
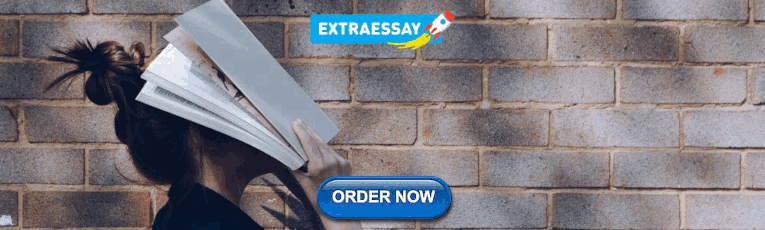
Step by Step Process of How to Solve Statistics Problems
“How to solve statistics problems?” is an obvious question students mostly search over the internet.
For many students, it is like a nightmare to solve statistics problems due to various reasons. In order to solve statistics problems correctly, practice is a primary requirement, and you should know how and where to collect data and analyze and interpret it to draw valuable information.
Putting the right formulas to solve the problems is equally important as collecting the data from authentic and reliable sources. If you collect data from random sources, you can not conclude from that data.
So, if you are one of those who are facing problems when solving statistics problems, we are here to assist you.
In this blog, we will provide you with a step by step process of how to solve statistics problems. We will also cover statistical terms and definitions of statistics.
What is statistics?
Table of Contents
“Statistics is a science, not a branch of mathematics, but use mathematical models as essential tools” John Tukey |
Statistics is considered as the science that deals with methods and tools of collection, analysis, Interpretation and presentation of data. Statistics is majorly used for research and study purposes as through stats we can make significant decisions. It deals with both quantitative and qualitative data and structured and unstructured data.
So everyone is scared of statistics and they always search how to solve statistics problems. The general method of solving statistics problems is to write your question then collect data required for solving such a question and lastly you are required to analyse such data and to draw conclusion.
Statistical and non-statistical problem
Let’s know the difference between a statistical problem and non statistical problem.
Question1. How many states are there in India?
Question 2. In which state girl ratio is maximum in India?
What do you understand from these both questions?
Have you noticed any difference?
Let me explain you-
The major difference between these questions is that Question number 1 is non statistical and question number 2 is statistical.
What makes these problems statistical and non statistical?
Four thing or factors make a problem statistical and non statistical that are given below-
- Way to ask the question
- Role of data and its nature
- Way to examine the data
- Types of interpretation you bring from research
Hence the question first is simple and factual and its answer does not need any type of research and collection of data whereas second question need to collect data from all the states, analyze data, research is required and at last we can conclude that which state have the maximum ratio of girls.
Terminologies used in statistics problems –
There are n number of terminologies which are used in statistics this is why it is said that statistics has its own language which you should command first. So if you are searching for How to solve statistics problems then firstly you have to learn the meaning of basic terms used in Statistics. Following are the most essential terms –
When we solve any statistics problem then we are required to collect data from the people who are affiliated with the given question. So we have to decide whom we want to study. Thus, in statistics, people or individuals you want to study or you are studying are called as population. In short, the group of people whom you are studying is the population.
If you understand the term population then it is very easy to learn samples. The sample is just a subset of the total population. For example your population has 10 individuals then each individual is a sample for your study.
The next term to learn on How to solve statistics problems as the name suggests it is the scope of the study. That is the quantitative characteristics of the population you are studying or testing. For example you want to know how many people use Colgate. Then this question is a parameter. So your population and sample and other required details will be based on such parameters.
Descriptive Statistic
Next terminology to learn in How to solve statistics problems is Descriptive statistics.
When you analyze the data after determining the hypothesis and collection of data then you will get certain results on such study and such result is called descriptive statistics.
Procedure – How to solve statistics problems
Determine your Question
The first step to solve the statistics problem is to decide the problem that is the question or hypothetical test. Unless you know the question you can’t process with other steps because this step will decide the parameter and population for your study. This is why this is the first and foremost step in How to solve statistics problems.
Collection of Data
Next step is to collect the data as per your hypothesis. Here you will decide the population and you can use different methodologies of collection of data like questionnaires OR survey etc. It is also a very important step because you can’t get true and correct results unless you have correct data.
Analysis of data
By now you have collected the required data and also you have your hypothesis so your next step in How to solve statistics problems is to analyse the data accordingly. There are various tools to analyse the data like Microsoft Excel, Python, R, etc. So you must be skilled in data analysis.
Interpretation of data
Next step in How to solve statistics problems is to interpret the data you have collected. Point to note here is always remember your questions while interpreting because data speaks a lot so you have to scrutinize in such a way that you can get desired results. After this step you will get the results of your study so lastly you will have to just present the data. And for presentation also there are n number tools and methods which you can use. So presentation of data shall also be up to mark so that you can analyze the data easily and speedily. You can present the data through pie charts, graphs or tables etc.
Statistical formulas
Statistical problems are solved through statistical formulas so the technique to learn such formulas is to break them down. For example if you are solving mean, median or mode or standard deviation you shall be well versed with these formulas then only you can get correct results.
Let’s take a statistics problem and solve it.
Suppose there are 10 students in a class and we are asked to find out the average weight of students of that class. For this we need to know about the weight of individual students so that we can calculate the average weight of those students.
Average weight- We can calculate average weight with the mean formula
Mean = sum of all terms/ total terms
Hence the average weight of students is 47.8 kg
Mode = the frequent term in the list is known as the median.
In the above question 45 is mode because it is repeated three times.
Median= The central term is known as median. But in this question we have ten terms and we have two middle terms.
Now the mean of these two terms is median. But before that we have to arrange the values in any order.
35 40 43 45 45 45 54 55 56 60
Here 45+45/2= 90/2 = 45 is median
Hence to solve statistics problems you should know these formulas or tactics.
In this competitive world, data analysis is the key stream to earn more profits and to beat the competition. Statistics is used for the same as it is the type of science which deals with data analysis and much more. Many people struggle with How to solve statistics problems so this article is inclined to that only. In case, if you need any help with statistics assignment , then you can get the best help from our statistics assignment helper .
In this blog we have also differentiate between statistical and non statistical questions so that you can better understand what statistics problems require.
What are the types of Statistics?
Statistics is mainly of two types-
Descriptive- It just describe what the data shows
Inferential- It helps in generalization of data and draws valuable conclusions.
What are the components of a statistics problem?
- Ask a question
- Gather Data
- Data analysis
- Interpret Results
Which formulas should we know about how to solve statistics problems?
In statistics, we use numerous formulas to solve the different problems. Statistics problems require simple as well as complex formulas to give answers. We have to use formulas of mean, mode, median, and other probability formulas in statistics.
Similar Articles

13 Best Tips To Write An Assignment
Whenever the new semester starts, you will get a lot of assignment writing tasks. Now you enter the new academic…

How To Do Homework Fast – 11 Tips To Do Homework Fast
Homework is one of the most important parts that have to be done by students. It has been around for…
Leave a Comment Cancel Reply
Your email address will not be published. Required fields are marked *
This site uses Akismet to reduce spam. Learn how your comment data is processed .
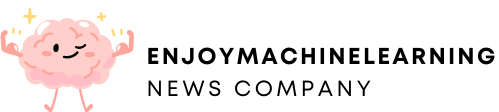
How to Solve Statistical Problems Efficiently [Master Your Data Analysis Skills]
- November 17, 2023
Are you tired of feeling overstimulated by statistical problems? Welcome – you have now found the perfect article.
We understand the frustration that comes with trying to make sense of complex data sets.
Let’s work hand-in-hand to unpack those statistical secrets and find clarity in the numbers.
Do you find yourself stuck, unable to move forward because of statistical roadblocks? We’ve been there too. Our skill in solving statistical problems will help you find the way in through the toughest tough difficulties with confidence. Let’s tackle these problems hand-in-hand and pave the way to success.
As experts in the field, we know what it takes to conquer statistical problems effectively. This article is adjusted to meet your needs and provide you with the solutions you’ve been searching for. Join us on this voyage towards mastering statistics and unpack a world of possibilities.
Key Takeaways
- Data collection is the foundation of statistical analysis and must be accurate.
- Understanding descriptive and inferential statistics is critical for looking at and interpreting data effectively.
- Probability quantifies uncertainty and helps in making smart decisionss during statistical analysis.
- Identifying common statistical roadblocks like misinterpreting data or selecting inappropriate tests is important for effective problem-solving.
- Strategies like understanding the problem, choosing the right tools, and practicing regularly are key to tackling statistical tough difficulties.
- Using tools such as statistical software, graphing calculators, and online resources can aid in solving statistical problems efficiently.
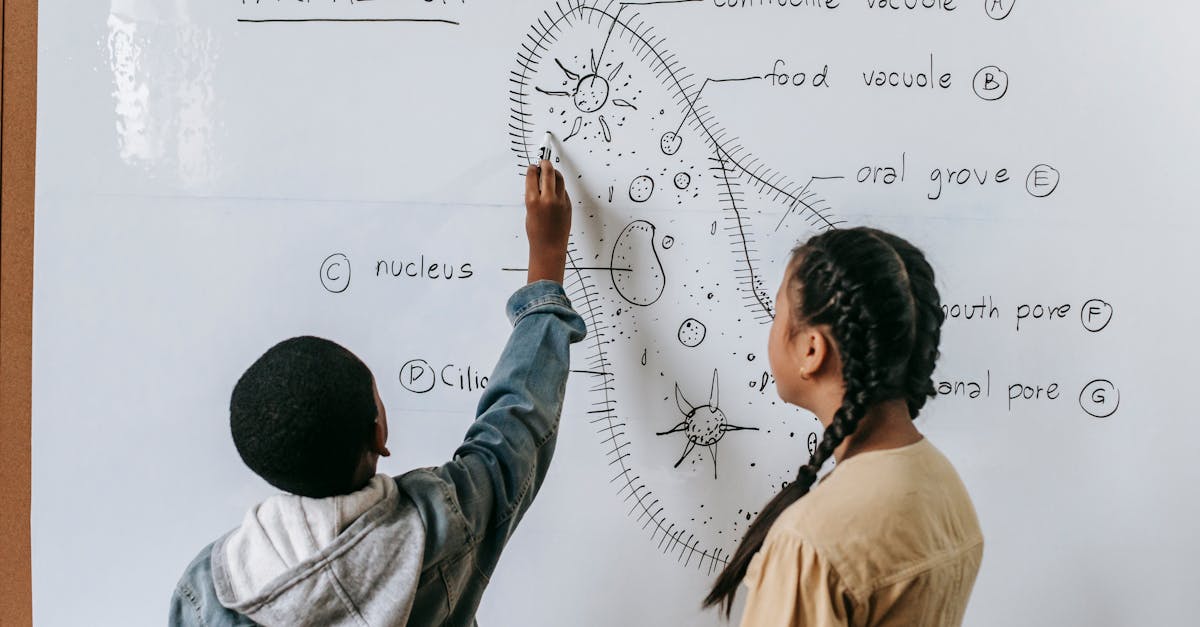
Understanding Statistical Problems
When exploring the world of statistics, it’s critical to assimilate the nature of statistical problems. These problems often involve interpreting data, looking at patterns, and drawing meaningful endings. Here are some key points to consider:
- Data Collection: The foundation of statistical analysis lies in accurate data collection. Whether it’s surveys, experiments, or observational studies, gathering relevant data is important.
- Descriptive Statistics: Understanding descriptive statistics helps in summarizing and interpreting data effectively. Measures such as mean, median, and standard deviation provide useful ideas.
- Inferential Statistics: This branch of statistics involves making predictions or inferences about a population based on sample data. It helps us understand patterns and trends past the observed data.
- Probability: Probability is huge in statistical analysis by quantifying uncertainty. It helps us assess the likelihood of events and make smart decisionss.
To solve statistical problems proficiently, one must have a solid grasp of these key concepts.
By honing our statistical literacy and analytical skills, we can find the way in through complex data sets with confidence.
