- Study Guides
- Homework Questions
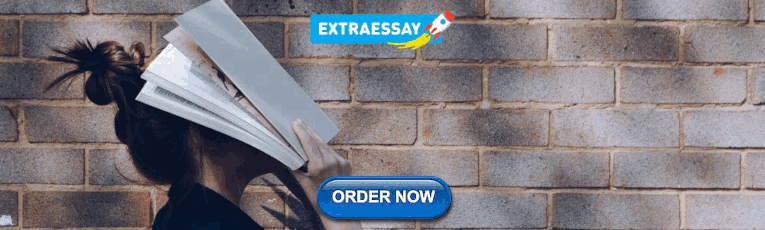
CASE STUDY 9 THE TENNIS MATCH Noel is a computer programmer at...
CASE STUDY 9 THE TENNIS MATCH
Noel is a computer programmer at GIAV Corporation. He was delighted to know that part of the company's' athletic program is a mixed double tennis tournament to be held at the corporate tennis court. Grace, who work on the same department used to play with noel in many occasions and the two would like to compete in the tournament. They feel that there is a great chance to win the annual tennis competition. There is a growing interest among employees who are tennis players in the corporation as it develops more productivity at work. The management themselves are all tennis enthusiasts. The tournament is scheduled on Friday afternoon and management declared this as a "TENNIS HOLIDAY". It is a day of fun and workers can cheer for their respective teams. Management supported the program with free snacks and fun fairs to make the occasion memorable. It was a four-consecutive-Fridays tournament.
Noel and grace advanced to the final round. They were leading in the third set which was the deciding point. On a smash, Noel twisted his ankle badly. Despite the injury Noel and Grace continued the game until they won the set and were declared tennis-champion. Noels' injury became worse and he had to be confined in the hospital for two days as the X ray results showed hairline fractures.
Noel missed two days of his work activities. He has no more sick leave or vacation leave because company policy and ECC policy do not cover non-related work accidents. Workers' compensation provides payment if the workers is "functioning within the scope of Employment".
To Do: Identify and explain the problems and solutions to this case study.
CASE STUDY 10 PERMAJA TRADING AND BUILDERS' CORPORATION
Donato Martinez is a long-time employee of Permaja Trading and Builders Corporation (PTBC) and was promoted as foreman in the construction department. Donato or Ato rose from the ranks as foreman, a position usually given to a college or technical education graduate. Ato is a high school graduate but his 15 years with the company has proven his worth for his good performance and his loyalty to PTBC.
Ato's personal life is not unknown to the management; however, since it does not interfere much with his work in the company, it is not given much attention. Ato has been married for ten years but never had a child. His wife does not pay attention to him and this led him to drinking sprees after office hours. Family matters worsened as Ato drinking too much, he was absent from his work especially after weekends.
The management called his attention to his absences and he was reprimanded and was given written warnings. For a time, Ato would pay attention to the advice of the management and his performance improved for the time being. His projects were completed on time and costs estimates were met on a target. Atos' performance was still considered by the management as very satisfactory. It is the policy of the company not to let matter of personal concern interfere with work efficiency or allow nature of his personal life affect the PTBC image in the community.
In a new project in Cebu, Ato was made to handle the construction of the big mall. PTBC has made a name in mall construction as a reliable company due to completion of projects on time. Ato being a specialist in this type of construction was lead foreman under the supervision of the construction superintendent, Walter Samson, a civil engineer seasoned in such projects.
Ato got involved with another women in Cebu and still continued with his drinking sessions with some of the local workers. Walter was not happy about the situation especially when Ato was absent from work. The worst thing happened when Ato's wife discovered his love affair when she went to Cebu without the knowledge of Ato. The quarrel ended with the wife complaining to the police and Ato was picked up for investigation. The management was alarmed but no replacement was available to take the place of Ato at the Cebu site.
To Do: Identify and explain the problems and solutions to this case study. note: DO NOT USE Ai Chat, need asap
Answer & Explanation

