NTRS - NASA Technical Reports Server
Available downloads, related records.
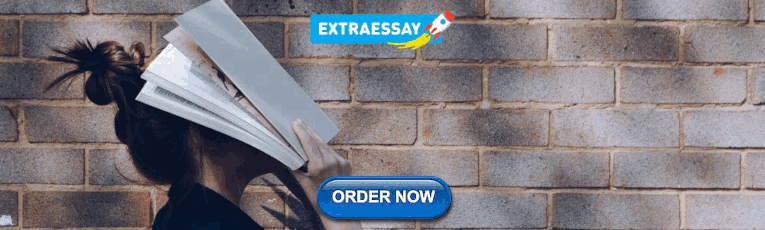
A Comprehensive Review of Speech Emotion Recognition Systems
Ieee account.
- Change Username/Password
- Update Address
Purchase Details
- Payment Options
- Order History
- View Purchased Documents
Profile Information
- Communications Preferences
- Profession and Education
- Technical Interests
- US & Canada: +1 800 678 4333
- Worldwide: +1 732 981 0060
- Contact & Support
- About IEEE Xplore
- Accessibility
- Terms of Use
- Nondiscrimination Policy
- Privacy & Opting Out of Cookies
A not-for-profit organization, IEEE is the world's largest technical professional organization dedicated to advancing technology for the benefit of humanity. © Copyright 2024 IEEE - All rights reserved. Use of this web site signifies your agreement to the terms and conditions.
Feature fusion: research on emotion recognition in English speech
- Published: 30 May 2024
Cite this article
- Yongyan Yang 1
English speech incorporates numerous features associated with the speaker’s emotions, offering valuable cues for emotion recognition. This paper begins by briefly outlining preprocessing approaches for English speech signals. Subsequently, the Mel-frequency cepstral coefficient (MFCC), energy, and short-time zero-crossing rate were chosen as features, and their statistical properties were computed. The resulting 250-dimensional feature fusion was employed as input. A novel approach that combined gated recurrent unit (GRU) and a convolutional neural network (CNN) was designed for emotion recognition. The bidirectional GRU (BiGRU) method was enhanced through jump-joining to create a CNN-Skip-BiGRU model as an emotion recognition method for English speech. Experimental evaluations were conducted using the IEMOCAP dataset. The findings indicated that the fusion features exhibited superior performance in emotion recognition, achieving an unweighted accuracy rate of 70.31% and a weighted accuracy rate of 70.88%. In contrast to models like CNN-long short-term memory (LSTM), the CNN-Skip-BiGRU model demonstrated enhanced discriminative capabilities for different emotions. Moreover, it stood favorably against several existing emotion recognition methods. These results underscore the efficacy of the improved method in English speech emotion identification, suggesting its potential practical applications.
This is a preview of subscription content, log in via an institution to check access.
Access this article
Price includes VAT (Russian Federation)
Instant access to the full article PDF.
Rent this article via DeepDyve
Institutional subscriptions
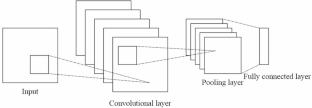
Data availability
The data in this paper are available from the corresponding author.
Ahmed, M. R., Islam, S., Islam, A. M., & Shatabda, S. (2023). An ensemble 1D-CNN-LSTM-GRU model with data augmentation for speech emotion recognition. Expert Systems with Applications , 218 , 119633.
Article Google Scholar
Ayadi, S., & Lachiri, Z. (2022). Visual emotion sensing using convolutional neural network. Przeglad Elektrotechniczny , 98 (3), 89–92.
Google Scholar
Chattopadhyay, S., Dey, A., Singh, P. K., Ahmadian, A., & Sarkar, R. (2023). A feature selection model for speech emotion recognition using clustering-based population generation with hybrid of equilibrium optimizer and atom search optimization algorithm. Multimedia Tools and Applications , 82 (7), 9693–9726.