Let’s investigate more into the area of statistics and unpack its secrets.
Identifying Common Statistical Roadblocks
When tackling statistical problems, identifying common roadblocks is important to effectively find the way in the problem-solving process.
Let’s investigate some key problems individuals often encounter:
- Misinterpretation of Data: One of the primary tough difficulties is misinterpreting the data, leading to erroneous endings and flawed analysis.
- Selection of Appropriate Statistical Tests: Choosing the right statistical test can be perplexing, impacting the accuracy of results. It’s critical to have a solid understanding of when to apply each test.
- Assumptions Violation: Many statistical methods are based on certain assumptions. Violating these assumptions can skew results and mislead interpretations.
To overcome these roadblocks, it’s necessary to acquire a solid foundation in statistical principles and methodologies.
By honing our analytical skills and continuously improving our statistical literacy, we can adeptly address these tough difficulties and excel in statistical problem-solving.
For more ideas on tackling statistical problems, refer to this full guide on Common Statistical Errors .
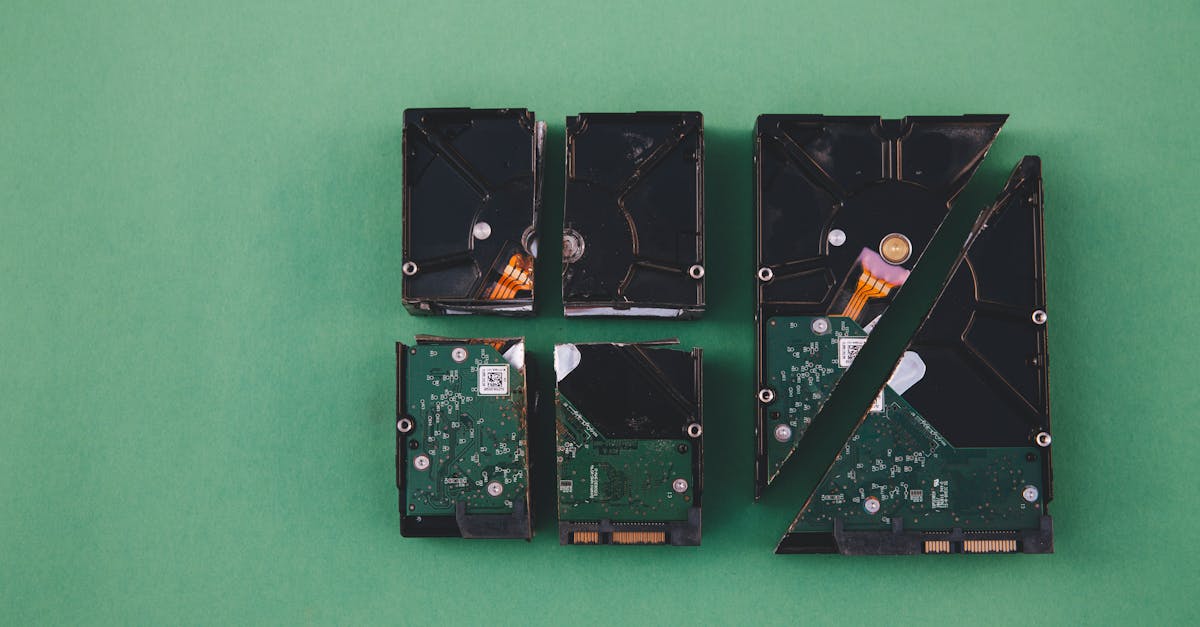
Strategies for Tackling Statistical Tough difficulties
When facing statistical tough difficulties, it’s critical to employ effective strategies to find the way in through complex data analysis.
Here are some key approaches to tackle statistical problems:
- Understand the Problem: Before exploring analysis, ensure a clear comprehension of the statistical problem at hand.
- Choose the Right Tools: Selecting appropriate statistical tests is important for accurate results.
- Check Assumptions: Verify that the data meets the assumptions of the chosen statistical method to avoid skewed outcomes.
- Consult Resources: Refer to reputable sources like textbooks or online statistical guides for assistance.
- Practice Regularly: Improve statistical skills through consistent practice and application in various scenarios.
- Seek Guidance: When in doubt, seek advice from experienced statisticians or mentors.
By adopting these strategies, individuals can improve their problem-solving abilities and overcome statistical problems with confidence.
For further ideas on statistical problem-solving, refer to a full guide on Common Statistical Errors .
Tools for Solving Statistical Problems
When it comes to tackling statistical tough difficulties effectively, having the right tools at our disposal is important.
Here are some key tools that can aid us in solving statistical problems:
- Statistical Software: Using software like R or Python can simplify complex calculations and streamline data analysis processes.
- Graphing Calculators: These tools are handy for visualizing data and identifying trends or patterns.
- Online Resources: Websites like Kaggle or Stack Overflow offer useful ideas, tutorials, and communities for statistical problem-solving.
- Textbooks and Guides: Referencing textbooks such as “Introduction to Statistical Learning” or online guides can provide in-depth explanations and step-by-step solutions.
By using these tools effectively, we can improve our problem-solving capabilities and approach statistical tough difficulties with confidence.
For further ideas on common statistical errors to avoid, we recommend checking out the full guide on Common Statistical Errors For useful tips and strategies.
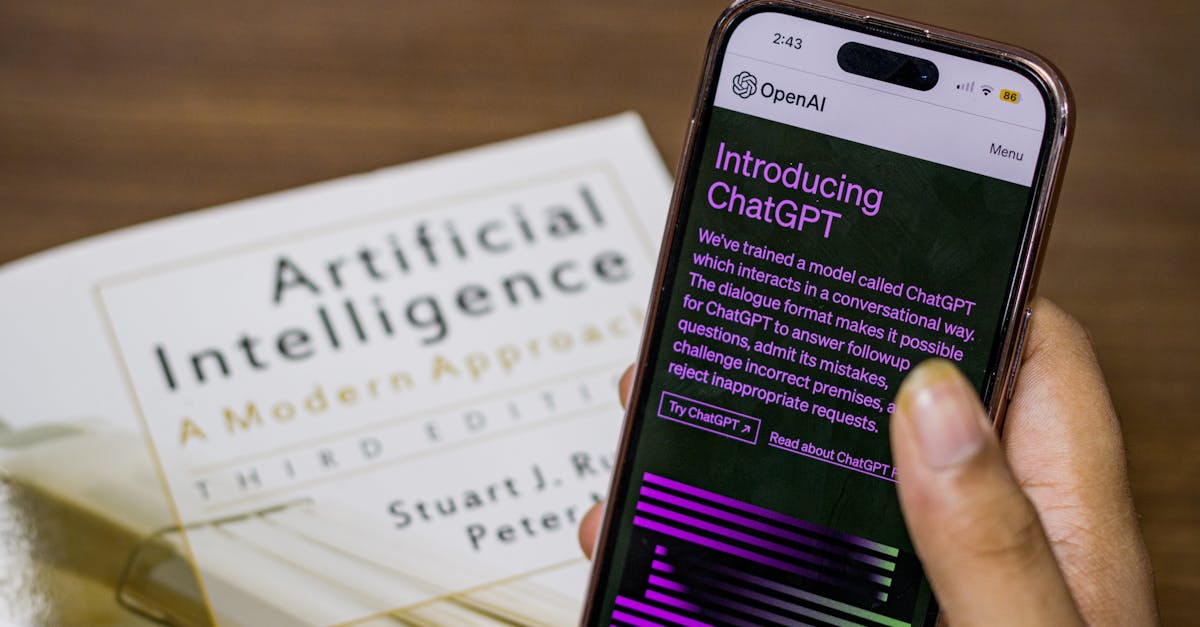
Putting in place Effective Solutions
When approaching statistical problems, it’s critical to have a strategic plan in place.
Here are some key steps to consider for putting in place effective solutions:
- Define the Problem: Clearly outline the statistical problem at hand to understand its scope and requirements fully.
- Collect Data: Gather relevant data sets from credible sources or conduct surveys to acquire the necessary information for analysis.
- Choose the Right Model: Select the appropriate statistical model based on the nature of the data and the specific question being addressed.
- Use Advanced Tools: Use statistical software such as R or Python to perform complex analyses and generate accurate results.
- Validate Results: Verify the accuracy of the findings through strict testing and validation procedures to ensure the reliability of the endings.
By following these steps, we can streamline the statistical problem-solving process and arrive at well-informed and data-driven decisions.
For further ideas and strategies on tackling statistical tough difficulties, we recommend exploring resources such as DataCamp That offer interactive learning experiences and tutorials on statistical analysis.
- Recent Posts
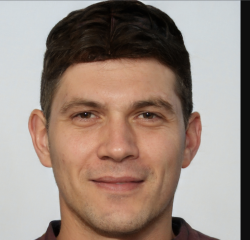
- What do Beginner Software Engineers Do? [Unlock Success Secrets] - August 11, 2024
- Using ATEM Software Without Hardware: Options & Recommendations [Must-Read Insights] - August 11, 2024
- How much does AT&T pay big data software engineers? Here’s what you need to know [Maximize Your Earnings] - August 11, 2024

Effective Use of Statistics in Research – Methods and Tools for Data Analysis
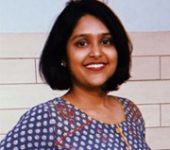
Remember that impending feeling you get when you are asked to analyze your data! Now that you have all the required raw data, you need to statistically prove your hypothesis. Representing your numerical data as part of statistics in research will also help in breaking the stereotype of being a biology student who can’t do math.
Statistical methods are essential for scientific research. In fact, statistical methods dominate the scientific research as they include planning, designing, collecting data, analyzing, drawing meaningful interpretation and reporting of research findings. Furthermore, the results acquired from research project are meaningless raw data unless analyzed with statistical tools. Therefore, determining statistics in research is of utmost necessity to justify research findings. In this article, we will discuss how using statistical methods for biology could help draw meaningful conclusion to analyze biological studies.
Table of Contents
Role of Statistics in Biological Research
Statistics is a branch of science that deals with collection, organization and analysis of data from the sample to the whole population. Moreover, it aids in designing a study more meticulously and also give a logical reasoning in concluding the hypothesis. Furthermore, biology study focuses on study of living organisms and their complex living pathways, which are very dynamic and cannot be explained with logical reasoning. However, statistics is more complex a field of study that defines and explains study patterns based on the sample sizes used. To be precise, statistics provides a trend in the conducted study.
Biological researchers often disregard the use of statistics in their research planning, and mainly use statistical tools at the end of their experiment. Therefore, giving rise to a complicated set of results which are not easily analyzed from statistical tools in research. Statistics in research can help a researcher approach the study in a stepwise manner, wherein the statistical analysis in research follows –
1. Establishing a Sample Size
Usually, a biological experiment starts with choosing samples and selecting the right number of repetitive experiments. Statistics in research deals with basics in statistics that provides statistical randomness and law of using large samples. Statistics teaches how choosing a sample size from a random large pool of sample helps extrapolate statistical findings and reduce experimental bias and errors.
2. Testing of Hypothesis
When conducting a statistical study with large sample pool, biological researchers must make sure that a conclusion is statistically significant. To achieve this, a researcher must create a hypothesis before examining the distribution of data. Furthermore, statistics in research helps interpret the data clustered near the mean of distributed data or spread across the distribution. These trends help analyze the sample and signify the hypothesis.
3. Data Interpretation Through Analysis
When dealing with large data, statistics in research assist in data analysis. This helps researchers to draw an effective conclusion from their experiment and observations. Concluding the study manually or from visual observation may give erroneous results; therefore, thorough statistical analysis will take into consideration all the other statistical measures and variance in the sample to provide a detailed interpretation of the data. Therefore, researchers produce a detailed and important data to support the conclusion.
