
An official website of the United States government
Here’s how you know
The .gov means it’s official. Federal government websites often end in .gov or .mil. Before sharing sensitive information, make sure you’re on a federal government site.
The site is secure. The https:// ensures that you are connecting to the official website and that any information you provide is encrypted and transmitted securely.
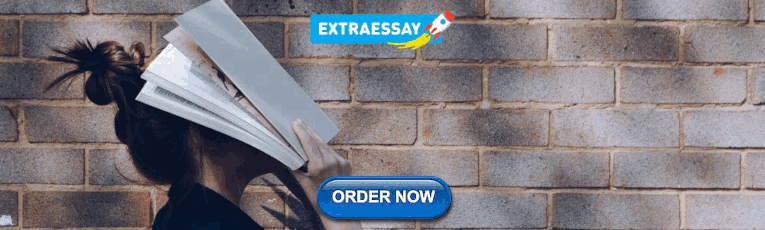
Executive Summary
Lessons learned, related information, department of transportation case study: enterprise data inventory.
In response to the Open Government Directive, DOT developed a strategic action plan to inventory and release high-value information through the Data.gov portal. The Department sustained efforts in building its data inventory, responding to the President’s memorandum on regulatory compliance with a comprehensive plan that was recognized as a model for other agencies to follow.
Department of Transportation
Case studies & examples
Originally published January 8, 2013
Open Data at DOT - A Case Study for Maturing Data Release Practices to Drive Innovation and Increase Accountability
Kristen Baldwin, Associate Chief Information Officer for IT Policy Oversight Office of the Chief Information Officer, Department of Transportation
The DOT performs a wide range of business services aimed at solving the complex safety issues associated with our Nation’s transportation systems. The DOT relies heavily on its data resources to drive business and investment decisions. These include data compiled by State, local, and tribal governments, as well as private sector entities. The Department is committed to unlocking more public data to:
- Drive innovation by tapping into the ingenuity of the American people;
- Increase agency accountability; and
- Solidify the connection between the Department’s services and individual citizens, business, governmental bodies, universities, and other non-government organizations.
In response to the Open Government Directive , DOT developed a strategic action plan to inventory and release high-value information through the Data.gov portal. In the winter of 2010, the DOT Associate Chief Information Officer for IT Policy Oversight, serving as the Senior Accountable Official for Open Government, convened a wide-ranging group of stakeholders from around the Department to develop the required strategic action plan. The plan was detailed in DOT’s Open Government Plan, version 1.2 (released on June 25, 2010), and included three tracks:
- Strategy : In the near-term, transform DOT’s data release posture and in the long-term, sustain openness.
- Policy : Provide guidelines for identifying datasets and prioritizing them for release. Institute standing policies for approving, publishing, and managing releases.
- Inventory : Build list of source information systems using budget exhibits. Compare these against data already released on websites and against information collections. Utilize these lists to develop a complete inventory of datasets, prioritize it, and sequence data for release.
DOT detailed the results of its strategic action plan on its open government Web page . The Department sustained efforts in building its data inventory, responding to the President’s memorandum on regulatory compliance with a comprehensive plan that was recognized as a model for other agencies to follow.
The DOT has a long-standing tradition of making data available to the public. As a partner agency involved in the stand-up of Fedstats.gov, now USA.gov , the Bureau of Transportation Statistics helped to lead the development of an important pre-cursor to Data.gov . In addition, the DOT operates a number of transparency portals that provide information on railroad safety , commercial motor vehicle safety , vehicle safety , and pipeline safety . The Department’s leaders identified a number of fundamental issues that must be considered in order to provide sustained access to high-value transportation data. Many of these issues are being addressed and managed through internal policies. These issues include:
- Determining a process to release data and contextualize that data;
- Creating a data inventory and selecting the right datasets to release;
- Determining how to approach developing a DOT-wide data architecture;
- Providing data in a format usable by industry and individuals, consistent with security, privacy and confidentiality interests;
- Portioning and organizing the data so that it is manageable and searchable; and
- Maintaining the quality of the data and creating and maintaining a dialogue with stakeholders about changes in data availability.
To achieve the objectives outlined in the Open Government Directive and address the concerns outlined above, DOT developed a strategic action plan, outlined below:
The plan contained three primary tracks. The Strategy track provided overarching guidance for the Department’s approach to information access. In the short term, DOT’s strategy focused on achieving the open data objectives outlined in the Open Government Directive . The Department developed longer-term information access strategy that addresses the methods for creating enduring access to the Department’s data. These strategies are underpinned by policies focused on approving and publishing data and the necessary procedures to managing those data releases. Finally, the Department met Open Government Directive requirements for identifying and releasing high value data using a structured, standard process for creating its data inventory .
The DOT data inventory process involved a review of the Department’s information technology (IT) portfolio and data that is already released on DOT Web sites. Information that was published in an unstructured format (for instance, embedded in reports) was traced back to its source information system wherever possible. This step was necessary to ensure that the release of underlying structured data (for instance, data that are included in tables or used to generate graphs inside a report) could be automated from an authoritative source. Further, the Department reviewed its active and expired information collections (authorized under the Paperwork Reduction Act (PRA)) and its Electronic Information Systems inventory (required to meet Federal records management requirements) to trace these items back to the appropriate authoritative information systems. To assist in maintaining this inventory, DOT leveraged its Enterprise Architecture to develop a registry. The DOT Chief Enterprise Architects also developed a structured process to identify and prioritize datasets for release.
The DOT’s Open Government Policy Working Group, that was convened to recommend a policy development strategy to senior leadership, consisted of subject matter experts from policy, budget, performance, strategic planning, human resources, technology operations, and legal. Developing a unified Open Government policy for the DOT was a large task. Some major policy areas were well understood, including policies around data transparency and the selection and use of social media tools. However, to create a policy framework that drives towards the DOT’s strategic goals for Open Government, a unified policy DOT considered more than those well-understood areas. The figure to the right outlines DOT’s approach to an open government policy framework.
Once the stage was set for employees to understand what tools are available to increase public engagement in their daily work, the Department explored establishing processes for decision-makers to prioritize the Department’s resources in posting information. Those processes ensured that information posted is “releasable” and “usable” both in content and format.
Content releasability is determined with value, quality, security, privacy, and confidentiality interests in mind. Format guidelines differ depending on whether the information is structured or unstructured. The DOT will continue to release DOT data in a timely manner by proactively making it available online in consistent, open formats, while assuring accuracy and protecting privacy, security, and confidentiality.
The DOT has released 765 datasets or tools to Data.gov. The processes and policies that have been established under DOT’s approach to Open Government helped DOT build a comprehensive plan responding to the President’s memorandum on regulatory compliance that was recognized as a model for other agencies to follow. In May of 2012, DOT, in partnership with the Department of Justice, Department of Labor, and the Consumer Product Safety Commission, launched Safety.Data.gov with 713 datasets, four (4) mobile apps, 14 resources and public domain software tools, and three (3) challenges or competitions. In September 2012, we held the first safety datapalooza, and the community grew to 869 datasets, 10 mobile apps, and six (6) challenges or competitions encouraging public use of safety data from around the government.
DOT has already recognized benefits as a result of regulatory transparency efforts. For example, for the first time, FMCSA has begun releasing the information behind its Safety Measurement System (SMS) to the public in bulk, downloadable formats. Business and private citizens are mining this data for potential opportunities. For instance, a windshield repair company looking to grow its business is mining FMCSA information about safety violations to identify new customers. DOT and the public realize a benefit because the commercial vehicle operator customer is using a safer vehicle and operating in compliance with Federal Motor Carrier Safety Regulations. In addition, DOT has pursued innovation in its data release practices:
- FMCSA released an API and mobile application to help people easily access a bus company’s safety performance record, file a complaint and more.
- FRA released public access to its safety data APIs .
- Transparency, collaboration, and participation depend upon information which is available, easy to find, and easily manipulated, aggregated, and/or re-published. While this task is daunting for an enterprise such as the DOT, it can be achieved incrementally. Getting a handle on the data inventory and ensuring that the public can find that information is an important first step, and improvements in release practices can be prioritized as a second step.
- While CIOs have a great detail of statutory authority and responsibility for open data, they must engage with policymakers and business process owners to develop a complete inventory of datasets and understand their releasability. In developing the Department’s response to the President’s memorandum on regulatory compliance, the CIO collaborated closely with the Office of General Counsel. Similarly, in building the safety community on Data.gov, the CIO collaborated closely with the DOT Safety Council.
- Ensuring that all of the DOT datasets were listed on Data.gov provided a solid foundation for further innovation. DOT took the vision for the safety community on Data.gov from concept to functioning prototype in just five months. The existing data releases helped us frame the foundational categories and taxonomy for the community, and it enabled us to quickly bring other Federal agencies on board. The shared, centralized platform of Data.gov was essential to this rapid development process.
- DOT Open Government
- DOT Data Inventory
- DOT Regulatory Compliance Plan
- DOT on Data.gov
References to the product and/or service names of the hardware and/or software products used in this case study do not constitute an endorsement of such hardware and/or software products.
resources.data.gov
An official website of the Office of Management and Budget, the General Services Administration, and the Office of Government Information Services.
This section contains explanations of common terms referenced on resources.data.gov.

Case studies across North America
With more than 10,000 projects every month, StreetLight’s analytics have informed transportation analyses in all lower 48 States, Canada and Mexico. Below are some of the entities using StreetLight, followed by numerous case studies we invite you to peruse.

CITIES & MPOs
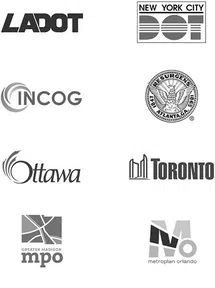
CONSULTING FIRMS

Read about our customers' successes
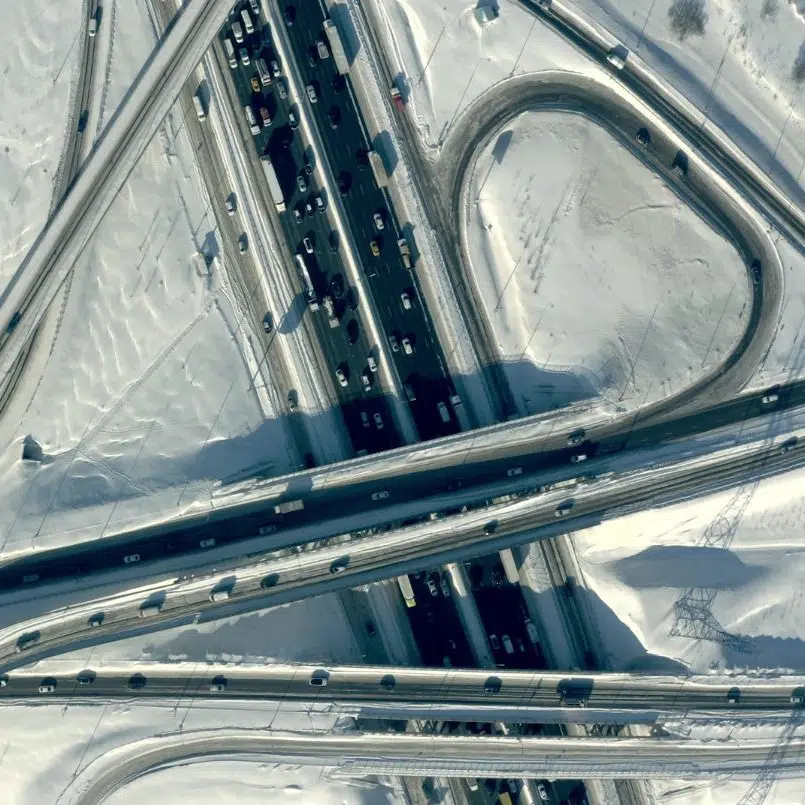
Analytics Secured Federal Funding for Columbus
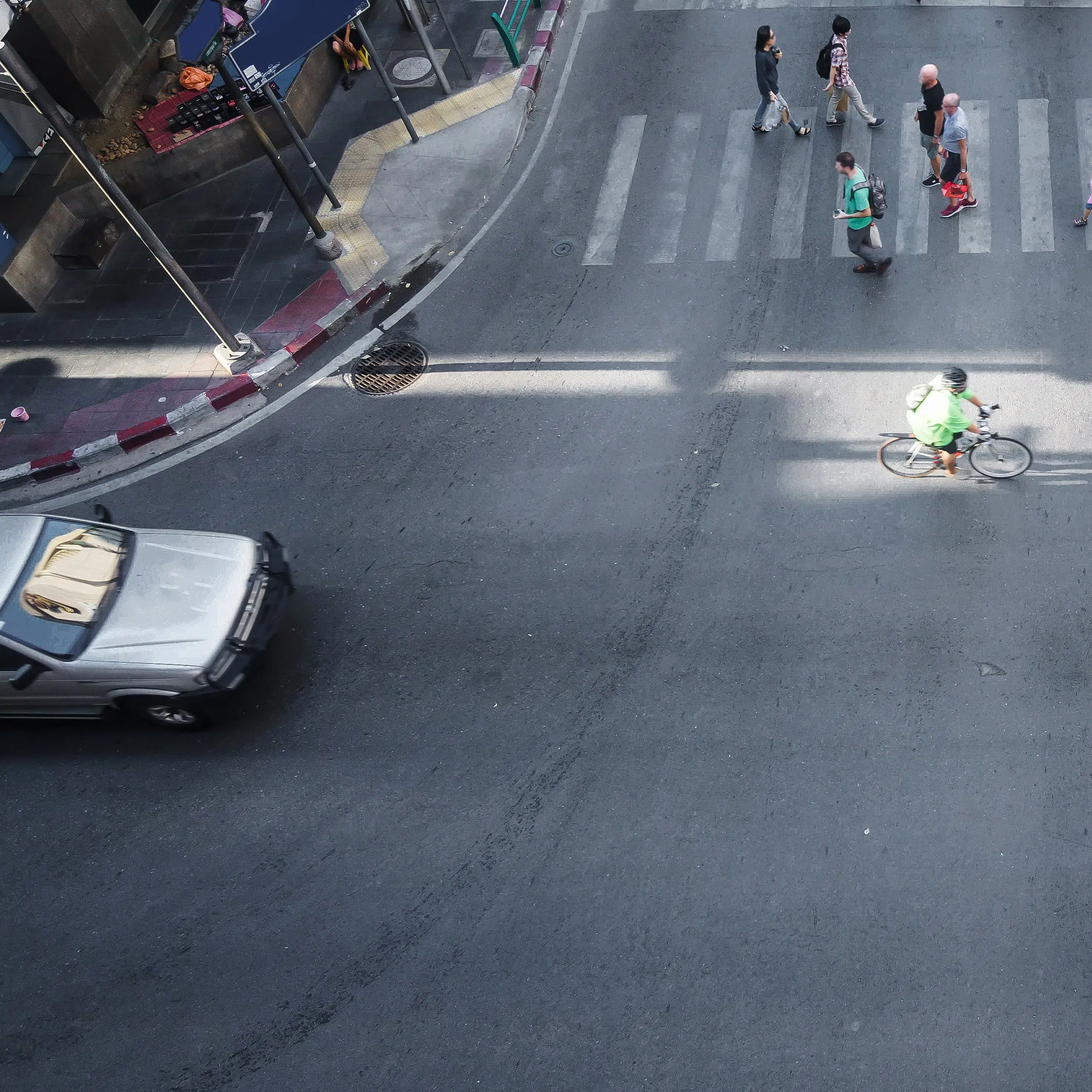
Enhancing Bicycle and Pedestrian Safety in Pittsburgh

Know Where to Place EV Charging Station Locations

Cost-Effective Congestion Analysis in Ft. Lauderdale
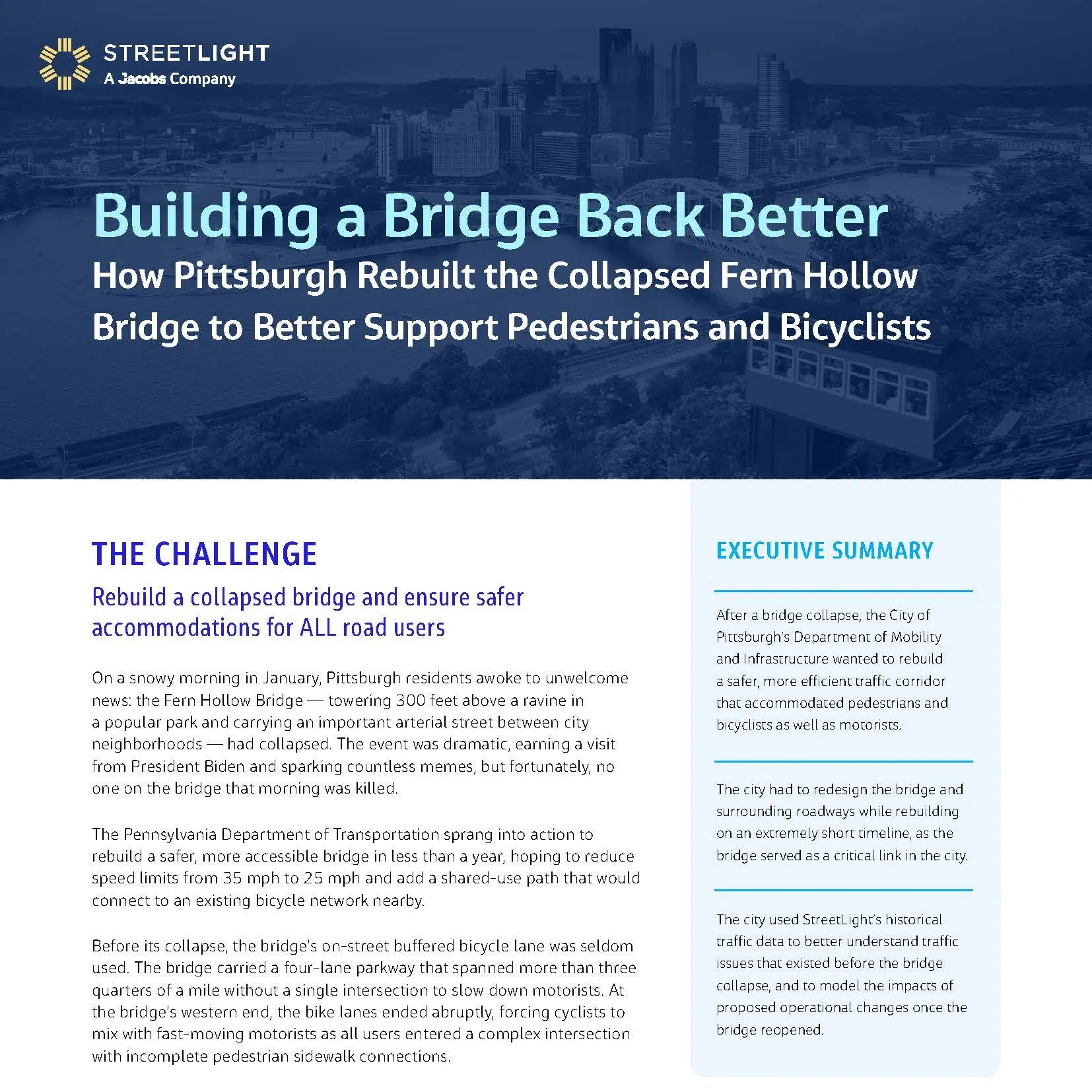
How Pittsburgh Built Bike and Pedestrian Safety Into Fern Hollow Bridge After Its Collapse
After the Fern Hollow Bridge collapse made headlines, the City of Pittsburgh needed to rebuild quickly. See how they used traffic data from StreetLight to meet tight deadlines while also improving bike and pedestrian safety on this important connector.
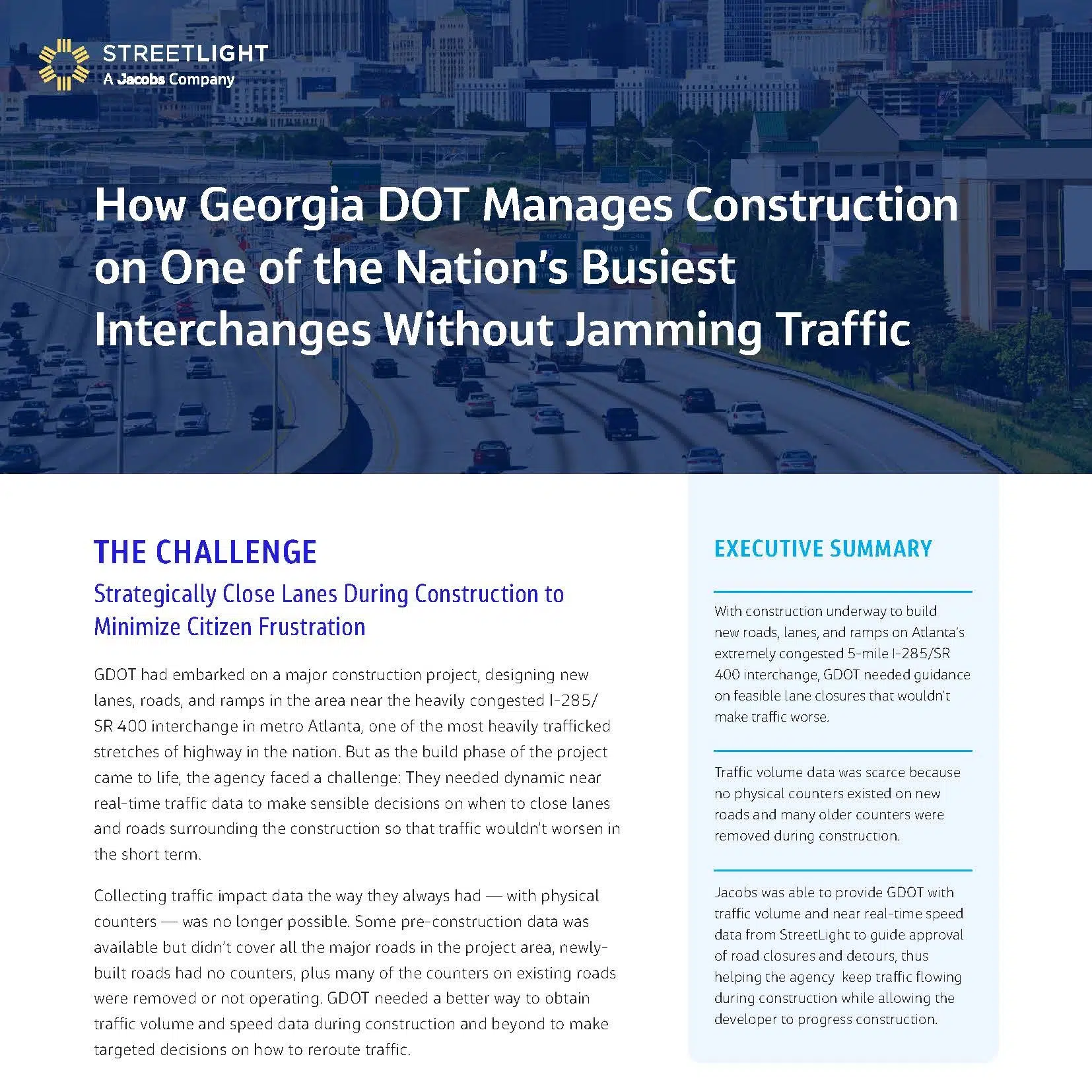
How Georgia DOT Manages Construction on One of the Nation’s Busiest Interchanges
To improve safety and fix congestion along a major highway in Atlanta, Georgia DOT needed to construct new roads, lanes, and ramps around the hectic I-285/SR 400 interchange. See how they used StreetLight to ensure lane closures and detours wouldn’t worsen traffic as developers worked to complete the re-design.
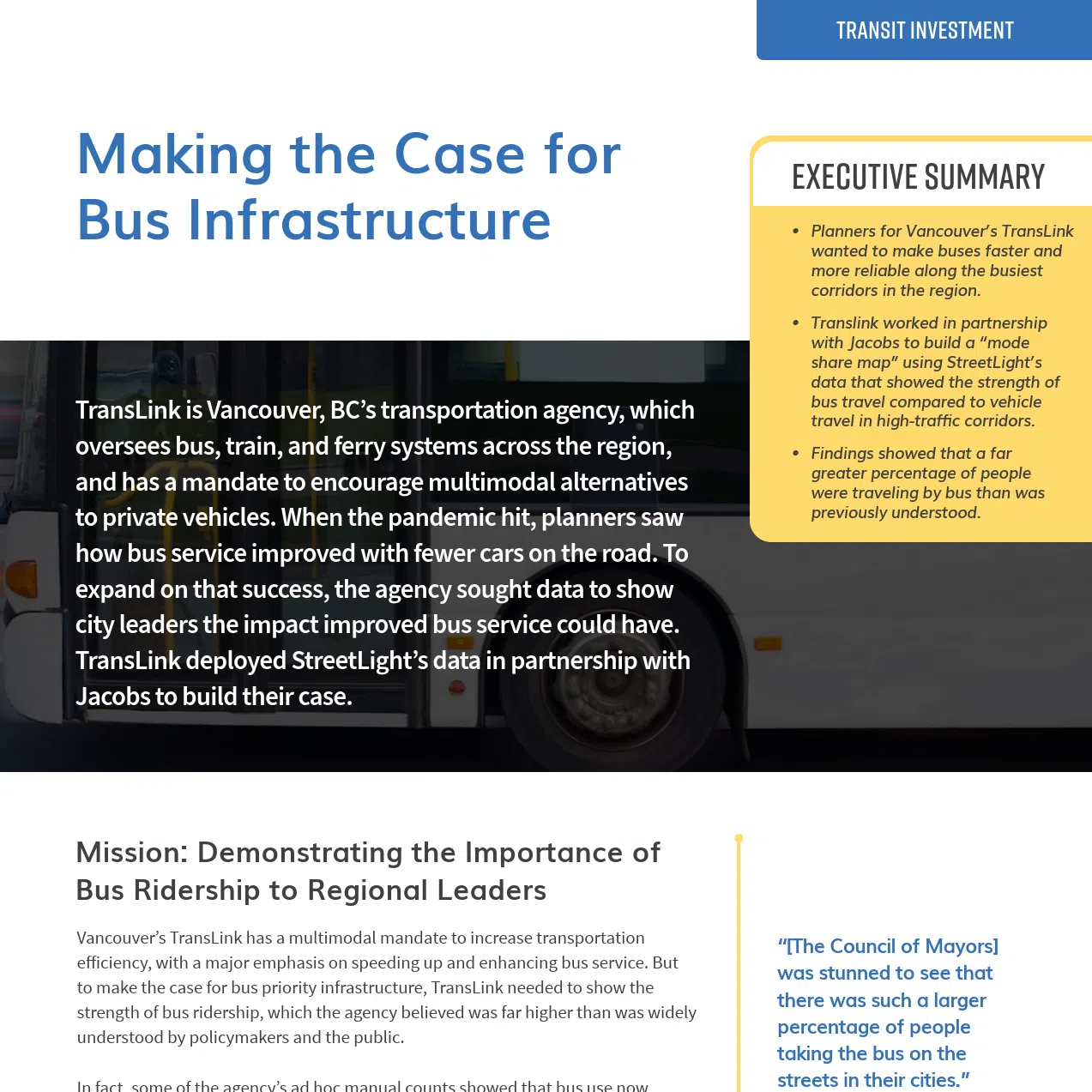
Making the Case for Bus Infrastructure
To encourage multimodal alternatives to personal vehicles and increase transportation efficiency, Vancouver’s TransLink needed to prove the value of bus service and show how strong bus ridership was compared to vehicle activity.
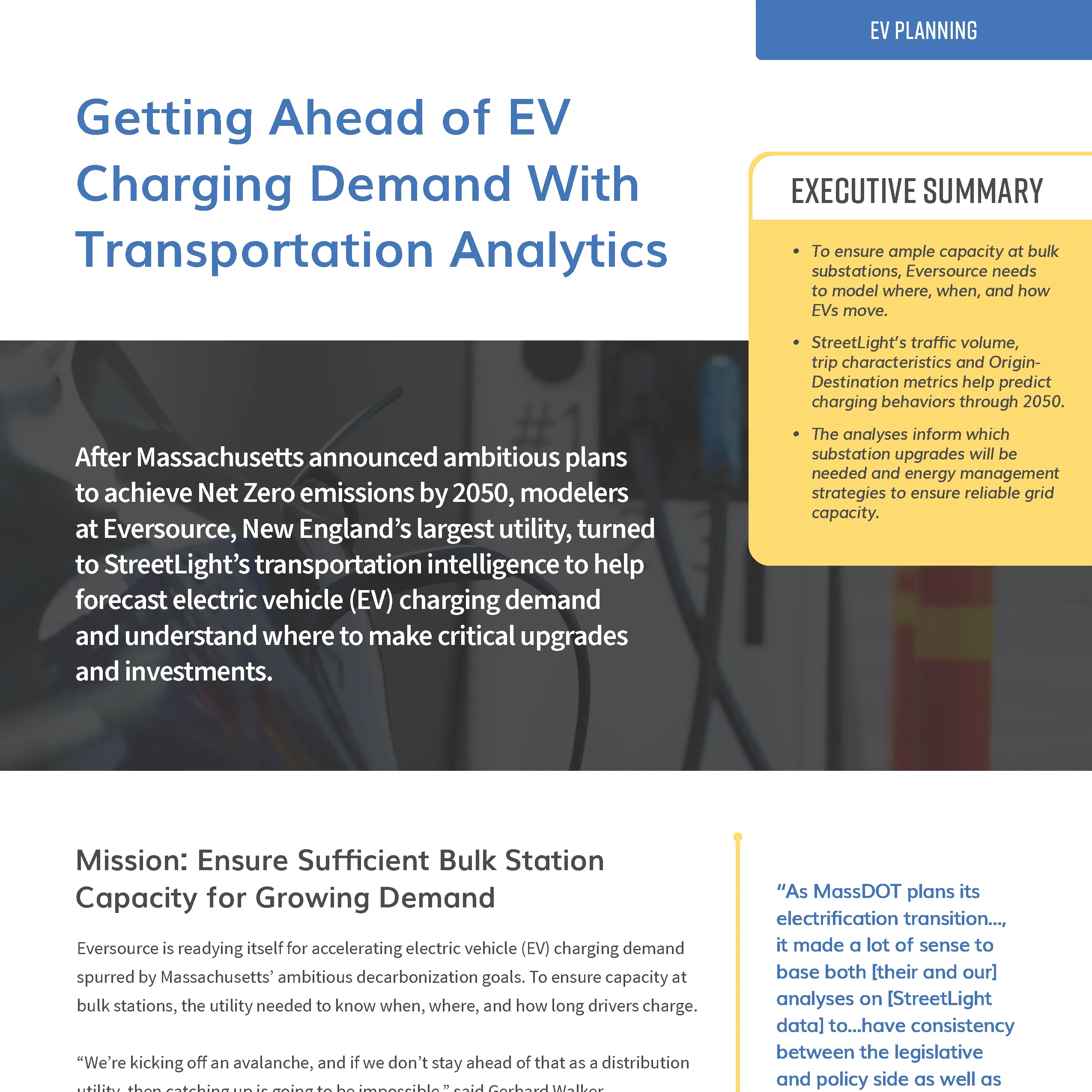
Getting Ahead of EV Charging Demand With Transportation Analytics
To ensure charging capacity keeps up with accelerating demand at electric vehicle charging stations, Massachusetts’s largest electric utility powers their demand models with mobility data.