Chen, Y., Liu, G., Huang, X., Chen, K., Hou, J., & Zhou, J. (2021). Development of a surrogate method of groundwater modeling using gated recurrent unit to improve the efficiency of parameter auto-calibration and global sensitivity analysis. Journal of Hydrology , 598 (3), 1–16.
Guo, L., Wang, L., Dang, J., Chng, E. S., & Nakagawa, S. (2022). Learning affective representations based on magnitude and dynamic relative phase information for speech emotion recognition - ScienceDirect. Speech Communication , 136 , 118–127.
Hansen, L., Zhang, Y. P., Wolf, D., Sechidis, K., Ladegaard, N., & Fusaroli, R. (2021). A generalizable speech emotion recognition model reveals depression and remission. Acta Psychiatrica Scandinavica , 145 (2), 186–199.
Hu, D., Chen, C., Zhang, P., Li, J., Yan, Y., & Zhao, Q. (2021). A two-stage attention based modality fusion framework for multi-modal speech emotion recognition. IEICE Transactions on Information and Systems , E104.D (8), 1391–1394.
Hu, Z., Wang, L., Luo, Y., Xia, Y., & Xiao, H. (2022). Speech emotion recognition model based on attention CNN Bi-GRU fusing visual information. Engineering Letters, 30 (2).
Hyder, H. (2021). The pedagogy of English language teaching using CBSE methodologies for schools. Advances in Social Sciences Research Journal , 8 , 188–193.
Li, Z., Wang, S. H., Fan, R. R., Cao, G., Zhang, Y. D., & Guo, T. (2019). Teeth category classification via seven-layer deep convolutional neural network with max pooling and global average pooling. International Journal of Imaging Systems and Technology , 29 (4), 577–583.
Liu, L. Y., Liu, W. Z., Zhou, J., Deng, H. Y., & Feng, L. (2022). ATDA: Attentional temporal dynamic activation for speech emotion recognition. Knowledge-based Systems , 243 (May 11), 1–11.
Nfissi, A., Bouachir, W., Bouguila, N., & Mishara, B. L. (2022). CNN-n-GRU: End-to-end speech emotion recognition from raw waveform signal using CNNs and gated recurrent unit networks. In 21st IEEE international conference on machine learning and applications (ICMLA) , (pp. 699–702).
Niu, D., Yu, M., Sun, L., Gao, T., & Wang, K. (2022). Short-term multi-energy load forecasting for integrated energy systems based on CNN-BiGRU optimized by attention mechanism. Applied Energy , 313 , 1–17.
Ocquaye, E. N. N., Mao, Q., Xue, Y., & Song, H. (2021). Cross lingual speech emotion recognition via triple attentive asymmetric convolutional neural network. International Journal of Intelligent Systems , 36 (1), 53–71.
Pandey, S. K., Shekhawat, H. S., & Prasanna, S. R. M. (2022). Attention gated tensor neural network architectures for speech emotion recognition. Biomedical Signal Processing and Control , 71 (2), 1–16.
Peng, Z., Zhu, Z., Unoki, M., Dang, J., Akagi, M. (2018). Auditory-inspired end-to-end speech emotion recognition using 3D convolutional recurrent neural networks based on spectral-temporal representation. In 2018 IEEE international conference on, multimedia, & expo. (ICME) (pp. 1–6), San Diego, CA, USA.
Ponmalar, A., & Dhanakoti, V. (2022). Hybrid whale tabu algorithm optimized convolutional neural network architecture for intrusion detection in big data. Concurrency and Computation: Practice and Experience , 34 (19), 1–15.
Qiao, D., Chen, Z. J., Deng, L., & Tu, C. L. (2022). Method for Chinese speech emotion recognition based on improved speech-processing convolutional neural network. Computer Engineering , 48 (2), 281–290.
Requardt, A. F., Ihme, K., Wilbrink, M., & Wendemuth, A. (2020). Towards affect-aware vehicles for increasing safety and comfort: Recognising driver emotions from audio recordings in a realistic driving study. IET Intelligent Transport Systems , 14 (10), 1265–1277.