Types of Statistical Research Methods That Aid in Data Analysis
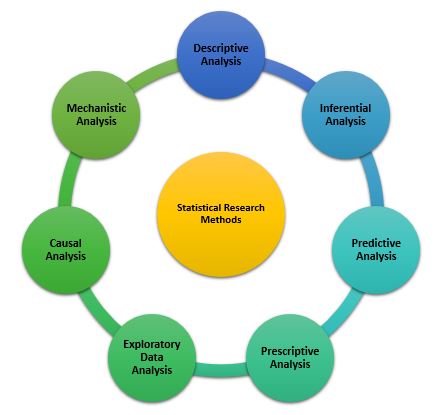
Statistical analysis is the process of analyzing samples of data into patterns or trends that help researchers anticipate situations and make appropriate research conclusions. Based on the type of data, statistical analyses are of the following type:
1. Descriptive Analysis
The descriptive statistical analysis allows organizing and summarizing the large data into graphs and tables . Descriptive analysis involves various processes such as tabulation, measure of central tendency, measure of dispersion or variance, skewness measurements etc.
2. Inferential Analysis
The inferential statistical analysis allows to extrapolate the data acquired from a small sample size to the complete population. This analysis helps draw conclusions and make decisions about the whole population on the basis of sample data. It is a highly recommended statistical method for research projects that work with smaller sample size and meaning to extrapolate conclusion for large population.
3. Predictive Analysis
Predictive analysis is used to make a prediction of future events. This analysis is approached by marketing companies, insurance organizations, online service providers, data-driven marketing, and financial corporations.
4. Prescriptive Analysis
Prescriptive analysis examines data to find out what can be done next. It is widely used in business analysis for finding out the best possible outcome for a situation. It is nearly related to descriptive and predictive analysis. However, prescriptive analysis deals with giving appropriate suggestions among the available preferences.
5. Exploratory Data Analysis
EDA is generally the first step of the data analysis process that is conducted before performing any other statistical analysis technique. It completely focuses on analyzing patterns in the data to recognize potential relationships. EDA is used to discover unknown associations within data, inspect missing data from collected data and obtain maximum insights.
6. Causal Analysis
Causal analysis assists in understanding and determining the reasons behind “why” things happen in a certain way, as they appear. This analysis helps identify root cause of failures or simply find the basic reason why something could happen. For example, causal analysis is used to understand what will happen to the provided variable if another variable changes.
7. Mechanistic Analysis
This is a least common type of statistical analysis. The mechanistic analysis is used in the process of big data analytics and biological science. It uses the concept of understanding individual changes in variables that cause changes in other variables correspondingly while excluding external influences.
Important Statistical Tools In Research
Researchers in the biological field find statistical analysis in research as the scariest aspect of completing research. However, statistical tools in research can help researchers understand what to do with data and how to interpret the results, making this process as easy as possible.
1. Statistical Package for Social Science (SPSS)
It is a widely used software package for human behavior research. SPSS can compile descriptive statistics, as well as graphical depictions of result. Moreover, it includes the option to create scripts that automate analysis or carry out more advanced statistical processing.
2. R Foundation for Statistical Computing
This software package is used among human behavior research and other fields. R is a powerful tool and has a steep learning curve. However, it requires a certain level of coding. Furthermore, it comes with an active community that is engaged in building and enhancing the software and the associated plugins.
3. MATLAB (The Mathworks)
It is an analytical platform and a programming language. Researchers and engineers use this software and create their own code and help answer their research question. While MatLab can be a difficult tool to use for novices, it offers flexibility in terms of what the researcher needs.
4. Microsoft Excel
Not the best solution for statistical analysis in research, but MS Excel offers wide variety of tools for data visualization and simple statistics. It is easy to generate summary and customizable graphs and figures. MS Excel is the most accessible option for those wanting to start with statistics.
5. Statistical Analysis Software (SAS)
It is a statistical platform used in business, healthcare, and human behavior research alike. It can carry out advanced analyzes and produce publication-worthy figures, tables and charts .
6. GraphPad Prism
It is a premium software that is primarily used among biology researchers. But, it offers a range of variety to be used in various other fields. Similar to SPSS, GraphPad gives scripting option to automate analyses to carry out complex statistical calculations.
This software offers basic as well as advanced statistical tools for data analysis. However, similar to GraphPad and SPSS, minitab needs command over coding and can offer automated analyses.
Use of Statistical Tools In Research and Data Analysis
Statistical tools manage the large data. Many biological studies use large data to analyze the trends and patterns in studies. Therefore, using statistical tools becomes essential, as they manage the large data sets, making data processing more convenient.
Following these steps will help biological researchers to showcase the statistics in research in detail, and develop accurate hypothesis and use correct tools for it.
There are a range of statistical tools in research which can help researchers manage their research data and improve the outcome of their research by better interpretation of data. You could use statistics in research by understanding the research question, knowledge of statistics and your personal experience in coding.
Have you faced challenges while using statistics in research? How did you manage it? Did you use any of the statistical tools to help you with your research data? Do write to us or comment below!
Frequently Asked Questions
Statistics in research can help a researcher approach the study in a stepwise manner: 1. Establishing a sample size 2. Testing of hypothesis 3. Data interpretation through analysis
Statistical methods are essential for scientific research. In fact, statistical methods dominate the scientific research as they include planning, designing, collecting data, analyzing, drawing meaningful interpretation and reporting of research findings. Furthermore, the results acquired from research project are meaningless raw data unless analyzed with statistical tools. Therefore, determining statistics in research is of utmost necessity to justify research findings.
Statistical tools in research can help researchers understand what to do with data and how to interpret the results, making this process as easy as possible. They can manage large data sets, making data processing more convenient. A great number of tools are available to carry out statistical analysis of data like SPSS, SAS (Statistical Analysis Software), and Minitab.

nice article to read
Holistic but delineating. A very good read.
Rate this article Cancel Reply
Your email address will not be published.
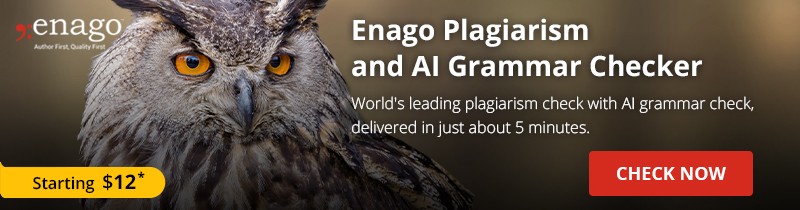
Enago Academy's Most Popular Articles
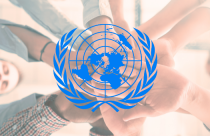
- Promoting Research
- Thought Leadership
- Trending Now
How Enago Academy Contributes to Sustainable Development Goals (SDGs) Through Empowering Researchers
The United Nations Sustainable Development Goals (SDGs) are a universal call to action to end…
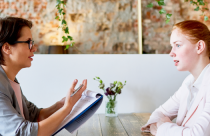
- Reporting Research
Research Interviews: An effective and insightful way of data collection
Research interviews play a pivotal role in collecting data for various academic, scientific, and professional…
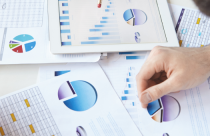
Planning Your Data Collection: Designing methods for effective research
Planning your research is very important to obtain desirable results. In research, the relevance of…
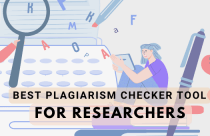
- Language & Grammar
Best Plagiarism Checker Tool for Researchers — Top 4 to choose from!
While common writing issues like language enhancement, punctuation errors, grammatical errors, etc. can be dealt…
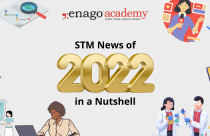
- Industry News
- Publishing News
2022 in a Nutshell — Reminiscing the year when opportunities were seized and feats were achieved!
It’s beginning to look a lot like success! Some of the greatest opportunities to research…
2022 in a Nutshell — Reminiscing the year when opportunities were seized and feats…

Sign-up to read more
Subscribe for free to get unrestricted access to all our resources on research writing and academic publishing including:
- 2000+ blog articles
- 50+ Webinars
- 10+ Expert podcasts
- 50+ Infographics
- 10+ Checklists
- Research Guides
We hate spam too. We promise to protect your privacy and never spam you.
- Publishing Research
- AI in Academia
- Career Corner
- Diversity and Inclusion
- Infographics
- Expert Video Library
- Other Resources
- Enago Learn
- Upcoming & On-Demand Webinars
- Peer-Review Week 2023
- Open Access Week 2023
- Conference Videos
- Enago Report
- Journal Finder
- Enago Plagiarism & AI Grammar Check
- Editing Services
- Publication Support Services
- Research Impact
- Translation Services
- Publication solutions
- AI-Based Solutions
- Call for Articles
- Call for Speakers
- Author Training
- Edit Profile
I am looking for Editing/ Proofreading services for my manuscript Tentative date of next journal submission:
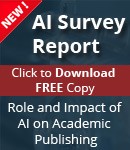
In your opinion, what is the most effective way to improve integrity in the peer review process?

What is Problem Solving? (Steps, Techniques, Examples)
By Status.net Editorial Team on May 7, 2023 — 5 minutes to read
What Is Problem Solving?
Definition and importance.
Problem solving is the process of finding solutions to obstacles or challenges you encounter in your life or work. It is a crucial skill that allows you to tackle complex situations, adapt to changes, and overcome difficulties with ease. Mastering this ability will contribute to both your personal and professional growth, leading to more successful outcomes and better decision-making.
Problem-Solving Steps
The problem-solving process typically includes the following steps:
- Identify the issue : Recognize the problem that needs to be solved.
- Analyze the situation : Examine the issue in depth, gather all relevant information, and consider any limitations or constraints that may be present.
- Generate potential solutions : Brainstorm a list of possible solutions to the issue, without immediately judging or evaluating them.
- Evaluate options : Weigh the pros and cons of each potential solution, considering factors such as feasibility, effectiveness, and potential risks.
- Select the best solution : Choose the option that best addresses the problem and aligns with your objectives.
- Implement the solution : Put the selected solution into action and monitor the results to ensure it resolves the issue.
- Review and learn : Reflect on the problem-solving process, identify any improvements or adjustments that can be made, and apply these learnings to future situations.
Defining the Problem
To start tackling a problem, first, identify and understand it. Analyzing the issue thoroughly helps to clarify its scope and nature. Ask questions to gather information and consider the problem from various angles. Some strategies to define the problem include:
- Brainstorming with others
- Asking the 5 Ws and 1 H (Who, What, When, Where, Why, and How)
- Analyzing cause and effect
- Creating a problem statement
Generating Solutions
Once the problem is clearly understood, brainstorm possible solutions. Think creatively and keep an open mind, as well as considering lessons from past experiences. Consider:
- Creating a list of potential ideas to solve the problem
- Grouping and categorizing similar solutions
- Prioritizing potential solutions based on feasibility, cost, and resources required
- Involving others to share diverse opinions and inputs
Evaluating and Selecting Solutions
Evaluate each potential solution, weighing its pros and cons. To facilitate decision-making, use techniques such as:
- SWOT analysis (Strengths, Weaknesses, Opportunities, Threats)
- Decision-making matrices
- Pros and cons lists
- Risk assessments
After evaluating, choose the most suitable solution based on effectiveness, cost, and time constraints.
Implementing and Monitoring the Solution
Implement the chosen solution and monitor its progress. Key actions include:
- Communicating the solution to relevant parties
- Setting timelines and milestones
- Assigning tasks and responsibilities
- Monitoring the solution and making adjustments as necessary
- Evaluating the effectiveness of the solution after implementation
Utilize feedback from stakeholders and consider potential improvements. Remember that problem-solving is an ongoing process that can always be refined and enhanced.
Problem-Solving Techniques
During each step, you may find it helpful to utilize various problem-solving techniques, such as:
- Brainstorming : A free-flowing, open-minded session where ideas are generated and listed without judgment, to encourage creativity and innovative thinking.
- Root cause analysis : A method that explores the underlying causes of a problem to find the most effective solution rather than addressing superficial symptoms.