To Improve Safety and Congestion, Expedite Signal Retiming with Big Data
See how Temecula used on-demand Turning Movement Counts to optimize signal timing, reduce congestion, and make intersection safer throughout the city.

How a Breakfast Chain Chooses New Store Locations That Drive Sales
To enhance its site selection analysis, a quick-serve breakfast chain used StreetLight to map travel behaviors and choose sites along high-traffic commuter routes.

Streamlining a Corridor Study With On-Demand Traffic Data
To understand existing conditions on PA Route 28, local agencies needed to undertake a rigorous corridor study. On-demand analytics from StreetLight helped stretch their budget, streamline data collection, and widen the scope of analysis.

Who Visits & When? Big Data Sheds New Light On State & Regional Park Access
To improve park management across all Minnesota state parks, researchers used StreetLight to gather park visitation data that shed light on time trends and visitor demographics.
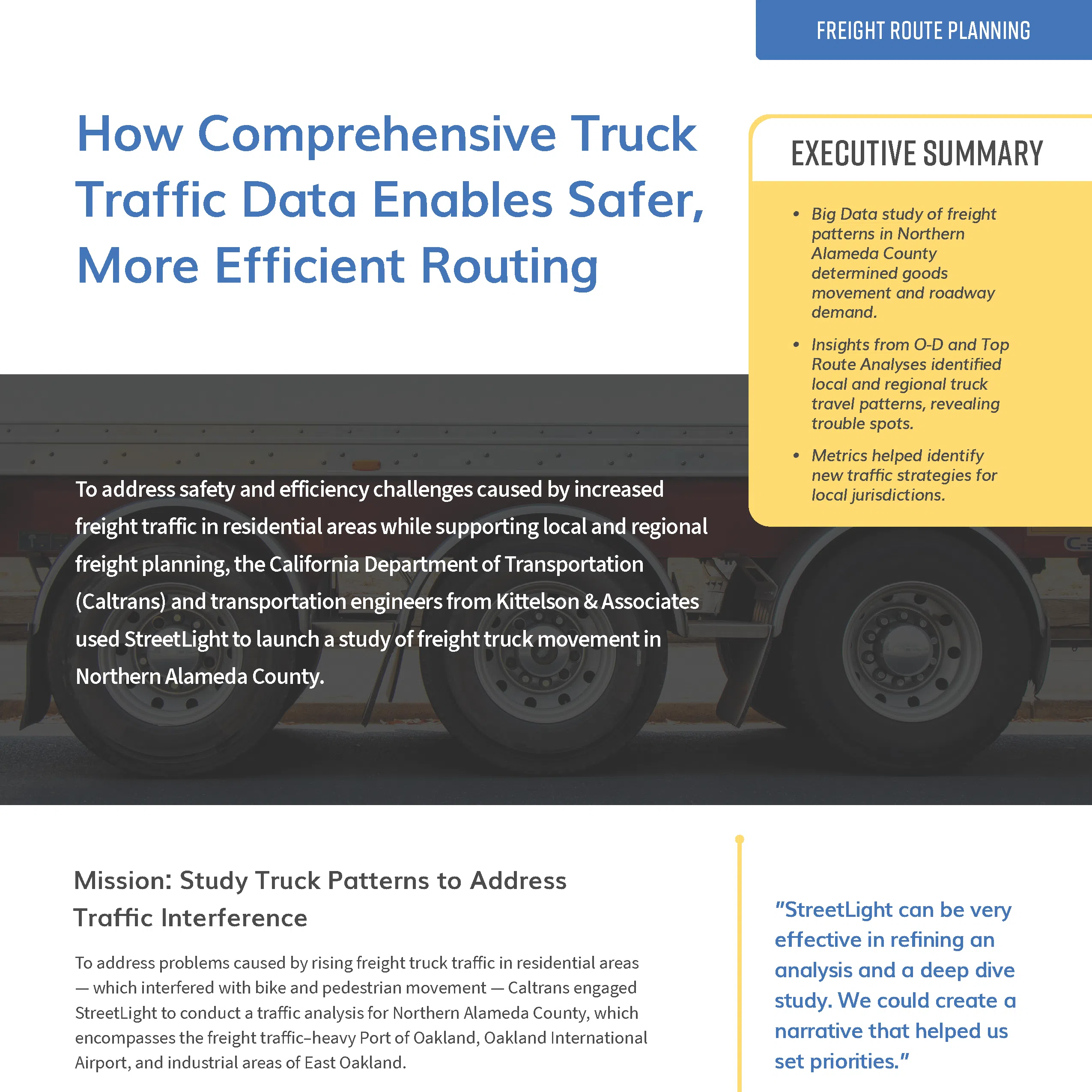
How Freight Traffic Data Enables Safer, More Efficient Truck Routing
To address issues caused by rising truck traffic in residential areas, Caltrans tapped StreetLight to analyze roadway freight traffic data in Northern Alameda County.

Using Big Data to Address Data Gaps and Validate Travel Demand Models
Learn how the City of Edmonton, Canada, used StreetLight InSight® to fill in their traffic volume data gap, validate their regional travel demand model and analyze insights to understand the changing mobility landscape.

Congestion Management Analytics Help Improve Traffic Flow and Road Safety
See how transportation planners in the Shreveport, Louisiana region partnered with StreetLight, saving time and resources to plan congestion management strategies, increase safety, and improve mobility in the region.
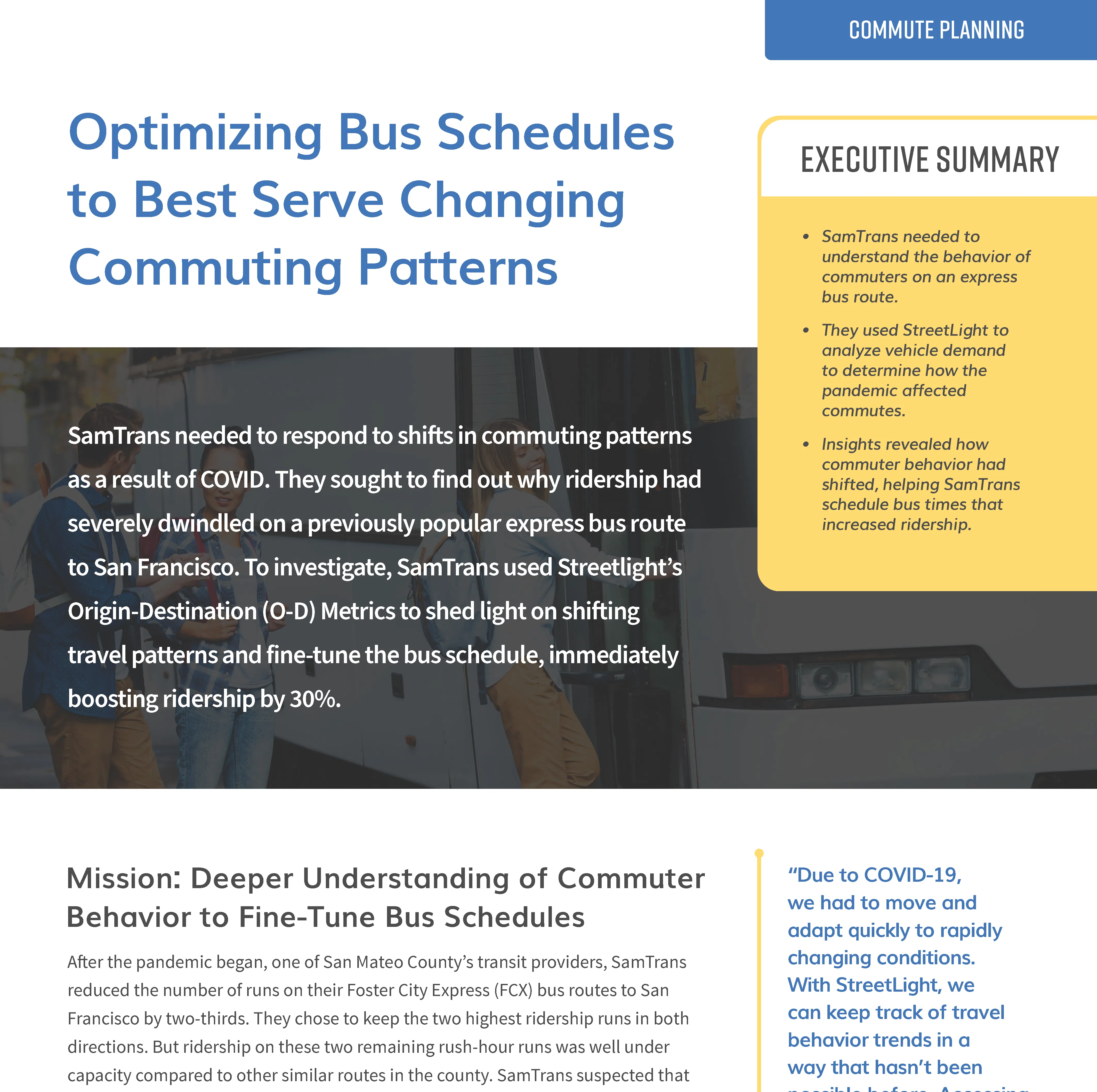
Optimizing Bus Schedules to Best Serve Changing Commuting Patterns
Learn how SamTrans used StreetLight InSight® to measure commute demand in the Bay Area to adjust bus times, leading to increased ridership.
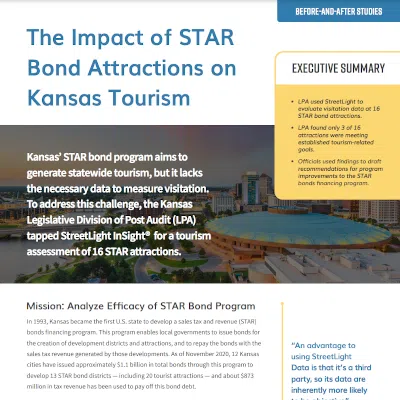
The Impact of STAR Bond Attractions on Kansas Tourism
Learn how the Kansas Legislative Division of Post Audit used StreetLight InSight® to measure before-and-after tourism visitation data at 16 state attraction sites, leading to surprising findings about the program’s success.

Sharing Regional Transportation Emissions Data
Learn how Streetlight helped the Twin Cities Metropolitan Council measure individual communities’ emissions from passenger and commercial vehicles.
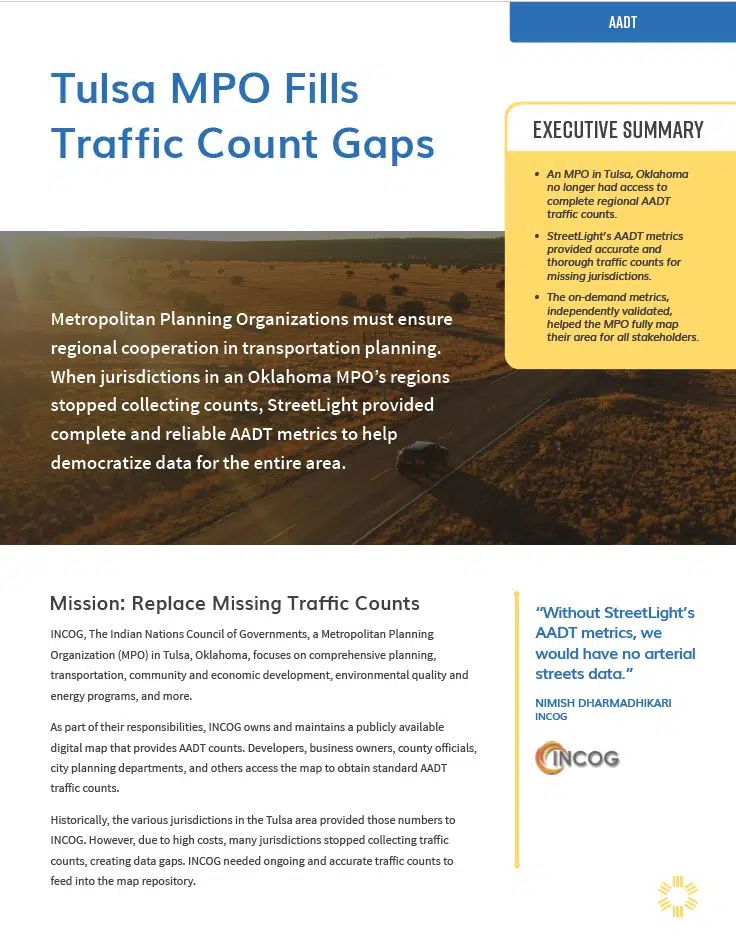
Tulsa MPO Fills Traffic Count Gaps
When jurisdictions in an Oklahoma MPO’s regions stopped collecting counts, StreetLight provided complete and reliable AADT metrics for the entire area.
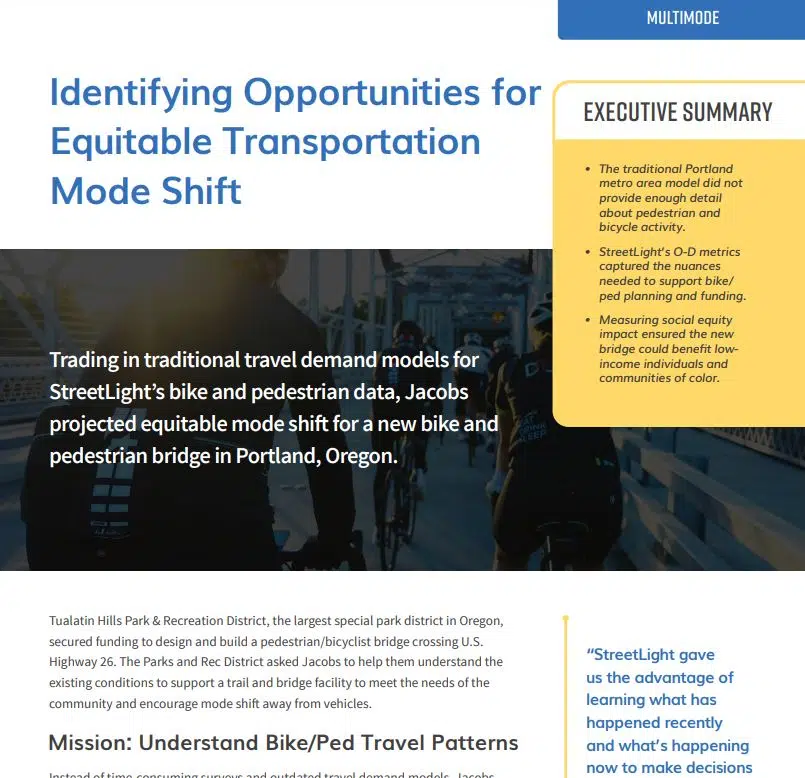
Opportunities for Equitable Mode Shift
Trading in traditional travel demand models for StreetLight’s bicycle and pedestrian data, Jacobs projected mode shift for a bike-ped bridge in Portland.
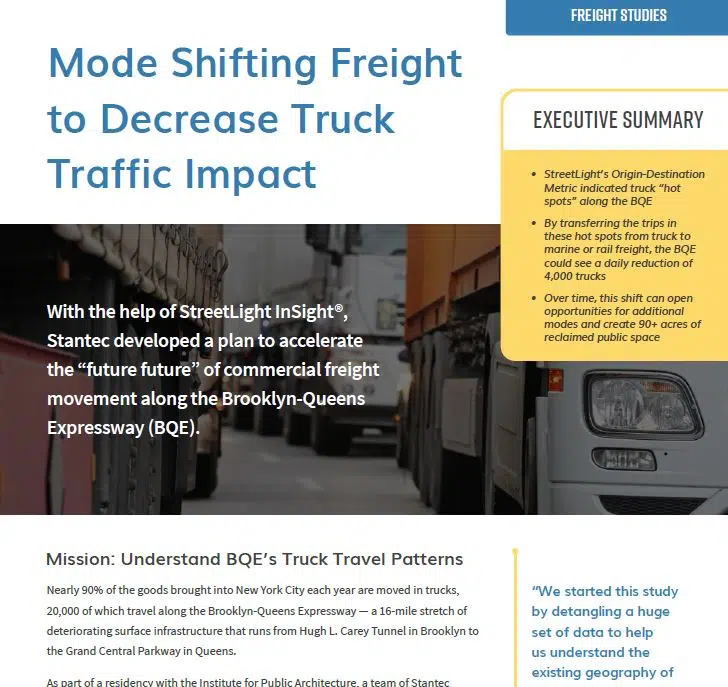
Shift Freight Modes for Truck Traffic Control
Stantec developed a truck traffic control plan along the Brooklyn-Queens Expressway to shift modes to marine vessels, reduce freight demand on the BQE, and enable other uses for the corridor
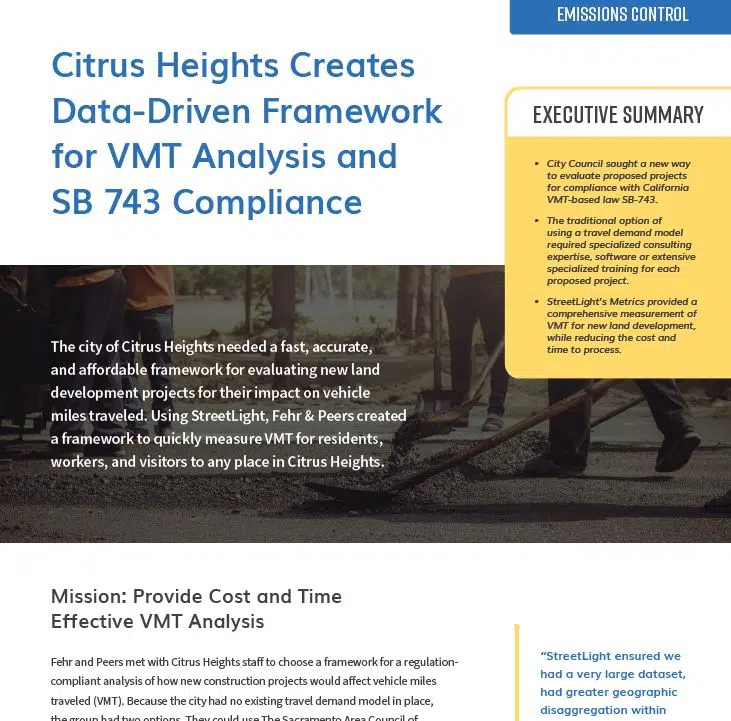
Unlimited VMT Calculations for California SB 743 Compliance
StreetLight’s Metrics offer unlimited measurements for California SB 743 compliance, while reducing cost and process time.

Dwell Time Analysis for National Parks
The amount of visitors in national parks has grown steadily over recent decades, leading to overcrowding. StreetLight’s dwell-time analysis helped the National Park Service better understand visitor-use management.
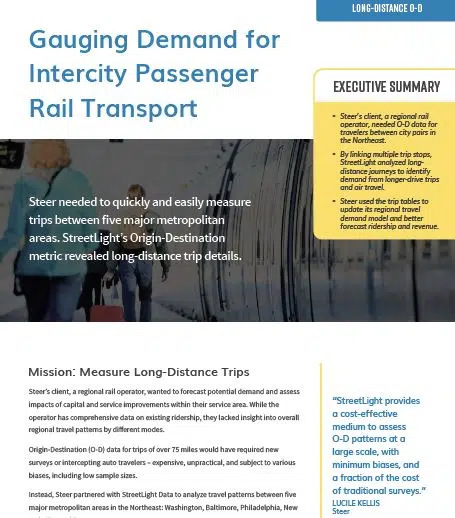
Gauging Potential Demand for Intercity Passenger Rail Transport
When a rail operator needed travel patterns between five major metropolitan areas in the Northeast to identify travel demand, StreetLight’s customized solution linked consecutive trip stops into a longer tour, using adjustable temporal thresholds.
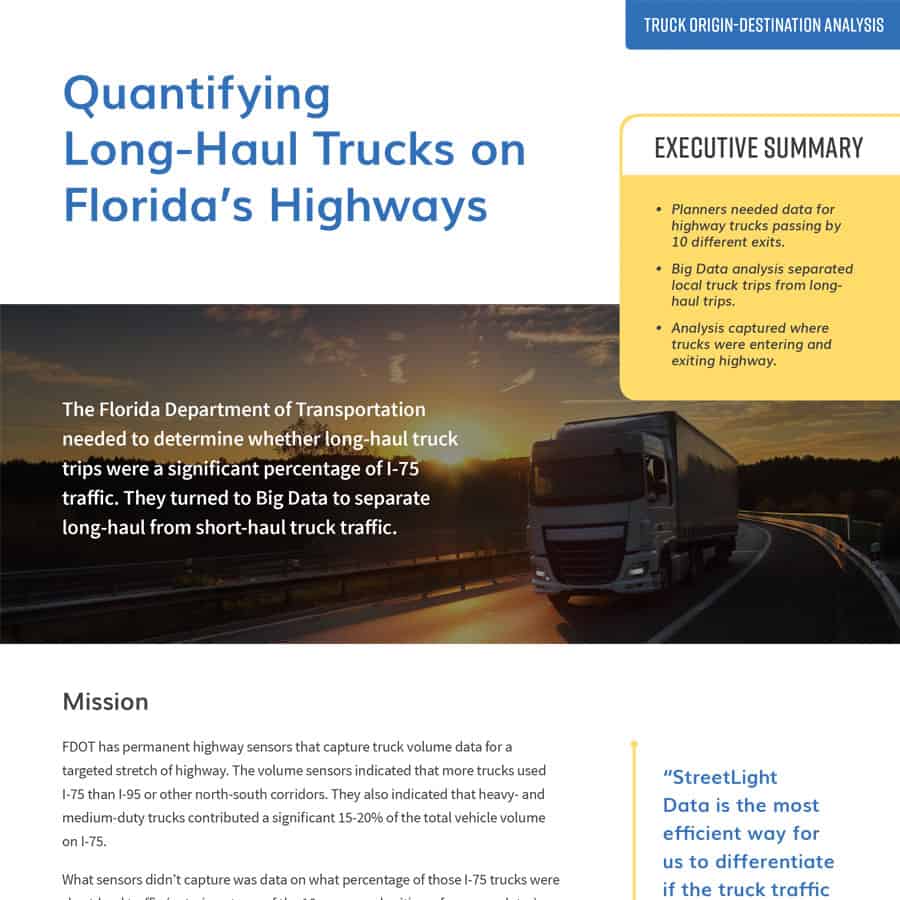
Quantifying Long Haul Trucks on Florida Highways
StreetLight identified what percentage of truck traffic was short-haul (entering or exiting at a ramp) vs long-haul (passing straight through the corridor).
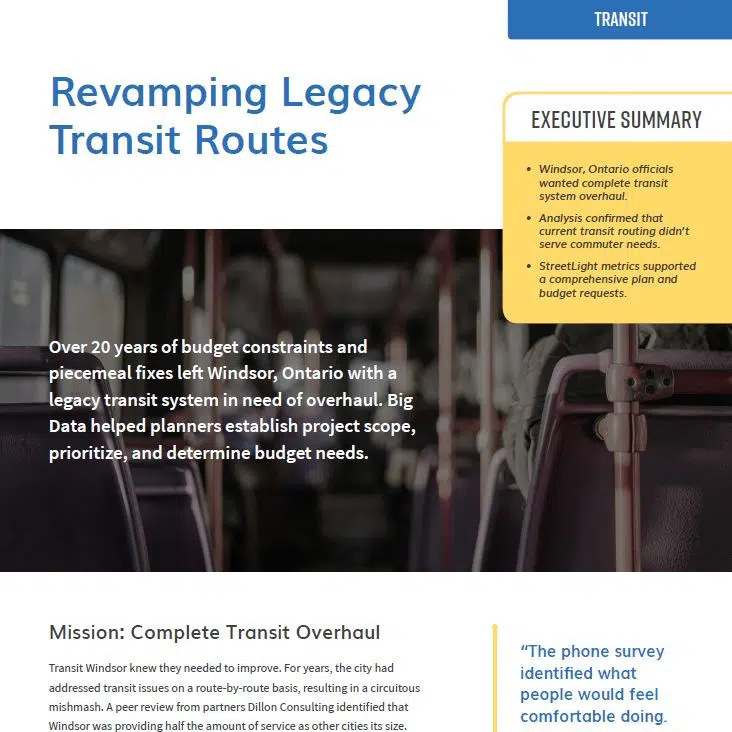
Revamping a Legacy Transit Plan in Ontario
Over 20 years of budget constraints left Windsor, Ontario with a legacy transit system in need of overhaul. Big Data helped planners establish project scope, prioritize, and determine budget needs.

