in Sino_American joint ventures to describe recent interpersonal conflicts with business partners. Each person described a situation with a same_culture manager and a different_cultural manger. First they generated separate lists of words from the intercultural and intracultural conflict narratives. They asked 3 expatriate managers to act as judges and to identify all the words that were related to conflict. They settled on a list of 542 conflict words from the intercultural list and 242 words from the intracultural list.
Jehn and Doucet then asked the three judges to sort the words into piles or categories. The experts identified 15 subcategories for the intercultural data—things like conflict, expectations, rules, power, and volatile—and 15 categories for the intracultural data—things like conflict, needs, standards, power, contentious, and lose. Taking into consideration the total number of words in each corpus, conflict words were used more in intracultural interviews and resolution terms were more likely to be used in intercultural interviews.
Jehn and Doucet (1996, 1997) also used traditional content analysis on their data. The had two coders read the 152 conflict scenarios (76 intracultural and 76 intercultural) and evaluated (on a 5_point scale) each on 27 different themes they had identified from the literature. This produced two 76x27 scenario_by_theme profile matrices—one for the intracultural conflicts and one for the intercultural conflicts. The first three factors from the intercultural matrix reflect: (1) interpersonal animosity and hostility; (2) aggravation; and (3) the volatile nature of the conflict. The first two factors from the intracultural matrix reflect: (1) hatred and animosity with a volatile nature and (2) conflicts conducted calmly with little verbal intensity.
Finally, Jehn and Doucet identified the 30 intracultural and the 30 intercultural scenarios that they felt were the most clear and pithy. They recruited fifty more expatriate managers to assess the similarities (on a 5_point scale) of 60–120 randomly selected pairs of scenarios. When combined across informants, the managers judgments produced two aggregate, scenario_by_scenario, similarity matrices—one for the intracultural conflicts and one for the intercultural conflicts.
Multidimensional scaling of the intercultural similarity data identified four dimensions: (1) open versus resistant to change, (2) situational causes versus individual traits, (3) high_ versus low_resolution potential based on trust, and (4) high_ versus low_resolution potential based on patience. Scaling of the intracultural similarity data identified four different dimensions: (1) high versus low cooperation, (2) high versus low confrontation, (3) problem_solving versus accepting, and (4) resolved versus ongoing.
The work of Jehn and Doucet is impressive because the analysis of the data from these tasks produced different sets of themes. All three emically induced theme sets have some intuitive appeal and all three yield analytic results that are useful. They could have also used the techniques of grounded theory or schema analysis to discover even more themes.
(2) When are the various techniques most appropriate?
The choice of techniques depends minimally on the kind and amount of text, the experience of the researcher, and the goals of the project. Word-based techniques (e.g., word repetitions, indigenous categories, and KWIC) are probably the least labor intensive. Computer software such as Anthropac and Code-a-text have little trouble in generating frequency counts of key words. 2 A careful look at the frequency list and maybe some quick pile sorts are often enough to identify quite a few themes. Word-based techniques are also the most versatile. They can easily be used with complex texts such as the complete works of Shakespear or the Bible, as well as, with simple short answers to open-ended questions. They can also be used relatively easily by novice and expert investigators alike. Given their very nature, however, they are best used in combination with other approaches.
Scrutiny-based techniques (e.g., compare and contrast, querying the text, and examining absences) are most appropriate for rich textual accounts and tend to be overkill for analyzing short answer responses. Investigators who are just beginning to explore a new topical area might want to start with compare-and-contrast techniques before moving on to the more difficult tasks of querying the text or searching for missing information. We do not advise using the latter two techniques unless the investigator is fluent in the language in which the data are collected. If the primary goal of the this portion of the investigation is to discover as many themes as possible, then nothing beats using these techniques on a line-by-line basis.
Like scrutiny-based techniques, linguist-based approaches are better used on narrative style accounts rather than short answer responses. Looking for transitions is the easiest technique to use, especially if the texts are actually written by respondents themselves (rather than transcribed from tape recordings of verbal interviews). Searching for metaphors is also relatively easy once novices have been trained on what kind of things to look for in the texts. Looking for connecting words and phrases is best used as a secondary wave of finding themes, once the investigator has a more definite idea of what kinds of themes he or she finds most interesting.
In the early stages of exploration, nothing beats a thorough reading and pawing through of the data. This approach is the easiest for novice researchers to master and is particularly good for identifying major themes. As the exploration progresses, investigators often find themselves looking for subthemes within these major themes. The cutting and sorting techniques are most helpful here. Investigators can identify all text passages that are related to a major theme, cut them out, and sort them into subthematic categories. Likewise, if they are marking texts for each newly discovered theme, then they can apply the unmarked text technique as they go. We have seen these three techniques applied successfully to both rich narrative data as well as simple responses to open-ended questions.
An even more powerful strategy would be to combine multiple techniques in a sequential manner. For example, investigators might begin by pawing through the data to see what kinds of themes just stick out. As part of this process, they might want to make comparisons between paragraphs and across informants. A quick analysis of word repetitions would also be appropriate for identifying themes at such an early stage of the analysis. If key words or indigenous phrases are present, researchers might followed-up by conducting more focused KWIC analyses. If the project is examining issues of equality, investigators might also look for texts that are indicative of power differentials and access to resources. Texts representing major themes can be marked either on paper or by computer. Investigators can then search areas that are not already marked for additional themes or cut and sort marked texts into subthemes.
Researchers also might consider beginning by looking for identifying all metaphors and similes, marking them, cutting them out and sorting them into thematic categories. There is no single way to discover themes. In theme discovery, we assume that more is always better.
(3) When do you know when you’ve found all the themes?
There is no magic formula to answer this question. The problem is similar to asking members of a population to list all the illnesses they know. One can never be sure of the full range of illnesses without interviewing the entire population. This is true because there is always the possibility that the last person interviewed will mention a new disease. We can simplify the process considerably, however, if we are willing to miss rarely-mentioned illness. One strategy would be to interview people until some number of respondents in a row (say five or more) fail to mention any new illnesses.
In text analysis, grounded theorists refer to the point at which no new themes are being identified as theoretical saturation (Strauss and Corbin 1990:188). When and how theoretical saturation is reached, however, depends the number of texts and their complexity, as well as on investigator experience and fatigue, and the number of investigators examining the texts. Again, more is better. Investigators who have more experience finding themes are likely to reach saturation latter than novices. Wilson and Hutchinson warn against premature closure where the researcher "fails to move beyond the face value of the content in the narrative (1990:123)."
Theme identification is one of the most fundamental tasks in qualitative research. It also one of the most mysterious. Explicit descriptions of theme discovery are rarely described in articles and reports and if so are often regulated to appendices or footnotes. Techniques are shared among small groups of social scientists and are often impeded by disciplinary or epistemological boundaries. The lack of clear methodological descriptions is most evident during the grant-writing phase of research. Investigators (ourselves included) struggle to clearly explain and justify plans for discovering themes in the qualitative data. These issues are particularly cogent when funding reviewers are unfamiliar with qualitative traditions.
In this article we have outlined a dozen techniques that social scientists have used to discover themes in texts. The techniques are drawn from across epistemological and disciplinary boundaries. They range from quick word counts to laborious, in-depth, line-by-line scrutiny. Some work well for short answers to open-ended questions while others are more appropriate for rich, complex narratives. Novices and non-native speakers may find some techniques easier than others. No single technique is does it all. To us, these techniques are simply tools to help us do better research.
1 ATLAS.ti (Scientific Software Development) and Nud•ist (Qualitative Solutions & Research) are qualitative analysis packages distributed in the United States by SCOLARI, Sage Publications, Inc., 2455 Teller Road, Thousand Oaks, CA 91320. Tel: (805) 499 1325. Fax: (805) 499 0871. E_mail: [email protected]. Web: www.scolari.com.
2 Anthropac (Analytic Technologies) and Coda-A-Text (Cartwright) are software packages that have the capacity to convert free flowing texts into word-by-document matrices. Code-A-Text is distributed in the United States by SCOLARI, Sage Publications. Anthropac is created and distributed by Analytic Technologies, Inc., Analytic Technologies, Inc., 11 Ohlin Lane, Harvard, MA 01451. Tel: (978) 456_7372. Fax: (978) 456_7373. E_mail: [email protected]. Web: www.analytictech.com.
References Cited
Agar, Michael.
1973 Ripping and running: A formal ethnography of urban heroin addicts . New York: Seminar Press.
Agar, Michael and Jerry Hobbs
1985 How to grow schemata out of interviews. In Directions in Cognitive Anthropology . Janet Dougherty, ed. Pp. 413-431. Urbana, IL: University of Illinois Press.
Barkin, Shari, Gery Ryan, Lillian Gelberg
1999 What clinicians can do to further youth violence primary prevention: A qualitative study. Injury Prevention , 5:53-58.
Becker, Howard
1993 How I learned what a crock was. Journal of Contemporary Ethnography 22:28-35.
1998 Tricks of the trade: How to think about your research while you’re doing it . Chicago: University of Chicago Press.
Berelson, Bernard
1952 Content analysis in communication research . Glencoe, IL: Free Press.
Bernard, H. Russell
2000 Social Research Methods: Qualitative and Quantitative Approaches . Thousand Oaks, CA: Sage Publications.
Bogdan, Robert, and Sari Knopp Biklen
1992 Qualitative Research for Education: An Introduction to Theory and Methods , 2d ed. Boston: Allyn and Bacon.
Borgatti, Stephen
1999 Elicitation Methods for Cultural Domain Analysis. In J. Schensul & M. LeCompte (Ed.) The Ethnographer's Toolkit, Volume 3 . Walnut Creek: Altamira Press, 115-151.
Bulmer, Martin
1979 Concepts in the analysis of qualitative data. Sociological Review 27 (4)651-677).
Charmaz, Kathy
1990 "Discovering" Chronic Illness: Using Grounded Theory. Social Science and Medicine 30 :1161–1172.
2000 Grounded theory: Objectivist and constructivist methods. In Handbook of Qualitative Research, 2 nd Edition . Norman Denzin and Yvonna Lincoln, eds. Thousand Oaks, CA: Sage Publications. Pp. 509-536.
D'Andrade, Roy
1995 The development of cognitive anthropology . Cambridge: Cambridge University Press.
1993 Qualitative Data Analysis: A User_Friendly Guide for Social Scientists . London: Routledge and Kegan Paul.
1991 Between speech and silence: The problematics of research on language and gender. In Gender at the crossroads of knowledge: Feminist anthropology in the postmodern era. Michaela di Leonardo, ed. Berkeley: University of California Press. Pp. 175-203.
George, A. L. 1959. Quantitative and qualitative approaches to content analysis. In Trends in content analysis I. de Sola Pool, ed. Pp. 7_32. : University of Illinois Press.