Tan, M., Wang, C., Yuan, H., Bai, J., & An, L. (2020). FDA-MIMO Beampattern synthesis with Hamming window weighted linear frequency increments. International Journal of Aerospace Engineering , 2020(2) , 1–8.
Tanko, D., Dogan, S., Demir, F. B., Baygin, M., Sahin, S. E., & Tuncer, T. (2022). Shoelace pattern-based speech emotion recognition of the lecturers in distance education: ShoePat23. Applied Acoustics , 190 , 1–9.
Wibawa, I. D. G. Y. A., & Darmawan, I. D. M. B. A. (2021). Implementation of audio recognition using mel frequency cepstrum coefficient and dynamic time warping in wirama praharsini. Journal of Physics: Conference Series, 1722 , 1–8.
Zhao, Z., Zheng, Y., Zhang, Z., Wang, H., Zhao, Y., & Li, C. (2018). Exploring spatio-temporal representations by integrating attention-based bidirectional-LSTM-RNNs and FCNs for speech emotion recognition. In Annual conference of the international speech communication association , (pp. 272–276).
Zhao, Z., Bao, Z., Zhao, Y., Zhang, Z., Cummins, N., Ren, Z., & Schuller, B. (2019). Exploring deep spectrum representations via attention-based recurrent and convolutional neural networks for Speech emotion recognition. IEEE Access: Practical Innovations, Open Solutions , 7 , 97515–97525.
Zhu, M., Cheng, J., & Zhang, Z. (2021). Quality control of microseismic P-phase arrival picks in coal mine based on machine learning. Computers & Geosciences , 156 , 1–12.
Download references
Not applicable.
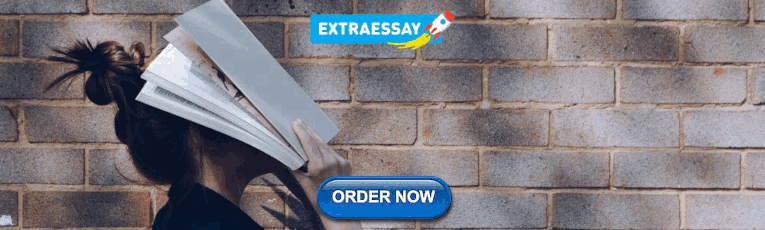
Author information
Authors and affiliations.
Department of General Foreign Languages Education, Haikou University of Economics, Haikou, Hainan, 571123, China
Yongyan Yang
You can also search for this author in PubMed Google Scholar
Contributions
YYY conceived the idea for the study, did the analyses, and wrote the paper.
Corresponding author
Correspondence to Yongyan Yang .
Ethics declarations
Conflict of interest.
The authors declare no conflict of interest.
Additional information
Publisher’s note.
Springer Nature remains neutral with regard to jurisdictional claims in published maps and institutional affiliations.
Rights and permissions
Springer Nature or its licensor (e.g. a society or other partner) holds exclusive rights to this article under a publishing agreement with the author(s) or other rightsholder(s); author self-archiving of the accepted manuscript version of this article is solely governed by the terms of such publishing agreement and applicable law.
Reprints and permissions
About this article
Yang, Y. Feature fusion: research on emotion recognition in English speech. Int J Speech Technol (2024). https://doi.org/10.1007/s10772-024-10107-7
Download citation
Received : 15 January 2024
Accepted : 09 May 2024
Published : 30 May 2024
DOI : https://doi.org/10.1007/s10772-024-10107-7
Share this article
Anyone you share the following link with will be able to read this content:
Sorry, a shareable link is not currently available for this article.