Golden Gate Park Visitor Patterns
Golden Gate Park needed to count visitors through unsanctioned entrances. With StreetLight’s help, they got counts, trip origins, demographics, and more.

Pinpointing Visitor Volume at California Park System
To balance public access and its environmental impact, the Nature Reserve of Orange County turned to StreetLight for detailed park visitor data.
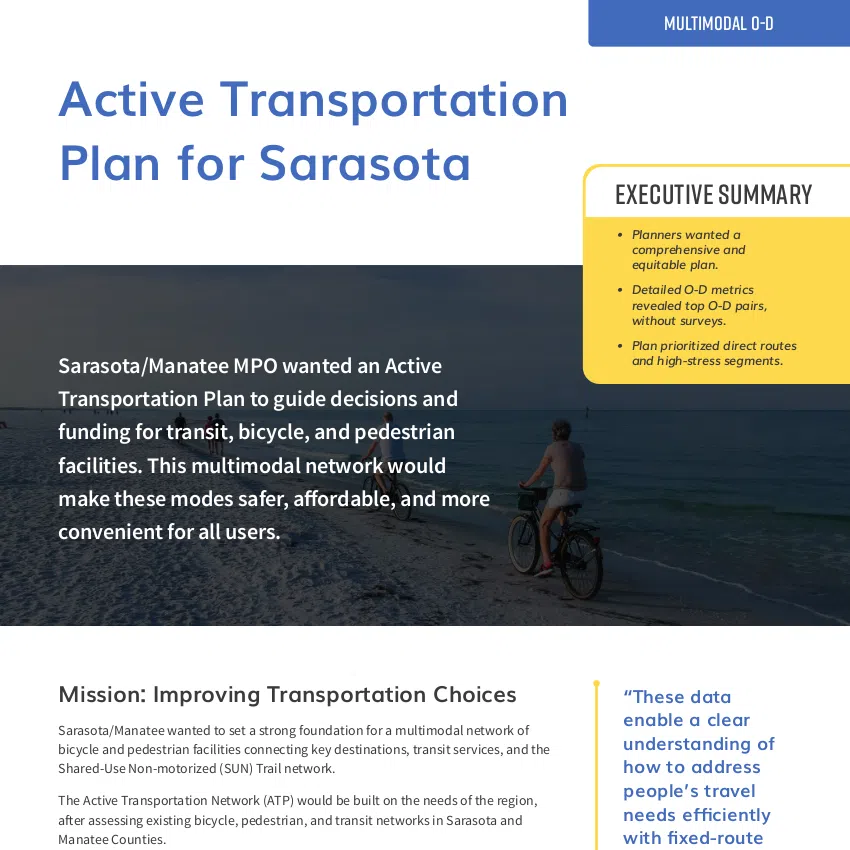
Active Transportation Plan for Sarasota
See Sarasota’s data-backed active transportation plan that guided decisions and funding for transit, bicycle, and pedestrian facilities.

Multimodal Planning in the City of Toronto
Analytics informed planning studies for “Gateway Mobility Hubs” that will provide convenient, affordable, multimodal modes and improve connections to transit options in Toronto’s outskirts.
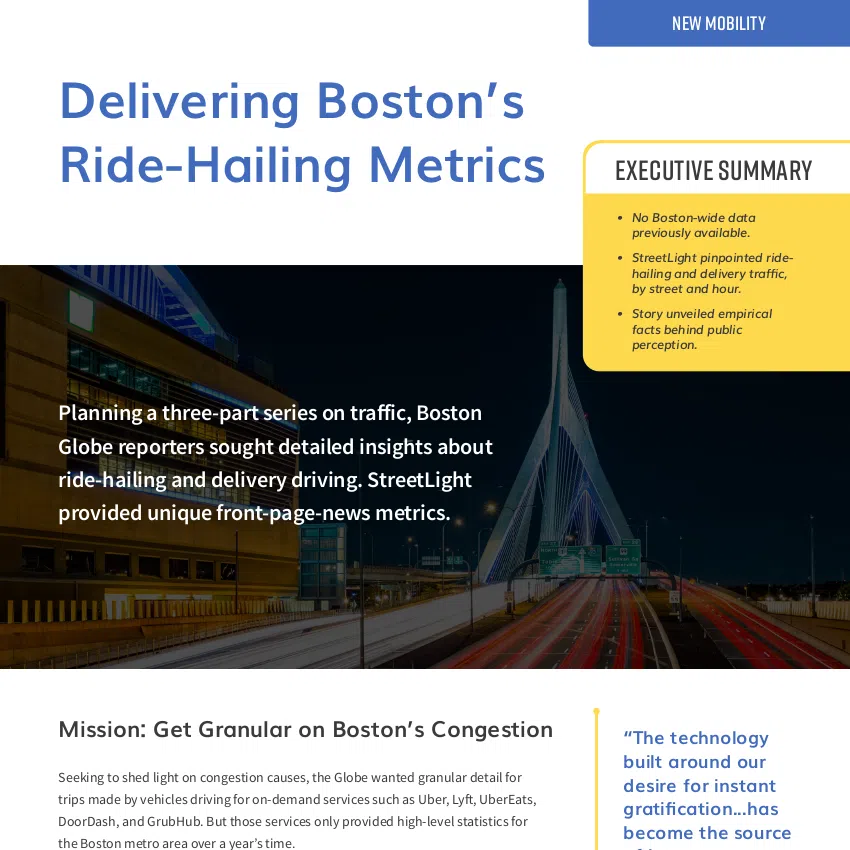
Delivering Boston’s Ride-Hailing Metrics
Learn how StreetLight’s analysis confirmed that ride-hailing vehicles contribute substantially to congestion, Download now to learn more about identifying ride-hailing and delivery travel.
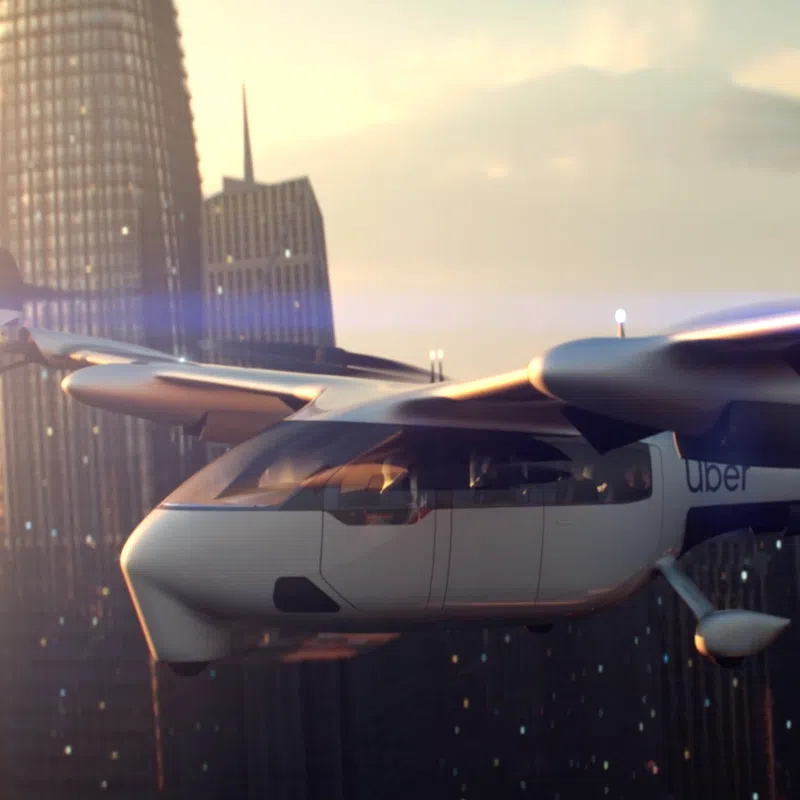
Choosing Locations for Uber Air
Download our case study to learn what metrics Uber Air used to optimize site locations, how many trips per day Uber Air predicts for demand, and how many terminals it needs to meet that demand
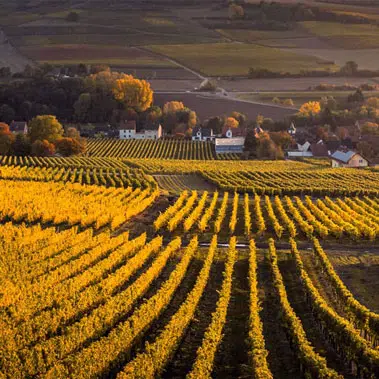
Fast Congestion Analysis in Napa
How Napa pinpointed the cause of congestion, saved time and money, and improved bus lines for commuters.

Quantifying Cut Through Traffic Measures in a California City
See how Big Data was used to measure and communicate congestion before and after cut through restrictions.
PERSONALIZED DEMO
Let's explore your mobility data needs via a live demo.

Football Hall of Fame Projects Tourism Explosion
The Pro Football Hall of Fame projected that annual visitors would jump from 300,000 to three million. To plan transportation for this tourism boost, planners turned to Big Data StreetLight for facts about where visitors came from, traffic hot spots, and parking options.
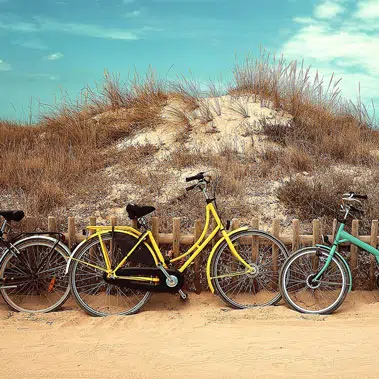
Virginia Bike Tourism: Measuring Economic Impact
Planners knew that tourists visited the Virginia Capital Trail, but they didn’t have metrics for measuring bicycle tourism’s economic impact. They turned to Big Data for detailed information about bike and pedestrian trips.

Detailed Truck Data for Virginia’s Port
To support transportation project prioritization, ongoing studies, and federal funding applications, Port of Virginia planners needed to know which routes were commonly used by port trucking. Learn how Big Data helped solve this transportation problem.

Busting Congestion with Big Data in Lafayette, CA
Helping the city prioritize policy and infrastructure to reduce congestion in downtown Lafayette.

Transportation Demand Management in Virginia
Planners analyzed hundreds of congested road segments to diagnose areas where certain techniques may have the biggest impact and to guide planning.
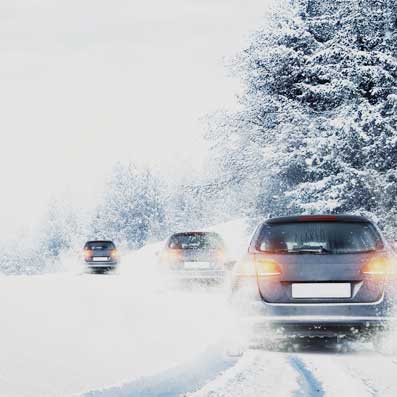
Transportation Demand Management for Snowbowl Traffic
Flagstaff’s planners needed transportation demand management data for increasing Snowbowl traffic. See how Big Data helped.
Join the Conversation
Subscribe to insights & research.
Please provide your contact information so we can send the latest insights and research from StreetLight to your inbox; you can unsubscribe at any time.
General Inquiries:
Contact streetlight, partnership inquiries:, [email protected], join the conversation, subscribe to insights & research.
- About StreetLight
- Website Privacy Notice
GETTING STARTED
- Why StreetLight
- Read Customer Stories
- Schedule a Demo
- StreetLight InSight® LOGIN
- StreetLight Training Portal
- Help Center
- Community Forum
- Customer Care
Copyright © 2011 - 2023, StreetLight Data, Inc. 4 Embarcadero Center Suite 3800 San Francisco, CA 94105. All rights reserved. Website Privacy Notice | Open Source
- How It Works
- Cost Savings
- 3rd Party Validations
- Testimonials
- AADT Traffic Counts
- Origin-Destination
- Turning Movement Counts
- Advanced Traffic Counts
- More Metrics…
- Personal Vehicles
- Bicycle & Pedestrian
- Bus & Rail
- Commercial Truck
- Delegate the work to us
- Get Data Driven Insights
- Developer Resources
- Transportation Planning
- Traffic Engineering & Operations
- Electric Vehicles (EVs)
- New Mobility Deployment
- Ride Hailing & Delivery
- AVs & UAM
- Financial Services
- Retail & Real Estate
- Climate Solutions
Congestion Management
Corridor studies, transportation modeling, roadway volumes, intersection studies.
- Grant Solutions
Safety Studies
- Social Equity
- Data Privacy
- Partnerships
- University Research
- Case Studies
- Research Reports
- White Papers
- Support & Training
Save time without relying on outdated equipment and get instant granular counts for any intersection at any time on any day.
Modes included:
Metrics included:
Total, Inbound, and Outbound Intersection Traffic Volumes, AM/PM Peak Hours, Peak Hour Factor
All plans include:
24/7 access to StreetLight InSight® & 100% coverage of Traffic Analysis Zones
Business Consulting & Services
Enhance value to clients with location intelligence and mobility insights informed by granular, validated transportation data..
Real Estate Site Selection, Portfolio Analysis, Due Diligence
Key metrics:
Vehicle, Truck, Pedestrian, Bicycle, and Transit Travel Patterns
Private Transportation
Model demand for your mobility service – airline, evtol, train, micro-mobility, and more – to plan routes and hubs that capture your target customers..
Travel Demand Modeling, Hub Site Selection, Route Planning
Origin-Destination, Travel Patterns for many modes, Demographics and Trip Purpose
Electric Vehicles, Energy & Utilities
Future-proof your electric vehicle infrastructure and investments with data-driven insights..
Electric Vehicle Infrastructure Site Selection, Grid Planning
Traffic Volumes, Trip Length, Travel Time, Dwell Time, EV Travel Patterns, Demographics
Technology, Data & Analytics
Uplevel your location intelligence solutions by partnering with streetlight to incorporate validated mobility metrics into your platform, models, or services., hospitality &tourism, analyze seasonal visitation to your region or property to forecast future tourism and manage traffic impacts..
Visitation, Cut-Through Analysis, Congestion Management
Traffic Counts, Origin-Destination, Top Routes for Travelers, Vehicle Speed
Position your projects for success with turnkey access to defensible and validated traffic counts for all roadways.
Average Annual Daily Traffic (AADT) and more
Active Transportation
Get the reliable data you need to justify your active transportation decisions and build infrastructure that reflects the reality of today..
Volume/Traffic Counts, Origin-Destination, Select Link, Speed, Demographics, Travel Time, and more
Develop, calibrate, and validate your travel demand models’ granular analytics to ensure that your models properly forecast travel patterns.
Volume/Traffic Counts, Origin-Destination, Select Link, Demographics, Trip Purpose, and more
Truck Studies
Measure up-to-date truck activity across all road types to identify congestion bottlenecks and evaluate the economic impact..
Volume, Travel Time, Trip Length, Origin-Destination, Routing, Vehicle Classification, and more
Diagnose traffic patterns for all hours of the day to develop data-informed strategies that mitigate traffic congestion.
Volume, Vehicle Miles Traveled (VMT), Vehicle Hours of Delay (VHD), Planning Index, Buffer Time Index, Travel Time, Speed, and more
Traffic Calming & Management
Easily fill data gaps, address residents’ top concerns, and justify infrastructure initiatives with insights into local and residential roads..
Volume, VMT/VKT, Speed, VHD, Congestion, Travel Time, Trip Length, Speed and Travel Time Percentiles, Demographics, and more
Visitation Studies
Effectively manage operations by accessing on-demand visitation metrics that show when, how, and from where people travel to key sites..
Volume, Trip Origins, Travel Time, Trip Length, Trip Speed, Traveler Demographics, Home and Work locations
Passenger Rail Studies
Evaluate network ridership for any time of day or day of week to help prioritize projects and advocate for bus and rail improvements..
Ridership, Travel Time, Origin-Destination Pairs, Rider Demographics
Retail & Restaurant
Choose the best locations for your business with mobility analytics that pinpoint customer demand to drive sales.
Key use cases:
Site Selection, Trade Area Analysis, Electric Vehicle Charger Site Selection
Traffic Counts, Trip Purpose, Demographics, Top Routes
Automotive Aftermarket & Fueling
Understand demand for your automotive product or service with validated nationwide vehicle travel patterns..
Supply Chain and Inventory Management, Market Research & Demand Forecasting
VMT / VKT, Personal and Commercial Vehicle Roadway Volumes, Fleet Characteristics
Leverage powerful multimodal insights to zero in on opportunities to keep your community safe and equitable.
Volume, VMT/VKT, Speed, VHD, Congestion, Travel Time, Demographics, Trip Purpose, Speed and Travel Time Percentiles, and more
Run multiple analyses for the same corridor in a few clicks to ensure that you are solving problems with a macro- and micro-level lens.
Volume, VMT/VKT, Speed, VHD, Congestion, Travel Time, and more
Analyze traffic patterns on popular routes near toll roads to evaluate the impact of toll changes on congestion, accessibility, and safety.
Origin-Destination, Top Routes between locations, Trip Speed, Travel Time, Trip Length, Traveler Demographics
Home › Case Studies › Unlocking Insights: Transportation Data Analytics for Smarter Mobility
Unlocking Insights: Transportation Data Analytics for Smarter Mobility
Written By: Sudeshna Ghosh
In today’s fast-paced global economy, transportation data analytics plays a pivotal role in driving operational efficiency, optimizing logistics, and enhancing customer satisfaction. By harnessing advanced analytics techniques, businesses can gain invaluable insights into transportation trends, route optimization, fleet management, and supply chain performance.
Quantzig’s Transportation Data Analytics Dashboard solution offers a comprehensive suite of tools and capabilities to help clients leverage their transportation data effectively. With real-time data visualization, predictive analytics, and performance monitoring features, our solution empowers businesses to make informed decisions, streamline operations, and mitigate risks in the transportation ecosystem. From route planning and vehicle tracking to demand forecasting and cost optimization, our transportation data analytics dashboard enables companies to unlock actionable insights, enhance decision-making processes, and drive sustainable growth in a competitive market environment.
Request a demo to experience the meaningful insights we derive from data through our analytical tools and platform capabilities. Schedule a demo today!
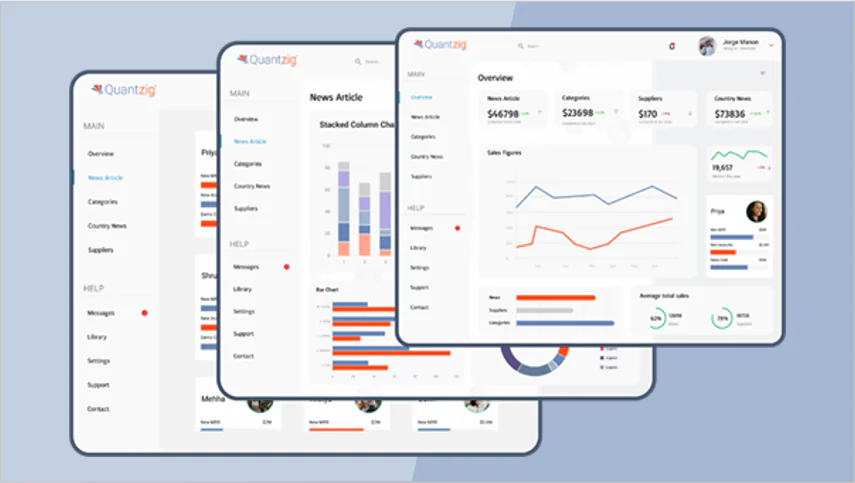
What You’ll Find in this Case Study:
- Engagement Overview
- Transportation Analytics Business Challenge
Transportation Analytics Solution Offered and Value Delivered
- Benefits of Transportation Analytics
Quantzig’s Success Story:
Client details.
Headquartered in Denmark, the client is a leading consumer packaged goods CPG manufacturer whose private-label products are distributed to several retail outlets across the globe. To sustain a competitive edge, the client wanted to deploy the right logistics management systems and efficient processes to transport their products to the end-users. Though they had an extensive delivery team spread across geographies to connect their warehouses and partner outlets, they faced major roadblocks in transporting their goods promptly.
Challenges Faced by the Client
Data obtained from every source can help you unearth actionable insights if analyzed accurately, and transportation data is no exception. Having said that, it’s crucial to note that transportation data analytics has the potential to improve logistics management, as well as enable businesses to optimize transit routes and services. Leveraging transportation analytics to develop route maps can help businesses optimize the logistic workflow, which in turn, will result in a drastic reduction in congestion levels and time spent in transit.
The manufacturer’s delivery team was complex and comprised of hundreds of employees and partners. The use of outmoded logistics tracking systems made it difficult to track, update, monitor, and deliver products in a timely manner. Also, the outdated legacy systems were difficult to maintain and proved to be of no use in logistics management. Such factors along with the ongoing loss made it crucial for the client to replace the legacy system with a more efficient logistics workflow.
The CPG manufacturer approached Quantzig to leverage its expertise in transportation data analytics and drive significant improvements in the logistics workflow. Our transportation analytics solutions helped the client to gain in-depth insights into their logistics routes through an advanced backend dashboard that provided real-time insights based on transportation data.
Through our transportation analytics solutions we helped the client to revamp their current logistics workflow and achieve the desired level of efficiency. In addition, it’s essential to note that transportation analytics improved their logistics and route planning capability and helped them achieve huge savings on maintenance and renewal.
Experience the advantages firsthand by testing a customized complimentary pilot designed to address your specific requirements. Pilot studies are non-committal in nature.
Introduction to Transportation Data Analytics
Transportation data analytics involves the collection, analysis, and interpretation of data related to transportation systems. It helps identify patterns and trends in transportation behavior, such as traffic congestion or public transport usage. Data analytics can be used to optimize transportation routes and schedules for efficiency and sustainability. It plays a crucial role in improving safety by identifying accidents-prone areas and suggesting targeted interventions. Transportation data analytics can also aid in predicting demand, optimizing pricing strategies , and enhancing overall user experience in transportation services.
Importance and Benefits of Transportation Data Analytics
Transportation data analytics offers numerous benefits to the transportation industry, leveraging data analytics, Big Data , AI, and alternative data sources to optimize operations, enhance decision-making, and capitalize on opportunities. Some of the key benefits include:
1. Capacity Constraints Management:
Through transportation data analytics, organizations can gain insights into capacity constraints within their networks, enabling them to optimize routes and resources efficiently. By analyzing historical data and real-time information, companies can proactively address bottlenecks, minimize delays, and ensure timely delivery of goods. This optimization not only enhances operational efficiency but also improves customer satisfaction by meeting demand effectively, ultimately driving competitive advantage in the market.
2. Mergers and Acquisitions Support:
Transportation data analytics plays a crucial role in supporting organizations undergoing mergers and acquisitions by facilitating the seamless integration of transportation operations. By harmonizing data from disparate systems and platforms, companies can streamline processes, reduce redundancies, and achieve synergies more effectively. This analysis ensures continuity of operations, minimizes disruptions, and accelerates the integration process, ultimately maximizing the value derived from mergers and acquisitions.
3. Freight Spending Optimization:
Leveraging transportation data analytics, organizations can optimize freight spending by identifying cost-saving opportunities and negotiating favorable rates with carriers. By analyzing freight spending data, companies can identify inefficiencies, consolidate shipments, and optimize transportation modes to reduce overall spending. This optimization not only improves cost-effectiveness but also enhances profitability, enabling organizations to allocate resources more efficiently and invest in strategic initiatives for growth and innovation.
4. Leadership Decision Support:
Transportation data analytics empowers organizational leaders to make informed decisions backed by data insights. By leveraging optimization techniques and predictive analytics , leaders can align transportation strategies with overall business objectives, driving strategic alignment and operational excellence. This data-driven approach enables proactive decision-making, fosters agility in response to market changes, and positions organizations for long-term success in a competitive landscape.
5. Utilization of Big Data:
Harnessing the power of big data, transportation data analytics uncovers patterns and trends that were previously inaccessible. By analyzing vast datasets from various sources, including GPS data, sensor data, and geospatial data, organizations gain actionable insights for proactive decision-making and strategy formulation. This utilization of big data enables companies to anticipate market trends, identify emerging opportunities, and mitigate risks, ultimately driving competitive advantage and sustained growth in the transportation industry.
6. Improving Road Safety with Data Analytics
It entails leveraging advanced technologies and vast datasets to identify and mitigate risks on roadways. By analyzing historical accident data, traffic patterns, and driver behavior, organizations can identify high-risk areas, implement targeted interventions, and improve overall road safety. Additionally, real-time monitoring and predictive analytics enable proactive measures such as adaptive traffic signal control and automated collision avoidance systems, further enhancing safety for drivers, pedestrians, and cyclists alike.
7. Enhancing Efficiency in Public Transportation Systems through Data Analytics
It involves optimizing routes, schedules, and resource allocation to improve service quality and reliability. By analyzing passenger demand, travel patterns, and operational performance metrics, transportation authorities can identify opportunities for route optimization, frequency adjustments, and capacity planning. Moreover, predictive maintenance and real-time monitoring enable proactive management of fleet assets, reducing downtime and ensuring seamless operations. Through data-driven insights and continuous optimization efforts, public transportation systems can provide more efficient, reliable, and sustainable mobility solutions for urban populations.
Types of Transportation Data Collected
In the dynamic field of transportation logistics, a wide range of data types are collected to facilitate informed decision-making, optimize operational processes, and enhance overall supply chain efficiency. These data types span from traditional metrics such as transport volumes, transit times, and associated costs to more sophisticated datasets, including real-time GPS tracking, sensor data from vehicles, and customer feedback. Moreover, geospatial data plays a pivotal role in understanding route optimization, traffic patterns, and location-based insights.
Additionally, integration with transportation management systems (TMS), freight invoices, and customer databases offers a holistic view of the transportation landscape. By harnessing this diverse range of data types, organizations can derive actionable insights, drive strategic initiatives, and maintain competitiveness in the ever-evolving transportation industry landscape.
Data Collection and Data Analysis Techniques
Data collection methods and technologies in transportation analytics encompass a diverse range of tools and techniques aimed at gathering comprehensive datasets for analysis. Traditional methods include manual data collection through surveys, interviews, and observations, while modern technologies such as GPS trackers, sensors, and IoT devices offer real-time data capture capabilities. Additionally, transportation management systems (TMS), traffic cameras, and satellite imagery technology provide valuable insights into traffic flow, vehicle movements, and infrastructure utilization. Furthermore, emerging technologies like drones and mobile apps enable crowdsourced data collection, enhancing the breadth and depth of transportation datasets. By employing a combination of these methods and technologies, organizations can capture accurate, timely, and granular data to inform decision-making and drive operational improvements in transportation systems.
On the other hand, Data analysis techniques and tools play a crucial role in extracting actionable insights from transportation datasets, enabling organizations to optimize routes, enhance operational efficiency, and improve overall performance. Statistical analysis methods such as regression analysis, clustering, and trend analysis allow for the identification of patterns, correlations, and anomalies within transportation data. Moreover, machine learning algorithms and predictive analytics models enable organizations to forecast demand, optimize resource allocation, and mitigate risks proactively. Additionally, data visualization tools such as dashboards, heat maps, and geographic information systems (GIS) facilitate the interpretation and communication of complex transportation data to stakeholders. By leveraging these analysis techniques and tools, organizations can unlock valuable insights, drive informed decision-making, and achieve strategic objectives in transportation management.
What is the impact of transport analysis?
Transport analytics has a profound impact across various domains, leveraging location intelligence, machine learning, and predictive analytics to optimize transport flow, enhance supply chain efficiency, improve road safety, and enable more efficient strategies. Here’s a breakdown of its impact across different areas:
1. Transport Flow Analysis:
This tool empowers organizations to analyze and optimize vehicle and foot traffic patterns, leading to improved route planning and reduced congestion. By leveraging location intelligence and predictive analytics, companies can enhance transport flow efficiency, minimize travel times, and ultimately improve overall operational performance. This optimization not only enhances customer satisfaction but also enables organizations to meet increasing demands and stay competitive in dynamic market environments.
2. Supply Chain Analysis:
Through transportation analytics, organizations gain valuable insights into supply chain dynamics, facilitating more efficient inventory management and logistics operations. By leveraging machine learning and predictive analytics, companies can optimize routes, streamline distribution processes, and enhance competitive intelligence. This enables organizations to improve supply chain resilience, minimize costs, and gain a strategic advantage in the marketplace.
3. Road Safety Management:
This tool plays a crucial role in road safety management by leveraging predictive analytics to identify high-risk areas and implement targeted interventions. By analyzing data from various sources such as weather reports and satellite imagery, authorities can proactively address safety concerns, reduce accidents’ rates, and improve overall road safety. This not only saves lives but also enhances public trust and confidence in transportation systems.
4. Automatic Scheduling and Planning:
Integration of machine learning algorithms into transportation analytics systems enables automatic scheduling and planning, optimizing transport routes and resource allocation. By leveraging advanced algorithms, companies can improve operational efficiency, minimize delays, and enhance service reliability. This automation streamlines processes, reduces manual effort, and enables organizations to adapt quickly to changing market conditions, ultimately driving cost savings and improving customer satisfaction.
5. Traffic Congestion Management:
This tool aids in traffic congestion management by utilizing predictive analytics and real-time data analysis to identify congestion hotspots and suggest alternative routes. By implementing measures such as high occupancy toll lanes, organizations can alleviate congestion, improve traffic flow, and enhance overall transportation efficiency. This not only reduces travel times and fuel consumption but also enhances air quality and mitigates environmental impact, contributing to sustainable urban development.
In summary, this tool has a significant impact on various aspects of transportation and logistics, ranging from optimizing transport flow and enhancing supply chain efficiency to improving road safety and managing traffic congestion. By leveraging advanced analytics techniques and integrating data from multiple sources, transportation analytics enables organizations to make data-driven decisions, improve operational efficiency, and enhance overall transportation systems.
Applications of Transportation Data Analytics in Urban Planning
In the domain of urban planning, transportation data analytics serves as a linchpin for informed decision-making, sustainable development, and enhanced quality of life for residents. By harnessing advanced analytics techniques and leveraging vast datasets, urban planners can gain invaluable insights into traffic patterns, commuter behavior, and infrastructure utilization. This enables them to optimize transportation networks, improve traffic flow, and alleviate congestion hotspots, ultimately leading to more efficient mobility solutions and reduced carbon emissions.
Additionally, transportation data analytics facilitates evidence-based policy formulation, enabling cities to prioritize investments in public transportation, cycling infrastructure, and pedestrian-friendly urban spaces. By embracing data-driven approaches, urban planners can create smarter, more resilient cities that cater to the evolving needs of residents while fostering economic growth and environmental sustainability.
What is the role of predictive analytics in cpg?
Predictive analytics plays a crucial role in the Consumer-Packaged Goods (CPG) industry by forecasting consumer demand, optimizing inventory levels, and enhancing supply chain efficiency. By analyzing historical sales data, market trends, and external factors, predictive analytics helps CPG companies anticipate future demand patterns, leading to more accurate production planning and inventory management. This ensures that companies can meet consumer demand effectively while minimizing excess inventory and associated costs, ultimately improving profitability and customer satisfaction.
Implementation of multimodal transport segment-wise analysis
In today’s complex transportation landscape, implementing multimodal transport segment-wise analysis is imperative for optimizing operational efficiency, enhancing customer satisfaction, and driving competitive advantage. By breaking down the multimodal transport system into distinct segments and analyzing each segment’s performance, organizations can uncover valuable insights to inform strategic decision-making and operational improvements.
1. Data Collection:
Gathering relevant data is the first step in segment-wise analysis of multimodal transport systems. This includes collecting information on transport volumes, costs, transit times, service levels, and customer preferences across different segments. By capturing comprehensive data sets, organizations can gain a holistic understanding of each segment’s dynamics and identify areas for improvement.
2. Data Integration:
Integrating data from various sources and modes of transport is essential for creating a unified dataset for analysis. This involves consolidating data from transportation management systems, GPS trackers, freight invoices, customer databases, and other relevant sources. By centralizing data, organizations can eliminate silos, enhance data quality, and enable more accurate analysis of segment-wise performance.
3. Segmentation Analysis:
Applying segmentation techniques to analyze the data allows organizations to identify patterns, trends, and differences across different segments. Using clustering analysis, regression analysis, or other statistical methods, companies can effectively segment the data and uncover insights into each segment’s performance drivers. This analysis provides a foundation for informed decision-making and targeted improvements.
4. Identify Opportunities and Challenges:
Utilizing insights gained from segment-wise analysis, organizations can identify opportunities for improvement and address challenges specific to each segment. Whether it’s optimizing routes, adjusting pricing strategies, or improving service levels, understanding segment dynamics enables organizations to tailor their strategies and initiatives for maximum impact. This proactive approach ensures that resources are allocated effectively, and operational efficiency is maximized across the entire transport network.
5. Decision Making:
Using the analysis results, organizations can make informed decisions regarding resource allocation, capacity planning, route optimization, pricing strategies, and overall operational improvements. By leveraging data-driven insights, companies can prioritize initiatives that offer the greatest potential for impact and ensure alignment with strategic objectives. This approach empowers organizations to drive continuous improvement and maintain a competitive edge in the dynamic transportation industry landscape.
Get started with your complimentary trial today and delve into our platform without any obligations. Explore our wide range of customized, consumption driven analytical solutions services built across the analytical maturity levels.
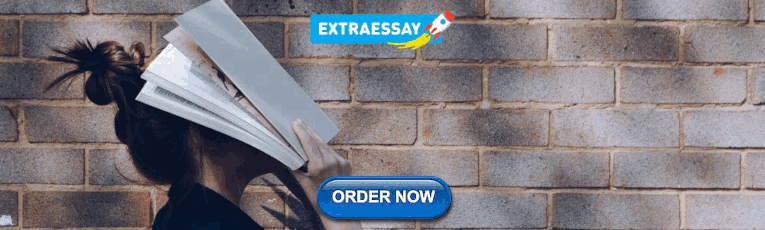
Future Trends and Challenges in Transportation Data Analytics
As the transportation industry continues to evolve, future trends and challenges in transportation data analytics are poised to reshape the landscape. Emerging technologies such as artificial intelligence, machine learning, and the Internet of Things (IoT) are expected to drive innovation, enabling more sophisticated data collection, analysis, and decision-making processes.
Predictive analytics and real-time data processing will become increasingly prevalent, empowering organizations to anticipate traffic patterns, optimize routes, and enhance operational efficiency. However, with these advancements come challenges such as data privacy concerns, cybersecurity risks, and the need for skilled talent to manage and interpret complex datasets.
Additionally, interoperability issues and data standardization across different modes of transportation pose obstacles to seamless integration and collaboration. Despite these challenges, the potential benefits of transportation data analytics are vast, offering opportunities for organizations to improve safety, reduce congestion, and create more sustainable transportation systems for the future.
Conclusion:
In conclusion, transportation data analysis is not just a tool but a catalyst for transformative change in the transportation industry. By harnessing the power of data analytics, organizations can optimize routes, enhance operational efficiency, and improve overall transportation systems’ safety and sustainability. As technology continues to evolve and data becomes increasingly abundant, the potential for innovation and improvement in transportation data analysis is boundless. Embracing data-driven decision-making will be key to navigating the challenges and seizing the opportunities that lie ahead, ensuring a smarter, more efficient, and more resilient transportation landscape for the future.
Frequently Asked Questions
1. how is data analytics used in transportation.
Data analytics is used in transportation to analyze various aspects of the system including route optimization, demand forecasting, vehicle maintenance scheduling, traffic management, and supply chain optimization. By analyzing large volumes of data from sources such as GPS, sensors, traffic cameras, and historical transportation records, data analytics enables transportation companies and authorities to make data-driven decisions, improve operational efficiency, reduce costs, and enhance overall service quality.
2. Why is transport analysis important?
Transport analysis is crucial for several reasons: – It helps identify inefficiencies and bottlenecks in transportation systems, enabling optimization and improvement. – It aids in forecasting future transportation needs and demands, allowing for better planning and resource allocation. – It assists in identifying safety hazards and risks, leading to improved road safety measures. – It enables transportation companies to optimize routes, reduce fuel consumption, and minimize environmental impact. – It supports policy-making and infrastructure development by providing insights into transportation trends and patterns.
3. What is the transportation problem in analytics?
The transportation problem in analytics refers to the classic optimization problems in which goods must be transported from a set of sources to a set of destinations while minimizing total transportation costs. These problems are characterized by constraints such as capacities at sources and demands at destinations. The goal is to determine the optimal allocation of goods to transportation routes to meet demand while minimizing costs.
4. What are the methods of data collection in transportation?
There are several methods of data collection in transportation, including: – GPS tracking: Utilizing GPS devices installed in vehicles to track their locations and movements in real-time. – Traffic sensors: Installing sensors along roadways to collect data on vehicle speeds, volumes, and congestion levels. – Traffic cameras: Using cameras to monitor traffic flow, detect incidents, and gather visual data for analysis. – Surveys: Conducting surveys of travelers to gather information on travel behavior, preferences, and patterns. – Electronic toll collection systems: Collecting data on vehicle movements and usage through toll collection systems. – Transport management systems: Utilizing software systems to collect and analyze data on transportation operations, including fleet management, scheduling, and routing.
5. How can transportation data analytics improve efficiency?
Transportation data analytics improves efficiency by providing insights into traffic patterns, route optimization, and resource allocation. By analyzing large datasets, organizations can identify bottlenecks, streamline operations, and reduce transit times, ultimately enhancing overall efficiency and cost-effectiveness.
6. What is the role of artificial intelligence in transportation data analytics?
Artificial intelligence plays a crucial role in transportation data analytics by enabling predictive modeling, real-time decision-making, and automation of tasks. AI algorithms can analyze complex data sets, identify patterns, and predict future trends, allowing organizations to proactively address issues and optimize transportation systems for maximum efficiency.
Recent Posts
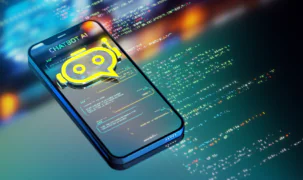
How Quantzig’s AI chatbot solutions enable higher transparency with a CPG major’s suppliers
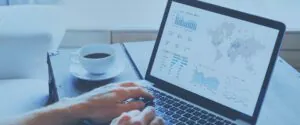
Optimizing Manufacturing Efficiency: Unleashing the Power of ERP Capacity Planning
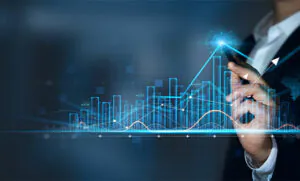
AI Capacity Planning: Optimizing Performance and Scalability
Privacy overview.
Urban Mobility: Leveraging AI, Machine Learning, and Data Analytics for Smart Transportation Planning- A case study on New York City
Ieee account.
- Change Username/Password
- Update Address
Purchase Details
- Payment Options
- Order History
- View Purchased Documents
Profile Information
- Communications Preferences
- Profession and Education
- Technical Interests
- US & Canada: +1 800 678 4333
- Worldwide: +1 732 981 0060
- Contact & Support
- About IEEE Xplore
- Accessibility
- Terms of Use
- Nondiscrimination Policy
- Privacy & Opting Out of Cookies
A not-for-profit organization, IEEE is the world's largest technical professional organization dedicated to advancing technology for the benefit of humanity. © Copyright 2024 IEEE - All rights reserved. Use of this web site signifies your agreement to the terms and conditions.
- City by city
- Current issues
- General election 2024
- Metro mayors
- Levelling up
- Productivity