Glaser, Barney G. and Anselm Strauss
1967 The Discovery of Grounded Theory: Strategies for Qualitative Research . New York: Aldine.
Gladwin, Christina
1989 Ethnographic Decision Tree Modeling . Newbury Park, CA: Sage Publications.
Greenhalgh, Susan
1994 Controlling births and bodies. American Ethnologist 21:3_30.
Henley, N.M.
1969 A Psychological Study of the Semantics of Animal Terms. Journal of Verbal Learning and Verbal Behavior 8:176-84.
Jehn, Karen A. and Lorna Doucet
1996 Developing Categories from Interview Data: Text Analysis and Multidimensional Scaling. Part 1. Cultural Anthropology Methods Journal 8 (2):15–16.
1997 Developing Categories for Interview Data: Consequences of Different Coding and Analysis Strategies in Understanding Text. Part 2. Cultural Anthropology Methods Journal 9 (1):1–7.
Lindsay, Peter H. and Donald A Norman
1972. Human information processing: An introduction to psychology . New York: Academic Press.
Maxwell, Joseph
1996 Qualitative research design: An interactive approach. Thousand Oaks, CA: Sage Publications.
Miles, Matthew and A. Michael Huberman
1994 Qualitative Data Analysis , 2d ed. Thousand Oaks, CA: Sage Publications.
Patton, Michael Q.
1990 Qualitative Evaluation and Research Methods . Thousand Oaks, CA: Sage Publications.
Pool, de Sola, Ithiel, ed.
1959 Trends in Content Analysis . Urbana: University of Illinois Press.
Price, Laurie
1987 Ecuadorian Illness Stories. In Cultural Models in Language and Thought . D. Holland and N. Quinn, eds. Pp. 313–342. Cambridge: Cambridge University Press.
1999 Measuring the typicality of text: Using multiple coders for more than just reliability and validity checks. Human Organization , 58(3):313-322.
Spradley, James
1972 Adaptive Strategies of Urban Nomads. In Culture and Cognition: Rules, Maps, and Plans. J. P. Spradley, ed. Pp. 235-278. New York: Chandler Publishing Company.
1979. The Ethnographic Interview . New York: Holt, Rinehart and Winston.
Strauss, Claudia
1992 What makes Tony run? Schemas as motive reconsideration. In Human motives and cultural models R. D'Andrade and C. Strauss, eds. Pp. 191-224. Cambridge: Cambridge University Press.
Strauss, Claudia and Naomi Quinn
1997 A cognitive theory of cultural meaning . Cambridge: Cambridge University Press.
Wiener, Jon
1997 Deconstruction goes pop. (The increasing use of the word 'deconstruction'). The Nation , April 7, 264(13):43-45.
Wilson, Holy Skodol and Sally Hutchinson
1990 Methodologic mistakes in grounded theory. Nursing Research , 45(2):122-124.
Wright, Joanne
1997 Deconstructing development theory: Feminism, the public/private dichotomy and the Mexican maquiladoras. The Canadian Review of Sociology and Anthropology , 34(1):71-92.
The Lesson Study Group
at Mills College
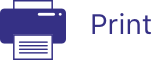
Develop a Research Theme
Access the teaching-learning plan, choose a topic.
10-30 minutes
- Develop or revisit your your long-term goals for student development
- Develop a draft of your “theory of action”—the approaches you will explore to build your long-term goals
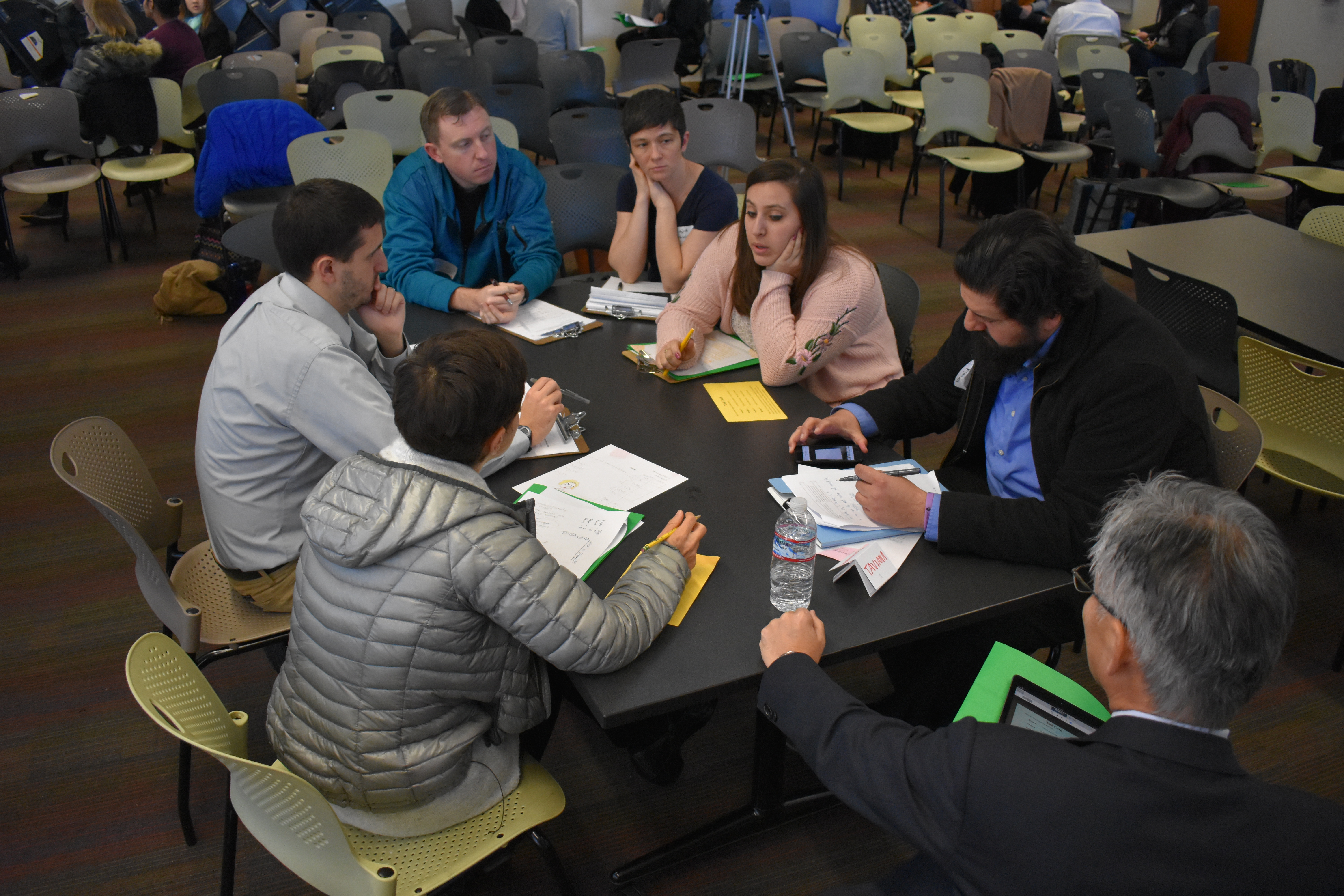
Identify Your Long-Term Goals
The building blocks of your theme.
A research theme expresses the long-term goals of your work. If your team or school has already developed a research theme, revisit it now to refresh your memory about your long-term goals and ideas about how to get there.
Begin by having team members individually jot down qualities in response to the following prompt:
- Ideally, what qualities do we hope students will have when they graduate from our school? ( If we bumped into our students in 5-10 years, what qualities do we hope they would have?)
Now, again working individually, spend a few minutes jotting down a list of qualities in response to a second prompt:
- What are the current qualities of our students? (For example, what qualities of our students inspire us? Anything that concerns us?)
Again, share your individual lists and write all the qualities on a second list labeled “Current.”
Compare the two lists–ideal and current–and notice gaps that really speak to you as educators. Find one or two gaps where you would like to invest your time and energy.
Your research theme positively states the qualities you will work toward. Some examples follow.
- “For students to value friendship, develop their own perspectives and ways of thinking, and enjoy science.”
- “Develop social-emotional skills and…a deeper understanding of mathematics”
- “Across both math and language arts, develop our students’ abilities to use evidence and reasoning to support and critique arguments.”
- “…to take responsibility and initiative as learners.”
A lot of [U.S.] schools develop mission statements, but we don’t do anything with them. The mission statements get put in a drawer and then teachers become cynical…Lesson Study gives guts to a mission statement, makes it real, and brings it to life.
Develop a Theory of Action
Moving from the what to the how.
The second part of your research theme is a “theory of action”—how you will work toward your long-term goals and the specific research questions you will examine. What experiences in school help students move toward a goal such as “students have their own thoughts and can explain them logically?” Teachers addressing this research theme focused their initial theory of action on two classroom routines: students’ presentation of ideas at the board and their use of reflective journals. They actively tested strategies to improve these two classroom routines and posed questions about them. For example, they asked what the features are of effective student presentations and how teachers help students see the power of these strategies (such as using visual models). In order to strengthen the impact of reflective mathematics journals, teachers strategically selected several student journals from the prior day to be read aloud at the beginning of each mathematics lesson, which built students’ interest in each other’s ideas and helped them see the impact of well-explained ideas. The first part of your research theme—your overarching goal—is likely to stay the same for several years. The second part of your research theme—your theory of action—is likely to change as you incorporate effective ideas into your practice and go on to experiment with additional changes designed to achieve your long-term goals. For example, the group that experimented with changes to student presentations and reflective journals went on to experiment with routines for discussion and lesson summarization that further built students’ capacity “to have their own thoughts and explain them logically.”
Developing a Research Theme Presentation
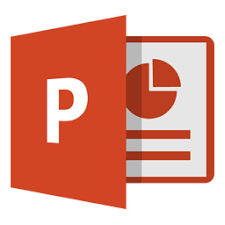
Examples of Research Themes
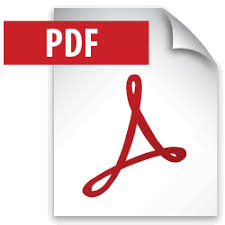
- What is Lesson Study?
- Why Lesson Study?