Unlock access to this and over 10,000 step-by-step explanations
Have an account? Log In
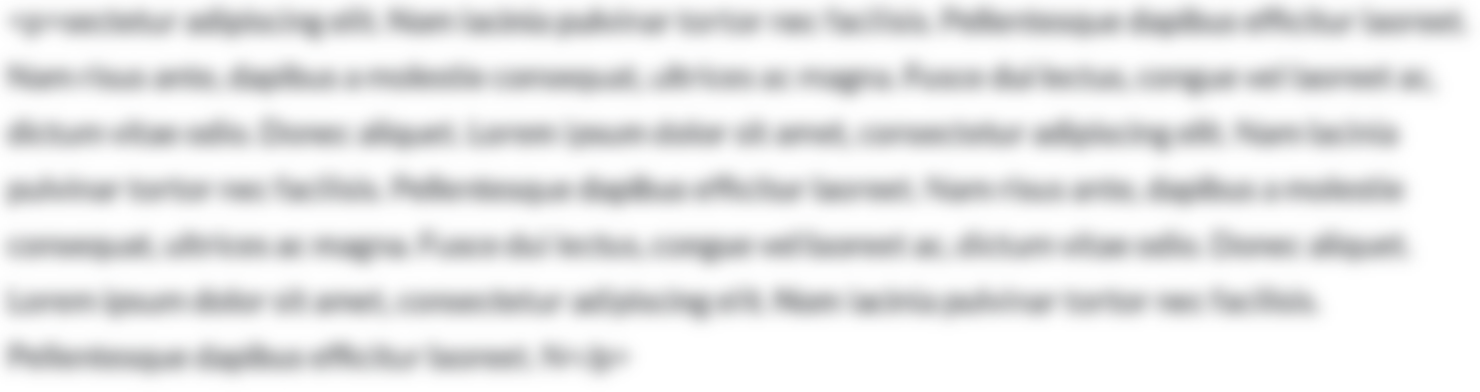
Step-by-step explanation
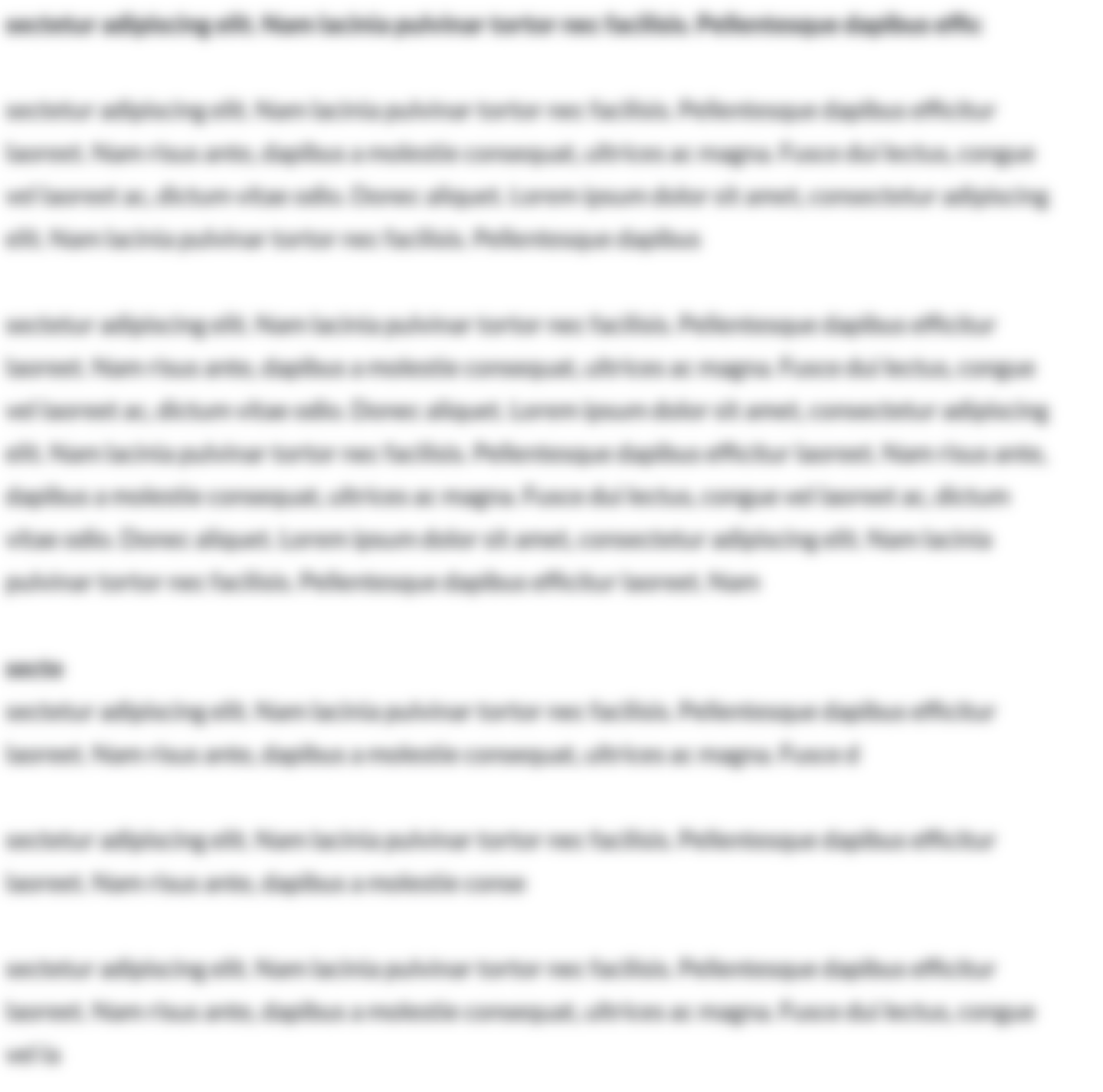
Get unstuck with a CliffsNotes subscription
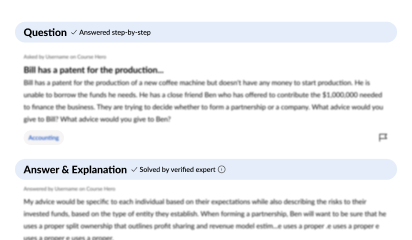
Related Q&A
- Q can you provide a response to the post below and include a question ask I'm really intrigued by the amount of info... Answered over 90d ago
- Q Your patient is Eva, an 86 year old female who weights 57kg. Eva is scheduled for theatre tomorrow for repair of a fract... Answered over 90d ago
- Q Graded Assignment - Unit 6 Speaking Assignment novel is on George Orwell 1984 Content Standard SL. 11-12.4 Total s... Answered over 90d ago
- Q Experiment 2 1) Which are generally larger, Prokaryotic or Eukaryotic cells? About how much larger? 2) List 5 generally ... Answered over 90d ago
- Q I am having trouble finding the cost of the last 2 questions PM.66 Real estate developer Russell Call was working with ... Answered over 90d ago
- Q . 3) When the Aggregate Supply curve contracts, an economy experiences a combination of low output and high inflation r... Answered over 90d ago
- Q Check your answer: Once you've solved the problem, it's important to check your answer. Double-check your calculations a... Answered over 90d ago
- Q . genow.com/ilrn/takeAssignment/takeCovalentActivity.do?locator=assignment-take [Ref unds [ Review Topics] Use the Refe... Answered over 90d ago
- Q PLEASE READ PICTURES FIRST AND ANSWER THE QUESTIONS IN YOUR OWN POINT OF VIEW PLEASE. Having been exposed to some gene... Answered over 90d ago
- Q . Problem 1 Effect of phase shift in demodulation Assume we have a modulated signal that we transmit . During transmiss... Answered over 90d ago
- Q Identify 2 state grade-level standards for any given content area. In the table provided below, write behavioral objecti... Answered over 90d ago
- Q issus/ problem What is the reason Nurse Home and ALF do not report falls? It is because of covering up negligence, fear ... Answered over 90d ago
- Q 1.75 moles oxygen gas and 3.09 moles of solid carbon are placed into 3.17 L container at 25.9 C and the container is sea... Answered over 90d ago
- Q Question 3 (16 marks) Consider the following information: State of Economy Probability of State Rate of Return if State ... Answered over 90d ago
- Q Paul addresses the qualifications to be a church leader in 1Timothy 3:1-13. 1. What are the qualifications a church lead... Answered over 90d ago
- Q Subjective: CC (chief complaint): Has been having headaches and stomachaches, and per the request of her pediatric provi... Answered over 90d ago
- Skip to main content
- Skip to primary sidebar
Tennis Analytics
Analysis & Reporting for College Teams, Coaches, Players
Jan 24 2020
2,086 Match Case Study: How Men’s College Players Win Matches
We’ve studied 2,086 college men’s college matches ( women’s case study here ) from August 1, 2017, through 2019. Our objective was to answer some of these questions.
- What is the difference between match winners and losers?
- Which statistical categories are most important?
- What should a college coach or player focus on, to improve faster?
Below are the results.