Transport Case Studies
How different cities are improving their transport connections using our case study library
Case study library
Why improve transport?
Effective transport is vital for the economy. Good transport connections have direct benefits to people, businesses, the environment, and the economy overall. They can support innovation, help people access jobs, shape greener and healthier places, and attract new firms.
Having more control over transport can help cities make the most of their infrastructure by reducing uncertainty and short timescale of funding, improving the bus system, and ensuring integration of transport, economic development and infrastructure.
Use the table below to find out more about how and what cities in the UK and abroad have done to improve transport. These case studies highlight what the cities did and their self-reported outcomes.
To find out more about what types of transport what types of policy interventions have an impact on local economic growth from across OECD countries, as well as evidence-based policy design guides, take a look at the What Works Centre pages on Transport.
Case studies
click the case study title to find out more
More Case Studies on Housing and Transport
Delivering change: making transport work for cities.
Zach Wilcox and Nada Nohrová
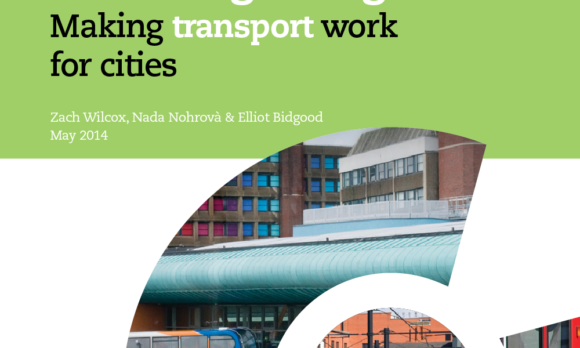
Delivering change: Putting city centres at the heart of the local economy
Louise McGough and Elli Thomas

Open Data or Closed Doors?
Maire Williams

Funding and financing inclusive growth in cities
Naomi Clayton, Simon Jeffrey and Anthony Breach