- Teacher Learning
- Content Resources
- Teaching Through Problem-solving (TTP)
- School-wide Lesson Study
- U.S. Networks
- International Networks
- Architecture and Design
- Asian and Pacific Studies
- Business and Economics
- Classical and Ancient Near Eastern Studies
- Computer Sciences
- Cultural Studies
- Engineering
- General Interest
- Geosciences
- Industrial Chemistry
- Islamic and Middle Eastern Studies
- Jewish Studies
- Library and Information Science, Book Studies
- Life Sciences
- Linguistics and Semiotics
- Literary Studies
- Materials Sciences
- Mathematics
- Social Sciences
- Sports and Recreation
- Theology and Religion
- Publish your article
- The role of authors
- Promoting your article
- Abstracting & indexing
- Publishing Ethics
- Why publish with De Gruyter
- How to publish with De Gruyter
- Our book series
- Our subject areas
- Your digital product at De Gruyter
- Contribute to our reference works
- Product information
- Tools & resources
- Product Information
- Promotional Materials
- Orders and Inquiries
- FAQ for Library Suppliers and Book Sellers
- Repository Policy
- Free access policy
- Open Access agreements
- Database portals
- For Authors
- Customer service
- People + Culture
- Journal Management
- How to join us
- Working at De Gruyter
- Mission & Vision
- De Gruyter Foundation
- De Gruyter Ebound
- Our Responsibility
- Partner publishers

Your purchase has been completed. Your documents are now available to view.
Topical themes and thematic progression: the “picture” of research articles
Leong Ping Alvin lectures at the Language and Communication Centre, Nanyang Technological University, Singapore. He obtained his PhD degree from the National University of Singapore in 2001 under a research scholarship. His book-length publications include Transforming Literacies and Language (co-editor; Continuum, 2011) and Theme and Rheme (Peter Lang, 2004). His research interests are in systemic-functional grammar, discourse analysis, and literacy studies.
Although much has been written about the features of academic writing, there is a lack of research attention on macro issues related to the development of ideas, particularly in the writing of research articles. A concept that is useful in investigating such issues is the Hallidayan notion of theme. However, the thematic structure of research articles has received only modest attention over the years. It is also rare for thematic diagrams to be used even though they can be helpful in clarifying the thematic structure of the text. In this exploratory study, the patterning of topical themes in research articles was investigated using a diagrammatic approach. Twenty biology-related research articles were divided into t-units and analyzed for topical themes. Thematic diagrams were generated for all the articles. The diagrams revealed a progressive thematic pattern in the introduction sections of all the articles. At the whole-text level, an anchored-development pattern was observed in the majority of the articles. These findings suggest that research articles at the macro level share similarities in their thematic structure. They also shed light on how authors achieve focus in the writing through the systematic use of clause-initial elements.
About the author
Appendix: articles analyzed in the study, articles from database.
Hishigaki, Haretsugu & Satoru Kuhara. 2011. hERGAPDbase: A database documenting hERG channel inhibitory potentials and APD-prolongation activities of chemical compounds. Database 2011. doi: 10.1093/database/bar017.
Green, Jason M., Jaturon Harnsomburana, Mary L. Schaeffer, Carolyn J. Lawrence & Chi-Ren Shyu. 2011. Multi-source and ontology-based retrieval engine for maize mutant phenotypes. Database 2011. doi: 10.1093/database/bar012.
Harper, Lisa C., Mary L. Schaeffer, Jordan Thistle, Jack M. Gardiner, Carson M. Andorf, Darwin A. Campbell, Ethalinda K. S. Cannon, Bremen L. Braun, Scott M. Birkett, Carolyn J. Lawrence & Taner Z. Sen. 2011. The MaizeGDB genome browser tutorial: One example of database outreach to biologists via video. Database 2011. doi: 10.1093/database/bar016.
Visendi, Paul, Wanjiku Ng’ang’a, Wallace Bulimo, Richard Bishop, James Ochanda & Etienne P. de Villiers. 2011. TparvaDB: A database to support Theileria parva vaccine development. Database 2011. doi: 10.1093/database/bar015.
Williams, G. W., P. A. Davis, A. S. Rogers, T. Bieri, P. Ozersky & J. Spieth. 2011. Methods and strategies for gene structure curation in WormBase. Database 2011. doi: 10.1093/database/baq039.
Blankenberg, Daniel, Nathan Coraor, Gregory Von Kuster, James Taylor & Anton Nekrutenko. 2011. Integrating diverse databases into an unified analysis framework: A Galaxy approach. Database 2011. doi: 10.1093/database/bar011.
Vellozo, Augusto F., Amélie S. Véron, Patrice Baa-Puyoulet, Jaime Huerta-Cepas, Ludovic Cottret, Gérard Febvay, Federica Calevro, Yvan Rahbé, Angela E. Douglas, Toni Gabaldón, Marie-France Sagot, Hubert Charles & Stefano Colella. 2011. CycADS: An annotation database system to ease the development and update of BioCyc databases. Database 2011. doi: 10.1093/database/bar008.
Magrane, Michele & UniProt Consortium. 2011. UniProt Knowledgebase: A hub of integrated protein data. Database 2011. doi: 10.1093/database/bar009.
Costanzo, Maria C., Julie Park, Rama Balakrishnan, J. Michael Cherry & Eurie L. Hong. 2011. Using computational predictions to improve literature-based Gene Ontology annotations: A feasibility study. Database 2011. doi: 10.1093/database/bar004.
Bluhm, Wolfgang F., Bojan Beran, Chunxiao Bi, Dimitris Dimitropoulos, Andreas Prlić, Gregory B. Quinn, Peter W. Rose, Chaitali Shah, Jasmine Young, Benjamin Yukich, Helen M. Berman & Philip E. Bourne. 2011. Quality assurance for the query and distribution systems of the RCSB protein data bank. Database 2011. doi: 10.1093/database/bar003.
Articles from DNA Research
Chi, Yunhua, Yansong Cheng, Jeevanandam Vanitha, Nadimuthu Kumar, Rengasamy Ramamoorthy, Srinivasan Ramachandran & Shu-Ye Jiang. 2011. Expansion mechanisms and functional divergence of the glutathione s-transferase family in sorghum and other higher plants. DNA Research 18(1). 1–16.
Le, Dung Tien, Rie Nishiyama, Yasuko Watanabe, Keiichi Mochida, Kazuko Yamaguchi-Shinozaki, Kazuo Shinozaki & Lam-Son Phan Tran. 2011. Genome-wide expression profiling of soybean two-component system genes in soybean root and shoot tissues under dehydration stress. DNA Research 18(1). 17–29.
Kenny, Elaine M., Paul Cormican, William P. Gilks, Amy S. Gates, Colm T. O’Dushlaine, Carlos Pinto, Aiden P. Corvin, Michael Gill & Derek W. Morris. 2011. Multiplex target enrichment using DNA indexing for ultra-high throughput SNP detection. DNA Research 18(1). 31–38.
Satbhai, Santosh B., Takafumi Yamashino, Ryo Okada, Yuji Nomoto, Takeshi Mizuno, Yuki Tezuka, Tomonori Itoh, Mitsuru Tomita, Susumu Otsuki & Setsuyuki Aoki. 2011. Pseudo-response regulator (PRR) homologues of the moss physcomitrella patens: Insights into the evolution of the PRR family in land plants. DNA Research 18(1). 39–52.
Garg, Rohini, Ravi K. Patel, Akhilesh K. Tyagi & Mukesh Jain. 2011. De novo assembly of chickpea transcriptome using short reads for gene discovery and marker identification. DNA Research 18(1). 53–63.
Sato, Shusei, Hideki Hirakawa, Sachiko Isobe, Eigo Fukai, Akiko Watanabe, Midori Kato, Kumiko Kawashima, Chiharu Minami, Akiko Muraki, Naomi Nakazaki, Chika Takahashi, Shinobu Nakayama, Yoshie Kishida, Mitsuyo Kohara, Manabu Yamada, Hisano Tsuruoka, Shigemi Sasamoto, Satoshi Tabata, Tomoyuki Aizu, Atsushi Toyoda, Tadasu Shin-i, Yohei Minakuchi, Yuji Kohara, Asao Fujiyama, Suguru Tsuchimoto, Shin’ichiro Kajiyama, Eri Makigano, Nobuko Ohmido, Nakako Shibagaki, Joyce A. Cartagena, Naoki Wada, Tsutomu Kohinata, Alipour Atefeh, Shota Yuasa, Sachihiro Matsunaga & Kiichi Fukui. 2011. Sequence analysis of the genome of an oil-bearing tree, Jatropha Curcas L. DNA Research 18(1). 65–76.
Gourcilleau, Delphine, Catherine Lenne, Claudia Armenise, Bruno Moulia, Jean-Louis Julien, Gisèle Bronner & Nathalie Leblanc-Fournier. 2011. Phylogenetic study of plant q-type C2H2 zinc finger proteins and expression analysis of poplar genes in response to osmotic, cold, and mechanical stresses. DNA Research 18(2). 77–92.
Doorduin, Leonie, Barbara Gravendeel, Youri Lammers, Yavuz Ariyurek, Thomas Chin-A-Woeng & Klaas Vrieling. 2011. The complete chloroplast genome of 17 individuals of pest species Jacobaea vulgaris : SNPs, microsatellites and barcoding markers for population and phylogenetic studies. DNA Research 18(2). 93–105.
Sayama, Takashi, Tae-Young Hwang, Kunihiko Komatsu, Yoshitake Takada, Masakazu Takahashi, Shin Kato, Hiroko Sasama, Ayako Higashi, Yumi Nakamoto, Hideyuki Funatsuki & Masao Ishimoto. 2011. Development and application of a whole-genome simple sequence repeat panel for high-throughput genotyping in soybean. DNA Research 18(2). 107–115.
Lamprea-Burgunder, Estelle, Philipp Ludin & Pascal Mäser. 2011. Species-specific typing of DNA based on palindrome frequency patterns. DNA Research 18(2). 117–124.