- SWOT analysis : A tool used to evaluate the strengths, weaknesses, opportunities, and threats related to a problem or decision, providing a comprehensive view of the situation.
- Mind mapping : A visual technique that uses diagrams to organize and connect ideas, helping to identify patterns, relationships, and possible solutions.
Brainstorming
When facing a problem, start by conducting a brainstorming session. Gather your team and encourage an open discussion where everyone contributes ideas, no matter how outlandish they may seem. This helps you:
- Generate a diverse range of solutions
- Encourage all team members to participate
- Foster creative thinking
When brainstorming, remember to:
- Reserve judgment until the session is over
- Encourage wild ideas
- Combine and improve upon ideas
Root Cause Analysis
For effective problem-solving, identifying the root cause of the issue at hand is crucial. Try these methods:
- 5 Whys : Ask “why” five times to get to the underlying cause.
- Fishbone Diagram : Create a diagram representing the problem and break it down into categories of potential causes.
- Pareto Analysis : Determine the few most significant causes underlying the majority of problems.
SWOT Analysis
SWOT analysis helps you examine the Strengths, Weaknesses, Opportunities, and Threats related to your problem. To perform a SWOT analysis:
- List your problem’s strengths, such as relevant resources or strong partnerships.
- Identify its weaknesses, such as knowledge gaps or limited resources.
- Explore opportunities, like trends or new technologies, that could help solve the problem.
- Recognize potential threats, like competition or regulatory barriers.
SWOT analysis aids in understanding the internal and external factors affecting the problem, which can help guide your solution.
Mind Mapping
A mind map is a visual representation of your problem and potential solutions. It enables you to organize information in a structured and intuitive manner. To create a mind map:
- Write the problem in the center of a blank page.
- Draw branches from the central problem to related sub-problems or contributing factors.
- Add more branches to represent potential solutions or further ideas.
Mind mapping allows you to visually see connections between ideas and promotes creativity in problem-solving.
Examples of Problem Solving in Various Contexts
In the business world, you might encounter problems related to finances, operations, or communication. Applying problem-solving skills in these situations could look like:
- Identifying areas of improvement in your company’s financial performance and implementing cost-saving measures
- Resolving internal conflicts among team members by listening and understanding different perspectives, then proposing and negotiating solutions
- Streamlining a process for better productivity by removing redundancies, automating tasks, or re-allocating resources
In educational contexts, problem-solving can be seen in various aspects, such as:
- Addressing a gap in students’ understanding by employing diverse teaching methods to cater to different learning styles
- Developing a strategy for successful time management to balance academic responsibilities and extracurricular activities
- Seeking resources and support to provide equal opportunities for learners with special needs or disabilities
Everyday life is full of challenges that require problem-solving skills. Some examples include:
- Overcoming a personal obstacle, such as improving your fitness level, by establishing achievable goals, measuring progress, and adjusting your approach accordingly
- Navigating a new environment or city by researching your surroundings, asking for directions, or using technology like GPS to guide you
- Dealing with a sudden change, like a change in your work schedule, by assessing the situation, identifying potential impacts, and adapting your plans to accommodate the change.
- How to Resolve Employee Conflict at Work [Steps, Tips, Examples]
- How to Write Inspiring Core Values? 5 Steps with Examples
- 30 Employee Feedback Examples (Positive & Negative)
Using Statistics to Improve Problem Solving Skills
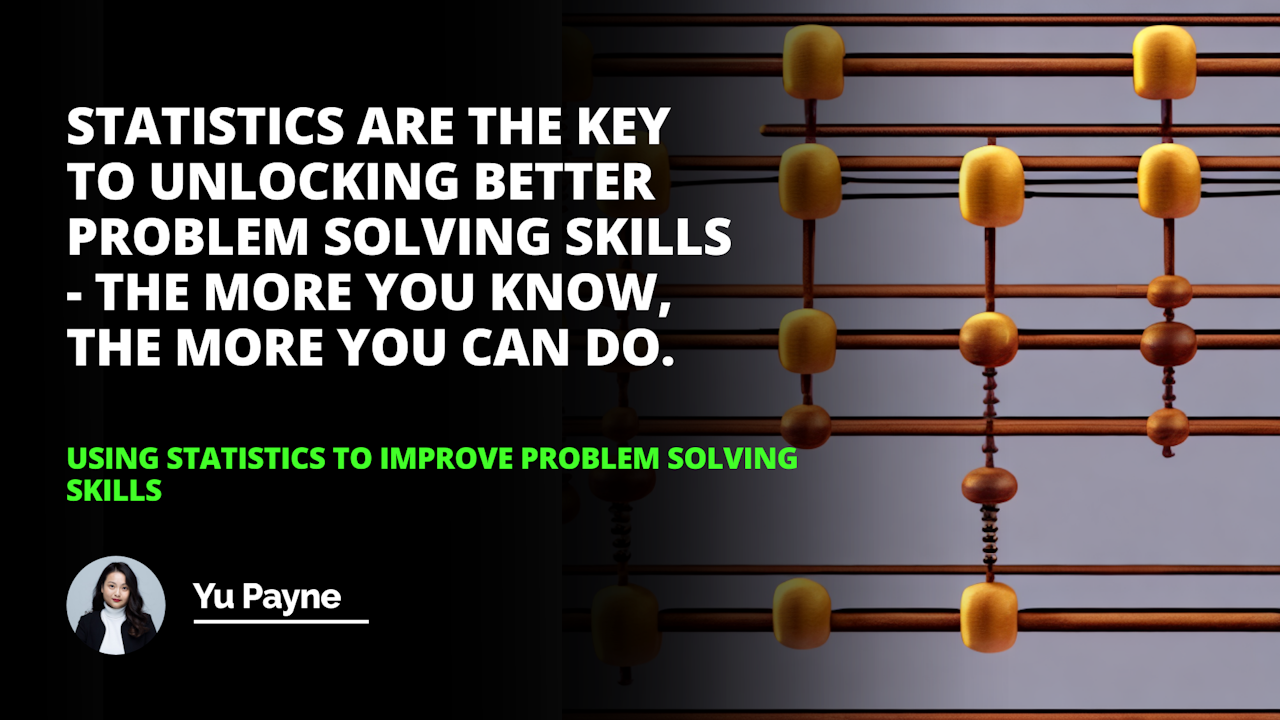
Statistical Method | Application | Advantage |
---|---|---|
Probability Theory | Used to analyze the likelihood of an event occurring in various fields including finance, economics, and engineering. | It provides a measure of how likely a specific event is to happen and can manage uncertainty. |
Correlation Analysis | Used to identify the strength of the relationship between two variables in fields like economics, finance, and psychology. | Helps in predicting one variable based on the other and helps in data forecasting. |
Estimation Theory | Helps estimate the value of a variable based on set data, commonly used in economics, finance, and engineering. | Enhances decision-making by providing an estimate even with limited data or resources |
Sampling Theory | Used in research to draw inference about a population from a sample. | It's efficient and cost-effective, making it possible to study large populations. |
Hypothesis Testing | Used to decide if a result of a study can reject a null hypothesis in a scientific experiment. | It helps to validate predictability and reliability of data. |
Least Squares Fitting | Used in regression analysis to approximate the solution of overdetermined systems. | It provides the best fit line for the given data. |
Chi-Square Testing | Used in statistics to test the independence of two events. | It offers a methodology to collect and present data in a meaningful way. |
Poisson Distribution | Used to model the number of times an event happens in a fixed interval of time or space. | Particularly useful for rare events. |
Binomial Distribution | Used when there are exactly two mutually exclusive outcomes of a trial. | It provides the basis for the binomial test of statistical significance. |
Solution via Statistics | End-to-end problem-solving tool using the power of statistics | Helps to make better decisions, manage uncertainty, and predict outcomes. |
Problem-solving is an essential skill that everyone must possess, and statistics is a powerful tool that can be used to help solve problems. Statistics uses probability theory as its base and has a rich assortment of submethods, such as probability theory, correlation analysis, estimation theory, sampling theory, hypothesis testing, least squares fitting, chi-square testing, and specific distributions.
Each of these submethods has its unique set of advantages and disadvantages, so it is essential to understand the strengths and weaknesses of each method when attempting to solve a problem.
Introduction
Overview of Problem-Solving
Role of statistics in problem-solving, probability theory, correlation analysis.
Introduction: Problem-solving is a fundamental part of life and an essential skill everyone must possess. It is an integral part of the learning process and is used in various situations. When faced with a problem, it is essential to have the necessary tools and knowledge to identify and solve it. Statistics is one such tool that can be used to help solve problems.
Problem-solving is the process of identifying and finding solutions to a problem. It involves understanding the problem, analyzing the available information, and coming up with a practical and effective solution. Problem-solving is used in various fields, including business, engineering, science, and mathematics.
Statistics is a powerful tool that can be used to help solve problems. Statistics uses probability theory as its base, so when your problem can be stated as a probability, you can reliably go to statistics as an approach. Statistics, as a discipline, has a rich assortment of submethods, such as probability theory, correlation analysis, estimation theory, sampling theory, hypothesis testing, least squares fitting, chi-square testing, and specific distributions (e.g., Poisson, Binomial, etc.).
Probability theory is the mathematical study of chance. It is used to analyze the likelihood of an event occurring. Probability theory is used to determine the likelihood of an event, such as the probability of a coin landing heads up or a certain number being drawn in a lottery. Probability theory is used in various fields, including finance, economics, and engineering.
Correlation analysis is used to determine the relationship between two variables. It is used to identify the strength of the relationship between two variables, such as the correlation between the temperature and the amount of rainfall. Correlation analysis is used in various fields, including economics, finance, and psychology.
Estimation Theory
Estimation theory is used to estimate the value of a variable based on a set of data. It is used to estimate the value of a variable, such as a city's population, based on a sample of the population. Estimation theory is used in various fields, including economics, finance, and engineering.
Conclusion: Statistics is a powerful tool that can be used to help solve problems. Statistics uses probability theory as its base, so when your problem can be stated as a probability, you can reliably go to statistics as an approach. Statistics, as a discipline, has a rich assortment of submethods, such as probability theory, correlation analysis, estimation theory, sampling theory, hypothesis testing, least squares fitting, chi-square testing, and specific distributions (e.g., Poisson, Binomial, etc.). Each submethod has unique advantages and disadvantages, so it is essential to select the one that best suits your problem. With the right approach and tools, statistics can be a powerful tool in problem-solving.
Statistics are the key to unlocking better problem-solving skills - the more you know, the more you can do. IIENSTITU
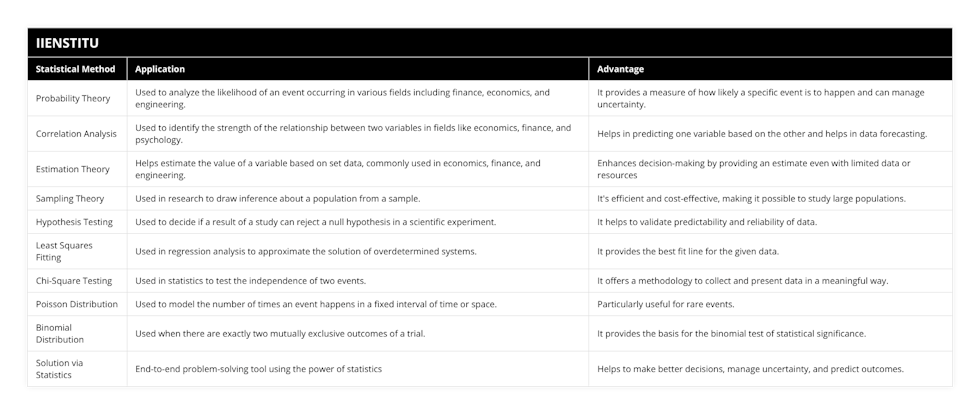
What role does probability theory play in using statistics to improve problem solving skills?