Provided by the Springer Nature SharedIt content-sharing initiative
- Feature fusion
- English speech
- Emotion recognition
- Gated recurrent unit
- Find a journal
- Publish with us
- Track your research
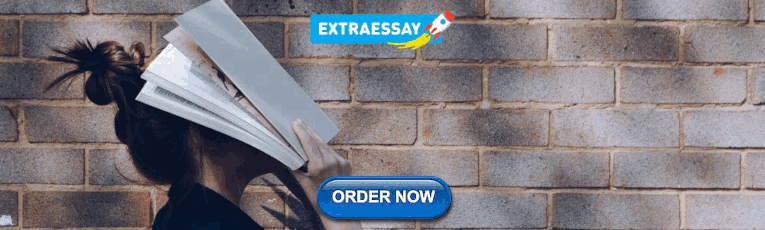
IMAGES
VIDEO
COMMENTS
A huge amount of research has been done in the field of speech signal processing in recent years. In particular, there has been increasing interest in the automatic speech recognition (ASR) technology field. ASR began with simple systems that responded to a limited number of sounds and has evolved into sophisticated systems that respond fluently to natural language. This systematic review of ...
Abstract: Over the past decades, a tremendous amount of research has been done on the use of machine learning for speech processing applications, especially speech recognition. However, in the past few years, research has focused on utilizing deep learning for speech-related applications. This new area of machine learning has yielded far better results when compared to others in a variety of ...
ABSTRACT Over the past decades, a tremendous amount of research has been done on the use of machine. learning for speech processing applications, especially speech recognition. However, in the ...
The last section concludes the paper. 2. Speaker recognition. In speech processing, speaker recognition and speech recognition are the two applications commonly used by researchers to analyze uttered speech [10]. Before delving further into the structure of speaker recognition, it is vital to understand the difference between speaker ...
Speech processing is a field dedicated to the study and application of methods for analyzing and manipulating speech signals. It encompasses a range of tasks, including automatic speech recognition (ASR) [1], [2], speaker recognition (SR) [3], and speech synthesis or text-to-speech [4].
Speech Recognition is a t echnology with the help of which a machine can. acknowledge the spoken word s and phrases, which can further be used to. generate text. Speech Recognition System works ...
This paper discusses how automatic speech recognition systems are and could be designed, in order to best exploit the discriminative information encoded in human speech. This contrasts with many recent machine learning approaches that apply general recognition architectures to signals to identify, with little concern for the nature of the input.
Dragon Naturally Speaking and Google speech recognition were the most popular technologies, and their most frequent application was providing feedback. According to the results, college students were involved in research more than any other group, most studies were carried out for less than one month, and most scholars administered a ...
Automatic Speech Emotion Recognition (ASER) has recently garnered attention across various fields including artificial intelligence, pattern recognition, and human-computer interaction. However, ASER encounters numerous challenges such as a shortage of diverse datasets, appropriate feature selection, and suitable intelligent recognition techniques. To address these challenges, a systematic ...
Recently great strides have been made in the field of automatic speech recognition (ASR) by using various deep learning techniques. In this study, we present a thorough comparison between cutting-edged techniques currently being used in this area, with a special focus on the various deep learning methods. This study explores different feature extraction methods, state-of-the-art classification ...
Speech-to-Text (STT) and Text-to-Speech (TTS) recognition technologies have witnessed significant advancements in recent years, transforming various industries and applications. STT allows for the conversion of spoken language into written text, while TTS enables the generation of natural-sounding speech from written text. In this research paper, we provide a comprehensive review of the latest ...
1.2 Our contribution. In this section, we will discuss how our research work is different from the earlier SR surveys. Most of the recent surveys have targeted specific areas in speech recognition like ASR survey on [], Acoustic-Phonetic Analysis [], Mandarin speech recognition [], Speech emotion recognition [44, 45], speech denoising methods [], ML and DL Applications [], state-of-the-art ...
This paper presents a brief survey on Automatic ... 1.2 Basic Model of Speech Recognition: Research in speech processing and communication for the most part, was motivated by people s desire to build ... 1.4 Applications of Speech Recognition: Various applications of speech recognition domain have been
pre-training has been underappreciated so far for speech recognition. We achieve these results without the need for the self-supervision or self-training techniques that have been a mainstay of recent large-scale speech recognition work. To serve as a foundation for further research on robust speech recognition, we release inference code and ...
Despite being introduced in 1943, machine learning (ML) only started to thrive in the 1990s. The applications of ML in many real-world scenarios, such as medical diagnosis, traffic alerts, speech ...