Bailey , Stephen . 2011 . Academic writing: A handbook for international students , 3rd edn . Oxford : Routledge . Search in Google Scholar
Bawarshi , Anis S. & Mary Jo Reiff . 2010 . Genre: An introduction to history, theory, research, and pedagogy . West Lafayette, IN : Parlor Press, WAC Clearinghouse . Search in Google Scholar
Breakthrough of the year . 2014 . http://news.sciencemag.org/scientific-community/2014/12/breakthrough-year-top-10-scientific-achievements-2014 (accessed 22 December 2014 ). Search in Google Scholar
Christie , Frances . 2007 . Ongoing dialogue: Functional linguistic and Bernstein sociological perspectives on education . In Frances Christie & James R. Martin (eds.), Language, knowledge, and pedagogy: Functional linguistic and sociological perspectives , 3 – 13 . London : Continuum . Search in Google Scholar
Craswell , Gail & Megan Poore . 2012 . Writing for academic success , 2nd edn . London : Sage . Search in Google Scholar
Daneš , František . 1970 . One instance of Prague school methodology: Functional analysis of utterance and text . In P. L. Garvin (ed.), Method and theory in linguistics , 132 – 140 . The Hague : Mouton . 10.1515/9783110872521.132 Search in Google Scholar
Daneš , František . 1974 . Functional sentence perspective and the organization of the text . In František Daneš (ed.), Papers on functional sentence perspective , 106 – 128 . The Hague : Mouton . 10.1515/9783111676524.106 Search in Google Scholar
Dubois , Betty Lou . 1987 . A reformulation of thematic progression typology . Text 7 ( 2 ). 89 – 116 . 10.1515/text.1.1987.7.2.89 Search in Google Scholar
First-time enrollment of international graduate students up 8 percent . 8 November 2012 . http://www.cgsnet.org/first-time-enrollment-international-graduate-students-8-percent (accessed 12 May 2013 ). Search in Google Scholar
Fries , Peter H. & Gillian Francis . 1992 . Exploring theme: Problems for research . Occasional Papers in Systemic Linguistics 6 . 45 – 59 . Search in Google Scholar
Halliday , Michael A. K . 1994 . An introduction to functional grammar , 2nd edn . London : Arnold . Search in Google Scholar
Halliday , Michael A. K. & Ruqaiya Hasan . 1976 . Cohesion in English . London : Longman . Search in Google Scholar
Halliday , Michael A. K. & Christian M. I. M Matthiessen . 2014 . Halliday’s introduction to functional grammar , 4th edn . Oxfordshire : Routledge . 10.4324/9780203783771 Search in Google Scholar
Harris , David P . 1990 . The use of “organizing sentences” in the structure of paragraphs in science textbooks . In Ulla Connor & Ann M. Johns (eds.), Coherence in writing: Research and pedagogical perspectives , 67 – 86 . Alexandria, VA : TESOL . Search in Google Scholar
Harris , Michael . 2012 . Do androids prove theorems in their sleep? In Apostolos Doxiadis & Barry Mazur (eds.), Circles disturbed: The interplay of mathematics and narrative , 130 – 182 . Princeton, NJ : Princeton University Press . 10.1515/9781400842681.130 Search in Google Scholar
Hasan , Ruqaiya . 1984 . Coherence and cohesive harmony . In James Flood (ed.), Understanding reading comprehension , 181 – 219 . Newark, DE : International Reading Association . Search in Google Scholar
Hunt , Kellogg . 1965 . Grammatical structures written at three grade levels . Champaign, IL : National Council of Teachers of English . Search in Google Scholar
Hyland , Ken . 2000 . Disciplinary discourses: Social interactions in academic writing . London : Longman . Search in Google Scholar
Hyland , Ken . 2008 . As can be seen: Lexical bundles and disciplinary variation . English for Specific Purposes 27 ( 1 ). 4 – 21 . 10.1016/j.esp.2007.06.001 Search in Google Scholar
Hyland , Ken & Marina Bondi (eds.). 2006 . Academic discourse across disciplines . Frankfurt : Peter Lang . 10.3726/978-3-0351-0446-2 Search in Google Scholar
Jalilifar , A. R . 2010 . The status of theme in applied linguistics articles . Asian ESP Journal 6 ( 2 ). 7 – 39 . Search in Google Scholar
Journal citation reports . 2013 . http://wokinfo.com/products_tools/analytical/jcr/ (accessed 22 December 2014 ). Search in Google Scholar
Kanoksilapatham , Budsaba . 2005 . Rhetorical structure of biochemistry research articles . English for Specific Purposes 24 ( 3 ). 269 – 292 . 10.1016/j.esp.2004.08.003 Search in Google Scholar
Laurens , Amanda . 2007 . Scientific writing skills: Guidelines for writing theses and dissertations . Stellenbosch : Sun Press . 10.18820/9781920689667 Search in Google Scholar
Leong , Ping Alvin . 2004 . Theme and rheme: An alternative account . Bern : Peter Lang . Search in Google Scholar
Leong , Ping Alvin . 2005 . Talking themes: The thematic structure of talk . Discourse Studies 7 ( 6 ). 701 – 732 . 10.1177/1461445605055423 Search in Google Scholar
Martin , James R . 1992 . English text: System and structure . Amsterdam & Philadelphia : John Benjamins . 10.1075/z.59 Search in Google Scholar
McCabe , Anne M . 1999 . Theme and thematic patterns in Spanish and English history texts . Birmingham : Aston University PhD thesis . Search in Google Scholar
North , Sarah . 2005 . Different values, different skills? A comparison of essay writing by students from arts and science backgrounds . Studies in Higher Education 30 ( 5 ). 517 – 533 . Search in Google Scholar
Nwogu , Kevin & Thomas Bloor . 1991 . Thematic progression in professional and popular medical texts . In Eija Ventola (ed.), Functional and systemic linguistics: Approaches and uses , 369 – 384 . Berlin & New York : Walter de Gruyter . 10.1515/9783110883527.369 Search in Google Scholar
Parsons , Gerald . 1991 . Cohesion coherence: Scientific texts . In Eija Ventola (ed.), Functional and systemic linguistics: Approaches and uses , 415 – 429 . Berlin & New York : Walter de Gruyter . 10.1515/9783110883527.415 Search in Google Scholar
Phakiti , Aek & Lulu Li . 2011 . General academic difficulties and reading and writing difficulties among Asian ESL postgraduate students in TESOL at an Australian university . RELC Journal 42 ( 3 ). 227 – 264 . 10.1177/0033688211421417 Search in Google Scholar
Postgraduate research program . 2013 . http://sydney.edu.au/stuserv/learning_centre/pgradjun+jul.shtml (accessed 9 May 2013 ). Search in Google Scholar
Rogers , Sandra H . 2004 . Evaluating textual coherence: A case study of university business writing by EFL and native English-speaking students in New Zealand . RELC Journal 35 ( 2 ). 135 – 147 . 10.1177/003368820403500203 Search in Google Scholar
Samraj , B . 2005 . An exploration of a genre set: Research article abstracts and introductions in two disciplines . English for Specific Purposes 24 . 141 – 156 . 10.1016/j.esp.2002.10.001 Search in Google Scholar
Swales , John M . 1981 . Aspects of article introductions . Birmingham : Aston University . Search in Google Scholar
Swales , John M . 1990 . Genre analysis: English in academic and research settings . Cambridge : Cambridge University Press . Search in Google Scholar
Swales , John M. & Christine B Feak . 2004 . Academic writing for graduate students: Essential tasks and skills , 2nd edn . Ann Arbor, MI : University of Michigan Press . Search in Google Scholar
Williams , Ian A . 1999 . Results sections of medical research articles: Analysis of rhetorical categories for pedagogical purposes . English for Specific Purposes 18 ( 4 ). 347 – 366 . 10.1016/S0889-4906(98)00003-9 Search in Google Scholar
Williams , Joseph M . 2000 . Style: Ten lessons in clarity and grace , 6th edn . New York : Longman . Search in Google Scholar
Williams , Ian A . 2009 . Discourse style and theme–rheme progression in biomedical research article discussions . Languages in Contrast 9 ( 2 ). 225 – 266 . 10.1075/lic.9.2.03wil Search in Google Scholar
Yates , Simeon J. , Noel Williams & Ann-Florence Dujardin . 2005 . Writing geology: Key communication competencies for geoscience . Planet 15 . 36 – 41 . 10.11120/plan.2005.00150036 Search in Google Scholar
Zmrzlá , Petra . 2013 . Co-referential cohesive chains in research articles . Brno: Masaryk University PhD dissertation. Search in Google Scholar
©2015 by De Gruyter Mouton
- X / Twitter
Supplementary Materials
Please login or register with De Gruyter to order this product.
Journal and Issue
Articles in the same issue.
Purdue Online Writing Lab Purdue OWL® College of Liberal Arts
Welcome to the Purdue Online Writing Lab
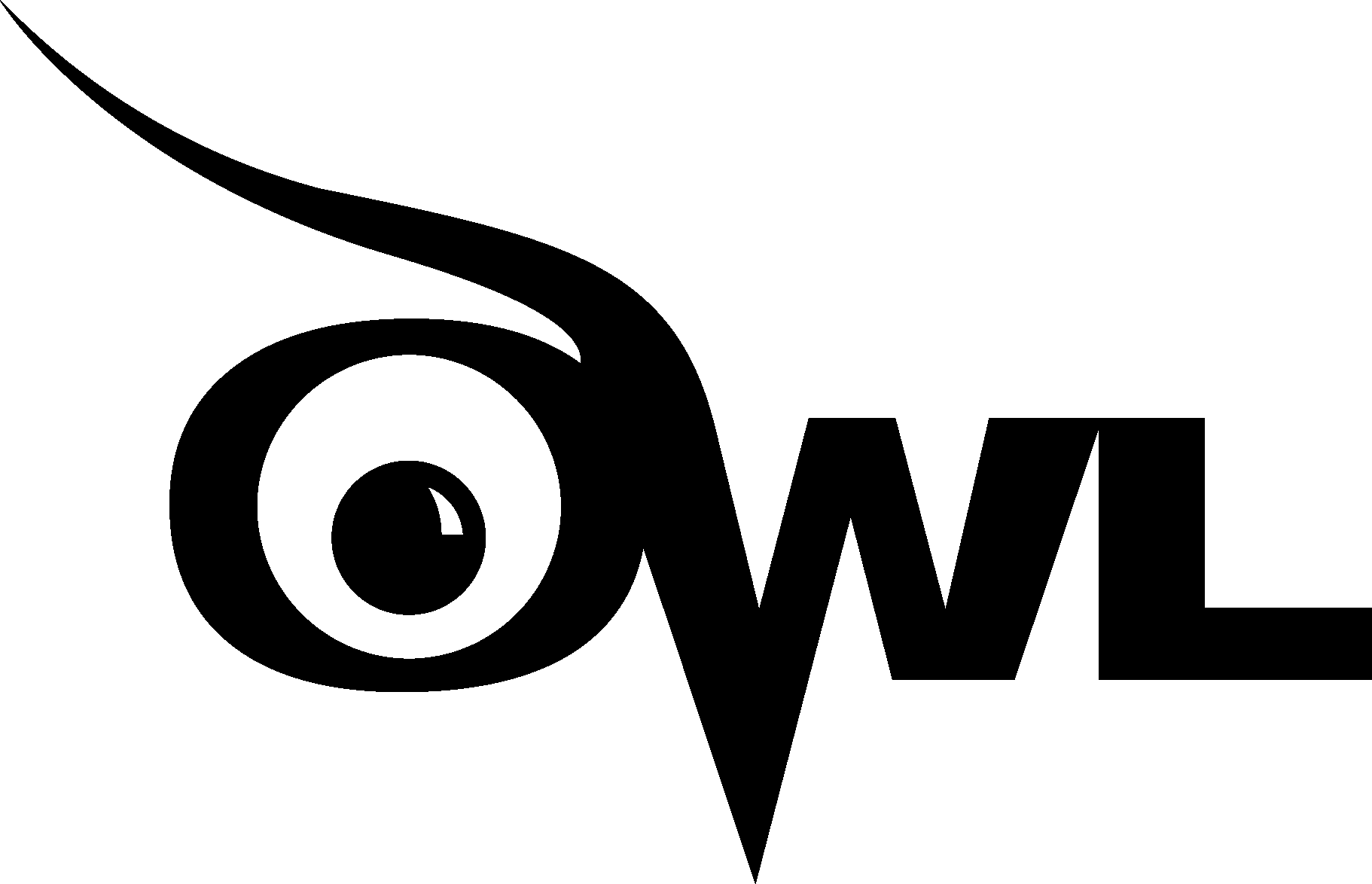
Welcome to the Purdue OWL
This page is brought to you by the OWL at Purdue University. When printing this page, you must include the entire legal notice.