Probability theory and statistics are both essential tools for problem-solving, and the two disciplines share an interdependent solid relationship. This article will discuss the role that probability theory plays in using statistics to improve problem-solving skills.
Probability theory provides a framework for understanding the behavior of random variables and their associated distributions. We can use statistics to make better predictions and decisions by understanding and applying probability theory. For example, when calculating the probability of a desired outcome, we can use statistical methods to determine the likelihood of that outcome occurring. This can be used to inform decisions and help us optimize our strategies.
Statistics also provide us with powerful tools for understanding the relationship between variables. By analyzing the correlation between two or more variables, we can gain valuable insights into the underlying causes and effects of a problem. For example, by studying a correlation between two variables, we can determine which variable is more likely to cause a particular outcome. This can help us to design more effective solutions to problems.
By combining probability theory and statistics, we can develop powerful strategies for problem-solving. Probability theory helps us understand a problem's underlying structure, while statistics provide us with the tools to analyze the data and make better predictions. By understanding how to use these two disciplines together, we can develop more effective solutions to difficult problems.
In conclusion, probability theory and statistics are both essential for problem-solving. Probability theory provides a framework for understanding the behavior of random variables, while statistics provide powerful tools for understanding the relationships between variables. By combining the two disciplines, we can develop more effective strategies for solving complex problems.
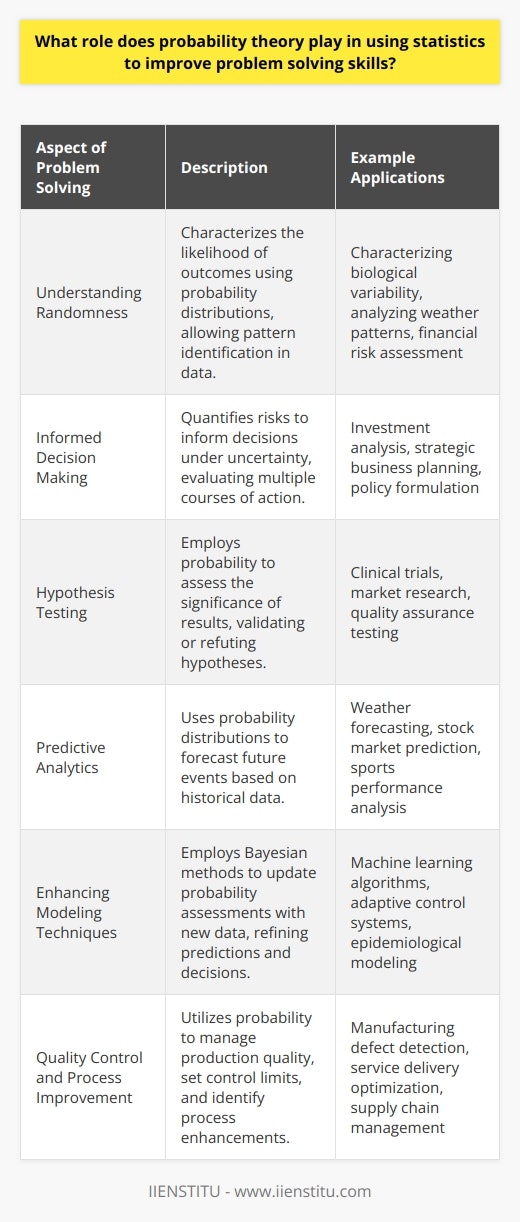
How can correlation analysis be used to identify relationships between variables when solving problems?
Correlation analysis is a powerful tool for identifying relationships between variables when solving problems. It is a statistical approach that measures how two variables are related. By analyzing the correlation between two variables, researchers can identify the strength and direction of their relationship. For example, a correlation analysis can determine if a change in one variable is associated with a change in the other.
When conducting correlation analysis, researchers often use Pearson’s correlation coefficient (r) to measure the strength of the association between two variables. This coefficient ranges from -1 to +1, where -1 indicates a perfect negative correlation, 0 indicates no correlation, and +1 indicates a perfect positive correlation. A perfect positive correlation indicates that when one variable increases, the other variable also increases, and a perfect negative correlation indicates that when one variable increases, the other variable decreases.
Correlation analysis helps identify relationships between variables when solving problems. For example, in a study of the relationship between dietary habits and body weight, a researcher may use correlation analysis to determine if there is a relationship between the two variables. Suppose the researcher finds a significant correlation between dietary habits and body weight. In that case, this can provide insight into the studied problem and help inform solutions.
Correlation analysis can also be used to identify causal relationships between variables. By examining the relationship between two variables over time, researchers can determine if a change in one variable is associated with a change in the other. For example, a researcher may use correlation analysis to determine if temperature changes are associated with changes in air quality. If a significant correlation is found, then the researcher can conclude that temperature changes are likely causing changes in air quality.
Overall, correlation analysis is a powerful tool for identifying relationships between variables when solving problems. By examining the strength and direction of the relationship between two variables, researchers can gain insight into the problem being studied and inform potential solutions.
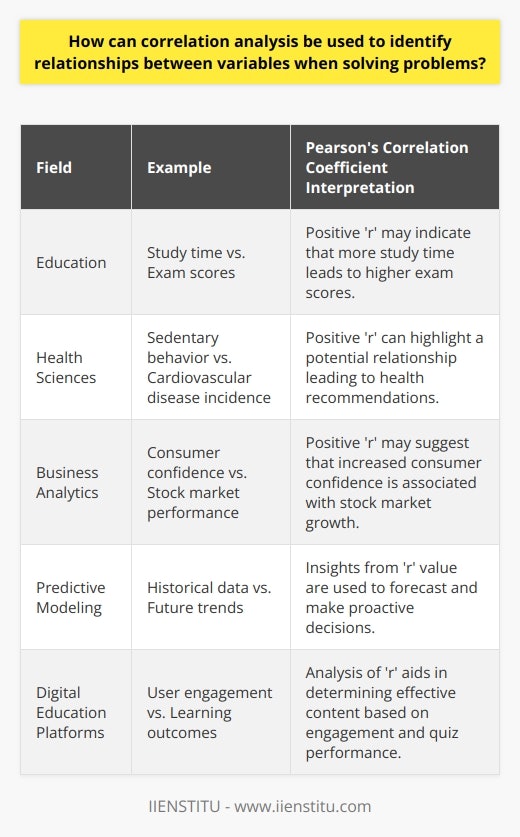
What are the benefits of using estimation theory when attempting to solve complex problems?
Estimation theory is a powerful tool when attempting to solve complex problems. This theory involves making educated guesses or estimations about the value of a quantity that is difficult or impossible to measure directly. By utilizing estimation theory, one can reduce uncertainty and make decisions more confidently.
The main benefit of using estimation theory is that it allows for the quantification of uncertainty. By estimating, one can determine the range of possible outcomes and make decisions based on the likelihood of each outcome. This helps to reduce the risks associated with making decisions as it allows one to make better decisions based on the available data.
Another benefit of using estimation theory is that it can be applied to many problems. Estimation theory can be used to solve problems in fields such as engineering, finance, and economics. It can also be used to estimate a stock's value, the project's cost, or the probability of a certain event. Estimation theory is also useful in predicting the behavior of a system over time.
Estimation theory can also be used to make decisions in cases where the data is limited. By estimating, one can reduce the amount of data needed to make a decision and make more informed decisions. Furthermore, estimation theory can be used to make decisions even when the data is incomplete or inaccurate. This is especially useful when making decisions in situations where the data is uncertain or incomplete.
In conclusion, estimation theory is a powerful tool for solving complex problems. It can be used to reduce uncertainty, make decisions in cases where data is limited or incomplete, and make predictions about the behavior of a system over time. By utilizing estimation theory, one can make more informed decisions and reduce the risks associated.
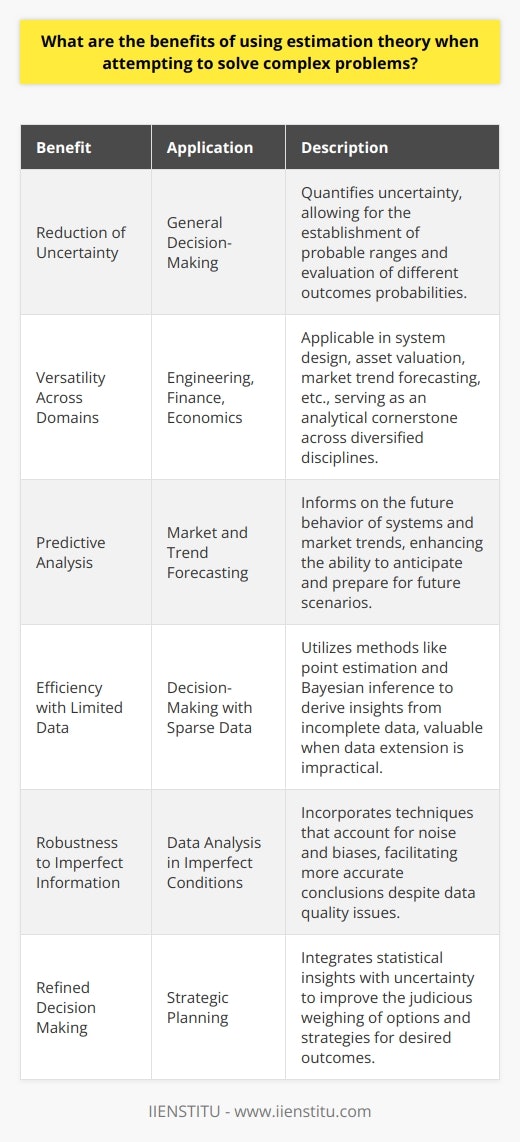
How does the application of statistical methods contribute to effective problem-solving in various fields?
**Statistical Methods in Problem-solving** Statistical methods play a crucial role in effective problem-solving across various fields, including natural and social sciences, economics, and engineering. One primary contribution lies in the quantification and analysis of data. **Data Quantification and Analysis** Through descriptive statistics, researchers can summarize, organize, and simplify large data sets, enabling them to extract essential features and identify patterns. In turn, this fosters a deeper understanding of complex issues and aids in data-driven decision-making. **Prediction and Forecasting** Statistical methods can help predict future trends and potential outcomes with a certain level of confidence by extrapolating obtained data. Such prediction models are invaluable in fields as diverse as finance, healthcare, and environmental science, enabling key stakeholders to take proactive measures. **Hypothesis Testing** In the scientific process, hypothesis testing enables practitioners to make inferences about populations based on sample data. By adopting rigorous statistical methods, researchers can determine the likelihood of observed results occurring randomly or due to a specific relationship, thus validating or refuting hypotheses. **Quality Control and Improvement** In industries and manufacturing, statistical methods are applied in quality control measures to ensure that products and services meet established standards consistently. By identifying variations, trends, and deficiencies within production processes, statistical techniques guide improvement efforts. **Design of Experiments** Statistical methods are vital in the design of experiments, ensuring that the collected data is representative, reliable, and unbiased. By utilizing techniques such as random sampling and random assignment, researchers can mitigate confounding variables, increase generalizability, and establish causal relationships. In conclusion, the application of statistical methods contributes to effective problem-solving across various fields by enabling data quantification, analysis, and prediction. Additionally, these methods facilitate hypothesis testing, quality control, and the design of experiments, fostering confidence in decision-making and enhancing outcomes.
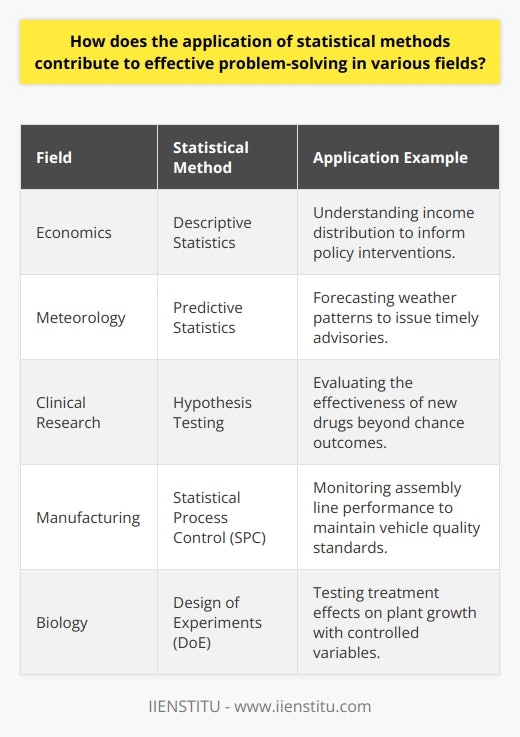
In what ways can statistical analysis enhance the decision-making process when facing complex challenges?