The Stats We Measured
Here’s how we performed this study.
We took data from 2,086 matches and divided the data into two categories.
- Match Winner
- Match Loser
For each match, we collected 230 stats per player. Here are 16 examples.
- Number of 1st Serves In
- Total # of 1st Serves Hit
- 1st Serve %
- 1st Serves Won
- 1st Serves Won %
- # Double Faults – Net
- # Double Faults – Wide
- # Double Faults – Long
- Return Errors – Combined Courts
- Return Errors – Deuce – 1st Serve
- Points Won on Net Appearance
- S+1 FH Point Won
- S+1 FH Point Won %
- 1-4 Contribution %
- Return Errors – Deuce – 1st Serve – Forehand
Want more stats & data? Sign up for our email newsletter for announcements on a brand new data analytics product coming soon. You will be able to access big data on 10,000’s of matches. It will have match data from juniors, college tennis, all the way to top ATP & WTA pros.
Sign Up for Our Newsletter Get the latest Tennis Analytics blog posts, stat of the week, special discounts, & more straight to your inbox!
As you can see, we got very detailed.
We were able to find out, for example, “how many more forehand return errors does a match loser average from the deuce court when the opponent makes the first serve ?”
Answer: 0.34 more errors
The best way to figure out what to do with all this data is to start with the high-level metrics, then drill down.
The Average Number of Points Per Match in College Tennis
On average, these matches had a total of 130.8 points.
- The match winner averages 71 points won.
- The match loser averages 59.8
So the difference between winning and losing, on average, is 11.2 points. That’s less than 1 point per game, not a huge difference.
Where does this 11 point difference come from?
The Difference Between the Winners & Losers
Next, we studied the high-level metrics to figure out where the match loser is falling short.
Here were the results.
1st Serves In | 41.56 | 41.16 | 0.41 |
1st Serves Won | 29.07 | 25.18 | 3.89 |
2nd Serves Won | 12.43 | 10.70 | 1.74 |
# Double Faults | 2.77 | 3.36 | -0.58 |
# of Aces | 3.14 | 2.26 | 0.88 |
Return Errors – Combined Courts | 11.28 | 12.75 | -1.48 |
Return Winners – Combined Courts | 0.87 | 0.63 | 0.24 |
Return Made – Combined Courts | 49.74 | 46.69 | 3.05 |
Points Won at Net Appearance | 14.65 | 12.43 | 2.22 |
When we look at this data, the largest differentials are on:
- 1st serve points won
- Returns made
These three stats combine for 6.94 points. If the match loser can close the gap on these stats, the tables are turned.
When you give the match loser 6.94 extra points, you’re taking away those points from the winner. So the real difference is 13.88 points , more than our total difference of 11.
Below we’ll analyze each of these stats further, and how a coach or player can use this information to practice.
First Serve Points Won
It’s not surprising that 1st serve points won is one of our metrics.
From these 2,086 matches, the first serve percentage for both players was 63.3%, meaning most points started with a first serve in the court.
What we need to do is figure out why the match winner is winning nearly 4 more points on their first serve.
1st Serves Won | 29.07 | 25.18 | 3.89 |
What can the match loser do to close this gap? Two things.
- Bring the 29.07 number down = improve returning 1st serves.
- Increase the 25.18 number = improve serving 1st serves.
Your first touch is key to determining the outcome of a match.
We know from our previous lessons that improving the first 4 shots and improving serve strategy is important.
This time we get to look at a larger data set for the serve +1 and return +1 data.
Match Winner Avg | 15.02 | 6.31 | |
Match Loser Avg | 12.92 | 5.74 | |
Differential | |||
Match Winner Avg | 9.54 | 8.14 | |
Match Loser Avg | 7.22 | 6.37 | |
Differential |
S+1 = the server’s 2nd shot, or the 3rd shot of the point. R+1 = the returner’s 2nd shot, or the 4th shot of the point.
Note: This data accounts for points with 3+ & 4+ shots. So double faults, aces, and return errors are not counted here. Our next stat, returns made, will help account for the return errors. We saw above that double faults have a -0.58 differential.
It’s clear from this table that most points that are 3+ shots are won when the player hits a forehand for their second touch. This is even more true for the serve +1 than the return +1.
A few takeaways from this data.
- Forehands have a larger differential than backhands for both S+1 and R+1.
- The match winner wins the “R+1 – point won” stat by a larger margin than they win the “S+1 – point won” stat. In other words, they create a larger margin on R+1 than they do on S+1.
This shows that college players should be practicing serve +1 forehand patterns, and return +1 forehand and backhand patterns in practice.
Practice more aggressive shots on serves and forehands when you can. Practice defensive shots with the backhand and returning 1st serves.
Again, keep in mind that these stats are interconnected. Improving the next stat on our list will help the previous stat as well.
Total Returns Made
Next, we look at total returns made.
Return Made – Combined Courts | 49.74 | 46.69 | 3.05 |
Match winners are making over 3 returns more per match than losers. Let’s see which areas of the court, and on which serves, making returns made matters most.
Return Made – Deuce | 25.67 | 24.29 | 1.38 |
Return Made – Ad | 24.07 | 22.40 | 1.67 |
Return Made – Combined Courts – 1st Serve | 31.58 | 29.53 | 2.05 |
Return Made – Combined Courts – 2nd Serve | 18.16 | 17.15 | 1.01 |
We can see that returns made on first serves have over a 2x bigger point differential than returns made on 2nd serves. This makes sense with the 63% first-serve percentage.
More surprising, the ad court accounts for a larger differential than the deuce court. This is surprising because any game won at 40-15 would have 3 points served to the deuce court and only 2 to the ad. There is never a game won with more points to the ad court.
Note: There could be an exception to this if the matches are no-ad scoring. Many college matches are no-ad scoring, so this could be the difference.
As we drilled down further we found that:
- On first serves, there is a slightly larger differential in backhand returns made.
- On second serves, forehand returns make a bigger difference.
College players should practice improving their returns , we already know that.
But we can now prioritize returning 1st serves, especially to the backhand, and especially on the ad court. This is where match winners are separating themselves.
On second serves, college players should prioritize practicing forehand returns.
What We Could Add to this Case Study
Any statistical analysis of tennis is going to have some gaps. It’s important to recognize those gaps to have a full understanding of what the data means, so we can create actionable steps to apply to our practice time.
This data might change if we focused on matches with left-handed players. Because matches that include lefties are a minority of our data set, we simply don’t know if the data would change for these players.
Perhaps, at some point, we can target data for lefties.
That said, there are some relevant takeaways that college coaches and players should pay attention to.
If you see anything else we may have missed, comment below!
Key Takeaways: How to Win in College Tennis
Tennis is made up of matches, sets, games, and points. Win more points, and you’ll usually win the match.
With over 230 stats, it can be overwhelming to decide what’s important and what isn’t. Focusing on these point differentials is a way to find the most important metrics. By finding these stats, your college team can practice the right things that will lead to winning more points, games, sets, and matches.
We’re not saying to only focus on these things in practice, but they should be a priority based on the data we’ve collected.
- First serves are more important than second serves.
- Practice your S+1 and R+1 forehand patterns most.
- Making returns on first serves is more important than second serves.
- Practice your R+1 backhand pattern, playing defensive or neutralizing shots.
- Practice returning first serves with the backhand in the ad court.
- Practice returning second serves with the forehand.
Next month, we’ll analyze the women’s matches.
About Warren Pretorius & Will Boucek
Each post is written by Will Boucek, in collaboration with Warren Pretorius.
Warren is the founder & CEO of Tennis Analytics. He is a USPTA Master Professional, speaker, and strategy coach for ATP & WTA pros.
Will is a writer and content strategist for Tennis Analytics. Will is also the founder of The Tennis Tribe and Tennis Tribe Marketing . He played college tennis and has over a decade of coaching experience.
Reader Interactions
December 23, 2021 at 10:13 am
Point differentials should be carefully analyzed, as they might show correlation rather than causation. While many aspects of the game are correlated (happen together with being a better player) with being a better player, not all of these actually cause the player to win more. A way of improving the analysis would be comparing matches of the same player, some where certain stats were high and some where they were low, and comparing the change in performance.
Warren Pretorius & Will Boucek says
December 23, 2021 at 12:41 pm
Great points Jose! We can definitely do more with this data to drill down on what’s affecting the outcome most.
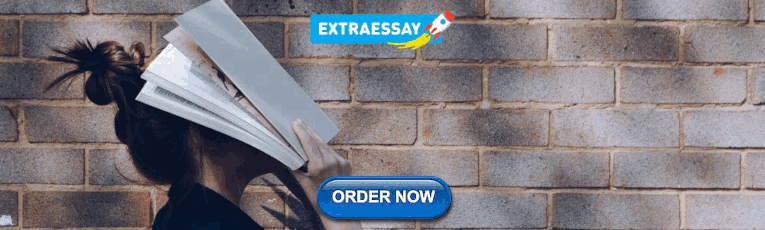
Leave a Reply Cancel reply
Your email address will not be published. Required fields are marked *