Copyright ©1995-2018 by The Writing Lab & The OWL at Purdue and Purdue University. All rights reserved. This material may not be published, reproduced, broadcast, rewritten, or redistributed without permission. Use of this site constitutes acceptance of our terms and conditions of fair use.
The Online Writing Lab at Purdue University houses writing resources and instructional material, and we provide these as a free service of the Writing Lab at Purdue. Students, members of the community, and users worldwide will find information to assist with many writing projects. Teachers and trainers may use this material for in-class and out-of-class instruction.
The Purdue On-Campus Writing Lab and Purdue Online Writing Lab assist clients in their development as writers—no matter what their skill level—with on-campus consultations, online participation, and community engagement. The Purdue Writing Lab serves the Purdue, West Lafayette, campus and coordinates with local literacy initiatives. The Purdue OWL offers global support through online reference materials and services.
A Message From the Assistant Director of Content Development
The Purdue OWL® is committed to supporting students, instructors, and writers by offering a wide range of resources that are developed and revised with them in mind. To do this, the OWL team is always exploring possibilties for a better design, allowing accessibility and user experience to guide our process. As the OWL undergoes some changes, we welcome your feedback and suggestions by email at any time.
Please don't hesitate to contact us via our contact page if you have any questions or comments.
All the best,
Social Media
Facebook twitter.
REVIEW article
The linkage between global financial crises, corporate social responsibility and climate change: unearthing research opportunities through bibliometric reviews provisionally accepted.
- 1 Kotebe University of Education, Ethiopia
- 2 University of Johannesburg, South Africa
The final, formatted version of the article will be published soon.
Financial matters, corporate social responsibility (CSR), climate change, and other sustainable solutions all work in tandem. In order to provide a thorough understanding of the integration between various components during crises, it is necessary to provide knowledge of the interaction between financial, societal, and environmental aspects. In order to accomplish this, hundreds of papers were examined and presented using bibliometric analysis. The study demonstrated that, when examining financial crises in relation to CSR and climate change, sustainability issues were clearly examined. Sustainability, environmental economics, governance approaches, and sustainable development are some of the main issues in this comprehensive subject. Besides, the emerging topics that need more research include organizational resilience, global financial crises, and sustainable performance, while there are no specific themes developed in the subject matter that integrate financial crises, CSR, and climate change. Thus, future researchers need to provide new insights on the integration of these concepts.
Keywords: Financial crises, CSR, Climate Change, sustainability, scientific mapping, thematic
Received: 19 Feb 2024; Accepted: 08 May 2024.
Copyright: © 2024 Chebo, Dhliwayo and Batu. This is an open-access article distributed under the terms of the Creative Commons Attribution License (CC BY) . The use, distribution or reproduction in other forums is permitted, provided the original author(s) or licensor are credited and that the original publication in this journal is cited, in accordance with accepted academic practice. No use, distribution or reproduction is permitted which does not comply with these terms.
* Correspondence: Mx. Abdella K. Chebo, Kotebe University of Education, Addis Ababa, Ethiopia
People also looked at
This paper is in the following e-collection/theme issue:
Published on 8.5.2024 in Vol 26 (2024)
Characteristic Changes of the Stance-Phase Plantar Pressure Curve When Walking Uphill and Downhill: Cross-Sectional Study
Authors of this article:

Original Paper
- Christian Wolff 1 , MSc ;
- Patrick Steinheimer 2 , MSc ;
- Elke Warmerdam 3 , MSc, PhD ;
- Tim Dahmen 1 , MSc, PhD ;
- Philipp Slusallek 1 , MSc, PhD ;
- Christian Schlinkmann 1 , MSc ;
- Fei Chen 1 , MSc ;
- Marcel Orth 2 , MD, PhD ;
- Tim Pohlemann 2 , MD, PhD ;
- Bergita Ganse 2, 3 , MD, PhD
1 German Research Center for Artificial Intelligence (DFKI), Saarbrücken, Germany
2 Department of Trauma, Hand and Reconstructive Surgery, Departments and Institutes of Surgery, Saarland University, Homburg/Saar, Germany
3 Innovative Implant Development (Fracture Healing), Departments and Institutes of Surgery, Saarland University, Homburg/Saar, Germany
Corresponding Author:
Bergita Ganse, MD, PhD
Innovative Implant Development (Fracture Healing)
Departments and Institutes of Surgery
Saarland University
Kirrberger Straße 1
Building 57
Homburg/Saar, 66421
Phone: 49 684116 ext 31570
Email: [email protected]
Background: Monitoring of gait patterns by insoles is popular to study behavior and activity in the daily life of people and throughout the rehabilitation process of patients. Live data analyses may improve personalized prevention and treatment regimens, as well as rehabilitation. The M-shaped plantar pressure curve during the stance phase is mainly defined by the loading and unloading slope, 2 maxima, 1 minimum, as well as the force during defined periods. When monitoring gait continuously, walking uphill or downhill could affect this curve in characteristic ways.
Objective: For walking on a slope, typical changes in the stance phase curve measured by insoles were hypothesized.
Methods: In total, 40 healthy participants of both sexes were fitted with individually calibrated insoles with 16 pressure sensors each and a recording frequency of 100 Hz. Participants walked on a treadmill at 4 km/h for 1 minute in each of the following slopes: −20%, −15%, −10%, −5%, 0%, 5%, 10%, 15%, and 20%. Raw data were exported for analyses. A custom-developed data platform was used for data processing and parameter calculation, including step detection, data transformation, and normalization for time by natural cubic spline interpolation and force (proportion of body weight). To identify the time-axis positions of the desired maxima and minimum among the available extremum candidates in each step, a Gaussian filter was applied (σ=3, kernel size 7). Inconclusive extremum candidates were further processed by screening for time plausibility, maximum or minimum pool filtering, and monotony. Several parameters that describe the curve trajectory were computed for each step. The normal distribution of data was tested by the Kolmogorov-Smirnov and Shapiro-Wilk tests.
Results: Data were normally distributed. An analysis of variance with the gait parameters as dependent and slope as independent variables revealed significant changes related to the slope for the following parameters of the stance phase curve: the mean force during loading and unloading, the 2 maxima and the minimum, as well as the loading and unloading slope (all P <.001). A simultaneous increase in the loading slope, the first maximum and the mean loading force combined with a decrease in the mean unloading force, the second maximum, and the unloading slope is characteristic for downhill walking. The opposite represents uphill walking. The minimum had its peak at horizontal walking and values dropped when walking uphill and downhill alike. It is therefore not a suitable parameter to distinguish between uphill and downhill walking.
Conclusions: While patient-related factors, such as anthropometrics, injury, or disease shape the stance phase curve on a longer-term scale, walking on slopes leads to temporary and characteristic short-term changes in the curve trajectory.
Introduction
Long-term monitoring of gait patterns and plantar-pressure distributions via insoles are increasingly popular ways to study behavior and activity in the field and in the everyday lives of people and patients, including healing, personalized prevention, and treatment or disease progression [ 1 - 3 ]. In recent years, the usability of instrumented insoles for gait analyses has increased. Several technical issues could be resolved, including calibration, hysteresis and drift, durability, usability, limited energy supply and battery life, data storage capacity, and the restriction to low sample frequencies associated with higher error rates, that is, when force peaks are missed [ 3 - 5 ]. The usability of instrumented insoles is currently still limited by difficulties in data analysis. Advanced algorithms and tools are needed and currently developed to be able to draw meaningful conclusions from such insole gait data [ 6 , 7 ]. When analyzing long-term field data and developing smart health care innovations, automated data annotation is desirable to determine and quantify the activities a person has conducted. Ideally, the activity type can be determined algorithmically from the plantar pressure data alone.
Characteristic gait changes have been reported for walking on slopes, such as changes in the contribution of the ankle joint to leg work [ 8 ]. In addition, uphill walking on a treadmill increases hip and knee flexion angles during the stance phase, as well as the forward tilt of the thorax [ 9 ]. Furthermore, a decrease in dorsiflexion was observed during downhill walking at initial contact, in midstance, and during the second half of the swing phase [ 9 ]. During uphill walking with increasing inclination, more positive joint work was identified for the ankle and hip joint, while negative joint work increased during downhill walking [ 10 ]. Older individuals were shown to have a disproportionate recruitment of hip muscles and smaller increases in activity of the medial gastrocnemius muscle with steeper uphill slopes than younger adults, resulting in difficulty walking on steep slopes [ 11 ].
The M-shaped curve of ground reaction forces or plantar pressure during the stance phase is mainly defined by the loading and unloading slope, 2 maxima, 1 minimum, as well as the force during defined periods [ 12 ]. When monitoring gait continuously via insoles, walking uphill or downhill on a slope could affect the gait cycle curve in characteristic ways. If these typical changes were known, one could correct for such confounders when analyzing insole data. We hypothesized that walking on a slope generates typical changes in the plantar pressure stance phase curve that vary between uphill and downhill walking.
Study Design
This study is part of the project Smart Implants 2.0—Weight-bearing and Gait Observation for Early Monitoring of Fracture Healing and Individualized Therapy after Trauma, funded by the Werner Siemens Foundation. It was registered in the German Clinical Trials Register (DRKS00025108).
Ethical Considerations
Ethical approval was obtained from the Institutional Review Board of Saarland Medical Board (Ärztekammer des Saarlandes, 30/21).
Data Collection
Inclusion criteria were the ability to walk on a treadmill, and aged 18 years and older. Exclusion criteria were aged under 18 years, use of walking aids, inability to give consent, pregnancy, immobility, and previous injury of the lower legs or pelvis. The aim was to collect data from healthy volunteers.