Statistical analysis in decision-making Statistical analysis plays a crucial role in the decision-making process when facing complex challenges by enabling evidence-based decisions. It provides a systematic approach to accurately interpret data and transform it into meaningful and actionable insights. In turn, these insights enhance decision-making by reducing uncertainty, minimizing risks, and increasing confidence in the chosen strategy. Quantitative approach By adopting a quantitative approach, decision-makers can objectively evaluate various options using statistical techniques, such as regression analysis or hypothesis testing. This process facilitates the identification of patterns and relationships within the data, highlighting crucial factors that can significantly impact desired outcomes. Consequently, leaders can make informed decisions that optimize available resources and maximize benefits, ultimately increasing the overall success rate of implemented strategies. Addressing biases Statistical analysis helps to address cognitive biases that may otherwise cloud judgment and impede the decision-making process. These biases could include confirmation bias, anchoring bias, and availability heuristic, among others. Employing quantitative methods illuminates the influence these biases may have on subjective interpretations and assists decision-makers in mitigating potential negative impacts. Risk analysis In the context of complex challenges, risk analysis plays an essential role in decision-making. By employing statistical models, decision-makers can quantify risk, estimate probabilities of potential outcomes, and determine the optimal balance between risk and reward. This information can be invaluable for organizations when allocating resources, prioritizing projects, and managing uncertainty in dynamic environments. Data-driven forecasts Statistical analysis enables decision-makers to create accurate forecasts by extrapolating historical data and incorporating current trends. These forecasts can inform strategic planning, budget allocations, and resource management, reducing the likelihood of unforeseen obstacles and ensuring long-term success. In addition to providing a strong basis for future planning, these data-driven predictions also enable organizations to quickly adapt and respond to emerging trends and challenges. In conclusion, statistical analysis is an invaluable tool for enhancing the decision-making process when facing complex challenges. By adopting a quantitative approach, addressing cognitive biases, conducting risk analysis, and producing data-driven forecasts, decision-makers can make informed choices that optimize outcomes and minimize potential risks.
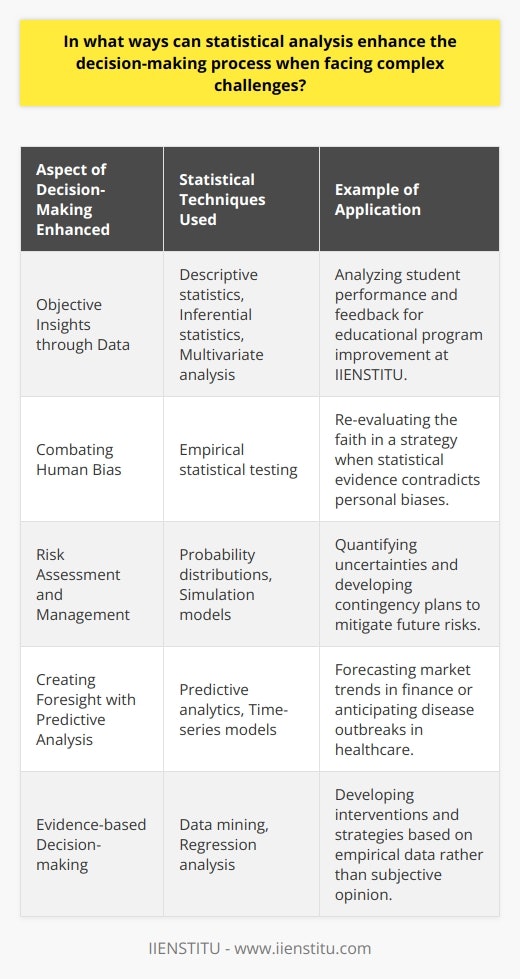
How can concepts like statistical hypothesis testing and regression analysis be applied to solve real-world problems and make informed decisions?
Applications of Hypothesis Testing Statistical hypothesis testing can be a vital tool in decision-making processes, particularly when it comes to addressing real-world problems. In business, for example, managers may use hypothesis testing to determine whether a new product or strategy will lead to higher revenues or customer satisfaction. This can then inform their decisions on whether to invest in the product or strategy or explore other options. In medicine, researchers can use hypothesis testing to compare the effectiveness of a new treatment or intervention compared to standard care, which can provide valuable evidence to guide clinical practice. Regression Analysis to Guide Decisions Similarly, regression analysis is a powerful statistical technique used to understand relationships between variables and predict future outcomes. By modeling the connections between different factors, businesses can make data-driven decisions and develop strategies based on relationships found in historical data. For instance, companies can use regression analysis to forecast future sales, evaluate the return on investment for marketing campaigns, or identify factors that contribute to customer churn. In fields like public health, policymakers can use regression analysis to identify the effects of various interventions on health outcomes, leading to more effective resource allocation and targeting of mass media campaigns. Assessing Real-World Solutions The implementation of statistical hypothesis testing and regression analysis enables stakeholders across diverse disciplines to evaluate and prioritize potential solutions to complex problems. By identifying significant relationships between variables and outcomes, practitioners can develop evidence-based approaches to improve decision-making processes. These methods can be applied to problems in various fields, such as healthcare, public policy, economics, and environmental management, ultimately providing benefits for both individuals and society. Ensuring Informed Decisions In conclusion, both statistical hypothesis testing and regression analysis have a vital role in solving real-world problems and informing decisions. These techniques provide decision-makers with the necessary evidence to evaluate different options, strategies, or interventions to make the most appropriate choices. By incorporating these statistical methods into the decision-making process, stakeholders can increase confidence in their conclusions and improve the overall effectiveness of their actions, leading to better outcomes in various fields.
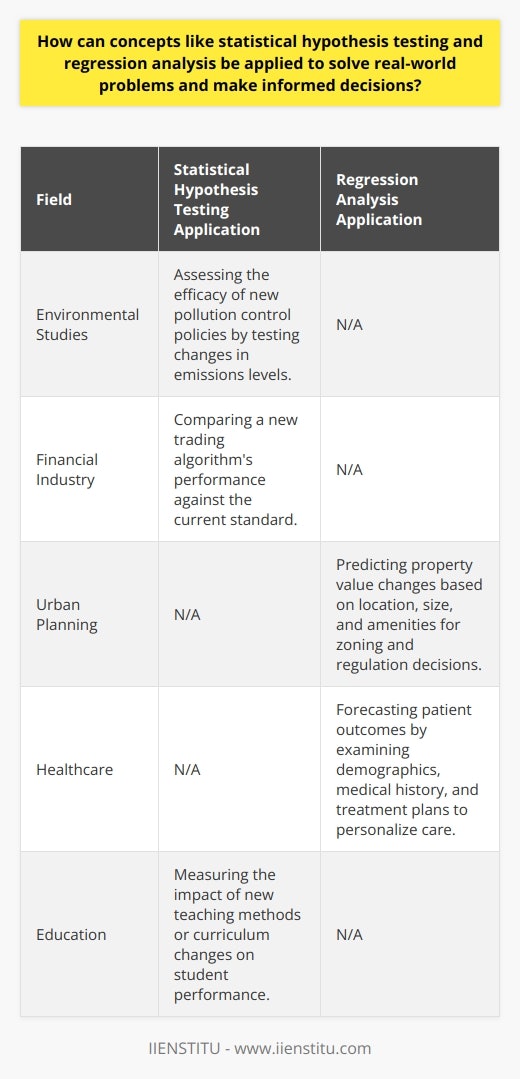
How does the use of descriptive and inferential statistics improve our understanding of complex problems and inform decision-making?
The Importance of Descriptive and Inferential Statistics in Problem Solving Descriptive statistics provide essential context Descriptive statistics summarize, organize, and simplify data, offering a comprehensive snapshot of a data set. By presenting data in a meaningful and easily interpretable manner, descriptive statistics enable researchers to understand and describe the key characteristics of a data set. This initial step in any data analysis is crucial for establishing context, identifying patterns, and generating hypotheses that contribute to a better understanding of complex problems. Inferential statistics as a tool for decision-making Inferential statistics, on the other hand, involve drawing conclusions and making generalizations about a larger population based on the analysis of a sample. Through hypothesis testing, confidence intervals, and regression analysis, researchers can determine relationships among variables, identify trends, and predict outcomes. By offering insights that go beyond the data at hand, inferential statistics enable researchers to make informed decisions and create strategies for tackling complex problems. The synergy of descriptive and inferential statistics In combination, both descriptive and inferential statistics enhance the understanding and decision-making process in various fields. Descriptive statistics provide a solid foundation by organizing and summarizing data, while inferential statistics enable researchers to delve deeper, uncovering relationships and trends that facilitate evidence-based decision-making. This combination empowers researchers to identify solutions and make more informed decisions when tackling complex problems.
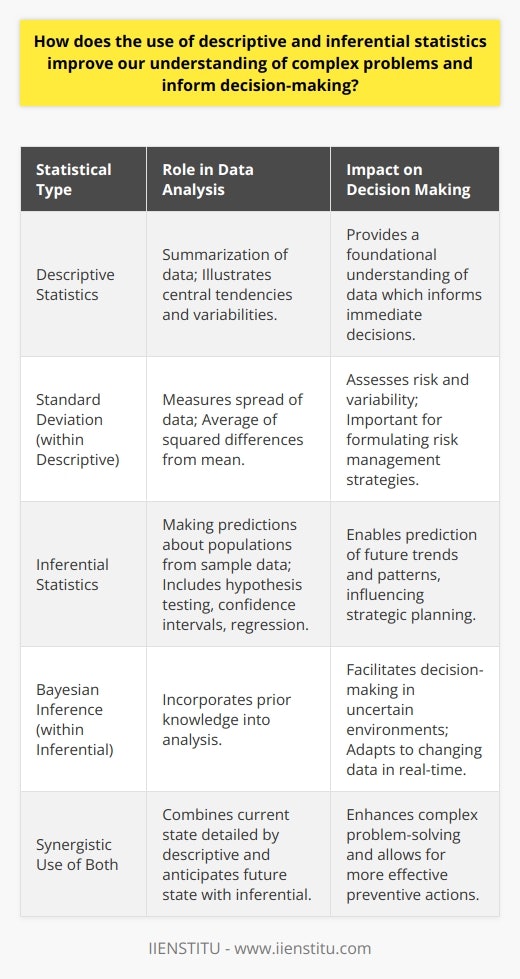
What is the role of experimental design and sampling techniques in ensuring reliable and accurate conclusions when utilizing statistical analysis for problem-solving?
Role of Experimental Design Experimental design plays a pivotal role in ensuring reliable and accurate conclusions in statistical analysis when solving problems. A well-defined experimental design outlines a systematic approach to conducting research, including the selection of participants, allocation of resources, and timing of interventions. It helps control potential confounding factors and biases, allowing researchers to attribute the study results to the intended interventions accurately. Moreover, experimental design enables researchers to quantify uncertainty in their findings through hypothesis testing, thereby establishing the statistical significance of their conclusions. Sampling Techniques Sampling techniques are another essential component in achieving valid and reliable results in statistical analysis. They ensure that the data collected from a population is representative of the whole, thus allowing for accurate generalizations. Proper sampling techniques, such as random sampling or stratified sampling, minimize the prevalence of sampling bias, which may otherwise lead to false or skewed conclusions. Additionally, determining the appropriate sample size—large enough to maintain statistical accuracy and minimize type I and type II errors—is crucial in enhancing the reliability and precision of study results. Achieving Accurate Conclusions To draw accurate conclusions in statistical analysis, researchers must ensure that their experimental design and sampling techniques are carefully planned and executed. This involves selecting the most appropriate methods in accordance with study goals and population demographics. Furthermore, vigilance regarding potential confounders and biases, and continuous monitoring of data quality, contribute to the validity and reliability of statistical findings for problem-solving. Overall, a skillful combination of experimental design and sampling techniques is imperative for researchers to derive reliable and accurate conclusions from statistical analysis. By addressing potential pitfalls and adhering to best practices, this potent mix of methodologies allows for efficient problem-solving and robust insights into diverse research questions.