Provide details on what you need help with along with a budget and time limit. Questions are posted anonymously and can be made 100% private.

Studypool matches you to the best tutor to help you with your question. Our tutors are highly qualified and vetted.

Your matched tutor provides personalized help according to your question details. Payment is made only after you have completed your 1-on-1 session and are satisfied with your session.

- Homework Q&A
- Become a Tutor

All Subjects
Mathematics
Programming
Health & Medical
Engineering
Computer Science
Foreign Languages

Access over 35 million homework & study documents
Sign up to view the full document!

24/7 Homework Help
Stuck on a homework question? Our verified tutors can answer all questions, from basic math to advanced rocket science !

Similar Documents

working on a homework question?

Studypool is powered by Microtutoring TM
Copyright © 2024. Studypool Inc.
Studypool is not sponsored or endorsed by any college or university.
Ongoing Conversations

Access over 35 million homework documents through the notebank

Get on-demand Q&A homework help from verified tutors

Read 1000s of rich book guides covering popular titles

Sign up with Google

Sign up with Facebook
Already have an account? Login
Login with Google
Login with Facebook
Don't have an account? Sign Up
Tennis Case Study

Students also viewed

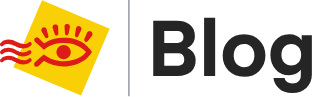
- X (Twitter)
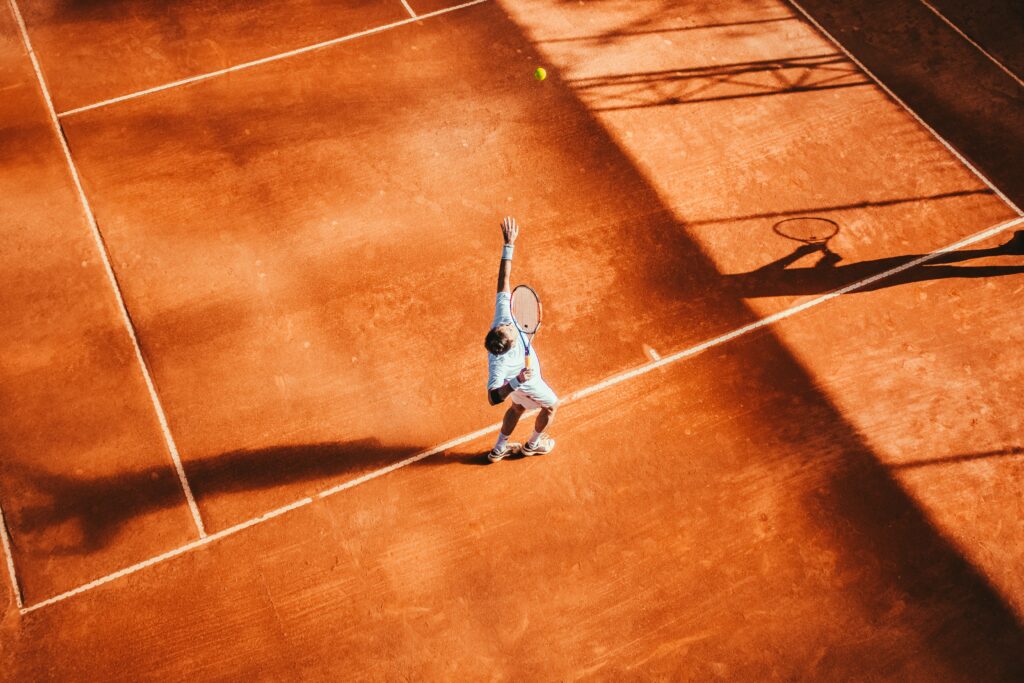
Best Tennis Analytics’s Case Studies
- 5 Video Tips for Coaches
- The Video Revolution: How & Why the Best Tennis Coaches are Using Video for Player Development
- 2,326 Match Case Study: How Women’s College Players Win Matches
- 2,086 Match Case Study: How Men’s College Players Win Matches
- Improve Your First 4 Shots in Singles
- How to Improve Your Return Strategy in Singles: Our Proven Method
- How to Improve Your Serve Strategy in Singles
- The Future of Tennis Coaching: A Data-Driven Approach
- Case Study: How Texas Won the NCAA D1 Championships
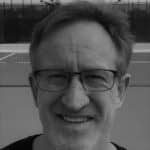
Comments are closed.
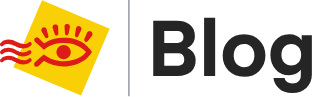
- Partnerships
- Press Release
- Video Replay
- Other Features
- Product Release
- Baseball/Softball
- Track and Field
- Other Sports
- Start Trial
Type above and press Enter to search. Press Esc to cancel.
Click through the PLOS taxonomy to find articles in your field.
For more information about PLOS Subject Areas, click here .
Loading metrics
Open Access
Peer-reviewed
Research Article
A study of forecasting tennis matches via the Glicko model
Roles Conceptualization, Formal analysis, Methodology, Project administration, Resources, Supervision, Writing – original draft, Writing – review & editing
Affiliation Department of Statistics, National Chengchi University, Taipei, Taiwan
Roles Investigation, Validation, Visualization, Writing – original draft, Writing – review & editing
* E-mail: [email protected]