How can UK cities clean up the air we breathe?
Adeline Bailly

Policy Officer
James Evans
Series: City Talks
Paul Swinney
Austin Kilroy
Combined authorities
- Contributors
- Links
- Translations
- What is Gendered Innovations ?
Sex & Gender Analysis
- Research Priorities
- Rethinking Concepts
- Research Questions
- Analyzing Sex
- Analyzing Gender
- Sex and Gender Interact
- Intersectional Approaches
- Engineering Innovation
- Participatory Research
- Reference Models
- Language & Visualizations
- Tissues & Cells
- Lab Animal Research
- Sex in Biomedicine
- Gender in Health & Biomedicine
- Evolutionary Biology
- Machine Learning
- Social Robotics
- Hermaphroditic Species
- Impact Assessment
- Norm-Critical Innovation
- Intersectionality
- Race and Ethnicity
- Age and Sex in Drug Development
- Engineering
- Health & Medicine
- SABV in Biomedicine
- Tissues & Cells
- Urban Planning & Design
Case Studies
- Animal Research
- Animal Research 2
- Computer Science Curriculum
- Genetics of Sex Determination
- Chronic Pain
- Colorectal Cancer
- De-Gendering the Knee
- Dietary Assessment Method
- Heart Disease in Diverse Populations
- Medical Technology
- Nanomedicine
- Nanotechnology-Based Screening for HPV
- Nutrigenomics
- Osteoporosis Research in Men
- Prescription Drugs
- Systems Biology
- Assistive Technologies for the Elderly
- Domestic Robots
- Extended Virtual Reality
- Facial Recognition
- Gendering Social Robots
- Haptic Technology
- HIV Microbicides
- Inclusive Crash Test Dummies
- Human Thorax Model
- Machine Translation
- Making Machines Talk
- Video Games
- Virtual Assistants and Chatbots
- Agriculture
- Climate Change
- Environmental Chemicals
- Housing and Neighborhood Design
- Marine Science
- Menstrual Cups
- Population and Climate Change
- Quality Urban Spaces
- Smart Energy Solutions
- Smart Mobility
- Sustainable Fashion
- Waste Management
- Water Infrastructure
- Intersectional Design
- Major Granting Agencies
- Peer-Reviewed Journals
- Universities
Public Transportation: Rethinking Concepts and Theories
- Full Case Study
The Challenge
Categories used in transportation surveys—and, hence, the way statistics are gathered and analyzed—may not properly account for caring work—that is unpaid labor performed by adults for children or other dependents, including labor related to the upkeep of a household. Public transport systems are typically designed around the needs of commuters (people traveling between their homes and places of paid employment). The mobility associated with caring work, including childcare and elder care, has typically not figured into transportation design.
Method: Rethinking Concepts and Theories
The innovative concept "mobility of care" provides a perspective for "recognizing and revaluing care work" (Sánchez de Madariaga, 2009). Incorporating "caring work" into user surveys helps to identify the significant number of trips that women and men make for this purpose. Understanding gender differences in public transportation is important for understanding climate change and planning efficient housing and neighborhoods .
Gendered Innovations:
- 1. Adding the concept, "mobility of care", to data collection variables may render public transportation more responsive to users' needs. 2. Understanding gender differences in travel has led to the concept of "trip chaining" with ramifications for the design of public transport systems. 3. Gathering data disaggregated by sex and other factors intersecting with sex and gender (such as income, family status, etc.) improves transportation research and policy.
- ●To/From Work ●Related Business ●Shopping ●Other Family/Personal Errands ●School/Church ●Social and Recreational ●Other
Categories used in transportation surveys—and, hence, the way statistics are gathered and analyzed—may not properly account for caring work, i.e., unpaid labor performed by adults for children or other dependents, including labor related to the upkeep of a household.
Gendered Innovation 1: Visualizing the Mobility of Care
Data for Spain are similar to data averaged across the EU; in the 15 countries surveyed, women performed an average of 32 minutes of childcare per day, and men performed an average of 12 minutes. In all EU countries surveyed, women spent significantly more time performing childcare than men—from 1.7 times more in Sweden to 4.8 times more in Latvia. This pattern holds in the U.S. as well—in 2010, the average U.S. woman spent 32 minutes caring for and helping children in her household, twice as much as the average U.S. man's 16 minutes (United States Bureau of Labor Statistics, 2011). It is important to recognize that men's caring work has increased over time. Care-giving among British fathers, for example, increased nine-fold from 1961 to 1999 (O'Brien et al., 2003).
That women spend significantly more time than men performing caring work implies that consideration of caring work is a key to gender equality in transportation; many forms of caring work rely on public transportation.
Method: Rethinking Language and Visual Representations The innovative concept "mobility of care" reveals significant travel patterns otherwise concealed in data collection variables (Sánchez de Madariaga, 2013; Sánchez de Madariaga, 2009). The charts below represent public transportation trips made in Spain in 2007. The first chart (left) graphs transportation data as traditionally collected and reported. It privileges paid employment by presenting it as a single, large category. Caring work (shown in red) is divided into numerous small categories and hidden under other headings, such as escorting, shopping and leisure. The second chart (right) reconceptualizes public transportation trips by collecting care trips into one category. Visualizing care trips in one dedicated category recognizes the importance of caring work and allows transportation engineers to design systems that work well for all segments of the population, improve urban efficiency, and guard against global warming (Sánchez de Madariaga, 2013). A number of innovations in transportation design have been implemented to support the mobility of care. In London, for example, these include: ● Step-free access to trains, subways, and buses. Transportation authorities are removing steps from streets to platforms to accommodate baby carriages, luggage, wheelchairs, and similar devices. By 2007, the London Underground had developed 47 step-free stations. ● Wide aisle gate access to transportation. ● Level access from platforms to trains (Transport for London, 2007). View General Method
Gendered Innovation 2: Conceptualizing and Studying Trip Chaining
A simplistic definition of a "trip" describes each trip as a journey from a single starting location to a single destination, typically using a single form of transportation. The concepts of "trip chaining" and "multipurpose trips" expand on this definition by recognizing that trips often involve a sequence of destinations and are multimodal (McGuckin et al., 2005b; Hanson, 1980). Research on trip chaining has examined the directions of trips, timing of travel, and purpose of the stops with attention to gender and other factors intersecting with sex and gender—see Methods below.
Method: Rethinking Concepts and Theories Analyzing gender in transportation requires challenging underlying concepts and identifying gaps in the way data are collected. It also requires introducing new concepts and theories that model the complex routes taken by real people. A study of travel in the United States between 1995 and 2001 using the concept of trip chaining has produced the following insights regarding women's and men's travel patterns: ● A greater number of women than men make multiple-stop trips when traveling between their homes and workplaces. This difference between women and men is decreasing, however, mainly as a result of an increase in trip chaining among men (between 1995 and 2001, the number of stops men made while returning home from work increased by 24%). ● Women make more short stops on the way to or from work than do men to perform household-sustaining activities, such as shopping and family errands, and working women in two-worker families were twice as likely as men in two-worker families to pick up and drop off school-age children at school during their commute. ● Other demographic variables interact with gender in predicting trip chaining. For example, having a child under age 5 increases trip chaining by 54% for working women and 19% for working men—see chart (McGukin et al., 2005a). View General Method
Gendered Innovation 3: Gathering Sex-Disaggregated Data Improves Transportation Research and Policy
Method: Intersectional Approaches Disaggregating data by sex is a first step. To serve a wide user base, other variables need to be considered and analyzed for how they interact with sex and gender (Audirac, 2008). These include: ● Age: Designing public transport systems to consider the mobility needs of older adults supports safe mobility for older people who have ceased driving for various health reasons (Currie et al., 2010). Gender also interacts with age in the context of driving cessation: researchers found that "older females were more likely than males to have planned ahead [for cessation], made the decision themselves, and stopped at appropriate times" (Oxley et al., 2011). The correlation between age and geographic location is a serious challenge in supporting the mobility of older people; more older people live in rural areas than do younger people (O'Neill, 2010). ● Family status: The presence of children—particularly young children—increases the number of caring trips and the need for routes to accommodate these needs (Crane, 2007). ● Ethnicity: In the United States, use of public transportation differs by both sex and self-reported ethnicity. In all ethnic groups, women make a larger proportion of their trips via public transportation than do men. These sex differences, however, vary by ethnicity (see chart below produced with data from Doyle et al., 2000). ● Geographic location: Locations differ in safety. New design features have made transportation safer. These include designated waiting areas, transparent bus shelters, emergency intercoms and surveillance mechanisms, and alternative services and routes, such as request-stop programs that allow nighttime users to disembark from the bus at locations closer to their final destination at night (Schulz et al., 1996). Researchers working on the Framework Programme 7 (FP7) G rowing O lder, St a ying Mobi l e (GOAL) project have created profiles of older people by analyzing gender alongside other factors, including demographic variables (age, financial resources, employment, etc.) and patterns of transit usage (Hoedemaeker et al., 2012). View General Method
Conclusions
Analyzing gender in public transportation requires:
- 1. Rethinking categories used in public transportation surveys. The innovative concept "mobility of care", captures significant travel patterns, and it can be used to render public transportation more equitable and responsive to users' needs. 2. Rethinking basic concepts, such as what constitutes a "trip"—whether to a single destination or a series of "chained"destinations. 3. Gathering data disaggregated by sex and other variables (such as income, self-reported ethnicity, family status, etc.) that may correlate with transit use.
The European Commission and other governmental organizations have identified how sex and gender analysis can enhance public transportation through both basic research and policymaking:
Basic Research:
- 1. Analyzing biological sex differences (in women's and men's height, weight, etc.) in designing steps and railings, positioning buttons, and other use factors. This analysis includes rethinking standards and reference models as necessary to recognize pregnancy as a normal physiological state (European Commission, 2007). 2. Collecting sex-disaggregated transportation data and including intersectional approaches (such as "socioeconomic and demographic indicators") in data analysis (European Commission, 2007).
Transport Policy:
- 1. Working to eliminate disparities between women's and men's representation on transit planning boards through efforts to increase the number of women experts in the transit work programme (European Commission, 2007). 2. Conducting regular and systematic "gender audits" to evaluate transport systems from a gender perspective (Hamilton et al., 2000). 3. Harmonizing transportation and time use statistics within the European Union in order to account for the mobility of care (European Parliament's Committee on Transport and Tourism, 2006).
Works Cited
Audirac, I. (2008). Universal Design and Accessible Transit Systems: Facts to Consider when Updating or Expanding your Transit System . Washington, D.C.: Project Action.
Crane, R. (2007). Is There a Quiet Revolution in Women's Travel? Revisiting the Gender Gap in Commuting. Journal of The American Planning Association, 73 (3) , 298-316.
Currie, G., & Delbose, A. (2010). Exploring Public Transport Usage Trends in an Ageing Population . Transportation, 37 (1), 151-164.
Doyle, D., & Taylor, B. (2000). Variation in Metropolitan Travel Behavior by Sex and Ethnicity. In United States Federal Highway Administration (Ed.), Travel Patterns of People of Color: Final Report , pp. 181-244. Washington, DC: United States Department of Transportation.
European Commission. (2007). Women in Transport: First Progresses in FP7—Sustainable Surface Transport . Brussels: European Commission .
European Parliament's Committee on Transport and Tourism. (2006) . Women and Transport Study, Provisional Version. Brussels: European Parliament.
Gather, M., Martin, J., Aragall., F., & Aslaksen, F. (2011). European Commission Project: Transport Needs for an Ageing Society.
Hamilton, K., & Jenkins, L. (2000). A Gender Audit for Public Transport: A New Policy Tool in the Tackling of Social Exclusion. Urban Studies, 37 (10) , 1793-1800.
Hanson, S. (1980) Spatial Diversification and Multipurpose Travel: Implications for Choice Theory. Geographical Analysis, 12 (3) , 245–257.
Harmonised European Time Use Survey (HETUS). (2007). Prepared Tables, Main Activities (2-Digit Level) by Sex and Country . Stockholm: Statistics Sweden Population and Welfare Statistics.
Hoedemaeker, M., Parik, M., Baldi, B., Qiao, Y., Sahr, C., & Nuñez-Fernández, F. (2012). GOAL: Profiles of Older People, First Draft.
Irschik, E. (2008). "Fair Shared City: Gender Mainstreaming Planning Strategy in Vienna, Austria", Proceedings of CaixaForum, November 17-18, Madrid.
McGuckin, N., & Nakamoto, Y. (2005a). Differences in Trip Chaining by Men and Women. In United States National Research Council, Research on Women's Issues in Transportation Report of a Conference, Vol. II: Technical Papers. Washington, D.C.: Government Publishing Office (GPO).
McGuckin, N., & Murakami, E. (2005b). Examining Trip-Chaining Behavior: A Comparison of Men and Women . Washington, D.C.: United States Department of Transportation.
O'Brien, M., & Shemilt, I. (2003). Working Fathers: Earning and Caring . Manchester: Equal Opportunities Commission.
O'Neil, D. (2010). Deciding on Driving Cessation and Transport Planning in Older Drivers with Dementia. European Geriatric Medicine, 1 (1), 22-25.
Oxley, J., & Charlton, J. (2011). Gender Differences in Attitudes to and Mobility Impacts of Driving Cessation. In Herbel, S., & Gaines, D. (Eds.), Women's Issues in Transportation: Summary of the Fourth International Conference, October 27-30, 2009, Irvine, California , Volume 2, pp. 64-73. Washington, D.C.: United States National Research Council (NRC) Transportation Research Board.
Sahlin, M., & Rosengren, B. (2001). Regeringens Proposition 2001/02:20— Infrastruktur för ett Långsiktigt Hållbart Transportsystem . Stockholm: Regeringskansliet. Sánchez de Madariaga, I. (2013). The Mobility of Care: A new Concept in Urban Transportation. In Sánchez de Madariaga, I., & Roberts, M. (Eds.), Fair Share Cities: The Impact of Gender Planning in Europe . London: Ashgate.
Sánchez de Madariaga, I. (2009). Vivienda, Movilidad, y Urbanismo para la Igualdad en la Diversidad: Ciudades, Género, y Dependencia. Ciudad y Territorio Estudios Territoriales, XLI (161-162) , 581-598.
Schulz, D., & Gilbert, S. (1996). "Women and Transit Security: A New Look at an Old Issue." Proceedings of the Women's Travel Issues Second National Conference, October 25-27, Baltimore.
Swedish Road Administration (SRA). (2010). The Road Transport Sector— Sectoral Report 2009. Stockholm: SRA.
Swedish Road Administration (SRA). (2009). The Road Transport Sector— Sectoral Report 2008 . Stockholm: SRA.
Transport for London. (2007). Gender Equality Scheme . London: Group Publishing.
United States Bureau of Labor Statistics. (2011) . American Time Use Survey—2010 Results . Washington, D.C.: United States Department of Labor.
United States Bureau of Labor Statistics, 2004). A merican Time Use Survey—2003 Results . Washington, D.C.: United States Department of Labor.
United States Federal Highway Administration. (2009). National Household Travel Survey (NHTS) Summary of Travel and Trends. Washington, D.C.: Government Publishing Office (GPO).
The chart on the right (above) shows what happens if you conceptualize "caring work" as a category in data collection. It becomes the second largest, and one that needs to be considered when designing travel routes.
Transportation, driven by data – Transport for Greater Manchester case study
An official website of the United States government Here's how you know
Official websites use .gov A .gov website belongs to an official government organization in the United States.
Secure .gov websites use HTTPS A lock ( Lock A locked padlock ) or https:// means you’ve safely connected to the .gov website. Share sensitive information only on official, secure websites.
Public Roads - Summer 2024
What's New
Postevent Connected-Vehicle Data Exploration—Lessons Learned
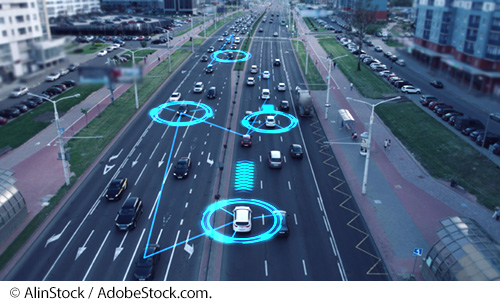
“As more connected and autonomous vehicles are getting adopted and operated on public roads, the amount of data generated by such vehicles is becoming more abundant. How to take advantage of such data to make public travel safer and more efficient is critically needed,” says Brian Cronin, acting director, Intelligent Transportation Systems (ITS) Joint Program Office (JPO), U.S. Department of Transportation.
The Federal Highway Administration’s Office of Highway Policy Information (HPPI) explored the original equipment manufacturer (OEM) and the USDOT ITS JPO connected-vehicle (CV) pilot project data. CV data are created for in-situ and real-time vehicle travel usage. However, postevent analyses of CV data offer significant and valuable information to facilitate the development of safer and more efficient roads. This short briefing introduces the CV data, their highly valuable information, the analytical platform and tools needed to analyze them, and data quality issues for both decisionmakers and data analysts.
Data Variables
Variables contained in any dataset offer a good indication of its value. The CV dataset HPPI explored came from two different sources: OEMs and the ITS JPO CV pilot. The OEM CV data are divided into two categories: vehicle movements and driving events. Vehicle movements have 14 specific variables, including ignition switch status, vehicle geolocations, speed and heading. The driving event dataset has over 40 variables that record different events and maneuvers, including vehicle movements (such as acceleration/deceleration and windshield wiper status), fuel level, odometer reading, and seatbelt statuses of both driver and front passenger.
Variables from the ITS JPO pilot CV datasets varied depending on the sites (Florida, Wyoming, and New York City) in which the pilots were conducted. Regardless of the sites, the ITS JPO pilot CV data had the vehicle movement variables, including speed, acceleration, and deceleration information HPPI intended to explore. However, the ITS JPO CV data did not contain information on seatbelt or wiper usage.
Overall, the explored CV data offer abundant variables enabling unique and otherwise nonexistent and relevant information extraction. The CV data show high potential value.
CV Data Size
The CV data are voluminous. For example, the OEM CV data for Florida, in one day, and with about 10-percent vehicle penetration rates, is approximately 60 gigabytes (GB). For the ITS JPO CV pilot data, the three sites generated over 33 GB per day. As a reference, the FHWA National Bridge Inventory annual data is approximately 1.5 GB, and the FHWA Highway Performance Monitoring System annual data is about 4 GB.
Attention should be directed to the data size issue from the start of any CV data project. Decisions related to whether an entity should acquire, own, or have access to such data should take this issue into full consideration.
Information Explored
HPPI hypothesized for this study that roadway geolocations experiencing a high frequency of extreme vehicle maneuvers may have potential geometric and other infrastructure or operational deficiencies. The identification of such potential deficiencies can facilitate solutions and strategies to improve roadway safety and operational efficiency.
OEM and ITS JPO pilot CV data enabled HPPI to identify such geolocations. The criteria used for the identification include sudden acceleration (hard pedal) and sudden deceleration (hard brake). Such maneuvering events can be easily identified in the OEM data, as the OEM data already tagged such events. For the ITS JPO pilot CV data, HPPI applied a deceleration rate of 3.4 meters per second squared (11.1 feet per second squared). The frequency can be ranked by per linear distance, per square area, or a normalized frequency (number of events divided by the annual average daily traffic) to account for the flow density differences among different roadways. In addition to the longitudinal brake and acceleration variables, the ITS JPO pilot CV data also have lateral acceleration information, which offers insight into vehicle stability during turning maneuvers. All the analyses are also coupled with speed and other environmental factors, such as precipitation and headlight status. For example, the graph on hard-brake densities shows a hard-brake density distribution together with average travel speed over the entire area of Florida.
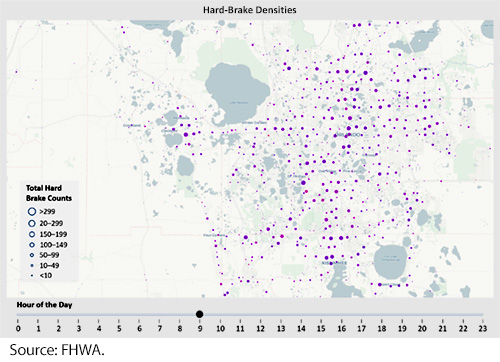
The second major exploration of the CV data was seatbelt usage. Seatbelt data collection is highly complex, often relies on surveys or observations, and is expensive. The OEM CV data offer seatbelt usage information in a highly detailed manner. For example, seatbelt usage for drivers and front passengers can be computed by distance and time traveled during a trip, regardless of the number of unbuckled or buckled events. In addition, seatbelt usage during a journey can be fully studied in sync with vehicle speed and any other vehicle movement data (e.g., acceleration and deceleration).
Data Analytical Platform
CV data are big, regardless of the angle from which it is reviewed. It is critical to select the right data analytical platform that supports broad program languages (e.g., Python, Standard Query Language, and R). HPPI analyzed OEM CV data and ITS JPO CV data through two different Databricks platforms (one provided by Wejo and one provided by the Turner-Fairbank Highway Research Center (TFHRC)). The latter platform should directly access the ITS JPO CV data sources through its cloud-based storage, as they are separated from the platform.
CV Data Quality
During the HPPI CV data exploration, the research team discovered that both the OEM and the ITS JPO pilot CV data had data quality issues even though they were machine generated. This issue is a reminder that users of such data should conduct data quality reviews from the start.
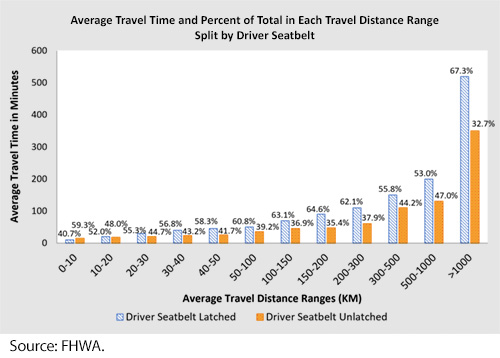
HPPI’s initial exploration of postevent CV data shows these data’s significant value in terms of deriving relevant but otherwise nonexistent information to enable safer and more efficient roads. The use of CV data to identify roadway geolocation where extreme vehicle maneuvers occurred and how drivers and frontal passengers used their seatbelts is unprecedented. Post-CV data analysis and exploration require a broad team effort given the data’s complexity, size, analytical expertise needed, and subject matter knowledge desired.
Acknowledgement
Wejo provided sample CV data and a Databricks platform for the Florida case evaluation. ITS JPO provided a portion of the CV data used in the analysis in this exploration. For the ITS JPO CV data analysis, TFHRC provided the Databricks platform through its Path to Advancing Novel Data Analytics Laboratory. FHWA and all its offices and units do not endorse products or manufacturers. Trademarks or names appear in this article only because they are considered essential to the objective of the document.
Shuqing Wang, P.E., Ph.D., is the National Traffic Data and Advanced Analytics Program Manager in the FHWA’s Travel Monitoring and Surveys division. He has Ph.D./M.S./B.S. degrees in bridge engineering from Tongji University and is a registered professional engineer in Virginia.
Tianjia Tang, P.E., Ph.D., is the chief of FHWA’s Travel Monitoring and Surveys division. Dr. Tang has a B.S. in civil engineering from the University of Central Florida and a Ph.D. from the University of Arkansas. He is a registered professional engineer in the State of Georgia.
Brian Brotsos, B.S., is an FHWA chief data officer managing digital solutions and data strategy. Brian has a B.S. in computer science from DePaul University.
David Winter, P.E., is the director of FHWA’s Office of Highway Policy Information. David is a registered professional engineer in Nebraska and Maryland, and has a B.S. in industrial engineering from the University of Nebraska.
For further information, please see the full report at https://www.fhwa.dot.gov/policyinformation/pubs/hpl24012/ .
Failure characteristics and fracture mechanism of overburden rock induced by mining: A case study in China
- Open access
- Published: 31 May 2024
- Volume 11 , article number 44 , ( 2024 )
Cite this article
You have full access to this open access article
- Jiawei Li 1 , 2 ,
- Meng Zhang 3 ,
- Changxiang Wang ORCID: orcid.org/0000-0002-4202-7231 3 ,
- Changlong Liao 3 &
- Baoliang Zhang 4
This study employs similar simulation testing and discrete element simulation coupling to analyze the failure and deformation processes of a model coal seam's roof. The caving area of the overburden rock is divided into three zones: the delamination fracture zone, broken fracture zone, and compaction zone. The caving and fracture zones' heights are approximately 110 m above the coal seam, with a maximum subsidence of 11 m. The delamination fracture zone's porosity range is between 0.2 and 0.3, while the remainder of the roof predominantly exhibits a porosity of less than 0.1. In addition, the numerical model's stress analysis revealed that the overburden rock's displacement zone forms an 'arch-beam' structure starting from 160 m, with the maximum and minimum stress values decreasing as the distance of advancement increases. In the stress beam interval of the overburden rock, the maximum value changes periodically as the advancement distance increases. Based on a comparative analysis between observable data from on-site work and numerical simulation results, the stress data from the numerical simulation are essentially consistent with the actual results detected on-site, indicating the validity of the numerical simulation results.
Avoid common mistakes on your manuscript.
1 Introduction
There are many investigations that focuses on solid mechanics of fractures in different mining cases (Zou et al. 2022 ; Hannah et al. 2022 ; Li et al. 2022 ) Various mathematical methods have been proposed to simulate the height of the pressure relief zone based on the geometric form of mining disc-shaped roof strata, including five novel methods introduced by Abbas et al. ( 2012 ). Guo et al. ( 2009 ) employed a combination of similar and numerical simulations to determine that the failure modes of overburden strata in coal mining are primarily bending, fracture, and separation. Similarly, Ye et al. ( 2018 ) conducted similar simulation experiments to discover that cracks frequently occur at both ends of the goaf and are distributed in a trapezoidal pattern under the influence of coal-rock mining effects. Chen et al. ( 2022 ) indicated that the dynamic load can connect the goaf and the separation layer by studying the dynamic load caused by the separation layer. Ma et al. ( 2019 ) reported that during the mining process, the overlying strata exhibited characteristics of periodic collapse, and the separation cracks also underwent a cycle of 'generation-development-expansion-continuous-disappearance-compaction'.