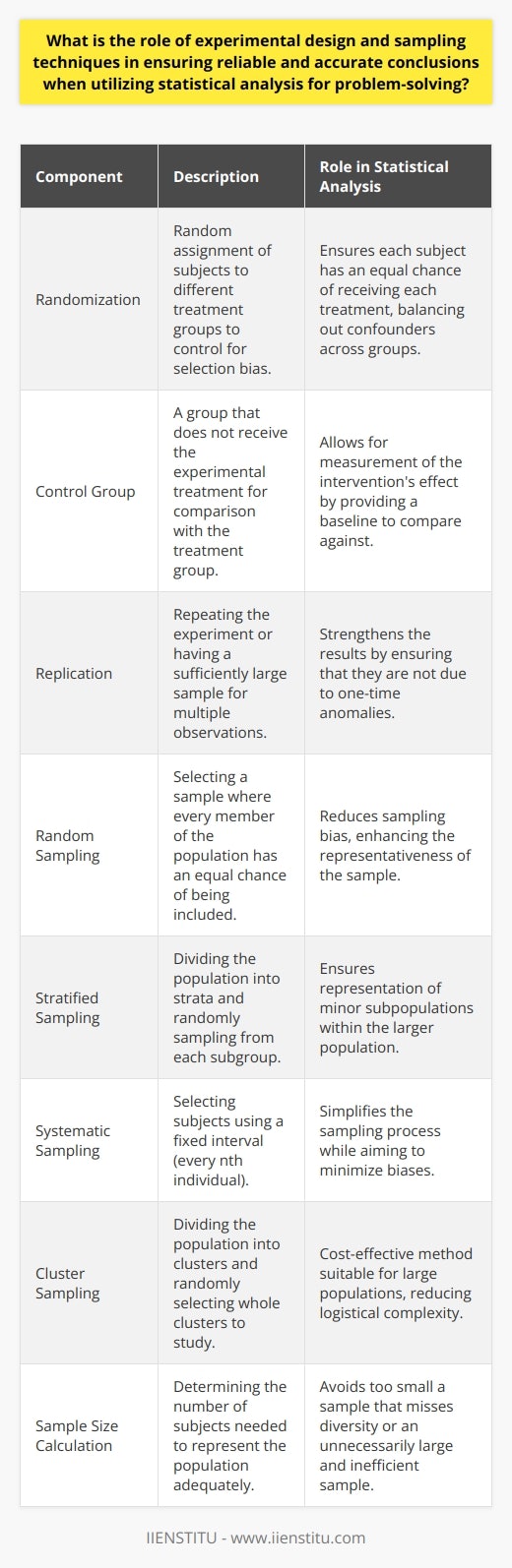
How do visualization techniques and exploratory data analysis contribute to a more effective interpretation of statistical findings in the context of real-world issues?
Enhancing Interpretation through Visualization Techniques Visualization techniques play a significant role in interpreting statistical findings related to real-world issues. By converting complex data into visually appealing and easy-to-understand formats, these techniques allow decision-makers to quickly grasp the underlying patterns and trends. Graphs, plots, and charts are some common visualization tools that make data more accessible, aiding in the identification of outliers and hidden relationships among variables. Exploratory Data Analysis: A Key Step Exploratory data analysis (EDA) is critical for effective interpretation of statistical findings. This approach involves an initial assessment of the data's characteristics, emphasizing summarizing and visualizing key aspects. Employing EDA allows researchers to identify errors, missing values, and inconsistencies in the data, which is instrumental when addressing real-world issues. By obtaining insights into the dataset's structure and potential biases, analysts can formulate appropriate statistical models and ensure more accurate predictions and inferences. Complementarity for Improved Data Interpretation Combining visualization techniques and EDA contributes to a more effective interpretation of statistical findings by offering a complementary approach. Visualization supports the exploration of data, enabling pattern and relationship identification, while EDA provides a deeper insight into data quality and potential limitations. Together, these methods facilitate a comprehensive understanding of the data, allowing for a more informed decision-making process when addressing real-world issues. In conclusion, visualization techniques and exploratory data analysis are essential tools for effectively interpreting statistical findings. By offering complementary benefits, they enhance decision-making processes and increase the likelihood of informed choices when examining real-world issues. As our world continues to produce vast amounts of data, these methods will remain critical to ensuring that statistical findings are accurate, relevant, and useful in solving pressing problems.
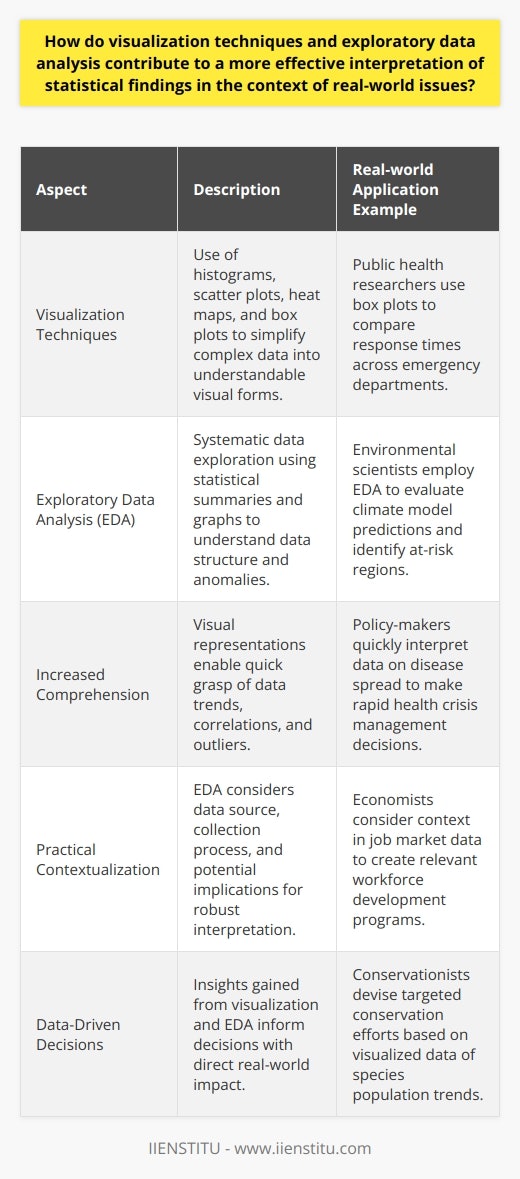
How does statistics help in problem-solving?
Role of Statistics in Problem-solving Understanding the Problem Statistics play a significant role in problem-solving by providing accurate data and quantitative evidence to better understand complex issues. The collection, analysis, and interpretation of numerical data enable decision-makers to observe trends, patterns, and relationships within the data, thus facilitating informed decision-making. To effectively solve problems, it is crucial to have a thorough understanding of the issue at hand, and statistics provide the necessary tools to explore and interpret the relevant data. Identifying Patterns and Trends Statistics help in identifying underlying patterns and trends within a dataset, which aids in understanding the problem's nature and behavior. By employing graphical and numerical techniques, statisticians can visualize relationships, fluctuations, and distributions within the data. Identifying these patterns can lead to the generation of hypotheses, proposing possible solutions, and implementing interventions to address the issues. Evaluating Solutions Once potential solutions are identified, statistics can be used to objectively evaluate their effectiveness by comparing the outcomes of different scenarios or interventions. Experimental designs such as controlled trials, surveys, and longitudinal studies are powerful tools for assessing the impact of problem-solving strategies. Furthermore, statistical significance testing allows decision-makers to determine the likelihood of results occurring by chance, providing more confidence in the selected solutions. Making Informed Decisions Through the use of statistical methods, decision-makers can be guided towards making more informed, evidence-based choices when solving problems. By basing decisions on empirical data, rather than relying on anecdotal evidence, intuition, or assumptions, organizations and policymakers can significantly improve the likelihood of producing successful outcomes. Statistical analysis enables the ranking of possible solutions according to their efficacy, which is crucial for resource allocation and prioritization within any setting. In conclusion, statistics play a crucial role in problem-solving by providing a systematic and rigorous approach to understanding complex issues, identifying patterns and trends, evaluating potential solutions, and guiding informed decision-making. The use of quantitative data and statistical methods allows for greater objectivity, accuracy, and confidence in the process of solving problems and ultimately leads to more effective and efficient solutions.
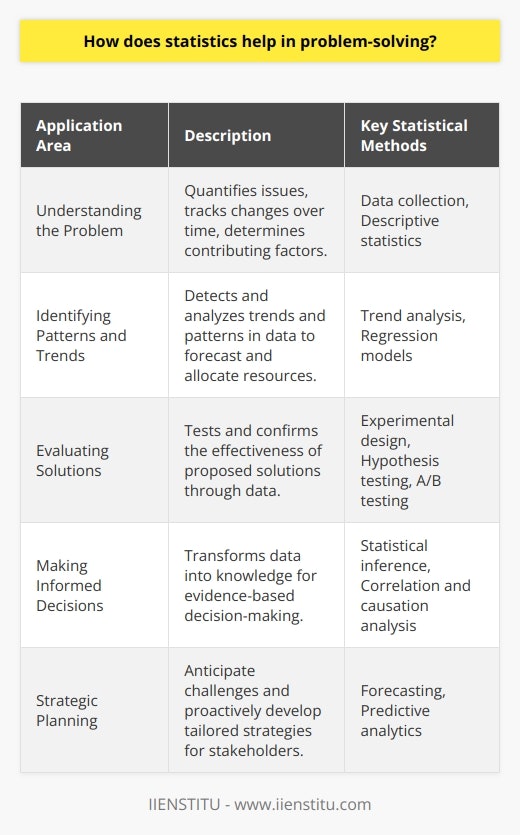
What are the five statistical processes in solving a problem?
Statistical Processes Overview The process of solving a problem using statistical methods involves five key steps. These steps enable researchers to analyze data and make inferences based on the results. 1. Defining the Problem The first step in any statistical problem-solving process is to clearly define the problem. This involves identifying the research question, objective, or hypothesis that needs to be tested. The problem should be specific and clearly stated to guide the subsequent steps in the process. 2. Data Collection Once the problem is defined, the next step is to collect data that will be used for analysis. Data can be collected through various methods, such as surveys, experiments, or secondary sources. The choice of data collection method should be based on the nature of the problem and the type of data required. It is important to collect data accurately and consistently to ensure the validity of the analysis. 3. Data Organization and Summarization After collecting the data, it needs to be organized and summarized in a way that makes it easy to analyze. This may involve using tables, graphs, or charts to display the data. Descriptive statistics, such as measures of central tendency (mean, median, mode) and measures of dispersion (range, variance, standard deviation), can be used to summarize the data. 4. Analysis and Interpretation At this stage, the data is analyzed using various statistical techniques to answer the research question or test the hypothesis. Inferential statistics, such as correlation analysis or hypothesis testing, can be employed to make inferences about the underlying population based on the sample data. It is crucial to choose the appropriate statistical method for the analysis, keeping in mind the research question and the nature of the data. 5. Drawing Conclusions and Recommendations The final step in the statistical process is to draw conclusions from the analysis and provide recommendations based on the findings. This involves interpreting the results of the analysis in the context of the research question and making generalizations or predictions about the population. The conclusions and recommendations should be communicated effectively, ensuring that they are relevant and useful for decision-making or further research. In conclusion, the five statistical processes in solving a problem are defining the problem, data collection, data organization and summarization, analysis and interpretation, and drawing conclusions and recommendations. These steps allow researchers to effectively analyze data and make informed decisions and predictions based on the results.