The healthy participants of both sexes (none of them identified as diverse) were fitted with individually calibrated OpenGO insoles (Moticon GmbH) with 16 pressure sensors in each insole to be used in regular running shoes. Calibration to the individual body weight was conducted using the Moticon OpenGO app by letting the participants walk and shift their body weight in a standardized way. The insole size was selected to fit the individual participant’s shoe size. Measurements were conducted with a recording frequency of 100 Hz in the record mode of the device. Raw data were exported for analyses. The participants walked on a treadmill at 4 km/h (Mercury, HP Cosmos) for 1 minute while insole data were collected with 3-minute breaks for recovery. Recordings were obtained for slopes of −20%, −15%, −10%, −5%, 0%, 5%, 10%, 15%, and 20%. The participants were asked to walk for 1 minute straight, and recording was only commenced when the walking was already in progress to avoid bias by including altered steps upon gait initiation.
Data Processing
The pressure readings of the force sensors in the insole device yield a weighted sum as a total vertical ground reaction force reading. To compute the force, every summand is weighted by its sensor area and a respective scaling factor accounting for the sensor’s surrounding area, as well as gaps between sensors that depend on the insole size. This process is conducted by the Moticon software as an automated processing step before file export. Insole data were exported as described previously [ 13 , 14 ]. A custom-developed data platform was then used for further processing and parameter calculation, in which step detection was conducted as follows. The stance phases were identified and extracted from the time series data by considering any activity with consecutive force readings above 30 N. A tolerance of up to 3 missing values was implemented to account for possible recording issues. Any activity with a duration of less than 300 milliseconds or more than 2000 milliseconds was discarded. Both the force and time axes were normalized. Force readings were transformed from Newton to a proportion of the body weight of the respective participant. Of note, as plantar pressure was measured instead of weight, due to acceleration, values regularly exceeded the body weight for peak load-bearing instances. Normalizing the time axis was more complex, as the lack of a fixed cadence resulted in varying step lengths and thus differing numbers of true measurement points for each step. Therefore, a natural cubic spline interpolation was conducted on the original raw data. Based on the resulting curve for each stance phase, 100 equidistant samples were taken, resulting in an interpolated force measurement point for every 1% of the overall stance phase length. This approach accounted for the lower recording frequency and higher sensor noise inherent to the insoles when compared with other gait measuring devices, such as sensor-equipped treadmills or force plates. Parameters that describe the trajectory of the stance phase curve are usually based on or derived from the characteristic local extrema, that is, the first and second force peak and the local minimum in-between force peaks. These maxima and the minimum are used as parameters themselves to describe the curve trajectory [ 13 ]. Sensor jitter may lead to the existence of multiple ambiguous candidates for the named extrema. As a solution to this, a Gaussian filter was applied to the original raw data in a repetition of the normalization process. The applied filtering strategy (σ=3, kernel size 7) was chosen to prioritize the elimination of extrema ambiguity at the expense of signal precision. This can result in overcorrection in areas with higher signal volatility, mostly at the start and end of the stance phase. Hence, to avoid loss of high-frequency detail, the filtered and normalized curve was not used for parameter analysis, but only to determine unambiguous time-axis positions (indices) for the extremum candidates. These indices were then reapplied to the nonfiltered, normalized data to identify the corresponding plantar pressure measurement closest to the original raw data. In case the use of the filtered data still led to inconclusive extremum candidates, the following additional detection strategies were applied in the named order: (1) time plausibility: extremum candidates occurring within the first or last 10 indices (first/last 10% of overall time span) were eliminated; (2) maximum or minimum-pool filtering: should multiple extremum candidates occur within a pool size of 5 indices (equals to 5% of overall time span), the candidate with the highest or lowest force value was chosen; (3) monotony-check: in case of multiple remaining extremum candidates, candidates where the curve did not display a strict monotonous decrease or increase in both directions within 5 indices each were eliminated; and (4) monotony grace: in case the monotony check had eliminated too many candidates (less than 2 maximum candidates or less than 1 minimum candidate remaining), the eliminated candidates were reinstated in descending order of their highest achieved monotony distance until the target number of candidates was reached.
After applying these strategies, every stance activity that remained with an irregular amount of unambiguous extremum candidates was removed from the data set. In total, 585 load-bearing events were excluded as not fitting the strict parameter definitions.
For each participant, across the minute of walking all stance phase curves were extracted. The parameters illustrated in Figure 1 were calculated for each stance phase and used to analyze changes in the trajectory of the stance phase curve. To do so, data from both feet were pooled. The curve is mainly described by 2 maxima and a minimum in between the maxima, Fz2 (the first maximum), Fz3 (the minimum), and Fz4 (the second maximum). The mean force over the entire stance phase is referred to as Fmean stance . The mean force between the start of the loading phase and Fz2 is Fmean load . The mean force between Fz2 and Fz4 is Fmean mid . The mean force between Fz4 and the end of the unloading phase is Fmean unload . All these parameters have the unit percent body weight. In addition, the loading and unloading slope have the units percent body weight or percent stance phase duration. The loading slope was computed as the slope of the line defined by the start of the loading phase and the first force reading equal to or higher than 80% of Fz2. The unloading slope was calculated as the slope of the line defined by the first force reading in the unloading phase below 80% of Fz4 and the end of the stance phase event.

Statistical Analyses
Statistical tests were executed with SPSS Statistics (version 29; IBM Corp). Significance was defined as P <.05. The normal distribution of data was tested by the Kolmogorov-Smirnov and Shapiro-Wilk tests. A linear regression analysis of variance was conducted for each of the gait parameters as the dependent variable, with the slope (−20% to 20%) as the independent variable. Mean values and SD are reported. Linear regression slopes are reported for comparability and to allow for correction, even though for some of the parameters other but differing regression types yielded higher R 2 values. The sample size of 40 was an estimate based on what is common in the field, and taking into account the aim to measure a very diverse group of volunteers. An a priori sample size calculation was not conducted due to a lack of comparable data.
Measurements were taken from 40 healthy participants (19 women and 21 men) with an average age of 43.90 (SD 17.30, range 18-87) years. Participant characteristics are summarized in Table 1 . Data were successfully recorded for all of the participants and slope levels, resulting in a complete data set ( Multimedia Appendix 1 ).
Data were normally distributed. Figure 2 visualizes the differences between the analyzed slope values on the stance phase curve. Figure 3 shows the normalized changes in the analyzed parameters with the slope of the treadmill. The analysis of variance revealed significant changes with the slope for Fmean load , Fmean unload , Fz2, Fz3, Fz4, loading and unloading slope (all P <.001). There was no significant correlation of the slope with Fmean stance ( P =.98) and Fmean mid ( P =.13). Other than the other parameters with significant changes related to slope, Fz3 had its peak at horizontal walking and values dropped when walking uphill and downhill alike. Thus, a simultaneous and short-term increase in loading slope and Fmean load combined with a decrease in Fmean unload , Fz2, Fz4, and the unloading slope indicates downhill walking, while the opposite indicates uphill walking. Fz3 is not a suitable parameter to distinguish between uphill and downhill walking, as its value decreases both when walking uphill as well as downhill. Mean values and the SD of the analyzed parameters for each treadmill slope level in absolute values are displayed in Table 2 . Table 3 indicates the linear regression slopes and R 2 -values for each of the curves shown in Figure 3 .

a Fmean stance : the mean force over the entire stance phase.
b Fmean load : the mean force between the start of the loading phase and Fz2.
c Fmean mid : the mean force between Fz2 and Fz4.
d Fmean unload : the mean force between Fz4 and the end of the unloading phase.
e Fz2: the first maximum.
f Fz3: the minimum.
g Fz4: the second maximum.
Principal Results
This study identified characteristic changes when walking with an uphill or downhill slope in insole plantar pressure data of healthy participants. The most pronounced changes with treadmill slope were found in the loading slope of the curve. A typical combination of changes in several parameters was reported that defines uphill and downhill walking and may be used for annotation and correction when analyzing such data. These changes in the trajectory of the force curve with different surface slopes relative to the force vector of Earth’s gravity are related to changes in plantar load distribution. When walking downhill, Fz2 was found to be higher compared to when walking uphill, which is caused by the more pronounced force transfer through the heel of the foot, followed by a lower second maximum due to the even lower surface at push-off.
While patient-related factors, such as curve characteristics related to body size, muscle power, degenerative disease, etc, would remain constant throughout an insole measurement, fatigue-related changes [ 15 ] may increasingly appear and then stay toward the later stages of a recording of a walking bout. Additionally, age, body height, body weight, BMI, and handgrip strength were shown to cause characteristic changes in the plantar pressure force curve, that would usually only change on a long-term scale [ 16 ]. In contrast, as shown in the present data set, walking on slopes leads to temporary and characteristic changes in specific properties of the stance-phase curve. Changes over time in the identified parameters should thus be considered and correctly interpreted when studying long-term field gait data collected via insoles. To analyze the healing process, that is, after an injury, slow changes in parameters would be expected, and a trend toward what is considered normal over several weeks [ 17 ]. Short-term changes over minutes or hours would thus not be explainable by the healing progress and should have a different cause. In addition, the asymmetry between the legs should slowly decrease throughout healing [ 18 ]. When walking on a slope, asymmetry could also be affected, if the injury causes increasing problems such as pain when walking uphill or downhill. It is also recommendable to identify the characteristics of walking with walking aids, such as crutches, to be able to classify the nature of the observed changes and the treatment stage better.
Limitations
Effects of walking speed were not analyzed in this study, even though it is known that lower extremity joint loading is affected by varying step length and cadence during graded uphill and downhill walking [ 19 ]. These parameters, however, do not seem to be necessary to successfully annotate gait data obtained by insoles. For participant or patient convenience, it would be desirable if insoles did not need to be combined with further devices or wearables. The present data suggest that at least the identification of walking on slopes does not require further sensors. It is also known that kinematic, kinetic, and electromyographic parameters differ between treadmill walking and overground gait, while spatiotemporal, kinematic, kinetic, electromyographic, and energy consumption outcome measures are largely comparable [ 20 ]. Another limitation of this study is that the parameters analyzed here can only be used when a regular gait curve is present. If this is not the case, other methods need to be applied, that is, machine learning for step detection and segmentation or the analysis of further parameters, possibly slopes and averages, or differences between individual sensors [ 21 ]. Differences between the 16 sensors embedded in each insole were not analyzed in this study and could be assessed in the future, for example, to distinguish between ground types (gravel, sand, etc). Another limitation is that the present characteristic changes that were assessed in healthy participants may differ for patients with gait disorders, depending on their disease or injury type. It will therefore be important to collect longitudinal data on different slopes from patients with defined diseases and injuries throughout the healing process or throughout different disease stages. These studies would serve to identify if the reported findings are valid also for patients, and for which patient groups this is true.