Guo et al. ( 2021 ) established two corresponding mechanical models for overlying strata deformation and obtained the maximum settlement equation of overlying strata bending basin. Jia et al. ( 2022 ) found through similar simulation experiments that the subsidence of overburden rock is nonlinear, and the form of subsidence is asymmetric. Zhang et al. ( 2010 ) discovered that the "arch-beam" structure formed by surrounding rock cracks is the main factor causing damage and instability, leading to roof collapse. Ren et al. ( 2013 ) found through similar simulation experiments that in the mining process of shallow-buried longwall working faces, the fracture of the main roof can easily extend the fracture zone to the surface. Liu ( 2011 ) observed that in the coal seam mining process, the overlying strata collapse begins from the immediate roof and gradually expands to the loose layer.
Zhang et al. ( 2014 ) demonstrated that the numerical simulation method can be effectively applied to study the rock fracture migration law. Xiao et al. ( 2022 ) determined that after a limited number of periodic weightings, the height of the fault zone no longer changes vertically but only horizontally with mining. Liu et al. ( 2022 ) analyzed the subsidence of overburden rock, noting that the overall subsidence trend features significant subsidence in the middle and upper parts and minor subsidence in the lower part, with an asymmetric arch collapse shape.
Based on similarity simulation theory and displacement sensing technology, Zhao et al. ( 2018 ) captured the entire process from the gradual development of the abscission layer to catastrophic evolution. Li et al. ( 2005 ) determined that the limit span of the roof is 110 m by combining numerical and similar simulations. Zhang et al. ( 2014 ) proved the reliability and complementarity between similar simulation experiments and field detection studies. Li et al. ( 2018 ) derived the fracture evolution law of floor strata under the influence of coal seam mining through similar simulation experiments. Yang et al. ( 2009 ) analyzed the mechanical characteristics of rock strata with different mining thicknesses using similar simulations and determined the displacement change of overburden rock and the distribution law of collapsed rock.
Through the verification of field monitoring data, Coggan John et al. ( 2012 ) demonstrated that numerical simulation can provide valuable insights into the behavior of coal mine working face roofs and related reinforcement designs. They also suggested that simulation can confirm the adverse effects of unfavorable geostress direction. Ma et al. ( 2021 ) identified the failure characteristics and fracture evolution patterns of overburden rock during the mining process by combining numerical simulation with similar experimental methods. Cai et al. ( 2007 ) established the effectiveness of PFC and FLAC software and their coupling method in engineering analysis. Yang et al. ( 2006 ) discovered that in PFC2D, the stress–strain relationship of the model is primarily influenced by the elastic modulus and is minimally affected by particle size. Yasitli et al. ( 2005 ) reported that coal above the fracture zone cannot be completely shattered or broken into large blocks through numerical simulation and field observation.
This study analyzes the failure mechanisms of strata in the working face by examining the development trends of overburden fractures and the displacement of survey lines and validating the experimental results through numerical simulation. It also investigates the subsequent mining process, which is challenging to achieve in actual experiments. In addition, this research emphasizes conducting specific investigations based on the local conditions of different geological occurrences. It explores the overburden failure of the 40204 working face in a Chinese coal mine, using similar simulation experiments and the discrete element simulation technique. It focuses on the rock mass's failure characteristics by analyzing the roof's stress distribution and fracture development characteristics during deformation and failure. The findings provide insights for identifying air leakage positions in the goaf and predicting subsequent pressure build-ups.
2 Coal mine working face background
The fully mechanized caving face, 40204, of a coal mine in China, examined in this study, is part of the fourth coal seam. The 40204 working face is the second fully mechanized caving face situated in the southern wing of the 402 mining area. It boasts a strike length of 1871 m, a dip length of 220 m, a recoverable length of 1721 m, and is buried at a depth of approximately 590 m. The average thickness of the coal seam is 11.6 m, with a recoverable thickness of 10.0 m. This working face is configured with one access point and three slopes for transportation, return air, and drainage. To the east of the 40204 working face lies the 40202 goaf, with the goaf of the Jiangjiahe mine field to the south, solid coal to the west, and the protective coal pillar of the fourth coal roadway to the north. The geological cross-section of the 40204 working face is depicted in Fig. 1 .
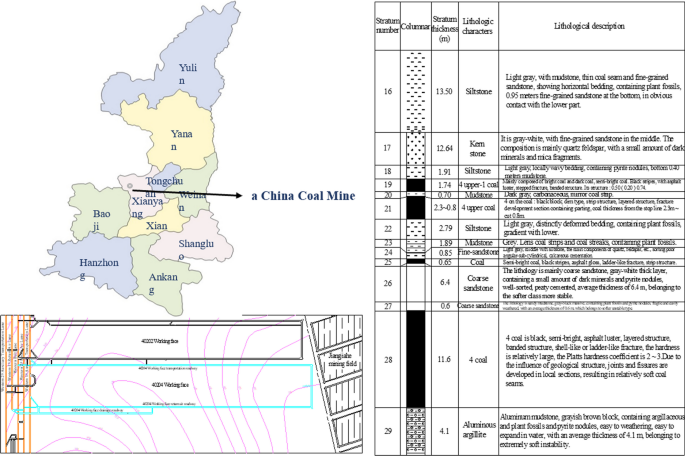
40204 working faces primary condition
The study considers the lithology, thickness, and mechanical characteristics within 50 m above the fourth coal seam through the physical and mechanical properties of the stratum analogous to that of the 40204 working faces found in the mine's geological data, as listed in Table 1 .
3 Similar simulation experiment
3.1 laying experimental model.
Based on the principles of similarity theory, accurate experimental results reflecting the actual conditions can only be achieved when the scale model and the prototype share geometric and mechanical parameter similarities (Yang 2005 ). Given the proportionality between the coal seam thickness and the overlying rock thickness in this experiment, a geometric similarity ratio \(C_{\text{L}}\) of 1:200 was adopted for the model. Based on this ratio, the model measures 190 cm in length, 22 cm in thickness, and 110 cm in height, as illustrated in Fig. 2 . During the simulation test, the overburden load was regulated using a load control system.
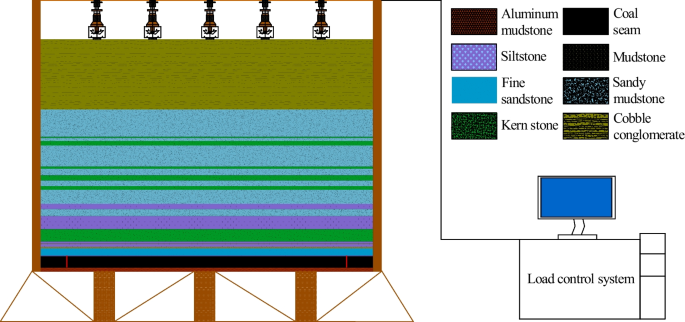
Actual model diagram
Similar materials primarily consist of fillers (or aggregates) and cements. In this experiment, river sand was selected as the filler for the model and mixed with gypsum in proportion. Calcium carbonate was added to enhance its strength. Layers of rock with varying strengths were created using different material ratios. The ratio for each rock layer was determined based on the mechanical parameters of the rock layer in Table 1 . The quantities of sand, calcium carbonate, and gypsum in each layer were calculated according to the layer's bulk density. The water content was approximately half that of the gypsum. These layers were constructed in multiple segments to simulate the stratification phenomenon in thicker rock layers accurately. Table 2 details the specific layout and material usage for each layer.
During the model construction, the model's front and rear restraint guard plates were first attached to the experimental frame sequentially with the layering work. Then, the materials required for each layer were measured according to Table 2 , mixed with water, and stirred until homogeneous. The mixture was then poured into the model, leveled, and compacted with a scraper. After completing a layer, a thin layer of mica powder was spread to separate it from the subsequent rock layer, continuing upwards until reaching the designed height. Due to spatial constraints, external loads were applied at the model's top to simulate the pressure of the unreached rock formations.
After the construction, to precisely monitor the overburden rock movement and surface deformation, grid lines were drawn on the model's front using ink, with a grid size of 10 × 10 cm. Reflective sheets, sized 2 × 2 cm, were placed at grid intersections to serve as markers, facilitating displacement observations. A total of ten displacement observation lines were established above the coal seam, monitoring ten data points from bottom to top: A-10.0 cm survey line (10.0 cm above the coal seam), B-20.0 cm survey line, C-30.0 cm survey line, D-40.0 cm survey line, E-50.0 cm survey line, F-60.0 cm survey line (with subsequent lines following in sequence), and each line has 18 measurement points.
3.2 Analysis of overlying rock failure law
The test model was dried approximately 15 days after formation and then excavated. The open-off cut was positioned 15 cm from the model's left boundary to mitigate boundary effects, establishing a boundary-protective coal pillar of 15 cm. The model extended 190 cm in length, with 160 cm being recoverable.
Kong et al. ( 2019 ) discovered through rock failure experiments and numerical simulations that the uniaxial compressive strength of mudstone is low, making it susceptible to destruction during the mining process at the working face, leading to roof collapse. The influence range of the abutment pressure on the working face extends approximately 40 m. Therefore, during coal seam mining, excavation proceeded in 20 cm increments, allowing the bedrock to stabilize for 2 h after each excavation. In order to compare the results against the field monitoring measurements, the data used in the subsequent analysis are scaled field data at a 1:200 ratio.
The process of the overlying strata's movement and the roof's subsidence are depicted in Fig. 3 .
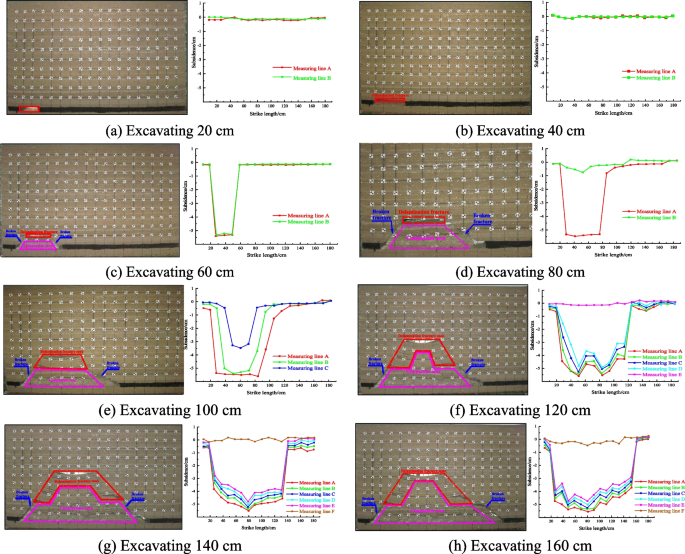
Roof failure diagram under different excavation lengths
When the working face advances to 40 m, the direct roof does not collapse, and the bending settlement of the overlying strata is hardly noticeable. As the advance reaches 80 m, the direct roof begins to collapse, but the surrounding rock's integrity remains unchanged. Monitoring the subsidence curve of the roof survey line indicates that roof subsidence is almost negligible during this phase.
As the working face progresses to 120 m, the rock beam on both sides of the model roof fractures and begins to show cracks. A distinct delamination fracture occurs approximately 20 m above the coal seam. Concurrently, the roof subsidence curve shows significant changes, with the maximum roof subsidence reaching 11 m, nearly equivalent to the thickness of the coal seam.
When the working face progresses to 160 m, the separation position expands upward, and the fracture extends laterally. At an advancement of 200 m, distinct zoning phenomena become evident in the caving area, categorized into delamination fracture zone, broken fracture zone, and compaction zone. Both the delamination fracture and compaction zones assume a trapezoidal shape. The separation fracture's height develops to 60 m. Roof subsidence curves indicate subsidence occurring below the separation fracture at the caving area's top, with the maximum subsidence remaining unchanged.
Upon excavating the coal seam to 240 m, the highest point of the separation fracture zone develops to 100 m above the coal seam, and the cantilever rock layer in the goaf undergoes periodic breaks. The delamination fracture zone transitions from trapezoidal to arch-shaped, while the compaction zone adopts a tower-like form. Upon excavating to 280 m, the delamination fracture zone rises to 110 m above the coal seam and expands horizontally. At 320 m excavation, the vertical height of the delamination fracture zone ceases to change, extending only horizontally with the continuous coal seam advancement. Roof subsidence curves from various survey lines reveal that roof subsidence decreases as the vertical height increases.
Visible fractures generally do not occur in rock formations above 110 m from the coal seam in the final stages of mining. Consequently, the height of the caving and fracture zones in the bedrock is determined to be 110 m above the coal seam. The distribution of subsidence curves at different advancement distances is approximately symmetrical, with the center point of the subsidence curve progressively shifting forward as the advancing distance increases.
3.3 Distribution law of expansion coefficient and porosity
The dilatancy coefficient is defined as the ratio of the rock mass volume after fracturing to the volume of the rock mass before fracturing.
where \(K\) is bulking coefficient; \(V{\prime}\) is rock mass volume after rock breaking, m 3 ; \(V\) is rock mass volume before rock breaking, m 3 .
Constraints exist on the strike and tendency of the working face following the collapse of the broken immediate roof, where bulking is predominantly vertical. Thus, the bulking coefficient after the goaf's immediate roof collapses can approximately equal its vertical bulking. This approximation allows for a simplified analysis of the structural changes post-collapse.
where \(h_{n\sim n + 1}{\prime}\) is the vertical distance between two points before rock breaking, m; \(h_{n\sim n + 1}\) is the vertical distance between two points after rock breaking, m.
The difference in distance between the n and n + 1 measuring points reflects the subsidence difference between the two points. Hence, the initial equation can be modified to account for this spatial variation
where \(\Delta w\) is the sinking difference between two points, m; \(E_{n\sim n + 1}\) is n and n + 1 vertical deformation between two points.
The porosity can be expressed as follows:
Based on the definitions provided, the average bulking factor and porosity variation across each material layer in the working face simulation test are calculated using measuring points, as depicted in Figs. 4 and 5 . Given that the expansion coefficient and porosity distribution tend to align, the analysis focuses solely on the changes in porosity.
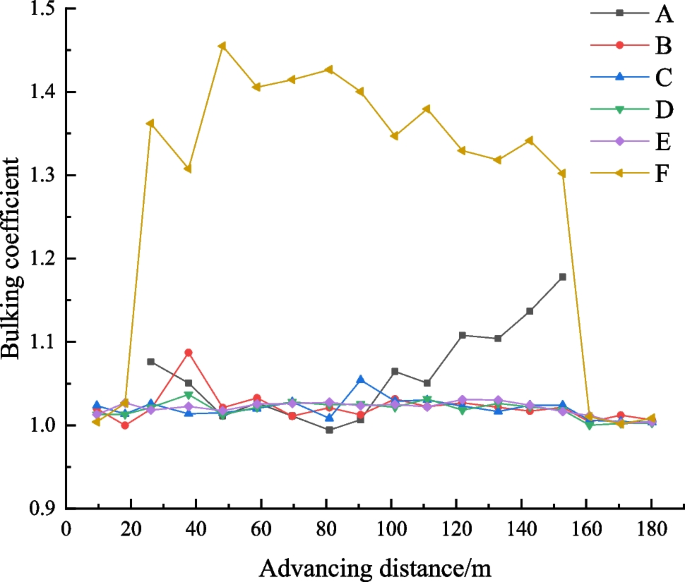
Distribution law of bulking coefficient of each layer after excavation
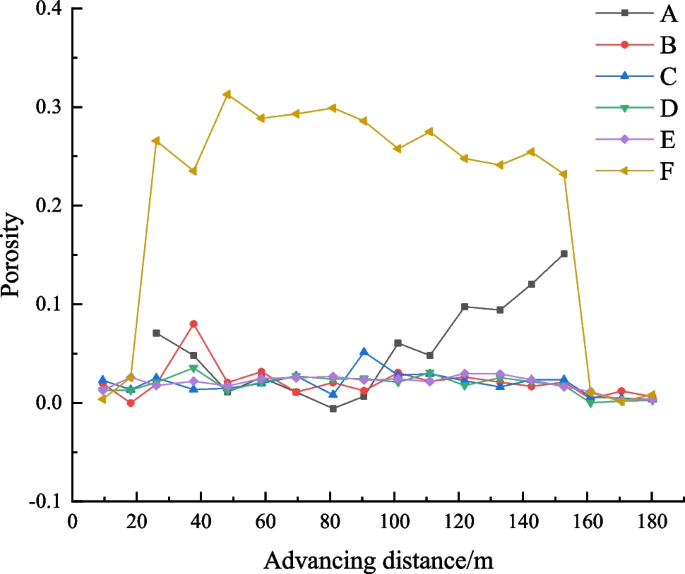
Porosity distribution law of each layer after excavation
Figure 5 indicates that the maximum porosity of the layer appears at the overlying strata 120 m away from the working face, and the porosity of the rock layer increases first and then decreases with the advance of the working face. Hence, it can be inferred that the height of the fracture zone in the working face is about 120 m, which is also basically consistent with the 110 m obtained by similar simulation tests. In addition, this figure also illustrates that the maximum porosity of the fractured zone can reach more than 0.3, but in most mining cases, the porosity of the fractured zone is between 0.2 and 0.3, which correlates with the bedding distribution of the rock formation area. Furthermore, it can be observed from the interlayer porosity curve at 20 m of the overlying strata that the porosity at both ends of the cantilever beam area is the highest, about 0.1. The remaining areas experience continuous compaction during the mining process, resulting in porosity far less than 0.1.
4 Numerical simulation experiment
4.1 building the model and parameter calibration.
When employing the numerical simulation method to address the issue of roof collapse, the modeler needs to make appropriate assumptions, simplifications, calibrations, and verifications of the model to obtain reliable output. The material constitutive model should be capable of simulating strength reduction after rock collapse to enhance collapse analysis (Le et al. 2017 ). Due to computing power limitations, the specific model cannot be entirely built to the surface during modeling. Hence, this study simulates the rock pressure of the surface by adjusting the rock density at the top of the model. In the earth model proposed by Dziewonski and Anderson ( 1981 ), pressure increases by 3 GPa for every 100 km increase in underground rock strata.
where \(P\) is the pressure at different underground depths, MPa; \(\rho\) is the rock stratum density, kg/m 3 ; \(g\) is the acceleration of Gravity Normal, N/kg; \(h\) is the rock stratum height, m.
Considering the buried depth of the coal seam in the 40204 working face, the rock pressure between the surface and the top of the model is obtained, and the density of the upper rock layer can be calculated by applying it to Eq. ( 5 ), facilitating the assignment of model attributes for discrete elements.
Since this experiment observes the failure characteristics of the overburden rock in the advancing direction of the working face, the model is established using PFC2D. The macroscopic mechanical parameters of each rock stratum are presented in Table 1 . The model is 500 m long, and the height of the rock layer is 142.99 m, adhering to a 1:1 ratio. In the discrete element model, the minimum radius of particles is 300 mm, and the maximum radius is 600 mm. The constitutive model between particles is the Linear Parallel Bond Model. In the final model, the number of particles is 92,172, as depicted in Fig. 6 .
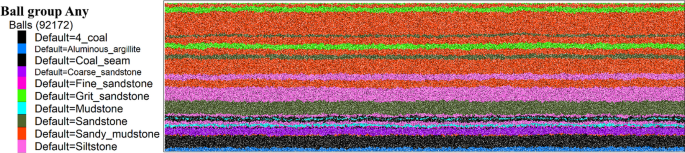
Model diagram of 40204 working face before coal seam mining
In applying constitutive contact to the model, it is essential to calibrate the rock strata's parameters at the specific working face. Previous literature and engineering practices (Yoon 2007 ) have typically employed a 'trial and error' method for parameter calibration. However, this approach demands considerable computing power and proves time-consuming and inefficient. Therefore, this study adopts the parameter fitting formula developed by Feng et al. ( 2022 ) through multiple fitting tests to calibrate the parameters. It can derive the microscopic mechanical parameters required for the model by inserting the macroscopic mechanical parameters of the rock into Eq. ( 6 ).
The macroscopic parameters of each rock stratum in working face 40204 were input into Eq. ( 6 ) to calculate the microscopic parameters for each stratum in the numerical model.
where \(k_{\text{n}}\) is the micro-normal stiffness of the model, GPa; \(K_{\text{n}}\) is the macro normal stiffness of the model, GPa.
The micro-normal stiffness values were determined as follows: mudstone at 9.75 GPa for ball-ball and 11.8 GPa for ball-facet; sandstone at 13.3 GPa for ball-ball and 20.8 GPa for ball-facet; and Coal 4 at 10.6 GPa for ball-ball and 10.9 GPa for ball-facet.
where \(k_{\text{s}}\) is the micro tangential stiffness of the model, GPa; \(K_{\text{s}}\) is the macro tangential stiffness of the model, GPa.
The micro-shear stiffness values were found to be: for mudstone, 3.1 GPa for ball-ball and 5.2 GPa for ball-facet; for sandstone, 12.9 GPa for ball-ball and 107.5 GPa for ball-facet; and for Coal 4, 3.1 GPa for ball-ball and 3.4 GPa for ball-facet.
Based on these values, the micro-elastic modulus of the constitutive model was calculated using Eq. ( 8 ), with the specific modulus distribution illustrated in Fig. 7 .
where \(\overline{\varphi }\) is the micro-internal friction angle of the model, º; \(\varphi\) is the macro internal friction angle of the model, º.
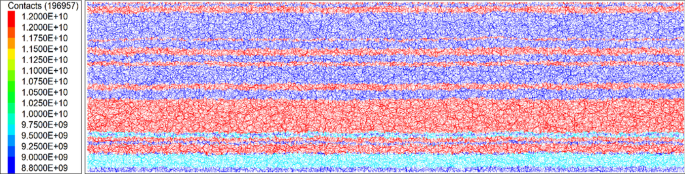
Constitutive model diagram with different rock elastic modulus
The micro-internal friction angles for mudstone, sandstone, and Coal 4 were calculated using Eq. ( 9 ) to be 41°, 50°, and 28°, respectively. Figure 8 displays the distribution of the internal friction angle for different lithologies.
where \(c_{2}\) is the micro cohesion of the model, MPa;
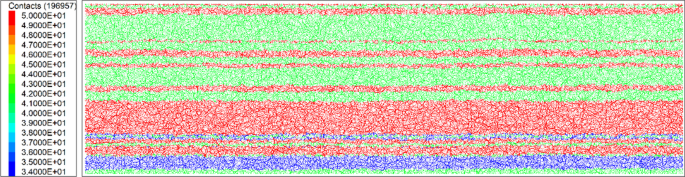
The constitutive model diagram of applying different internal friction angles of rock strata
Based on Eq. ( 10 ), the micro-cohesion values for mudstone, sandstone, and Coal 4 were found to be 0.47, 0.62, and 0.41 MPa, respectively.
where \(c_{\text{b}}\) is the micro-bond strength of the model, MPa; \(c\) is the macro bond strength of the model, MPa.
The micro-bond strengths for mudstone, sandstone, and Coal 4, calculated using Eq. ( 11 ), were 5.3, 2.3, and 2.6 MPa, respectively. The cohesion distribution for different lithologies is shown in Fig. 9 .
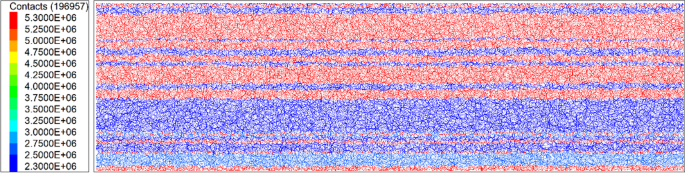
The constitutive model diagram of applying different cohesion of rock strata
4.2 Visual analysis of overburden rock movement
The previous section highlights the simulation experiment's limitations, as it can only simulate overburden failure up to a mining depth of 320 m. This study addresses these limitations by employing numerical simulation methods to model overburden movement and stress change as mining advances. This approach confirms the previous experimental results. Figure 9 depicts the roof displacement at various advancing distances.
Figure 10 demonstrates that as the distance advances from 80 to 440 m, the displacement distribution of roof strata in the caving area exhibits a trapezoidal shape. During the mining process, up to 320 m, the displacement of the roof in most areas of the caving strata ranges from 9 to 12 m, with the maximum displacement reaching 13.7 m at the bottom of the caving strata. The maximum discrepancy in displacement between these findings and those from similar simulations varies from approximately 3.4% to 12%. This indicates that the numerical simulation results largely agree with similar simulation outcomes.
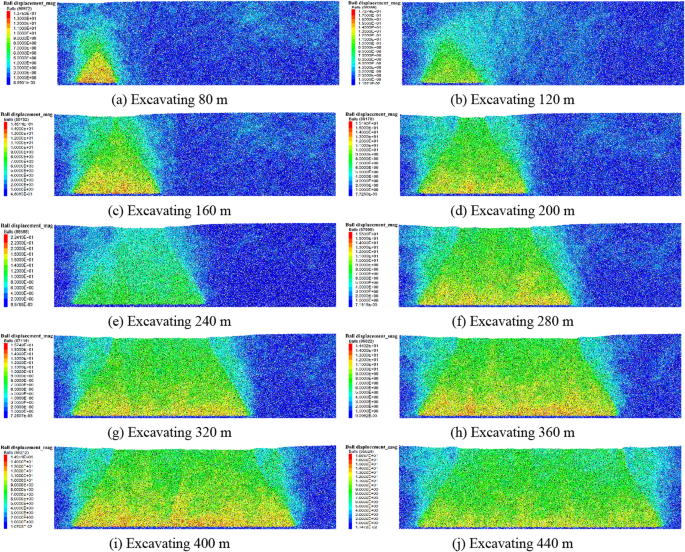
Rock displacement diagram under different advance distances
As the mining distance extends from 320 to 440 m, the normal height of the overlying strata migration area ceases to change, continuing to expand horizontally, and the maximum displacement of the roof gradually stabilizes between 12 and 13 m.
Figure 11 illustrates the stress distribution of rock strata under different advancing distances.
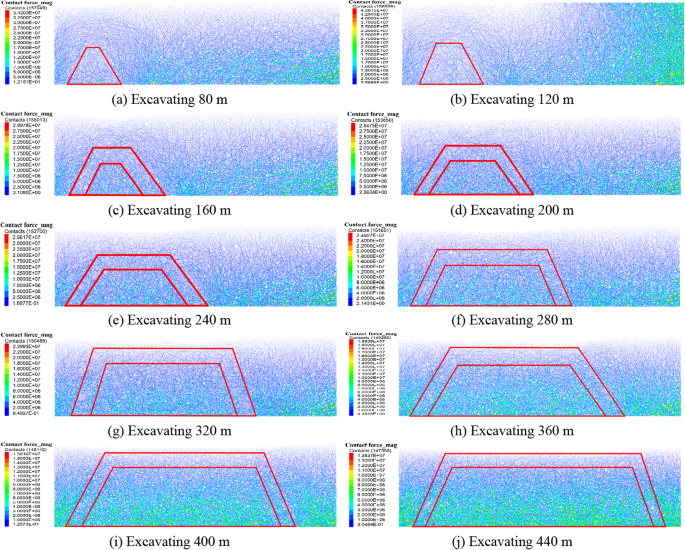
The stress distribution diagram of rock strata under different advancing distances
Figures 11 , 12 , and 13 reveal that during the mining process, from 80 to 120 m, the stress distribution in the displacement area of the overlying strata maintains a trapezoidal shape. The 'arch-beam' structure appears in the overburden displacement area at 160 m. At an advancing distance of 80 m, the minimum and maximum stresses in the roof displacement area are 7.5 and 10 MPa, respectively. When the advancing distance reaches 120 m, these stresses are 7.5 and 12.5 MPa, respectively.
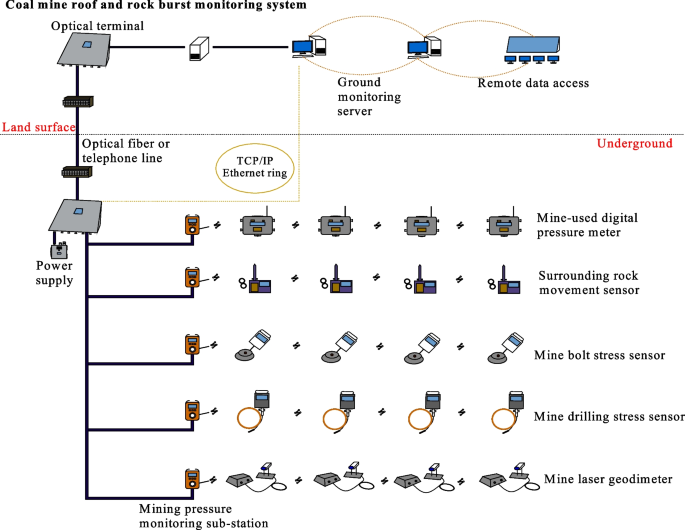
The distribution of the monitoring system
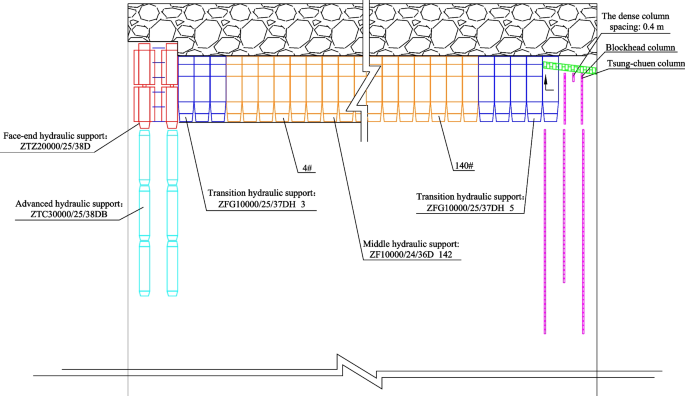
Working face support layout diagram
It is observed from an analysis of stress distribution during the mining process of the working face that the vertical stress distribution in the rock stratum shows a gradual decrease in stress values and a more dispersed stress distribution with increasing distance from the coal seam. This suggests that the stress distribution follows a normal distribution pattern, gradually reducing the dynamic influence area caused by mining in the vertical direction. The distribution of overburden stress in the goaf forms a symmetrical trapezoidal shape, aligning with patterns observed in analogous simulation tests where overburden fissures develop. These fissures originate at the top and propagate laterally on both sides of the working face. In addition, as the working face progresses in the mining process, the stress reduction and dispersion areas tend to re-concentrate during subsequent continuous mining, leading to the re-compaction of fractures in previously mined areas. The accuracy of this analysis is confirmed through Figs. 2 f–h.
5 Field monitoring results
5.1 monitoring system and support distribution.
The newly established KJ24 coal mine roof and rock burst monitoring system at Dafosi Mine, part of the Binchang Mining Industry, was officially launched. This system enables real-time online monitoring, transmission, intelligent analysis, and early warning of critical data such as roadway roof separation and anchor cable stress. It enhances the mine's safety monitoring capabilities and assurance coefficient, providing robust and scientific data support for optimizing roadway support parameters. The specific layout is depicted in Fig. 12 .
The working face is equipped with 150 hydraulic supports, comprising eight head and tail transition supports and 142 middle supports. It is outfitted with 18 pressure sensor monitoring substations within the middle supports, each overseeing four measurement points on a set of hydraulic supports. The measurement points are distributed approximately every seven units from the #4 frame of the working face to the machine's tail. The sequence of frames includes #4, #12, #20, #28, #36, #44, #52, #60, #68, #76, #84, #92, #100, #108, #116, #124, #132, and #140. The support layout is illustrated in Fig. 13 . The collected data are then transmitted to the surface computer and analyzed by technicians.
5.2 Analysis of monitoring results
The exported real-time monitoring data undergoes post-processing, and the segments that conform to the specified advancing distance intervals are compiled into a table, as listed in Table 3 .
The stress interval diagram is illustrated in Figs. 14 and 15 using the numerical simulation results and the field monitoring data.
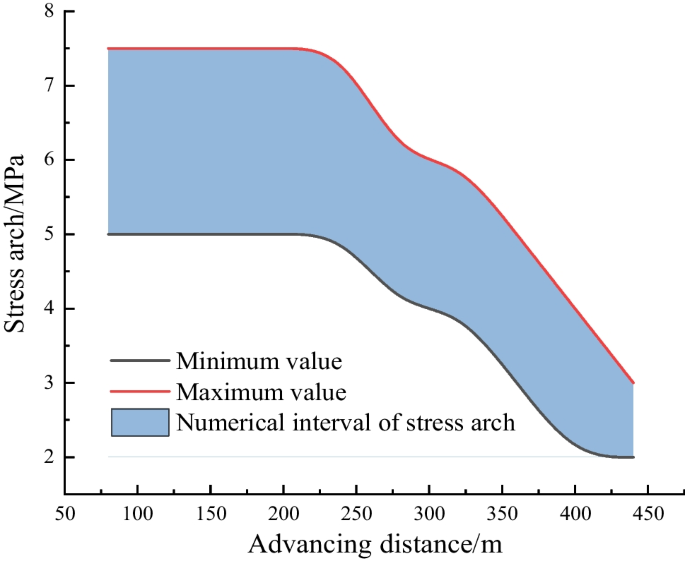
Value range of stress arch under different advancing distance
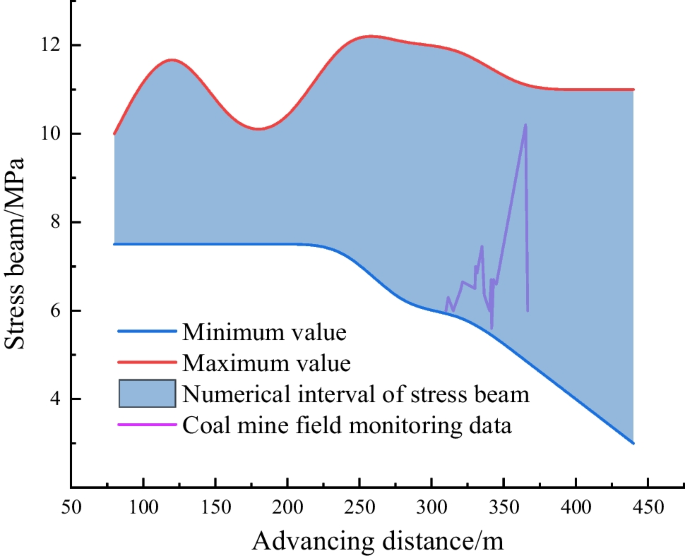
Value range of stress beam under different advancing distance
Analysis of the stress interval diagram reveals that within the overburden rock's failure stress arch interval, the maximum and minimum stress values decrease as the advancing distance increases. In the overburden rock's stress beam interval, the maximum stress value fluctuates periodically with an increase in the advancing distance, while the minimum value consistently declines. Figure 15 compares the real-time monitoring data from the coal mine site with the stress beam interval range. It indicates that the in-field stress fluctuates within the simulated stress range, validating the simulation study's accuracy.
6 Conclusions
This research innovatively analyzed the failure characteristics of overlying strata in the working face of specific coal mines, utilizing the coupling of field similarity simulation experiments and discrete element numerical simulations. This analysis provides reference for solving engineering problems. The main conclusions are as follows:
The similarity simulation experiment results indicated that the fracture zone's height in the 40204 working face is approximately 110 m above the coal seam. The fractures predominantly extend laterally, with separation cracks and compaction areas developing in vertical and horizontal directions. In addition, throughout the entire excavation process, the maximum subsidence of the roof stabilizes at 11 m, corresponding to the coal seam's thickness. The porosity in the area where the separation fracture occurred ranges from 0.2 to 0.3, whereas the porosity of the remaining roof is less than 0.1.
The caving zone at a mining depth of 200 m presents a trapezoidal shape, composed of an 'arch-beam' structure and can be categorized into three zones: 'delamination fracture zone', 'broken fracture zone', and 'compaction zone'. The numerical simulation displacement results indicated that most roof strata areas displace between 9 and 12 m, with a maximum displacement of 13.7 m. Compared to the similarity simulation's roof subsidence results, the error range is between 3.4 and 12%, which aligns closely with the similarity simulation outcomes.
Failure stress analysis indicated that the stress distribution in the overburden displacement area remains trapezoidal during mining at depths of 80 to 120 m. The overlying strata's displacement area exhibits an 'arch-beam' structure from a mining depth of 160 m. The maximum and minimum values of the failure stress arch interval of the overburden rock decrease as the advancing distance increases. In the stress beam interval of the overburden rock, the maximum value shows periodic changes with the increase in advancing distance, while the minimum value continues to decrease.