Roles Resources
Affiliation Physical Education Office, National Chengchi University, Taipei, Taiwan
Roles Data curation, Formal analysis, Visualization
- Jack C. Yue,
- Elizabeth P. Chou,
- Ming-Hui Hsieh,
- Li-Chen Hsiao
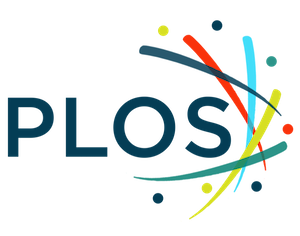
- Published: April 8, 2022
- https://doi.org/10.1371/journal.pone.0266838
- Reader Comments
Tennis is a popular sport, and professional tennis matches are probably the most watched games globally. Many studies consider statistical or machine learning models to predict the results of professional tennis matches. In this study, we propose a statistical approach for predicting the match outcomes of Grand Slam tournaments, in addition to applying exploratory data analysis (EDA) to explore variables related to match results. The proposed approach introduces new variables via the Glicko rating model, a Bayesian method commonly used in professional chess. We use EDA tools to determine important variables and apply classification models (e.g., logistic regression, support vector machine, neural network and light gradient boosting machine) to evaluate the classification results through cross-validation. The empirical study is based on men’s and women’s single matches of Grand Slam tournaments (2000–2019). Our analysis results show that professional tennis ranking is the most important variable and that the accuracy of the proposed Glicko model is slightly higher than that of other models.
Citation: Yue JC, Chou EP, Hsieh M-H, Hsiao L-C (2022) A study of forecasting tennis matches via the Glicko model. PLoS ONE 17(4): e0266838. https://doi.org/10.1371/journal.pone.0266838
Editor: Maurizio Naldi, LUMSA: Libera Universita Maria Santissima Assunta, ITALY
Received: September 1, 2021; Accepted: March 28, 2022; Published: April 8, 2022
Copyright: © 2022 Yue et al. This is an open access article distributed under the terms of the Creative Commons Attribution License , which permits unrestricted use, distribution, and reproduction in any medium, provided the original author and source are credited.
Data Availability: All data are available from GitHub, which are organized by Jeff Sackmann at https://github.com/JeffSackmann .
Funding: The author(s) received no specific funding for this work.
Competing interests: The authors have declared that no competing interests exist.
Introduction
Data analytics has become very popular due to the rapid development of big data. Organizations and individuals create more opportunities by making use of the available information. For example, industries utilize big data to attain more customers and higher profits, while governments exploit big data to obtain more efficient resource allocations and better operations. Ever since IBM proposed that there are four characteristics of big data, namely, the 4 Vs (volume, velocity, variety, and veracity), many researchers have suggested expanding the characteristics of big data. Value, variability, and viability are some examples of new Vs. Nonetheless, the key to applying big data is to uncover the patterns hidden in the data, regardless of how many Vs are involved.
Ideally, we would apply data-driven techniques to discover the value of data and let the data tell the story. However, in practice, it is difficult to rely solely on automated data analytics, i.e., performing data analysis with little human intervention, since the process of selecting important variables depends on the study objective and domain knowledge. For example, suppose we are interested in professional tennis matches. If we want to predict the winners of matches, the related variables may include the players’ tournament history and personal information [ 1 , 2 ]. On the other hand, if our focus is on the upset probability of matches, then we may want to consider other variables, such as the match records against common opponents and the format of matches [ 3 , 4 ].
In reality, it is not possible to record all the available information, and it is also unlikely that it is all available digitally. Even though we can collect as many materials as possible, another question arises: should we include all variables in the study? In other words, the consideration is whether more data are better or if we can distinguish signals from noise. Answering this question is not easy, and it somewhat influences how we deal with the problem. In the case of predicting the outcomes of tennis matches, most studies plug all available variables into models (especially machine learning models) without exploring the characteristics of input variables for example, [ 5 – 7 ], although this in some way conflicts with how we conduct statistical analysis.
Selecting appropriate models is also a critical issue in predicting match results, in addition to recognizing important variables. The models for predicting tennis matches can be separated into three categories: regression-based, point-based, and paired comparison [ 8 ]. We can use macro- and microlevel forecasts [ 9 ] as an analogue to describe the difference between regression- and point-based models. It is not necessarily that microlevel models can provide more accurate predictions, which would depend on the study goal and data attributes. In tennis match prediction, regression-based models are the most popular, while point-based models tend to have less accurate predictions [ 10 ].
In order to predict the tennis match results, researchers have considered various predictors into their classification algorithms. Some of the relevant variables are player’s physical and mental characteristics, such as age, height, handedness, and career wins [ 11 , 12 ]. The results of the games are also considered as predictors, like winning percentages on the first, second, and return serve, total point win, and different type of court [ 1 ]. In addition, tournament factors and some indicators from the matches are also used. Furthermore, the most common predictor for the models is player’s rank [ 8 ].
In this study, we aim to evaluate the prediction accuracy of regression-based models, given the same variables, and explore whether introducing new variables can improve model prediction. We generate new variables via a Bayesian approach, namely, the Glicko model, which was originally used in professional chess. In addition, we will apply tools of exploratory data analysis (EDA) to identify important variables. These variables will be plugged into statistical models and machine learning models to check which models produce more accurate results. The model comparison is based on the single matches of Grand Slam tournaments (2000–2019), and error calculation is via cross-validation. In the rest of this paper, we first introduce the data and models used in the next section, followed by the key findings of EDA in Section 3. We show the results of the model comparison in Section 4 and provide our suggestions in Section 5.
Data and methodology
We study the single matches of Grand Slam tournaments, namely, the Australian Open, the French Open, Wimbledon, and the US Open, which are the world’s four most important annual tennis events. For each Grand Slam tournament, there are 7 rounds of single-elimination games (1st round, 2nd round, 3rd round, 4th round, quarter-final, semi-final, and final), with a total of 127 matches (and 128 players). Fig 1 shows the bracket of single matches in Wimbledon. Grand Slam tournaments seed the players according to their rankings and currently adopt the 32-seed format. We consider 20 years of Grand Slam single matches (2000–2019) for men and women in this study and use the data collected (49 variables) from GitHub, which are organized by Jeff Sackmann at https://github.com/JeffSackmann .
- PPT PowerPoint slide
- PNG larger image
- TIFF original image
https://www.interbasket.net/wp-content/uploads/Wimbledon-bracket-1-of-2-print-768x590.jpg .
https://doi.org/10.1371/journal.pone.0266838.g001