Use of Wearables in Patients
The insole technology and present data may be valuable in real-world settings when investigating changes in mechanical properties during walking, that is, in occupational health research, sport and exercise science, for urban planning, and to plan inclusive architecture. For instance, the global average slope of urban areas is about 3.70° [ 22 ]. Wearables such as pressure insoles are increasingly used to study gait and movement, as well as for fall detection, fall classification, and fall risk assessment in the daily life of patients, and furthermore for lifestyle and health monitoring [ 1 , 3 , 23 - 27 ]. Long-term monitoring, especially if combined with additional sensors, may produce large amounts of data that require advanced strategies for analyses. Apart from regression statistics, among the options is the use of machine learning algorithms trained with annotated data for pattern recognition [ 24 , 26 ]. For longer-term monitoring of patients, it would be desirable if such algorithms were trained to identify various key activities of daily life that might indicate the level of healing progress. For example, when a patient with a tibial fracture is capable of cycling again, this is likely an indication for advances in the healing process. It would also be of interest to identify risky behavior, possibly leading to excessive forces, and to warn the patient by giving, for example, an audible or haptic warning signal. To guarantee meaningful data interpretation, machine learning may be combined with conventional regression-based analyses, such as the ones proposed in this paper to best tackle data complexity. Furthermore, prediction algorithms could be implemented for falls and diseases that enable more refined individual recommendations. Ideally, such interventions would be based on live data analyses. Limitations in the computing power of small wearable devices can increasingly be mitigated by both algorithmic optimization techniques in machine learning, such as dimensionality reduction, reservoir computing, and network pruning, as well as hardware innovations [ 27 , 28 ]. In the near future, such advances will likely allow real-time feedback based on data from various sources combined [ 29 , 30 ]. Alternatively, extracting decision-making systems (symbolic artificial intelligence), such as threshold-based methods, might offer an immediate route to real-time feedback.
Sensors in Orthoses and Implants
Apart from insoles, very similar data might be collected from mechanical sensors embedded in orthoses [ 31 ] or implants [ 32 ]. Potentially, walking on a slope in these recordings changes the data in similar ways as described here. It would be highly desirable if patients did not need to use separate wearables such as insoles anymore, but if orthoses and implants had sensors embedded, not only to monitor healing progress but also to identify healing problems or complications and the need for surgical revision [ 33 ]. If similar load data could be collected by sensors in artificial hip or knee joints, or potentially even by plates or nails that stabilize bone fractures, recovery regimen could be monitored continuously and advice given on time [ 34 ]. Alarms could go off if forces exceeded certain thresholds or if live pattern analyses revealed unfavorable patterns known to be associated with exceeding forces or problems. As these developments seem to have a high potential with regard to rehabilitation and postoperative treatment, data analyses of insole data should be further studied and ideally, details such as algorithms and characteristics should be published to enable for the further development and widespread application of the named interventions.
Conclusions
Characteristic changes in the plantar-pressure stance phase curve were identified, which reflect uphill and downhill walking. Automated annotation and continuous analyses of gait data via wearables could enable improved rehabilitation and feedback systems for prevention and treatment. A combination of traditional regression statistics embedded in heuristics combined with artificial intelligence methods may yield the best results.
Acknowledgments
The Werner Siemens Foundation (project Smart Implants 2.0) funded this work. The authors would like to acknowledge the help of Aynur Gökten and Jacqueline Orth during the measurements, as well as the help of Lisa-Marie Jost in designing Figure 1 .
Authors' Contributions
CW contributed to the data processing platform, data analysis, methods, and Figure 2 . P Steinheimer conducted the measurements. BG contributed to the idea; ran the statistical analyses; interpreted the data; made the tables; and drafted, submitted, and revised this paper. TD, CS, and FC took part in the data platform implementation. EW, TD, P Slusallek, CS, FC, MO, and TP helped with data interpretation. All authors have contributed to this paper’s drafting and revision, and read and approved the submitted version of this paper.
Conflicts of Interest
TP is President and Board Member of the AO-Foundation, Switzerland, and Extended Board Member of the German Society of Orthopedic Trauma Surgery (DGU), the German Society of Orthopedic Surgery and Traumatology (DGOU), and the German Society of Surgery (DGCH). TP is also the speaker of the Medical Advisory Board of the German Ministry of Defence. The other authors do not have a conflict of interest.
- Braun BJ, Veith NT, Rollmann M, Orth M, Fritz T, Herath SC, et al. Weight-bearing recommendations after operative fracture treatment-fact or fiction? Gait results with and feasibility of a dynamic, continuous pedobarography insole. Int Orthop. 2017;41(8):1507-1512. [ CrossRef ] [ Medline ]
- Ramirez-Bautista JA, Huerta-Ruelas JA, Chaparro-Cardenas SL, Hernandez-Zavala A. A review in detection and monitoring gait disorders using in-shoe plantar measurement systems. IEEE Rev Biomed Eng. 2017;10:299-309. [ CrossRef ] [ Medline ]
- Subramaniam S, Majumder S, Faisal AI, Deen MJ. Insole-based systems for health monitoring: current solutions and research challenges. Sensors (Basel). 2022;22(2):438. [ FREE Full text ] [ CrossRef ] [ Medline ]
- Elstub LJ, Grohowski LM, Wolf DN, Owen MK, Noehren B, Zelik KE. Effect of pressure insole sampling frequency on insole-measured peak force accuracy during running. J Biomech. 2022;145:111387. [ CrossRef ] [ Medline ]
- North K, Kubiak EN, Hitchcock RW. Sensor packaging design for continuous underfoot load monitoring. Biomed Microdevices. 2012;14(1):217-224. [ CrossRef ] [ Medline ]
- Anderson W, Choffin Z, Jeong N, Callihan M, Jeong S, Sazonov E. Empirical study on human movement classification using insole footwear sensor system and machine learning. Sensors (Basel). 2022;22(7):2743. [ FREE Full text ] [ CrossRef ] [ Medline ]
- Chatzaki C, Skaramagkas V, Kefalopoulou Z, Tachos N, Kostikis N, Kanellos F, et al. Can gait features help in differentiating Parkinson's disease medication states and severity levels? A machine learning approach. Sensors (Basel). 2022;22(24):9937. [ FREE Full text ] [ CrossRef ] [ Medline ]
- Montgomery JR, Grabowski AM. The contributions of ankle, knee and hip joint work to individual leg work change during uphill and downhill walking over a range of speeds. R Soc Open Sci. 2018;5(8):180550. [ FREE Full text ] [ CrossRef ] [ Medline ]
- Strutzenberger G, Leutgeb L, Claußen L, Schwameder H. Gait on slopes: differences in temporo-spatial, kinematic and kinetic gait parameters between walking on a ramp and on a treadmill. Gait Posture. 2022;91:73-78. [ CrossRef ] [ Medline ]
- Alexander N, Strutzenberger G, Ameshofer LM, Schwameder H. Lower limb joint work and joint work contribution during downhill and uphill walking at different inclinations. J Biomech. 2017;61:75-80. [ CrossRef ] [ Medline ]
- Franz JR, Kram R. How does age affect leg muscle activity/coactivity during uphill and downhill walking? Gait Posture. 2013;37(3):378-384. [ FREE Full text ] [ CrossRef ] [ Medline ]
- Larsen AH, Puggaard L, Hämäläinen U, Aagaard P. Comparison of ground reaction forces and antagonist muscle coactivation during stair walking with ageing. J Electromyogr Kinesiol. 2008;18(4):568-580. [ CrossRef ] [ Medline ]
- Braun BJ, Veith NT, Hell R, Döbele S, Roland M, Rollmann M, et al. Validation and reliability testing of a new, fully integrated gait analysis insole. J Foot Ankle Res. 2015;8:54. [ FREE Full text ] [ CrossRef ] [ Medline ]
- Stöggl T, Martiner A. Validation of Moticon's OpenGo sensor insoles during gait, jumps, balance and cross-country skiing specific imitation movements. J Sports Sci. 2017;35(2):196-206. [ FREE Full text ] [ CrossRef ] [ Medline ]
- Chardon M, Barbieri FA, Penedo T, Santos PCR, Vuillerme N. The effects of experimentally-induced fatigue on gait parameters during obstacle crossing: a systematic review. Neurosci Biobehav Rev. 2022;142:104854. [ CrossRef ] [ Medline ]
- Wolff C, Steinheimer P, Warmerdam E, Dahmen T, Slusallek P, Schlinkmann C, et al. Effects of age, body height, body weight, body mass index and handgrip strength on the trajectory of the plantar pressure stance-phase curve of the gait cycle. Front Bioeng Biotechnol. 2023;11:1110099. [ FREE Full text ] [ CrossRef ] [ Medline ]
- Agres AN, Alves SA, Höntzsch D, El Attal R, Pohlemann T, Schaser KD, et al. Improved weight bearing during gait at 6 weeks post-surgery with an angle stable locking system after distal tibial fracture. Gait Posture. 2024;107:169-176. [ CrossRef ] [ Medline ]
- Rosenbaum D, Macri F, Lupselo FS, Preis OC. Gait and function as tools for the assessment of fracture repair—the role of movement analysis for the assessment of fracture healing. Injury. 2014;45(Suppl 2):S39-S43. [ CrossRef ] [ Medline ]
- Schwameder H, Lindenhofer E, Müller E. Effect of walking speed on lower extremity joint loading in graded ramp walking. Sports Biomech. 2005;4(2):227-243. [ CrossRef ] [ Medline ]
- Semaan MB, Wallard L, Ruiz V, Gillet C, Leteneur S, Simoneau-Buessinger E. Is treadmill walking biomechanically comparable to overground walking? A systematic review. Gait Posture. 2022;92:249-257. [ CrossRef ] [ Medline ]
- Blades S, Marriott H, Hundza S, Honert EC, Stellingwerff T, Klimstra M. Evaluation of different pressure-based foot contact event detection algorithms across different slopes and speeds. Sensors (Basel). 2023;23(5):2736. [ FREE Full text ] [ CrossRef ] [ Medline ]
- Shi K, Liu G, Zhou L, Cui Y, Liu S, Wu Y. Satellite remote sensing data reveal increased slope climbing of urban land expansion worldwide. Landsc Urban Plan. 2023;235:104755. [ CrossRef ]
- Cates B, Sim T, Heo HM, Kim B, Kim H, Mun JH. A novel detection model and its optimal features to classify falls from low- and high-acceleration activities of daily life using an insole sensor system. Sensors (Basel). 2018;18(4):1227. [ FREE Full text ] [ CrossRef ] [ Medline ]
- Kraus M, Saller MM, Baumbach SF, Neuerburg C, Stumpf UC, Böcker W, et al. Prediction of physical frailty in orthogeriatric patients using sensor insole-based gait analysis and machine learning algorithms: cross-sectional study. JMIR Med Inform. 2022;10(1):e32724. [ FREE Full text ] [ CrossRef ] [ Medline ]
- Subramaniam S, Faisal AI, Deen MJ. Wearable sensor systems for fall risk assessment: a review. Front Digit Health. 2022;4:921506. [ FREE Full text ] [ CrossRef ] [ Medline ]
- Harris EJ, Khoo IH, Demircan E. A survey of human gait-based artificial intelligence applications. Front Robot AI. 2022;8:749274. [ FREE Full text ] [ CrossRef ] [ Medline ]
- Hou CKJ, Behdinan K. Dimensionality reduction in surrogate modeling: a review of combined methods. Data Sci Eng. 2022;7(4):402-427. [ FREE Full text ] [ CrossRef ] [ Medline ]
- Tanaka G, Yamane T, Héroux JB, Nakane R, Kanazawa N, Takeda S, et al. Recent advances in physical reservoir computing: a review. Neural Netw. 2019;115:100-123. [ FREE Full text ] [ CrossRef ] [ Medline ]
- Chiasson-Poirier L, Younesian H, Turcot K, Sylvestre J. Detecting gait events from accelerations using reservoir computing. Sensors (Basel). 2022;22(19):7180. [ FREE Full text ] [ CrossRef ] [ Medline ]
- Zhang Q, Jin T, Cai J, Xu L, He T, Wang T, et al. Wearable triboelectric sensors enabled gait analysis and waist motion capture for IoT-Based smart healthcare applications. Adv Sci (Weinh). 2022;9(4):e2103694. [ FREE Full text ] [ CrossRef ] [ Medline ]
- Moreira L, Figueiredo J, Cerqueira J, Santos CP. A review on locomotion mode recognition and prediction when using active orthoses and exoskeletons. Sensors (Basel). 2022;22(19):7109. [ FREE Full text ] [ CrossRef ] [ Medline ]
- Alves SA, Polzehl J, Brisson NM, Bender A, Agres AN, Damm P, et al. Ground reaction forces and external hip joint moments predict in vivo hip contact forces during gait. J Biomech. 2022;135:111037. [ FREE Full text ] [ CrossRef ] [ Medline ]
- Warmerdam E, Orth M, Pohlemann T, Ganse B. Gait analysis to monitor fracture healing of the lower leg. Bioengineering (Basel). 2023;10(2):255. [ FREE Full text ] [ CrossRef ] [ Medline ]
- Ganse B, Orth M, Roland M, Diebels S, Motzki P, Seelecke S, et al. Concepts and clinical aspects of active implants for the treatment of bone fractures. Acta Biomater. 2022;146:1-9. [ FREE Full text ] [ CrossRef ] [ Medline ]
Abbreviations
Edited by G Eysenbach, T Leung; submitted 24.01.23; peer-reviewed by M Kraus, S Okita; comments to author 21.12.23; revised version received 11.01.24; accepted 17.02.24; published 08.05.24.