Data availability
Some or all data, models, or codes generated or used during the study are available from the corresponding author by request.
Abbas M, Ferri PH, Mehdi YN (2012) Prediction of the height of destressed zone above the mined panel roof in longwall coal mining. Int J Coal Geol 98:62–72. https://doi.org/10.1016/j.coal.2012.04.005
Cai M, Kaiser PK, Morioka H, Minami M, Maejima T, Tasaka Y, Kurose H (2007) FLAC/PFC coupled numerical simulation of AE in large-scale underground excavations. Int J Rock Mech 44:550–564. https://doi.org/10.1016/j.ijrmms.2006.09.013
Article Google Scholar
Chen LW, Ou QH, Peng ZH, Wang YX, Chen YF, Tian Y (2022) Numerical simulation of abnormal roof water-inrush mechanism in mining under unconsolidated aquifer based on overburden dynamic damage. Eng Fail Anal 133:106005
Coggan J, Gao F, Stead D, Elmo D (2012) Numerical modelling of the effects of weak immediate roof lithology on coal mine roadway stability. Int J Coal Geol 90–91:100–109. https://doi.org/10.1016/j.coal.2011.11.003
Dziewonski AM, Anderson DL (1981) Preliminary reference Earth model. Phys Earth Planet Inter 25:297–356. https://doi.org/10.1016/0031-9201(81)90046-7
Feng HT, Chen JZ, Ren CF, Ma YT, Zhang MY (2022) Study on calibration method of cemented structure plane parameters in numerical test. China J U S Eng 18:1824–1833
Google Scholar
Guo GL, Zha JF, Miao XX, Wang Q, Zhang XN (2009) Similar material and numerical simulation of strata movement laws with long wall fully mechanized gangue backfilling. Procedia Earth Planet Sci 1:1089–1094. https://doi.org/10.1016/j.proeps.2009.09.167
Guo WB, Zhao GB, Bai EH, Guo MJ, Wang Y (2021) Effect of overburden bending deformation and alluvium mechanical parameters on surface subsidence due to longwall mining. Bull Eng Geol Environ 80:2751–2764. https://doi.org/10.1007/s10064-020-02091-4
Hannah M, Sudeshna B, Alberto S, Melanie M (2022) Advances of nanotechnologies for hydraulic fracturing of coal seam gas reservoirs: potential applications and some limitations in Australia. Int J Coal Sci Technol 9(1):27. https://doi.org/10.1007/s40789-022-00497-x
Jia D, Jiang DY, Chen J, Fan JY, Ren YW (2022) Similar simulation study on failure characteristics and fracture evolution of overlying strata in fully-mechanized caving face. J Saf Sci Technol 18(03):19–25. https://doi.org/10.11731/j.issn.1673-193x.2022.03.003
Kong DZ, Han CH, Lou YH, Zheng SS, Pu SJ, Wang ZJ (2019) Study on ground pressure behavior law of a large-cutting-height panel with large dip angle. Geotech Geol Eng 37(5):3805–3815. https://doi.org/10.1007/s10706-019-00869-2
Le TD, Mitra R, Oh J, Hebblewhite B (2017) A review of cavability evaluation in longwall top coal caving. Int J Min Sci Technol 27:907–915. https://doi.org/10.1016/j.ijmst.2017.06.021
Li HL, Bai HB, Ma D, Tian CD, Zhang Q (2018) Physical simulation testing research on mining dynamic loading effect and induced coal seam floor failure. J Min Saf Eng 35(02):366–372
Li YJ, Song LH, Tang YJ, Zuo JP, Xue DJ (2022) Evaluating the mechanical properties of anisotropic shale containing bedding and natural fractures with discrete element modeling. Int J Coal Sci Technol 9(1):18. https://doi.org/10.1007/s40789-022-00473-5
Li XY, Li JP, Zhou CB, Xiang WF (2005) Comparative study on numerical simulation and similarity simulation of overburden deformation in abandoned stope. Rock Soil Mech 12:1907–1912. https://doi.org/10.3969/j.issn.1000-7598.2005.12.008
Liu C, Li ZH, Yang K, Chi XL, Zhou P, Zhao SH (2022) Similarity simulation study on mine pressure behavior of intelligent mining face with large dip angle. Min Re Dev 42(07):86–91
Liu CG (2011) Similar simulation study on the movement behavior of overlying strata in shallow seam mining in Majiliang Coal Mine. J China Coal Soc 36(01):7–11
Ma HW, Sui WH, Ni JM (2019) Environmentally sustainable mining: a case study on surface subsidence control of grouting into overburden. Environ Earth Sci. https://doi.org/10.1007/s12665-019-8313-1
Majdi A, Hassani FP, Nasiri MY (2012) Prediction of the height of destressed zone above the mined panel roof in longwall coal mining. Int J Coal Geol 98:62–72. https://doi.org/10.1016/j.coal.2012.04.005
Ma JH, Hou C, Hou JT (2021) Numerical and similarity simulation study on the protection effect of composite protective layer mining with gently inclined thick coal seam. Shock Vib. https://doi.org/10.1155/2021/6679199
Ren YF, Ning Y, Qi QX (2013) Physical analogous simulation on the characteristics of overburden breakage at shallow longwall coalface. J China Coal Soc 38(01):61–66
Xiao P, Han K, Shuang HQ, Wu MC, Gao Z (2022) Similar material simulation test study on evolution law of overburden fracture based on microseismic monitoring. J Coal Sci Technol 50(09):48–56. https://doi.org/10.13199/j.cnki.cst.2020-1400
Yang BD, Jiao Y, Lei ST (2006) A study on the effects of microparameters on macroproperties for specimens created by bonded particles. Eng Comput 23:607–631
Yang J (2005) Similarity theory and structural model test. Wuhan University of Technology Press, Wuhan
Yang K, Xie GX, Chang JC (2009) Experimental investigation into mechanical characteristics of surrounding rock with different mining thickness. J China Coal Soc 34(11):1446–1450. https://doi.org/10.3321/j.issn:0253-9993.2009.11.002
Yasitli NE, Unver B (2005) 3D numerical modeling of longwall mining with top-coal caving. Int J Rock Mech 42:219–235. https://doi.org/10.1016/j.ijrmms.2004.08.007
Ye Q, Wang G, Jia Z, Zheng C, Wang W (2018) Similarity simulation of mining-crack-evolution characteristics of overburden strata in deep coal mining with large dip. J Pet Eng 165:477–487. https://doi.org/10.1016/j.petrol.2018.02.044
Yoon J (2007) Application of experimental design and optimization to PFC model calibration in uniaxial compression simulation. Int J Rock Mech 44:871–889. https://doi.org/10.1016/j.ijrmms.2007.01.004
Zhang MJ, Gao JH, Wei SY, Chen XM, Chen WZ (2010) Similarity simulation study of failure characteristics of surrounding rocks of tilted strata roadway. Chin J Mech Eng 29(S1):3259–3264
Zhang XC, Sun Y, Wang Z, Zhou X, Wang R, Liu TL (2014) Simulation sensitivity analysis of migration velocity of CO 2 in geological sequestration based on single joint. Eng Sci Technol 33(14):147–151. https://doi.org/10.3969/j.issn.1671-1815.2014.33.026
Zhang J, Wang JP (2014) Similar simulation and practical research on the mining overburden roof strata “three-zones” height. J Min Saf Eng 31(02):249–254
Zhao QF, Zhang N, Peng R, Li GC, Zhang KX, Han CL, Shi JJ (2018) Similarity simulation experimental study on abrupt collapse of roof separation in large cross-section argillaceous roadway. J Min Saf Eng 35(06):1107–1114
Zou GG, Zhang QH, Peng SP, She JS, Teng DL, Jin CC, Che YY (2022) Influence of geological factors on coal permeability in the Sihe coal mine. Int J Coal Sci Technol 9(1):6. https://doi.org/10.1007/s40789-022-00475-3
Download references
This research was financially supported by the National Key R&D Program of China (2023YFC3009100, 2023YFC3009102), National Natural Science Foundation of China (52304198) and Open Fund of the National and Local Joint Engineering Research Center for Safe and Accurate Coal Mining(EC2021016).
Author information
Authors and affiliations.
Department of Energy and Power Engineering, College of Mechanical and Transportation Engineering, China University of Petroleum (Beijing), Beijing, China
Department of Energy and Power Engineering, Tsinghua University, Beijing, China
College of Safety Science and Engineering, Anhui University of Science and Technology, Huainan, 232000, China
Meng Zhang, Changxiang Wang & Changlong Liao
School of Architecture and Civil Engineering, Liaocheng University, Liaocheng, 252000, China
Baoliang Zhang
You can also search for this author in PubMed Google Scholar
Contributions
All the authors contributed to publishing this paper. J. Li and C. Wang conceived the main idea of the paper; M. Zhang contributed to the theoretical analysis analyzed the data; C. Wang and B. Zhang wrote the paper; C Liao modified the figures.
Corresponding authors
Correspondence to Changxiang Wang or Baoliang Zhang .
Ethics declarations
Ethics approval.
Ethical approval is not applicable because this article does not contain any studies with human or animal subjects.
Consent to publish
The authors confirm: That the work described has not been published before; That it is not under consideration for publication elsewhere; That its publication has been approved by all co-authors, if any; That its publication has been approve by the responsible authorities at the institution where the work is carried out.
Conflict of interest
The authors declare that they have no financial or personal relationships with other people or organizations that can inappropriately influence this work, and there is no professional or other personal interest of any nature or kind in any product, service and/or company that could be construed as influencing the position presented in, or the review of, the manuscript "Failure characteristics and fracture mechanism of overburden rock induced by mining: A case study in a China coal mine."
Additional information
Publisher's note.
Springer Nature remains neutral with regard to jurisdictional claims in published maps and institutional affiliations.
Rights and permissions
Open Access This article is licensed under a Creative Commons Attribution 4.0 International License, which permits use, sharing, adaptation, distribution and reproduction in any medium or format, as long as you give appropriate credit to the original author(s) and the source, provide a link to the Creative Commons licence, and indicate if changes were made. The images or other third party material in this article are included in the article's Creative Commons licence, unless indicated otherwise in a credit line to the material. If material is not included in the article's Creative Commons licence and your intended use is not permitted by statutory regulation or exceeds the permitted use, you will need to obtain permission directly from the copyright holder. To view a copy of this licence, visit http://creativecommons.org/licenses/by/4.0/ .
Reprints and permissions
About this article
Li, J., Zhang, M., Wang, C. et al. Failure characteristics and fracture mechanism of overburden rock induced by mining: A case study in China. Int J Coal Sci Technol 11 , 44 (2024). https://doi.org/10.1007/s40789-024-00693-x
Download citation
Received : 09 July 2023
Revised : 01 August 2023
Accepted : 16 April 2024
Published : 31 May 2024
DOI : https://doi.org/10.1007/s40789-024-00693-x
Share this article
Anyone you share the following link with will be able to read this content:
Sorry, a shareable link is not currently available for this article.
Provided by the Springer Nature SharedIt content-sharing initiative
- Fracture development characteristics
- Similar simulation experiment
- Layer porosity
- Discrete element numerical simulation
Advertisement
- Find a journal
- Publish with us
- Track your research
What are you looking for?
Sand in Newport Beach was replenished in 2023. (Photo/iStock)
Beach erosion will make Southern California coastal living five times more expensive by 2050, USC study predicts
The region’s sandy coastlines are vanishing at an alarming rate. It’s a warning sign for coastal communities worldwide, USC research suggests.
Rising sea levels and urban development are accelerating coastal erosion at an alarming rate in Southern California with significant ripple effects on the region’s economy, a USC study reveals.
The study, published in Communications Earth & Environment , predicts that Southern California’s coastal living costs will surge fivefold by 2050 as a direct result of beach erosion. This erosion will require more frequent and costly beach nourishment projects to maintain the state’s treasured shorelines, consequently driving up the cost of living along the coast.
“Our study presents compelling evidence of the rapid deterioration of Southern California’s coastal landscapes,” said Essam Heggy , a geoscientist in the Ming Hsieh Department of Electrical and Computer Engineering/Electrophysics at the USC Viterbi School of Engineering and the study’s corresponding author.
“The challenges facing Southern California mirror a growing threat shared by coastal communities worldwide. The environmental and economic implications of coastal erosion reach far beyond California’s shores and demand interdisciplinary, global solutions,” he said.
Coastal erosion: Cost of living sure to surge as sandy beaches disappear
To predict future changes along California’s sandy coastlines, the researchers focused on the Gulf of Santa Catalina, which stretches over 150 miles from the Palos Verdes Peninsula in Los Angeles County to the northern tip of Baja California in Mexico.
They used a combination of historical and recent satellite images as well as advanced algorithms to analyze coastline movement and predict future erosion based on different trends and environmental factors.
The study predicts a tripling of erosion rates by 2050, increasing from an average of 1.45 meters per year to 3.18 meters by 2100. Consequently, the annual sand requirement for beach nourishment could triple by 2050, with costs rising fivefold due to the global increase in sand prices. This will exacerbate economic and logistical pressures on coastal communities.
Beach nourishment is adding sand to an eroded beach to rebuild it and create a wider barrier against waves and storms.
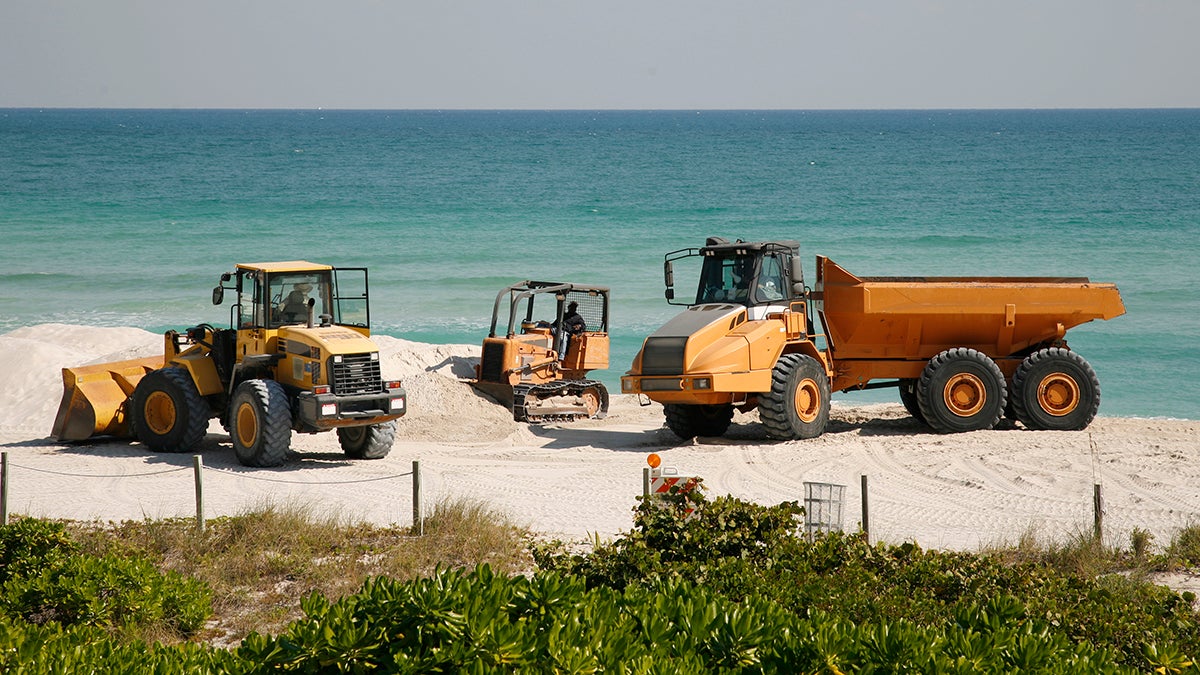
“Our investigation suggests that coastal problems start inland due to the rapid growth of cities along the coast, which compromise inland sediment replenishment of sandy beaches,” said Heggy, whose research focuses on understanding water evolution in Earth’s arid environments.
“As our beaches shrink, the cost of maintaining them will rise. Finding innovative solutions is key to securing a sustainable future for our shores and local economies,” he said.
Coastal erosion in California: A case study for a global problem
Coastal cities in Southern California and those in North Africa bordering the Mediterranean Sea face a common challenge: a semi-arid climate year-round coupled with the growing threats of rising sea levels and eroding shorelines.
A significant portion of Earth’s landmass, roughly 41%, falls under arid or semi-arid classifications, and these areas support over a third of the global population.
To understand this global challenge, the researchers focused on two specific locations: Corona del Mar in Orange County, Calif. — an example of the typical Southern California coastline — and Hammamet North Beach in Tunisia. Both are densely populated and share similar climates, prone to increasing droughts, flash floods and unpredictable rainfall patterns. These characteristics mirror the challenges faced by countless coastal communities worldwide.
The findings showed that the average rate of shoreline retreat in these areas varies. In Southern California, beaches are receding between 0.75 and 1.24 meters per year. In Hammamet North Beach, the retreat rate ranges from 0.21 to about 4.49 meters annually.
“While beach nourishment can temporarily combat erosion, however, it presents significant challenges for developing countries,” said Oula Amrouni, a sedimentologist at the National Institute of Marine Sciences and Technologies at the University of Carthage, Tunis, Tunisia, and one of the study’s co-authors. “The high cost of acquiring the right sand, with the specific grain size, quality and composition, and the technical complexity of extracting and laying it are major hurdles. Additionally, worsening erosion in previously stable areas compels more frequent nourishment projects, straining already limited budgets and leading to unplanned expenditures for many communities.”
About the study: Co-authors of the study include Oula Amrouni and Abderraouf Hzami of the National Institute of Marine Sciences and Technologies at the University of Carthage, Tunis, Tunisia.
This research is supported by the Arid Climates and Water Research Center at USC under contract from the NASA Jet Propulsion Laboratory (AWD#00630), the USC Zumberge Research and Innovation Fund, and the USC Sea Grant.
Related Articles
How the usc stem cell master’s program changed the lives of 10 alumni, usc medical school dean appointed to california institute of regenerative medicine board, optimizing earthquake data flow allows scientific research on ‘the big one’.
- Open access
- Published: 24 May 2024
Integration of case-based learning and three-dimensional printing for tetralogy of fallot instruction in clinical medical undergraduates: a randomized controlled trial
- Jian Zhao 1 na1 ,
- Xin Gong 1 na1 ,
- Jian Ding 1 ,
- Kepin Xiong 2 ,
- Kangle Zhuang 3 ,
- Rui Huang 1 ,
- Shu Li 4 &
- Huachun Miao 1
BMC Medical Education volume 24 , Article number: 571 ( 2024 ) Cite this article
233 Accesses
Metrics details
Case-based learning (CBL) methods have gained prominence in medical education, proving especially effective for preclinical training in undergraduate medical education. Tetralogy of Fallot (TOF) is a congenital heart disease characterized by four malformations, presenting a challenge in medical education due to the complexity of its anatomical pathology. Three-dimensional printing (3DP), generating physical replicas from data, offers a valuable tool for illustrating intricate anatomical structures and spatial relationships in the classroom. This study explores the integration of 3DP with CBL teaching for clinical medical undergraduates.
Sixty senior clinical medical undergraduates were randomly assigned to the CBL group and the CBL-3DP group. Computed tomography imaging data from a typical TOF case were exported, processed, and utilized to create four TOF models with a color 3D printer. The CBL group employed CBL teaching methods, while the CBL-3DP group combined CBL with 3D-printed models. Post-class exams and questionnaires assessed the teaching effectiveness of both groups.
The CBL-3DP group exhibited improved performance in post-class examinations, particularly in pathological anatomy and TOF imaging data analysis ( P < 0.05). Questionnaire responses from the CBL-3DP group indicated enhanced satisfaction with teaching mode, promotion of diagnostic skills, bolstering of self-assurance in managing TOF cases, and cultivation of critical thinking and clinical reasoning abilities ( P < 0.05). These findings underscore the potential of 3D printed models to augment the effectiveness of CBL, aiding students in mastering instructional content and bolstering their interest and self-confidence in learning.
The fusion of CBL with 3D printing models is feasible and effective in TOF instruction to clinical medical undergraduates, and worthy of popularization and application in medical education, especially for courses involving intricate anatomical components.
Peer Review reports
Tetralogy of Fallot (TOF) is the most common cyanotic congenital heart disease(CHD) [ 1 ]. Characterized by four structural anomalies: ventricular septal defect (VSD), pulmonary stenosis (PS), right ventricular hypertrophy (RVH), and overriding aorta (OA), TOF is a focal point and challenge in medical education. Understanding anatomical spatial structures is pivotal for learning and mastering TOF [ 2 ]. Given the constraints of course duration, medical school educators aim to provide students with a comprehensive and intuitive understanding of the disease within a limited timeframe [ 3 ].
The case-based learning (CBL) teaching model incorporates a case-based instructional approach that emphasizes typical clinical cases as a guide in student-centered and teacher-facilitated group discussions [ 4 ]. The CBL instructional methods have garnered widespread attention in medical education as they are particularly appropriate for preclinical training in undergraduate medical education [ 5 , 6 ]. The collection of case data, including medical records and examination results, is essential for case construction [ 7 ]. The anatomical and hemodynamic consequences of TOF can be determined using ultrasonography, computed tomography (CT), and magnetic resonance imaging techniques. However, understanding the anatomical structures from imaging data is a slow and challenging psychological reconstruction process for undergraduate medical students [ 8 ]. Three-dimensional (3D) visualization is valuable for depicting anatomical structures [ 9 ]. 3D printing (3DP), which creates physical replicas based on data, facilitates the demonstration of complex anatomical structures and spatial relationships in the classroom [ 10 ].
During the classroom session, 3D-printed models offer a convenient means for hands-on demonstration and communication, similar to facing a patient, enhancing the efficiency and specificity of intra-team communication and discussion [ 11 ]. In this study, we printed TOF models based on case imaging data, integrated them into CBL teaching, and assessed the effectiveness of classroom instruction.
Research participants
The study employed a prospective, randomized controlled design which received approval from the institutional ethics committee. Senior undergraduate students majoring in clinical medicine at Wannan Medical College were recruited for participation based on predefined inclusion criteria. The researchers implemented recruitment according to the recruitment criteria by contacting the class leaders of the target classes they had previously taught. Notably, these students were in their third year of medical education, with anticipation of progressing to clinical courses in the fourth year, encompassing Internal Medicine, Surgery, Obstetrics, Gynecology, and Pediatrics. Inclusion criteria for participants encompassed the following: (1) proficient communication and comprehension abilities, (2) consistent attendance without absenteeism or truancy, (3) absence of failing grades in prior examinations, and (4) capability to conscientiously fulfill assigned learning tasks. Exclusion criteria were (1) absence from lectures, (2) failure to complete pre-and post-tests, and (3) inadequate completion of questionnaires. For their participation in the study, Students were provided access to the e-book “Localized Anatomy,” authored by the investigators, as an incentive for their participation. Voluntary and anonymous participation was emphasized, with participants retaining the right to withdraw from the study at any time without providing a reason.
The study was conducted between May 1st, 2023, and June 30, 2023, from recruitment to completion of data collection. Drawing upon insights gained from a previous analogous investigation which yielded an effect size of 0.95 [ 10 ]. Sample size was computed, guided by a statistical consultant, with the aim of 0.85 power value, predicated on an effect size of 0.8 and a margin of error set at 0.05. A minimum of 30 participants per group was calculated using G*Power software (latest ver. 3.1.9.7; Heinrich-Heine-Universität Düsseldorf, Düsseldorf, Germany), resulting in the recruitment of a total of 60 undergraduate students. Each participant was assigned an identification number, with codes placed in boxes. Codes drawn from the boxes determined allocation to either the CBL group or the CBL-3DP group. Subsequently, participants were randomly assigned to either the CBL group, receiving instruction utilizing the CBL methodology, or the CBL-3DP group, which received instruction integrating both CBL and 3D Printed models.
Printing of TOF models
Figure 1 A shows the printing flowchart of the TOF models. A typical TOF case was collected from the Yijishan Hospital of Wannan Medical College. The CT angiography imaging data of the case was exported. Mimics Research 20.0 software (Mimics Innovation Suite version 20, Materialize, Belgium) was used for data processing. The cardiovascular module of the CT-Heart tool was employed to adjust the threshold range, independently obtain the cardiac chambers and vessels, post-process the chambers and vessels to generate a hollow blood pool, and merge it with the myocardial volume to construct a complete heart model. The file was imported into Magics 24.0 software (version 24.0; Materialize, Belgium) for correction using the Shell tool page. After repairs, the model entered the smoothing page, where tools such as triangular surface simplification, local smoothing, refinement and smoothing, subdivision of components, and mesh painting were utilized to achieve varying degrees of smoothness. Finally, optimized data were obtained and exported as stereolithography (STL) files. An experienced cardiothoracic surgeon validated the anatomical accuracy of the digital model.
The STL files were imported into a 3D printer (J401Pro; Sailner 3D Technology, China) for model printing. This printer can produce full-color medical models using different materials. The models were fabricated using two distinct materials: rigid and flexible. Both materials are suitable for the observational discussion of the teaching objectives outlined in our study. From the perspective of observing pathological changes in the TOF, there is no significant difference between the two materials.
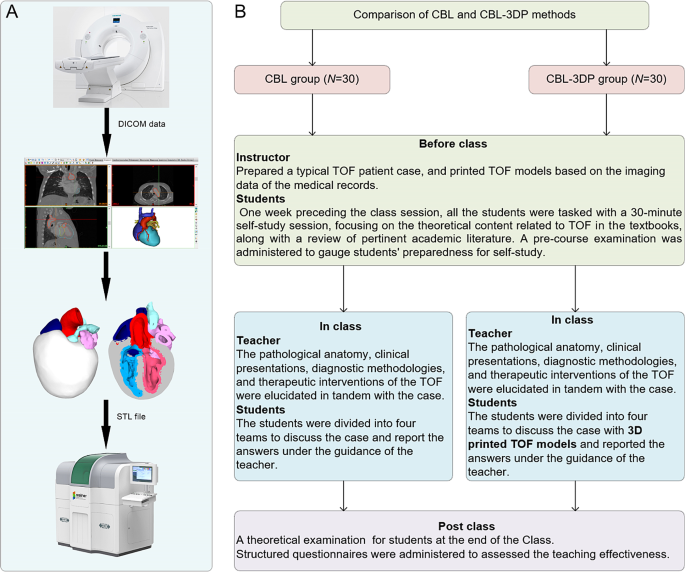
Experimental flow chart of this study. A TOF model printing flow chart. B The instructional framework
Teaching implementation
Figure 1 B illustrates the instructional framework employed in this study. One week preceding the class session, all the students were tasked with a 30-minute self-study session, focusing on the theoretical content related to TOF as outlined in the Pediatrics and Surgery textbooks, along with a review of pertinent academic literature. Both groups received co-supervision from two basic medicine lecturers boasting over a decade of teaching experience, alongside a senior cardiothoracic surgeon. Teaching conditions remained consistent across groups, encompassing uniform assessment criteria and adherence to predefined teaching time frames, all conducted in a Project-Based Learning (PBL) classroom at Wannan Medical College. Additionally, a pre-course examination was administered to gauge students’ preparedness for self-study.
In adherence to the curriculum guidelines, the teaching objectives aimed to empower students to master TOF’s clinical manifestations, diagnostic modalities, and differential diagnoses, while acquainting them with treatment principles and surgical methodologies. Additionally, the objectives sought to cultivate students’ clinical reasoning abilities and problem-solving skills. the duration of instruction for the TOF theory session was standardized to 25 min. The didactic content was integrated with the TOF case study to construct a coherent pedagogical structure.
During the instructional session, both groups underwent teaching utilizing the CBL methodology. Clinical manifestations and case details of TOF cases were presented to stimulate students’ interest and curiosity. Subsequently, the theory of TOF, including its etiology, pathogenesis, pathologic anatomy, clinical manifestations, diagnostic methods, and therapeutic interventions, was briefly elucidated. Emphasis was then placed on the case, wherein selected typical TOF cases were explained, guiding students in analysis and discussion. Students were organized into four teams under the instructors’ supervision, fostering cooperative learning and communication, thereby deepening their understanding of the disease through continuous inquiry and exploration (Fig. 2 L). In the routinely equipped PBL classroom with standard heart models (Fig. 2 J, K), all students had prior exposure to human anatomy and were familiar with these models. Both groups were provided with four standard heart models for reference, while the CBL-3DP group received additional four 3D-printed models depicting TOF anomalies, enriching their learning experience (Fig. 2 D, G). After the lesson, summarization, and feedback sessions were conducted to consolidate group discussions’ outcomes, evaluate teaching effectiveness, and assess learning outcomes.
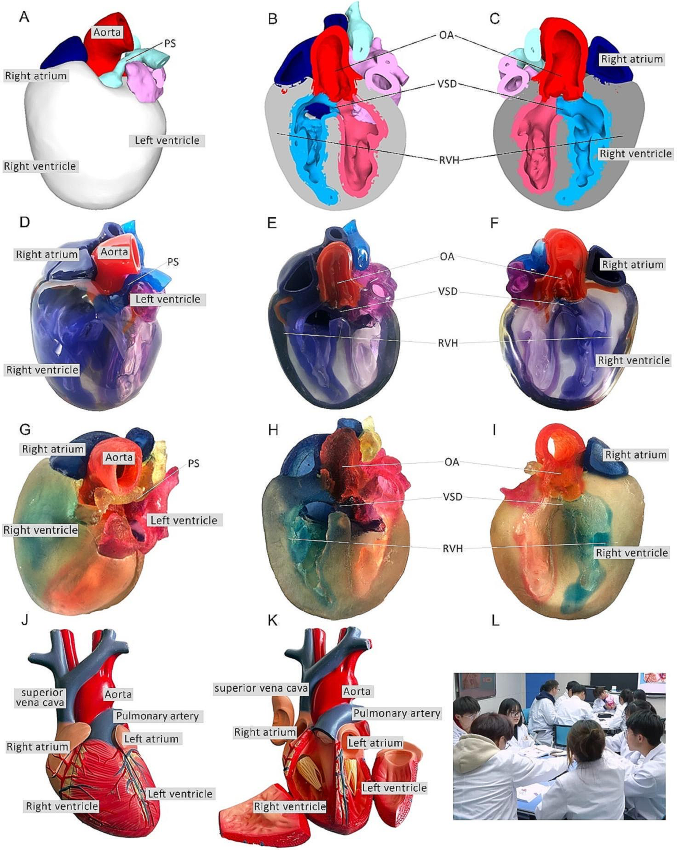