Judging from how Grand Slam tournaments seed the bracket, it seems that the ranking is believed to be a reliable index for measuring players’ strength. We will adapt this notion and introduce a rating index for predicting the results of tennis matches. The current ranking method used by the Association of Tennis Professionals (ATP) and the Women’s Tennis Association (WTA) is to determine the seeding of players and qualification for entry in a tournament. Players’ ranking points are decided by the stage of tournament they reached and the reputation of the tournament they participated in for the last 52 weeks. In other words, the ranking points will not be considered if players did not play for the last 52 weeks. The ranking points of tennis tournaments can be found on the official websites of the ATP and WTA.
Elo ratings [ 13 ] is a famous rating system for professional tennis and was initially used in rating chess players. This chess rating system has been used successfully for paired comparison in sports; it measures players’ strength based on their past performance in the sequence of matches, and ratings are updated after each competitive result. Many studies proved the efficiency and accuracy of Elo ratings in predicting the winner of tennis matches [ 8 ], and it is further extended for in-play tennis betting [ 14 ]. [ 15 ] used Elo-based ratings to predict ratings and proved that it works better than the official rating system. Additionally, the Elo rating system uses the normality assumption.
Another rating system is the Glicko model [ 16 ], developed by Mark Glickman to improve the Elo rating system. Three significant adjustments are involved in the Glicko formula, including an additional parameter presented as a standard deviation—rating deviation ( RD ), a time factor, and opponents’ ratings and deviations. RD represents variability/uncertainty in the rating. The deviation will increase at the beginning of the updating period and decrease at the end. Uncertainty should decrease and ratings become more stable as more games are played or the ratings converge to the player’s actual rating. The time factor in the Glicko system allows an increase in RD when a player has not played any rated game for a certain period, representing a higher uncertainty of the rating accuracy than a player playing regularly. The third factor takes opponents’ ratings and RD s into consideration.
The Glicko rating system is an approximate estimation of a player’s true strength via a Bayesian approach. It can accumulate previous information and continuously update the parameters with new match results. The Bayesian estimation is generally straightforward in the case of a conjugate prior, i.e., prior and posterior distributions belonging to the same distribution. However, this is not the case for the Glicko model since the match results are binary. Because of the rapid development of computer technology, Bayesian estimation has become more convenient, and simulation-based MCMC (Markov chain Monte Carlo) methods can be used to obtain Bayesian estimates. In this study, the Glicko ratings were calculated using the “PlayerRatings” package [ 18 ] in R, with an initial mean strength ( r ) of 1500, a deviation ( RD ) of 350, and a constant ( c ) of 10, after various experiments.
Exploratory data analysis
We will apply EDA tools to explore the tennis match data in this section. The goal of EDA is to detect important attributes of tennis matches and to identify useful variables for constructing predictive models. The chosen variables will be plugged into classification models, such as statistical and machine learning models, in the next section. The variables considered include players’ demographic information (e.g., residence, age, the year their career started, and the hand used) and the ranking data (rank and points) from 2000 to 2019. We aim to discover the relationships between player characteristics and match outcomes, which can provide explanatory power in relation to model effectiveness. Interestingly, we found that the Pareto principle (“80–20” rule) exists for the case where players’ advancement to later rounds is closely associated with their rankings. Pareto principle means that 80% of effects (winning results) come from 20% of causes (higher-ranked players). This can be adequately described by the power law.
We should first depict the relationship between the ranking and Grand Slam match result for male singles, since the ranking is believed to be a crucial factor and lower-ranked players defeating higher-ranked players are often called “upsets” (or shocks). Fig 2 shows the line chart of the ranking difference between higher- and lower-ranked players and the winning probability of higher-ranked players. Additionally, we apply the locally weighted regression (LOESS) method to smooth the winning probability. It appears that the winning probability increases with the ranking difference and levels off (to approximately 0.8) when the ranking difference reaches 80. Note that the results in Fig 2 imply that the predicted accuracy is very different for lower- and top-ranked players, similar to that in [ 8 ]. Additionally, the winning probability has higher fluctuations for larger ranking differences due to smaller exposures (or data points), which are shown in Fig 3 .
https://doi.org/10.1371/journal.pone.0266838.g002
https://doi.org/10.1371/journal.pone.0266838.g003
The increasing trend of winning probability in Fig reffig:fig2 implies two possible further developments. First, the ranking is a fine indicator and can serve as a threshold for evaluating the prediction models of tennis matches, although the prediction accuracy is at 80% if relying solely on the ranking. We may want to include more variables to increase the prediction accuracy. The other consideration is that the probability of advancing to further rounds should also be related to the ranking. In other words, the players’ ranking is a critical factor for deciding whether they can reach later rounds. For example, we would expect that the probability of advancing to the semi-final is higher for the top 10 players than for the non-top 10 players. We will use the cumulative probability to demonstrate this phenomenon.
https://doi.org/10.1371/journal.pone.0266838.g004
https://doi.org/10.1371/journal.pone.0266838.t001
The power law has been applied in many areas, such as physics, biology, and social science. For example, the Gutenberg-Richter law is a well-known power law, which is log 10 N = α − βM , where N is the number of earthquakes larger than magnitude M and α and β are parameters [ 19 ]. This power law function fits well in our case, with an R 2 larger than 0.9 if applying least square linear regression to derive the estimates of α and β . The power law indicates that the top players dominate the winners (finalists and semi-finalists) of Gram Slam tournaments, and the ranking would be an important variable if our goal were to predict the champion of a tournament. Note that we also tried other P 0 values, but the fitting results were not as good as that of P 0 = 0.8. This coincides with the concept behind the “80–20 Rule” (or Pareto principle).
We also explore other players’ variables (e.g., age, height, and professional years) and tournament information (e.g., hard, clay, and grass courts). Some of the variables have a strong connection with the winning probability, but the relationships between these variables and winning probability are not as strong. For example, in Fig 5 , the age structures of players advancing to the final, semi-final, and quarter-final are very similar, with the peak at an age of 25 years for all 3 cases. Additionally, the winning probability of players is related to the type of courts. In any case, we will consider all available variables for statistical and machine learning models to predict the winning probability of Grand Slam matches in the next section.
https://doi.org/10.1371/journal.pone.0266838.g005
In Fig 6 , the bigger the Glicko rating differences between two players, the higher the winning probability of higher ranking players. The higher the winning probability of higher ranking players approaches 100% when the rating difference approaches 800. It confirms the proposed variable “Glicko rating” is essential.
https://doi.org/10.1371/journal.pone.0266838.g006