©Christian Wolff, Patrick Steinheimer, Elke Warmerdam, Tim Dahmen, Philipp Slusallek, Christian Schlinkmann, Fei Chen, Marcel Orth, Tim Pohlemann, Bergita Ganse. Originally published in the Journal of Medical Internet Research (https://www.jmir.org), 08.05.2024.
This is an open-access article distributed under the terms of the Creative Commons Attribution License (https://creativecommons.org/licenses/by/4.0/), which permits unrestricted use, distribution, and reproduction in any medium, provided the original work, first published in the Journal of Medical Internet Research, is properly cited. The complete bibliographic information, a link to the original publication on https://www.jmir.org/, as well as this copyright and license information must be included.
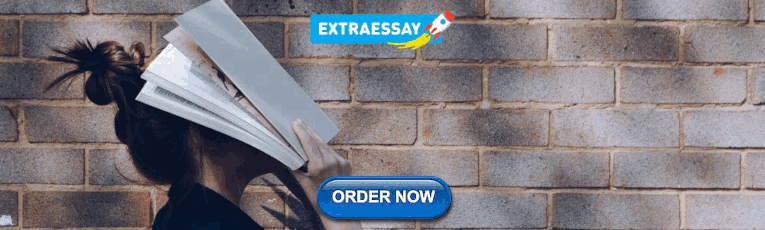
IMAGES
VIDEO
COMMENTS
Sifting through multiple qualitative research papers it can be seen that themes can be single terminologies, a combination of two words or even phrases, like the one suggested by Saldana . There is no singular rule as to what a final theme shall look like. They can be static words like nouns or action words (gerunds ending with 'ing') or ...
How to Do Thematic Analysis | Step-by-Step Guide & Examples. Published on September 6, 2019 by Jack Caulfield.Revised on June 22, 2023. Thematic analysis is a method of analyzing qualitative data.It is usually applied to a set of texts, such as an interview or transcripts.The researcher closely examines the data to identify common themes - topics, ideas and patterns of meaning that come up ...
Thematic analysis is a research method used to identify and interpret patterns or themes in a data set; it often leads to new insights and understanding (Boyatzis, 1998; Elliott, 2018; Thomas, 2006).However, it is critical that researchers avoid letting their own preconceptions interfere with the identification of key themes (Morse & Mitcham, 2002; Patton, 2015).
The term "theme" is a small word, but it can intimidate students when they see it on an assignment or test. To overcome the fear and develop confidence, especially with regard to research papers, understand what the word means and see the parallels with any work, including poems, essays, plays, novels and movies.
Step 1: Find a topic and review the literature. As we mentioned earlier, in a research paper, you, as the researcher, will try to answer a question.More specifically, that's called a research question, and it sets the direction of your entire paper. What's important to understand though is that you'll need to answer that research question with the help of high-quality sources - for ...
Techniques are compared. on six dimensions: (1) appropriateness for data types, (2) required labor, (3) required expertise, (4) stage of analysis, (5) number and types of themes to be gener-. ated ...
Analyzing text involves five complex tasks: (1) discovering themes and subthemes; (2) describing the core and peripheral elements of themes; (3) building hierarchies of themes or codebooks; (4) applying themes— that is, attaching them to chunks of actual text; and (5) linking themes into theoretical models.
Definition: Research Paper is a written document that presents the author's original research, analysis, and interpretation of a specific topic or issue. It is typically based on Empirical Evidence, and may involve qualitative or quantitative research methods, or a combination of both. The purpose of a research paper is to contribute new ...
The most common method of thematic analysis follows a 5 or 6 step process:1) familiarization; 2) coding; 3) generating themes; 4) reviewing themes; 5) defining and naming themes; and 6) reporting. These steps were defined by Braun & Clarke (2008) in this articlewhich is paywalled. The method is suitable for both inductiveand deductivestudies ...
Examples of literature reviews. Step 1 - Search for relevant literature. Step 2 - Evaluate and select sources. Step 3 - Identify themes, debates, and gaps. Step 4 - Outline your literature review's structure. Step 5 - Write your literature review.
Course: Qualitative Research Methods Objectives: To provide students with an experiential understanding of the six steps to conducting a thematic analysis: (1) gaining familiarity with the data ...
There are various approaches to conducting thematic analysis, but the most common form follows a six-step process: Familiarisation. Coding. Generating themes. Reviewing themes. Defining and naming themes. Writing up. This process was originally developed for psychology research by Virginia Braun and Victoria Clarke.
A research paper is a piece of academic writing that provides analysis, interpretation, and argument based on in-depth independent research. Research papers are similar to academic essays, but they are usually longer and more detailed assignments, designed to assess not only your writing skills but also your skills in scholarly research ...
Theme identification is one of the most fundamental tasks in qualitative research. It also is one of the most mysterious. Explicit descriptions of theme discovery are rarely found in articles and reports, and when they are, they are often relegated to appendices or footnotes. Techniques are shared among small groups of social scientists, but ...
Themes are identified with any form of qualitative research method, be it phenomenology, narrative. analysis, grounded theory, thematic analysis or any other form. However, the purpose and process ...
Thematic Analysis is a qualitative research method that involves identifying, analyzing, and interpreting recurring themes or patterns in data. It aims to uncover underlying meanings, ideas, and concepts within the dataset, providing insights into participants' perspectives and experiences.
Although theory building is often described as the ultimate goal of qualitative research, an examination of articles in Qualitative Health Research ( QHR) shows that themes are actually the typical format for reporting results. In addition, articles that rely on themes often present low-level theori …
The research on which this article is based is part of a National Science Foundation Grant, on "Methods for Conducting Systematic Text Analysis" (SRB-9811166). We wish to thank Stephen Borgatti for his helpful suggestions and two anonymous reviewers for their invaluable comments on earlier drafts of this paper. Introduction
Themes should be far away from the description of any facet of the context. Themes should be closer to explaining the endogenous constructs of a research. Further, often the contribution of a qualitative case study research (QCSR) emerges from the 'extension of a theory' or 'developing deeper understanding—fresh meaning of a phenomenon'.
Your research theme positively states the qualities you will work toward. Some examples follow. "For students to value friendship, develop their own perspectives and ways of thinking, and enjoy science.". "Across both math and language arts, develop our students' abilities to use evidence and reasoning to support and critique arguments ...
Although much has been written about the features of academic writing, there is a lack of research attention on macro issues related to the development of ideas, particularly in the writing of research articles. A concept that is useful in investigating such issues is the Hallidayan notion of theme. However, the thematic structure of research articles has received only modest attention over ...
research articles in Q ualitative Health Research ( QHR ), which has led me to two conclusions. First, themes are the most common format for reporting results. Second, models that connect. themes ...
The Online Writing Lab at Purdue University houses writing resources and instructional material, and we provide these as a free service of the Writing Lab at Purdue.
Sub-point of sub-point 1. Essentially the same as the alphanumeric outline, but with the text written in full sentences rather than short points. Example: First body paragraph of the research paper. First point of evidence to support the main argument. Sub-point discussing evidence outlined in point A.
How can academic scholars come up with great ideas, such that their research becomes even more important, relevant, and interesting? Based on ideation practices of sophisticated companies, this paper triggers academic researchers to self-reflect on: (1) the source used for ideation, (2) the scope applied to ideation, (3) the sharing of ideas during ideation, and (4) the selection of ideas.
The paper assesses the conceptual and methodological treatments in this field, uses literature evidence to identify research gaps, and suggests potential topics for future research. Our research firstly contributes to the use of STS approaches in the novel field of climate change mitigation infrastructure, asking how scholarship in the field ...
Financial matters, corporate social responsibility (CSR), climate change, and other sustainable solutions all work in tandem. In order to provide a thorough understanding of the integration between various components during crises, it is necessary to provide knowledge of the interaction between financial, societal, and environmental aspects. In order to accomplish this, hundreds of papers were ...
Background: Monitoring of gait patterns by insoles is popular to study behavior and activity in the daily life of people and throughout the rehabilitation process of patients. Live data analyses may improve personalized prevention and treatment regimens, as well as rehabilitation. The M-shaped plantar pressure curve during the stance phase is mainly defined by the loading and unloading slope ...