Heart models utilized in instructional sessions. A External perspective of 3D digital models. B, C Cross-sectional views following trans-septal sagittal dissection of the 3D digital model (PS: Pulmonary Stenosis; OA: Overriding Aorta; VSD: Ventricular Septal Defect; RVH: Right Ventricular Hypertrophy). D External depiction of rigid 3D printed model. E, F Sagittal sections of the rigid 3D printed model. G External portrayal of flexible 3D printed model. H, I Sagittal sections of the flexible 3D printed model. J, K The normal heart model employed in the instruction of the CBL group. L Ongoing classroom session
Teaching effectiveness assessment
Following the instructional session, participants from the two groups underwent a theoretical examination to assess their comprehension of the taught material. This assessment covered domains such as pathological anatomy, clinical manifestations, imaging data interpretation, diagnosis, and treatment relevant to TOF. Additionally, structured questionnaires were administered to evaluate the efficacy of the pedagogical approach employed. The questionnaire consisted of six questions designed to gauge participants’ understanding of the teaching content, enhancement of diagnostic skills, cultivation of critical thinking and clinical reasoning abilities, bolstering of confidence in managing TOF cases, satisfaction with the teaching mode, and satisfaction with the CBL methodology.
The questionnaire employed a 5-point Likert scale to gauge responses, with 5 indicating “strongly satisfied/agree,” 4 for “satisfied/agree,” 3 denoting “neutral,” 2 reflecting “dissatisfied/disagree,” and 1 indicating “strongly dissatisfied/disagree.” It comprised six questions, with the initial two probing participants’ knowledge acquisition, questions 3 and 4 exploring satisfaction regarding enhanced competence, and the final two assessing satisfaction with teaching methods and modes. Additionally, participants were encouraged to provide suggestions at the end of the questionnaire. To ensure the questionnaire’s validity, five esteemed lecturers in basic medical sciences with more than 10 years of experience verified its content and assessed its Content Validity Ratio and Content Validity Index to ensure alignment with the study’s objectives.
Statistical analysis
Statistical analyses were conducted utilizing GraphPad Prism 9.0 software. Aggregate score data for both groups were presented as mean ± standard deviation (x ± s). The gender comparisons were analyzed with the chi-square (χ2) test, while the other variables were compared using the Mann-Whitney U test. The threshold for determining statistical significance was set at P < 0.05.
Three-dimensional printing models
After configuring the structural colors of each component (Fig. 2 A, B, C), we printed four color TOF models using both rigid and flexible materials, resulting in four life-sized TOF models. Two color TOF models were created using rigid materials (Fig. 2 D, E, F). These models, exhibiting resistance to deformation, and with a firm texture, smooth and glossy surface, and good transparency, allowing visibility of the internal structures, were deemed conducive to teaching and observation. We also fabricated two color TOF models using flexible materials (Fig. 2 G, H, I), characterized by soft texture, opacity, and deformability, allowing for easy manipulation and cutting. It has potential utility beyond observational purposes. It can serve as a valuable tool for simulating surgical interventions and may be employed to create tomographic anatomical specimens. In this study, both material models were suitable for observation in the classroom. The participants were able to discern the four pathological changes characteristic of TOF from surface examination or cross-sectional analysis.
Baseline characteristics of the students
In total, 60 students were included in this study. The CBL group comprised 30 students (14 males and 16 females), with an average age of (21.20 ± 0.76) years. The CBL-3DP group consisted of 30 students (17 males and 13 females) with an average age of 20.96 years. All the students completed the study procedures. There were no significant differences in age, sex ratio, or pre-class exam scores between the two groups ( P > 0.05), indicating that the baseline scores between the two groups were comparable (Table 1 ).
Theoretical examination results
All students completed the research procedures as planned. The post-class theoretical examination encompassed assessment of pathological anatomy, clinical presentations, imaging data interpretation, diagnosis, and treatment pertinent to TOF. Notably, no statistically significant disparities were observed in the scores on clinical manifestations, diagnosis and treatment components between the cohorts, as delineated in Table 2 . Conversely, discernible distinctions were evident whereby the CBL-3DP group outperformed the CBL group notably in pathological anatomy, imaging data interpretation, and overall aggregate scores ( P < 0.05).
Results of the questionnaires
All the 60 participants submitted the questionnaire. Comparing the CBL and CBL-3DP groups, the scores from the CBL-3DP group showed significant improvements in many areas. This included satisfaction with the teaching mode, promotion of diagnostic skills, bolstering of self-assurance in managing TOF cases, and cultivation of critical thinking and clinical reasoning abilities (Fig. 3 B, C, D, E). All of which improved significantly ( P < 0.05 for the first aspects and P < 0.01 for the rest). However, the two groups were not comparable ( P > 0.05) in terms of understanding of the teaching content and Satisfaction with the CBL methodology (Fig. 3 A, F).
Upon completion of the questionnaires, participants were invited to proffer recommendations. Notably, in the CBL group, seven students expressed challenges in comprehending TOF and indicated a need for additional time for consolidation to enhance understanding. Conversely, within the CBL-3DP group, twelve students advocated for the augmentation of model repertoire and the expansion of disease-related data collection to bolster pedagogical efficacy across other didactic domains.
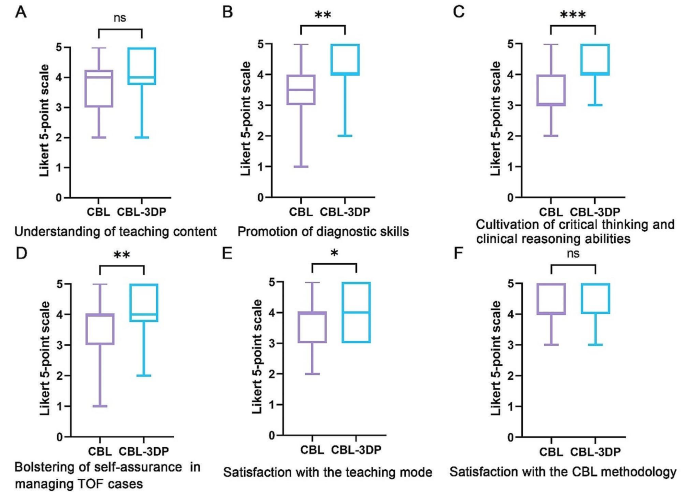
Five-point Likert scores of students’ attitudes in CBL ( n = 30) and CBL-3DP ( n = 30) groups. A Understanding of teaching content. B Promotion of diagnostic skills. C Cultivation of critical thinking and clinical reasoning abilities. D Bolstering of self-assurance in managing TOF cases. E Satisfaction with the teaching mode. F Satisfaction with the CBL methodology. ns No significant difference, * p < 0.05, ** p < 0.01, *** p < 0.001
TOF presents a significant challenge in clinical practice, necessitating a comprehensive understanding for effective diagnosis and treatment [ 12 ]. Traditional teaching methods in medical schools have relied on conventional resources such as textbooks, 2D illustrations, cadaver dissections, and radiographic materials to impart knowledge about complex conditions like TOF [ 13 ]. However, the limitations of these methods in fully engaging students and bridging the gap between theoretical knowledge and practical application have prompted a need for innovative instructional approaches.
CBL has emerged as a valuable tool in medical education, offering students opportunities to engage with authentic clinical cases through group discussions and inquiry-based learning [ 14 ]. By actively involving students in problem-solving and decision-making processes, CBL facilitates the application of theoretical knowledge to real-world scenarios, thus better-preparing students for future clinical practice [ 15 ]. Our investigation revealed that both groups of students exhibited comparable levels of satisfaction with the CBL methodology, devoid of discernible disparities.
CHD presents a formidable challenge due to the intricate nature of anatomical anomalies, the diverse spectrum of conditions, and individual variations [ 16 ]. Utilizing 3D-printed physical models, derived from patient imaging data, can significantly enhance comprehension of complex anatomical structures [ 17 ]. These models have proven invaluable in guiding surgical planning, providing training for junior or inexperienced pediatric residents, and educating healthcare professionals and parents of patients [ 18 ]. Studies indicate that as much as 50% of pediatric surgical decisions can be influenced by the insights gained from 3D printed models [ 19 ]. By providing tangible, anatomically accurate models, 3D printing offers a unique opportunity for people to visualize complex structures and enhance their understanding of anatomical intricacies. Our study utilized full-color, to-scale 3D printed models to illustrate the structural abnormalities associated with TOF, thereby enriching classroom sessions and facilitating a deeper comprehension of the condition.
Comparative analysis between the CBL-3DP group and the CBL group revealed significant improvements in post-test performance, particularly in pathological anatomy and imaging data interpretation. Additionally, questionnaire responses indicated higher levels of satisfaction and confidence among students in the CBL-3DP group, highlighting the positive impact of incorporating 3D printed models into the learning environment, improving the effectiveness of CBL classroom instruction. Despite the merits, our study has limitations. Primarily, participants were exclusively drawn from the same grade level within a single college, possibly engendering bias owing to shared learning backgrounds. Future research could further strengthen these findings by expanding the sample size and including long-term follow-up to assess the retention of knowledge and skills. Additionally, the influence of the 3D models depicting a normal heart on the learning process and its potential to introduce bias into the results warrants consideration, highlighting a need for scrutiny in subsequent studies.
As medical science continues to advance, the need for effective teaching methods becomes increasingly paramount. Our study underscores the potential of combining active learning approaches like CBL with innovative technologies such as 3D printing to enhance teaching effectiveness, improve knowledge acquisition, and foster students’ confidence and enthusiasm in pursuing clinical careers. Moving forward, further research and integration of such methodologies are essential for meeting the evolving demands of medical education, especially in areas involving complex anatomical understanding.
Conclusions
Integrating 3D-printed models with the CBL method is feasible and effective in TOF instruction. The demonstrated success of this method warrants broad implementation in medical education, particularly for complex anatomical topics.
Data availability
All data supporting the conclusions of this research are available upon reasonable request from the corresponding author.
Apitz C, Webb GD, Redington AN. Tetralogy of Fallot. Lancet. 2009;374:1462–71.
Article Google Scholar
Ghosh RM, Jolley MA, Mascio CE, Chen JM, Fuller S, Rome JJ, et al. Clinical 3D modeling to guide pediatric cardiothoracic surgery and intervention using 3D printed anatomic models, computer aided design and virtual reality. 3D Print Med. 2022;8:11.
Chakrabarti R, Wardle K, Wright T, Bennie T, Gishen F. Approaching an undergraduate medical curriculum map: challenges and expectations. BMC Med Educ. 2021;21:341.
Donkin R, Yule H, Fyfe T. Online case-based learning in medical education: a scoping review. BMC Med Educ. 2023;23:564.
Novack JP. Designing cases for case-based immunology teaching in large medical school classes. Front Immunol. 2020;11:995.
Chen HC, Van Den Broek WES, Ten Cate O. The case for use of entrustable professional activities in undergraduate medical education. Acad Med. 2015;90:431–6.
Wang M, Sun Z, Jia M, Wang Y, Wang H, Zhu X, et al. Intelligent virtual case learning system based on real medical records and natural language processing. BMC Med Inf Decis Mak. 2022;22:60.
Yoo S-J, Thabit O, Kim EK, Ide H, Yim D, Dragulescu A, et al. 3D printing in medicine of congenital heart diseases. 3D Print Med. 2015;2:3.
Yammine K, Violato C. A meta-analysis of the educational effectiveness of three-dimensional visualization technologies in teaching anatomy. Anat Sci Educ. 2015;8:525–38.
Miao H, Ding J, Gong X, Zhao J, Li H, Xiong K, et al. Application of 3D-printed pulmonary segment specimens in experimental teaching of sectional anatomy. BMC Surg. 2023;23:109.
Sun Z, Wong YH, Yeong CH. Patient-specific 3D-printed low-cost models in medical education and clinical practice. Micromachines (Basel). 2023;14:464.
Downing TE, Kim YY. Tetralogy of Fallot: general principles of management. Cardiol Clin. 2015;33:531–41. vii–viii.
Jia X, Zeng W, Zhang Q. Combined administration of problem- and lecture-based learning teaching models in medical education in China: a meta-analysis of randomized controlled trials. Med (Baltim). 2018;97:e11366.
McLean SF. Case-based learning and its application in medical and health-care fields: a review of worldwide literature. J Med Educ Curric Dev. 2016;3:JMECD.S20377.
Zeng N, Lu H, Li S, Yang Q, Liu F, Pan H, et al. Application of the combination of CBL teaching method and SEGUE framework to improve the doctor-patient communication skills of resident physicians in otolaryngology department. Bmc Med Educ. 2024;24:201.
Sun Z. Patient-specific 3D-printed models in pediatric congenital heart disease. Children. 2023;10:319.
Meyer-Szary J, Luis MS, Mikulski S, Patel A, Schulz F, Tretiakow D, et al. The role of 3D printing in planning complex medical procedures and training of medical professionals—cross-sectional multispecialty review. IJERPH. 2022;19:3331.
Sun Z, Wee C. 3D printed models in cardiovascular disease: an exciting future to deliver personalized medicine. Micromachines-basel. 2022;13:1575.
Valverde I, Gomez-Ciriza G, Hussain T, Suarez-Mejias C, Velasco-Forte MN, Byrne N, et al. Three-dimensional printed models for surgical planning of complex congenital heart defects: an international multicentre study. Eur J Cardio-thorac. 2017;52:1139–48.
Download references
Acknowledgements
We extend our sincere appreciation to the instructors and students whose invaluable participated in this study.
This paper received support from the Education Department of Anhui Province, China (Grant Numbers 2022jyxm1693, 2022jyxm1694, 2022xskc103, 2018jyxm1280).
Author information
Jian Zhao and Xin Gong are joint first authors.
Authors and Affiliations
Department of Human Anatomy, Wannan Medical College, No.22 West Wenchang Road, Wuhu, 241002, China
Jian Zhao, Xin Gong, Jian Ding, Rui Huang & Huachun Miao
Department of Cardio-Thoracic Surgery, Yijishan Hospital of Wannan Medical College, Wuhu, China
Kepin Xiong
Zhuhai Sailner 3D Technology Co., Ltd., Zhuhai, China
Kangle Zhuang
School of Basic Medical Sciences, Wannan Medical College, Wuhu, China
You can also search for this author in PubMed Google Scholar
Contributions
Jian Zhao and Huachun Miao designed the research. Jian Zhao, Xin Gong, Jian Ding, Kepin Xiong designed the tests and questionnaires. Kangle Zhuang processed the imaging data and printed the models. Xing Gong and Kepin Xiong implemented the teaching. Jian Zhao and Rui Huang collected the data and performed the statistical analysis. Jian Zhao and Huachun Miao prepared the manuscript. Shu Li and Huachun Miao revised the manuscript. Shu Li provided the Funding acquisition. All authors reviewed and approved the final manuscript.
Corresponding authors
Correspondence to Shu Li or Huachun Miao .
Ethics declarations
Ethics approval and consent to participate.
This investigation received ethical approval from the Ethical Committee of School of Basic Medical Sciences, Wannan Medical College (ECBMSWMC2022-1-12). All methodologies adhered strictly to established protocols and guidelines. Written informed consent was obtained from the study participants to take part in the study.
Consent for publication
Written informed consent was obtained from the individuals for the publication of any potentially identifiable images or data included in this article.
Competing interests
The authors declare no competing interests.
Additional information
Publisher’s note.
Springer Nature remains neutral with regard to jurisdictional claims in published maps and institutional affiliations.
Electronic supplementary material
Below is the link to the electronic supplementary material.
Supplementary Material 1
Supplementary material 2, supplementary material 3, rights and permissions.
Open Access This article is licensed under a Creative Commons Attribution 4.0 International License, which permits use, sharing, adaptation, distribution and reproduction in any medium or format, as long as you give appropriate credit to the original author(s) and the source, provide a link to the Creative Commons licence, and indicate if changes were made. The images or other third party material in this article are included in the article’s Creative Commons licence, unless indicated otherwise in a credit line to the material. If material is not included in the article’s Creative Commons licence and your intended use is not permitted by statutory regulation or exceeds the permitted use, you will need to obtain permission directly from the copyright holder. To view a copy of this licence, visit http://creativecommons.org/licenses/by/4.0/ . The Creative Commons Public Domain Dedication waiver ( http://creativecommons.org/publicdomain/zero/1.0/ ) applies to the data made available in this article, unless otherwise stated in a credit line to the data.
Reprints and permissions
About this article
Cite this article.
Zhao, J., Gong, X., Ding, J. et al. Integration of case-based learning and three-dimensional printing for tetralogy of fallot instruction in clinical medical undergraduates: a randomized controlled trial. BMC Med Educ 24 , 571 (2024). https://doi.org/10.1186/s12909-024-05583-z
Download citation
Received : 03 March 2024
Accepted : 21 May 2024
Published : 24 May 2024
DOI : https://doi.org/10.1186/s12909-024-05583-z
Share this article
Anyone you share the following link with will be able to read this content:
Sorry, a shareable link is not currently available for this article.
Provided by the Springer Nature SharedIt content-sharing initiative
- Medical education
- Case-based learning
- 3D printing
- Tetralogy of fallot
- Medical undergraduates
BMC Medical Education
ISSN: 1472-6920
- Submission enquiries: [email protected]
- General enquiries: [email protected]
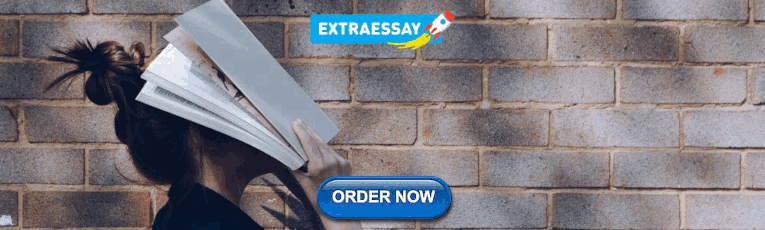
COMMENTS
Strategy: In the near-term, transform DOT's data release posture and in the long-term, sustain openness. Policy: Provide guidelines for identifying datasets and prioritizing them for release. Institute standing policies for approving, publishing, and managing releases. Inventory: Build list of source information systems using budget exhibits.
Nowadays detailed transportation data can be accessed and shared at unprecedented scales, which unveils new transportation paradigms and opportunities related to smart mobility. ... Their effectiveness is demonstrated through a traffic engineering case study, where route planning services are designed according to the proposed approach and then ...
Streamlining a Corridor Study With On-Demand Traffic Data. To understand existing conditions on PA Route 28, local agencies needed to undertake a rigorous corridor study. On-demand analytics from StreetLight helped stretch their budget, streamline data collection, and widen the scope of analysis. READ CASE STUDY.
Through transportation data analytics, organizations can gain insights into capacity constraints within their networks, enabling them to optimize routes and resources efficiently. By analyzing historical data and real-time information, companies can proactively address bottlenecks, minimize delays, and ensure timely delivery of goods.
2022. Data Governance to Data-Driven Safety Analysis: Florida's SAFE STRIDES 2 Zero. This case study documents how the Florida Department of Transportation's (FDOT's) multi-year data governance effort has enabled the Agency to embark upon a robust program of safety data collection, integration, and analysis.
Transportation systems play a major role in modern urban contexts, where citizens are expected to travel in order to engage in social and economic activities. Modern transportation systems incorporate technologies that generate huge volumes of data, which can be processed to extract valuable mobility information. This article describes a proposal for studying public transportation systems ...
The case study covers a global supply chain involving actual transport and logistics services managed by a large, international forwarding company. ... Applications of anomaly detection on transportation data. In recent years, Intelligent Transportation Systems (ITS) face the problem of detecting anomalies in vehicles movement. ...
This research paper explores the utilization of AI, machine learning, and data analytics for smart transportation planning to achieve sustainable urban mobility. The study aims to address two key research questions: (1) How can these advanced technologies be leveraged to optimize urban transportation systems? (2) What are the potential benefits associated with their implementation? The ...
This paper presents data, methods, and models that are essential for intelligent planning of transportation. This paper describes in particular, the current data sources for gathering information to control or forecast traffic, and connected vehicles (CVs) that bring smart and green transportation to modern life.
Data Management . Case Studies of Select Transportation Agencies . July 2018. Prepared for: Office of Planning . Federal Highway Administration . U.S. Department of Transportation . 2 ... The GIS in Transportation case study series is a means of conveying best practices and resources, and is informed directly by interviews with State DOT ...
One is to process heterogeneous transportation data collected from different types of traffic sensors and the other is to reduce the high computation intensity for processing massive transportation data. To overcome both challenges, this paper proposes an approach using parallelized fusion on multisensor transportation data.
The Case Studies of Multimodal Transportation Partnerships was performed under NCHRP Project 8-32(4) by. Kimley-Horn and Associates, Inc., in association with the management consulting firm Steve A. Martin, Inc., North. Carolina A & T State University, and Mr. Thomas F. Humphrey, P.E.
1. Using existing powers over bus stops, timetables and routes. 2. Integrating the planning of bus services with other modes of transport in the region. 3. Giving cities the power to directly regulate bus services. 4. Providing long-term funding certainty for infrastructure. 5.
The big data concept has been gaining strength over the last few years. With the arise and dissemination of social media and high access easiness to information through applications, there is a necessity for all kinds of service providers to collect and analyze data, improving the quality of their services and products. In this regard, the relevance and coverage of this niche of study are ...
Transportation data in a smart city environment is increasingly becoming available. This data availability allows building smart solutions that are viewed as meaningful by both city residents and city management authorities. ... Data-Driven Solutions to Understand Disruptive Problems in Transportation—The Lisbon Case Study" Energies 14, no ...
Collecting sex-disaggregated transportation data and including intersectional approaches (such as "socioeconomic and demographic indicators") in data analysis (European ... The gendered innovation in this case study is reconceptualizing how data are collected. This case study summarizes research by Inés Sánchez de Madariaga (2009, 2010, 2013a ...
Potential uses of transit smart card registration and transaction data to improve transit planning. Transportation Research Record: Journal of the Transportation Research Board, (1971):119--126, 2006. Google Scholar; R. C. W. Wong, A. Fu, K. Wang, P. S. Yu, and J. Pei. Can the utility of anonymized data be used for privacy breaches.
Recommended for you. Transport for Greater Manchester (TfGM) capitalized on technology modernization to continue providing smart, sustainable, and integrated transportation options for a fast-growing region.
One of the largest logistics platforms in the world, C.H. Robinsons is responsible with over $21 billion in freight, as well as 19 million shipments each year. The company helps keep crucial transportation elements moving in the modern world, but also solves complex logistics problems. During 2020 and 2021, the brand was forced to implement a ...
They present their results and conclusions in a case study where the methodology is evaluated using a traffic simulation model calibrated with data from Germany and applying it to a scenario in China. ... "Short-term Prediction of Freeway Travel Times by Fusing Input-output Vehicle Counts and GPS Tracking Data." Transportation Letters 1-8 ...
The crowdsourced data used in this study were provided by a third-party vendor, and the data agreements do not allow for sharing the data. Acknowledgments The authors would like to acknowledge the Arizona Department of Transportation (ADOT) for graciously sharing the crowdsourced and loop detector data that made this paper possible.
Improvement of residential areas with low accessibility to crucial destinations (e.g., hospitals, schools, markets, and recreation centers) is expected to improve traffic flow and reduce energy consumption and CO 2 emissions. This study intends to evaluate the multi-criteria accessibility measure of residential areas to key destinations in Metro Manila, using the gravity method and applying it ...
Know-How from more than 1,700 supply chain projects and 25+ years of experience. Gain full global network transparency. Achieve cost savings through supply chain optimization. Support for all phases, from conception to implementation into your supply chain. Deep knowledge of all modes of transport.
The Federal Highway Administration's Office of Highway Policy Information (HPPI) explored the original equipment manufacturer (OEM) and the USDOT ITS JPO connected-vehicle (CV) pilot project data. CV data are created for in-situ and real-time vehicle travel usage. However, postevent analyses of CV data offer significant and valuable ...
Comparative results with traditional approaches solely relying on K-means or DBSCAN methods demonstrate that the proposed approach achieves efficient location selection, through multiscale fusion site selection in the study area of 1.5∗1 km, and only 25 electronic fences need to be planned and deployed, covering a total area of 1691.88 square ...
The purpose of this study is to estimate the Origin-Destination Matrix (ODM) of Tehran city which is a large scale network, using three types of field data of count links, partial paths flows, and the difference in incoming and outgoing flows to/from the central traffic area. The data was collected from 482 Automatic Number Plate Recognition cameras on two cordon lines and 1957 inductive ...
4 CASE STUDY 4.1 System data. ... With regard to the transportation network, this paper employs the extended Nguyen-Dupuis network. The node coupling relationship between the distribution network and the transportation network is illustrated in Figure 1. Please note that nodes 2 and 3 of the distribution network are located at the same ...
This study employs similar simulation testing and discrete element simulation coupling to analyze the failure and deformation processes of a model coal seam's roof. The caving area of the overburden rock is divided into three zones: the delamination fracture zone, broken fracture zone, and compaction zone. The caving and fracture zones' heights are approximately 110 m above the coal seam, with ...
The study, published in Communications Earth & Environment, predicts that Southern California's coastal living costs will surge fivefold by 2050 as a direct result of beach erosion. This erosion will require more frequent and costly beach nourishment projects to maintain the state's treasured shorelines, consequently driving up the cost of ...
This study explores the integration of 3DP with CBL teaching for clinical medical undergraduates. Sixty senior clinical medical undergraduates were randomly assigned to the CBL group and the CBL-3DP group. Computed tomography imaging data from a typical TOF case were exported, processed, and utilized to create four TOF models with a color 3D ...