After multiple experiments with cross-validation, the subset of the variables that produce the highest accuracy rate is recorded as our final model. For example, first, we applied all the variables in the ATP dataset to the logistic regression, and we found that these variables: “ATP points”, “ATP ranking”, “age”, and “professional years” are highly significant at a significance level of 0.01. The variables and hyperparameters we used in the models are summarized in S1 Table .
Model comparison
We compare the performance of prediction models, including statistical and machine learning models, to the proposed model in this section. The empirical data considered are the single men’s and women’s matches of Grand Slam tournaments (2000–2019). These data are separated into training data and testing data, and the model performance is based on the prediction results of the testing data. We should use the ranking as a baseline model that predicts the winner as the player with the highest ranking. The statistical and machine learning methods considered are logistic regression, support vector machine (SVM), neural network, and LightGBM (light gradient boosting machine), which are often used to predict the winners of tennis matches. Basically, we apply all available variables to all models and use the training data to construct models. For prediction performance, we chose accuracy and area under the curve (AUC) as the evaluation criteria.
For time sequence data, such as the 2000–2019 tennis matches considered in this study, there are two possible settings for applying cross-validation: moving window and non-moving window. The moving window technique is commonly used for time series data, and we choose the format of 4-year training data and 2-year testing data. For example, the first moving window is the 2000–2005 data, i.e., 2000–2003 as the training set and 2004–2005 as the testing set, and other moving windows follow the same rule. There are 15 possible combinations of moving windows, as illustrated in Fig 7 . For the non-moving window, if applying the same 4/2 year setting of training/testing sets, we randomly select 4 years (out of 20 years) of data for the training set and 2 years out of the remaining 16 years as the testing set. Although we think that the prediction results of the moving window approach are more appropriate for evaluation, we will provide those of the non-moving window approach as a reference.
https://doi.org/10.1371/journal.pone.0266838.g007
The proposed Glicko model considers the estimates of parameters in the Glicko model, in addition to other variables, in logistic regression. Note that the extra variables introduced by the Glicko model can be further expanded. For example, we found that the parameter estimates of the Glicko model are very different for the matches on clay courts compared to those on grass/hard courts. Thus, we can add court-related Glicko variables to the proposed model. The prediction results of men’s single matches (moving window) are shown in Table 2 (averages of 15 possible combinations). The differences in prediction accuracy between models are not very obvious, and even the baseline model (using the players’ rankings as the criterion) can reach 72.4% accuracy. Nevertheless, the proposed models have slightly better performance with respect to AUC and accuracy. It seems that more sophisticated models (e.g., SVMs and neural networks) do not necessarily produce more accurate predictions [ 7 , 20 ].
https://doi.org/10.1371/journal.pone.0266838.t002
For the women’s matches, the results are very similar ( Table 3 ), but the overall performances of men’s single matches are slightly better than those of women’s single matches. The Glicko model also has better performance in prediction, but adding the court-related variables does not cause any obvious improvements. Interestingly, the differences between the baseline model and statistical or machine learning models are very small. It seems that the ATP/WTA ranking contains plenty of information for predicting the winners of single matches in Grand Slam tournaments, and it is competitive against more complex models including all available variables. Additionally, similar to the results in previous studies, the prediction performances of all quantitative models are very similar.
https://doi.org/10.1371/journal.pone.0266838.t003
The prediction performances for the non-moving window case are approximately the same, and we show the results for the ATP and WTA in Table 4 . The proposed Glicko models still have a small edge over all other models, but the advantage is smaller in the non-moving window case. This result is fairly reasonable since the Glicko model follows a Bayesian approach and the parameters should be updated according to its time sequence. On the other hand, the baseline model produces approximately the same prediction performances as the statistical and machine learning models. This indicates that the ranking covers almost all essential information for predicting the match results, since players selected to play in Grand Slam tournaments are quite homogeneous (in terms of playing strength). A line of evidence of homogeneity in the strength of players is the winning probability of higher-ranked players being at most 80%, as shown in Fig 2 , and it is not easy to find over-whelming matches, even for the cases of top-seeded players vs. lower-ranked players.
https://doi.org/10.1371/journal.pone.0266838.t004
Conclusion and discussion
Predictive analytics has recently become very popular, and many people are looking to construct models to explain observed phenomena in the form of “Observations = Model + Error”, which is often expressed as y = f ( x 1 , x 2 , …, x k ) + ε . There are two keys to constructing the models: selecting appropriate variables x 1 , x 2 , …, and x k and choosing the proper functional form f (). Some studies focus on variable definition (or data structurization), while others emphasize enhancing model performance, depending on the study goal and the data availability. In this study, our goal is to find models with the highest accuracy in predicting winners of tennis matches, with the help of EDA to uncover important variables. Our conjecture is that once all relevant data are included, the role of model construction will be limited. This is analogous to “complete information” in economics, and the advantage of any players (or models) is very slim if all players have the same information.
Our study confirms this conjecture, and the results show that the models have a smaller impact on the prediction when the variable ranking is included. Many past studies had similar findings, and the prediction accuracies of all models were approximately the same e.g. [ 7 , 21 ]. It seems that the ranking carries almost all information and adding more variables does not significantly increase the prediction accuracy. Thus, it is not surprising that the improvement by the proposed Glicko models is not large, with a prediction accuracy approximately 1% higher than that of the other models (approximately 70%). Nevertheless, the proposed approach provides the possibility of defining new variables without separating the models, for example, by different court surfaces. Of course, empirical results are often data dependent, and our findings do not necessarily apply to other ATP/WTA tournaments, since we think that the players’ strength in Grand Slam matches is fairly homogeneous.
The ranking variable provides competitive accuracy in predicting the winners of single matches. If we can treat the ranking as a sufficient statistic for prediction, then we may want to know, for example, whether sufficient statistics exist and how we define sufficient statistics. According to the seeding rules of Grand Slam matches, it seems that the public thinks that the ranking is a sufficient statistic and that the points earned in tournaments for the past 52 weeks are how the ranking should be decided. The Glicko model suggests an alternative index for measuring players’ strength. It would be interesting to explore whether we can improve the ATP/WTA ranking by applying a Bayesian approach.