With the speech recognition research intensifying gradually in recent years, it is particularly important to grasp the research direction of this filed. This paper summarizes the theoretical algorithms in the development of speech recognition. Firstly, we introduce the specific process of speech recognition, including biometrics acquisition, preprocessing, feature extraction, biometrics ...
A Temporal-Spatial Learnable Graph Convolutional Neural Network (TLGCNN) for speech emotion recognition that can simultaneously obtain temporal speech emotional information through Bi-LSTM and spatial speech emotional information through Learnable Graph Convolutional Neural (LGCN) network. The Graph Convolutional Neural Networks (GCN) method has shown excellent performance in the field of deep ...
Multilingual speech recognition has numerous applications across various fields. Here are a few of the most important applications: Language Translation: Multilingual speech recognition may create speech-to-text translation systems. These systems may take oral input in a single or multilingual language, turn it into text, and then translate it ...
Speech emotion recognition has lots of applications such as human-computer interaction and health management. The current methods are challenged with the problems of fuzzy decision boundary and imbalance between difficult and easy samples in the training data.
Speech emotion recognition (SER) as a Machine Learning (ML) problem continues to garner a significant amount of research interest, especially in the affective computing domain. This is due to its increasing potential, algorithmic advancements, and applications in real-world scenarios. Human speech contains para-linguistic information that can ...
The stuttered speech recognition consists of two stages namely classification using ANN and testing in ASR. The major phases of classification system are Re-sampling, Segmentation, Pre Emphasis, Epoch Extraction and Classification. The current work is carried out in UCLASS Stuttering dataset using MATLAB with 4% to 6% increase in accuracy by ANN.
2. Automatic Speech Recognition. Automatic speech recognition is one of the most automatic speech processing areas, allowing the machine to understand the. user's speech and convert it into a ...
Considering previous research indicating the presence of biases based on gender and accent in AI-based tools such as virtual assistants or automatic speech recognition (ASR) systems, this paper examines these potential biases in both Alexa and Whisper for the major Spanish accent groups. The Mozilla Common Voice dataset is employed for testing, and after evaluating tens of thousands of audio ...
In recent years, the application of automatic speech recognition has gained popularity across diverse industries, including aviation. While traditional applications focus on transcribing air traffic control communication, this paper explores a unique application of automatic speech recognition by converting the audio from planning ...
Hence, there are several applications that enhance speech recognition. This paper aims to study some models of the speech recognition system, its classification of speech, its significance, and its application. Published in: 2020 8th International Symposium on Digital Forensics and Security (ISDFS)
Speech recognition technology focuses on converting spoken language into text. It involves processes such as acoustic modeling, language modeling, and decoding, aiming to achieve high accuracy in transcriptions. Significant advancements have been made in this field, driven by machine learning algorithms and large datasets. These advancements enable more accurate and efficient speech ...
Recently, emotion recognition has gained attention because of its diverse application areas, like affective computing, healthcare, human-robot interactions, and market research. This paper provides a comprehensive and systematic review of emotion recognition techniques of the current decade.
This paper introduces a design method of intelligent English translation system based on TTS technology. On this basis, according to the actual needs of English translation, TTS technology (text analysis, prosody control and speech synthesis) is used to design the system software, which mainly includes continuous speech automatic segmentation and annotation, prosody control, speech synthesis ...
During the last decade, Speech Emotion Recognition (SER) has emerged as an integral component within Human-computer Interaction (HCI) and other high-end speech processing systems. Generally, an SER system targets the speaker's existence of varied emotions by extracting and classifying the prominent features from a preprocessed speech signal. However, the way humans and machines recognize and ...
English speech incorporates numerous features associated with the speaker's emotions, offering valuable cues for emotion recognition. This paper begins by briefly outlining preprocessing approaches for English speech signals. Subsequently, the Mel-frequency cepstral coefficient (MFCC), energy, and short-time zero-crossing rate were chosen as features, and their statistical properties were ...