Combining experts’ opinions and quantitative analysis is another way to construct prediction models. Relying solely on historical data and machine learning models overlooks the value of domain knowledge, ignoring the merit of human wisdom. However, only a few studies have mentioned the possibility of combining the prediction results of human judgements and data analysis [ 20 ]. On the other hand, experts’ opinions are similar to unstructured data, and it is difficult to format our thoughts into numerical values, i.e., perform structurization. This structurization process is crucial and would require personal intelligence and awareness of the problem. Perhaps we can adapt the idea used in the Delphi method, a popular method for structuring the opinions of a group of experts. Once human judgements are quantified, the remaining question might be how to properly weight human opinions and data analysis.
Supporting information
S1 table. hyper parameter and variables for each statistical and machine learning model..
https://doi.org/10.1371/journal.pone.0266838.s001
- 1. Somboonphokkaphan A, Phimoltares S, Lursinsap C. Tennis winner prediction based on time-series history with neural modeling. In: Proceedings of the International MultiConference of Engineers and Computer Scientists. vol. 1; 2009. p. 18–20.
- View Article
- Google Scholar
- 5. Hostavcný, Jakub p year = 2018. Non-Linear Classification as a Tool for Predicting Tennis Matches;.
- 18. Stephenson A, Sonas J, Stephenson MA, Elo G. PlayerRatings: Dynamic Updating Methods for Player Ratings Estimation. R package version 1.1-0. 2020;.
- PubMed/NCBI
- 21. Cornman A, Spellman G, Wright D. Machine learning for professional tennis match prediction and betting. Stanford Unverisity. 2017;.
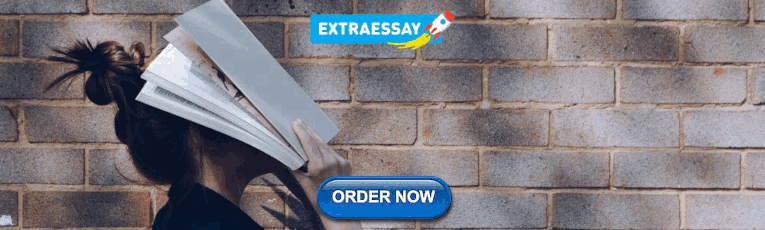
IMAGES
VIDEO
COMMENTS
CASE STUDY THE TENNIS MATCH. INSTRUCTIONS: (30 pts) Read and understand the case and make your own analysis. The facts are given. All you need is to supply the needed information by answering what is being asked in the given situation.
Case Study: The Tennis Match Noel is a computer programmer at Jonadel Corporation. He was delighted to know that part of the company's athletic program is a mixed doubles tennis tournament to be. Q&A. On January 1, 2023, psalm and trisha formed PS Partnership. The articles if co-partnership provides that profit or loss shall be distributed ...
View CASE STUDY ANALYSIS #9_BA150.docx from BSBA MARMA 201 at Capitol University - Corrales Ext., Cagayan de Oro City. CASE STUDY #9 THE TENNIS MATCH INSTRUCTIONS: (30 pts) Read and understand the
Case Study Title- The Tennis Match.docxv#9 - Free download as Word Doc (.doc / .docx), PDF File (.pdf), Text File (.txt) or read online for free.
Case Study 9: Problems: Lack of coverage for non-work-related injuries: The company and the ECC did not cover the injury of Noel that was not in the field of employment as defined by law. Exhaustion of sick and vacation leave: His sick and vacation days are over since it is a non-work-related injury.In addition, as per the company's policy and ECC policy, non-work-related injuries are not covered.
CASE STUDY 9 THE TENNIS MATCH Noel is a computer programmer at... School name. University of the Philippines Diliman
This is a theory proposed by. evolutionary psychologists. Herbert is a 32-year-old lawyer seeking treatment for his irrational fear of thunderstorms. He has had this fear since the age of 4, and throughout life he has developed various strategies for coping with his fear. Whenever possible, he avoids going outside when a storm is forecast.
Read and understand the case and make your own analysis.information by answering what is being asked in the given ... you need help with along with a budget and time limit. Questions are posted anonymously and can be made 100% private. Match with a Tutor ... African Studies ...
CASE STUDY THE TENNIS MATCH. INSTRUCTIONS: (30 pts) Read and understand the case and make your own analysis. The facts are given. All you need is to supply the needed information by answering what is being asked in the given situation. FACTS: Noel is a computer programmer at Jonadel Corporation.
The Average Number of Points Per Match in College Tennis. On average, these matches had a total of 130.8 points. The match winner averages 71 points won. The match loser averages 59.8. So the difference between winning and losing, on average, is 11.2 points. That's less than 1 point per game, not a huge difference.
1/5. CASE STUDY. THE TENNIS MATCH. Noel is a computer programmer at Jonadel Corporation. He was delighted to. know that part of the company's athletic p rogram is a mixed double s tennis tournament. to be held at t he corporate tennis court. Grace, who works in the same department,
**Case Study 9: The Tennis Match** **Problems:** 1. **Inadequate Leave Coverage:** Noel, a computer programmer at GIAV Corporation, faced a significant problem when he suffered a tennis-related injury, depleting his sick and vacation leave. Furthermore, the company's policies did not cover non-work-related accidents, leaving him without adequate leave benefits to recover.
Case Study 3 - The Tennis Match - Free download as Word Doc (.doc / .docx), PDF File (.pdf), Text File (.txt) or read online for free. Noel was injured during a company-sponsored tennis tournament and required a two-day hospital stay. As a result, he missed two days of work but had no remaining sick or vacation days. The document recommends that the company take responsibility for ensuring the ...
Lawn Tennis - middle class, Wingfield sold the game in a box, emancipation of women 1877 - lawn tennis at Wimbledon with 22 competitors Public Schools One: Informal hand and ball games against walls Two: Some fives courts built, racquets started to develop with squash Three: Lawn tennis low in boys but popular in girls
CASE STUDY THE TENNIS MATCH. INSTRUCTIONS: (30 pts) Read and understand the case and make your own analysis. The facts are given. All you need is to supply the needed information by answering what is being asked in the given situation. FACTS: Noel is a computer programmer at Jonadel Corporation.
He was delighted to know that part of the company's athletic program is a mixed doubles tennis tournament to be held at the corporate tennis court. Grace, who works in the same department, used to play with Noel on many occasions and the two would like to compete in the tournament. ... Dear student as per our guidelines we can answer only 1 ...
5 Video Tips for Coaches. The Video Revolution: How & Why the Best Tennis Coaches are Using Video for Player Development. 2,326 Match Case Study: How Women's College Players Win Matches. 2,086 Match Case Study: How Men's College Players Win Matches. Improve Your First 4 Shots in Singles. How to Improve Your Return Strategy in Singles: Our ...
Name: Niña Alexa B. Gomez Course/Year: BSBA-MM/First Year Subject/Schedule: HR_1/1:30PM-3:00PM (Tuesday, Thursday) Case Study Title: The Tennis Match Problem: Noel had an injury during the tennis match tournament. Because of this, he missed two days of his work and has no more sick or vacation leave. Unfortunately, the company and ECC policy do not cover non-related work accidents.
Tennis is a popular sport, and professional tennis matches are probably the most watched games globally. Many studies consider statistical or machine learning models to predict the results of professional tennis matches. In this study, we propose a statistical approach for predicting the match outcomes of Grand Slam tournaments, in addition to applying exploratory data analysis (EDA) to ...
Assignment #1 (Week Three): Case Assignment: "The Tennis Match: What Would You Do?" 50 points. Develop a traditional academic essay. Submit your assignment at the end of class latest, Week 3. INSTRUCTIONS : Read the scenario below "A question of ethics" and develop an essay based on the prompts below the scenario. A QUESTION OF ETHICS After the intramural, mixed-doubles tennis matches ...
Management of Performance Appraisal System Case Study - Free download as Word Doc (.doc / .docx), PDF File (.pdf), Text File (.txt) or read online for free. A case study analysis of Entrep