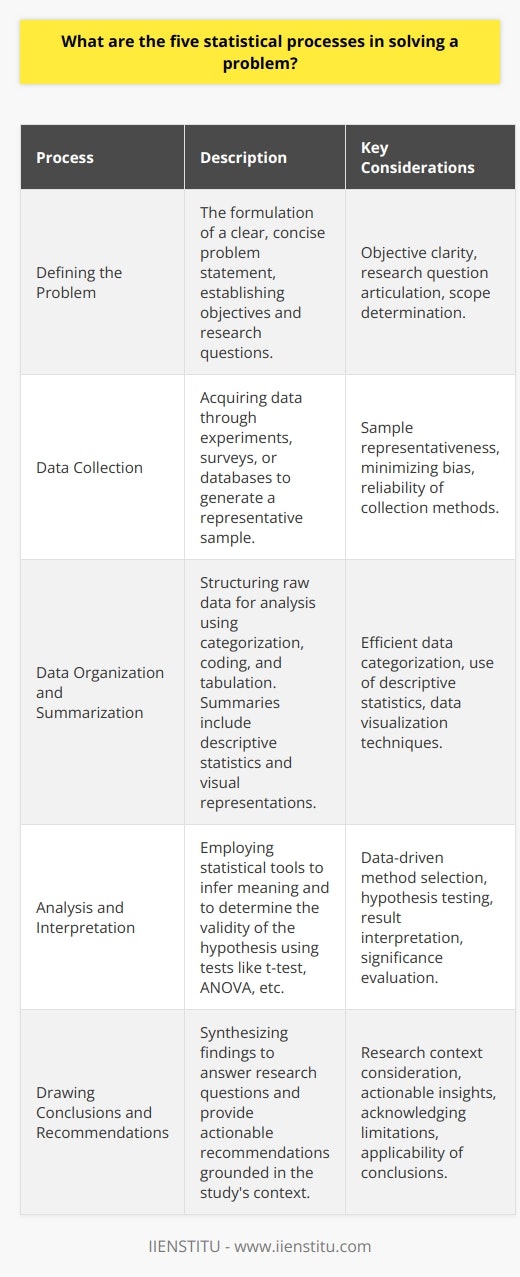
How can you use statistics effectively to resolve problems in everyday life?
Understanding the Basics of Statistics Statistics provides a systematic method for individuals to collect, analyze and interpret data. Through this approach, one can efficiently utilize these results to tackle issues they may encounter daily. In the ensuing discussion, we will delve into the process of incorporating statistics to address these everyday concerns effectively. Identifying the Problem Firstly, it is essential to accurately outline the issue at hand. This preliminary stage entails formulating definitive questions, which will guide the data gathering process. Such specificity ensures the assembled information directly pertains to the focal problem and eliminates the possibility of superfluous distractions. Collecting Relevant Data Next, amassing reliable and diverse information allows for well-informed interpretations. To successfully achieve this, it is crucial to identify suitable sources that offer the pertinent data required for a comprehensive analysis. Moreover, obtaining data from diverse sources helps mitigate the potential for biased or skewed outcomes. Implementing Appropriate Statistical Techniques Upon compiling a robust dataset, the implementation of applicable statistical methods becomes crucial. Techniques such as descriptive statistics (e.g., mean, median, mode) or inferential statistics (e.g., regression, ANOVA) empower individuals to systematically extract informative conclusions. Ultimately, this data-driven process leads to a deeper understanding of the issue at hand and facilitates informed decision-making. Interpreting Results and Drawing Conclusions The final step involves rigorously assessing the conclusions derived from statistical analyses. This careful evaluation demands a thorough examination of any potential limitations or biases. Additionally, acknowledging alternative interpretations strengthens the overall argument by mitigating the risk of oversimplifying complex matters. Incorporating Feedback and Adjustments A critical aspect of effectively applying statistics revolves around the willingness to reevaluate one's approach. Engaging in an iterative process and incorporating feedback helps refine the problem-solving strategy, ultimately leading to more accurate and reliable solutions. In summary, the proper use of statistics has the potential to greatly enhance individuals' ability to resolve problems in everyday life. By employing a methodical approach that involves identifying the issue, collecting relevant data, utilizing suitable techniques and critically evaluating conclusions, one can swiftly address concerns and make informed decisions.
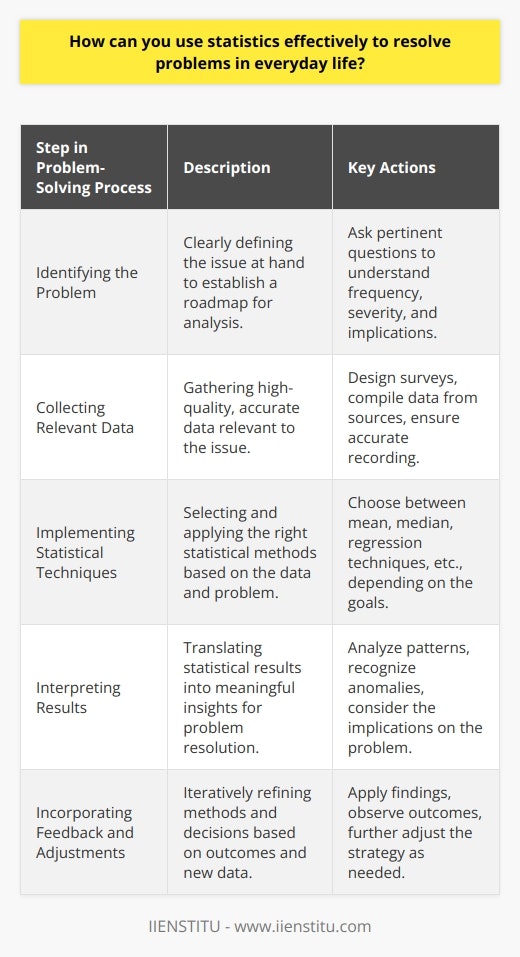
How can statistical inference be utilized to draw conclusions about a population when only a sample is available for analysis?
Statistical Inference and Population Analysis Statistical inference is an essential tool in understanding populations. It allows scientists to analyze a small, representative subset or sample of a larger population. This way, we can extract conclusions about an entire population from the analysis of a sample. Use of Sample Analysis In sample analysis, researchers collect data from a smaller subset instead of assessing the entire population. It significantly reduces the required resources and time. Nevertheless, a sample must adequately represent the characteristics of the population for valid inferences. Role of Probability Probability plays a pivotal role in statistical inference. The application of probability theories provides information about the likelihood of particular results. The conclusions drawn about the population feature a degree of certainty conveyed by probability. Statistical Tests Stepping further, statistical tests employed in the process illuminate the differences between groups within the sample. They provide guidelines for finding if observed differences occurred due to chance. By employing these tests, we can generalize findings from a sample to the entire population. Importance of Confidence Intervals Confidence intervals are another critical component of statistical inference. They present the range of values within which we expect the population value to fall a certain percent of the time, say 95%. Confidence intervals reveal more about the population parameter than a single point estimate. Conclusion and Future Predictions Between sample analysis, probability, statistical tests, and confidence intervals, statistical inference enables efficient, accurate conclusions about large population groups. Its effective use facilitates not only a comprehensive understanding of the present population status but also assists in predicting future trends. In a nutshell, statistical inference acts as a bridge connecting sample data to meaningful conclusions about the broader population. By analyzing a sample, predicting probabilities, applying statistical tests, and measuring confidence intervals, we can glean holistic insights about the entire population.
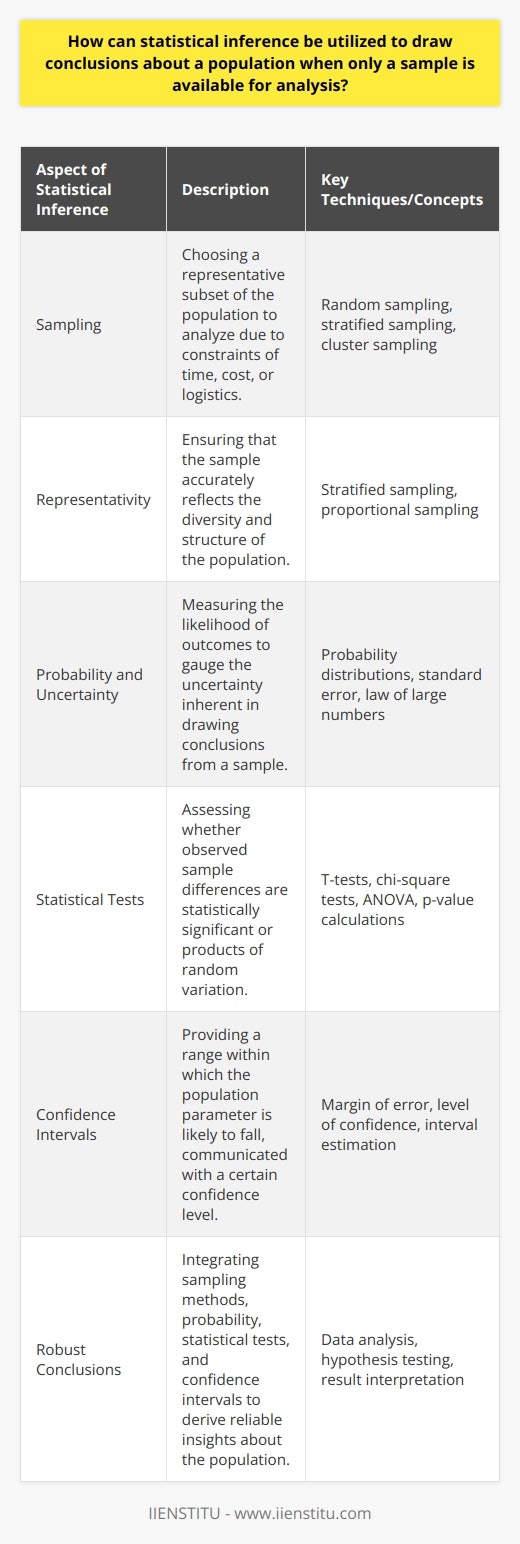
What are the key principles of robust statistical modeling, and how can these principles be applied to enhance the effectiveness of problem-solving efforts?
Understanding Robust Statistical Modeling Principles Robust statistical modeling works on three key principles. They are the use of robust measures, an effective model selection strategy, and consideration of outliers. These principles play a crucial role to ensure the robustness of statistical results. Applying Robust Measures The first principle revolves around applying robust measures. These measures are resistant to the outliers in the data set. They work by minimizing the effect of extreme values. By using these robust measures, researchers can increase the accuracy of their statistical models. Model Selection Strategy Next comes the strategy for selecting the model. It involves choosing an appropriate statistical model that aligns well with the provided data set. In this case, the most reliable models are ones that demonstrate significant results and fit the data well. Selecting an efficient model, hence, can lead to more accurate predictions or inferences. Addressing Outliers Finally, a detailed consideration of outliers is vital. Outliers can skew the results of a model significantly. They need careful handling to prevent any bias in the final results. Recognizing and appropriately managing these outliers aids in maintaining the integrity of statistical findings. Enhancing Problem-Solving Efforts These principles, when applied effectively, can significantly enhance problem-solving efforts. By using robust measures, researchers can achieve more accurate results, increasing the credibility of their findings. A well-chosen model can enhance the interpretability and usefulness of the results. Furthermore, careful handling of outliers can prevent skewed results, ensuring more reliable conclusions. In essence, by embracing these principles, one can substantially elevate their problem-solving capabilities, making the process more efficient and effective. Thus, robust statistical modeling acts as a powerful tool in addressing various research questions and solving complex problems.
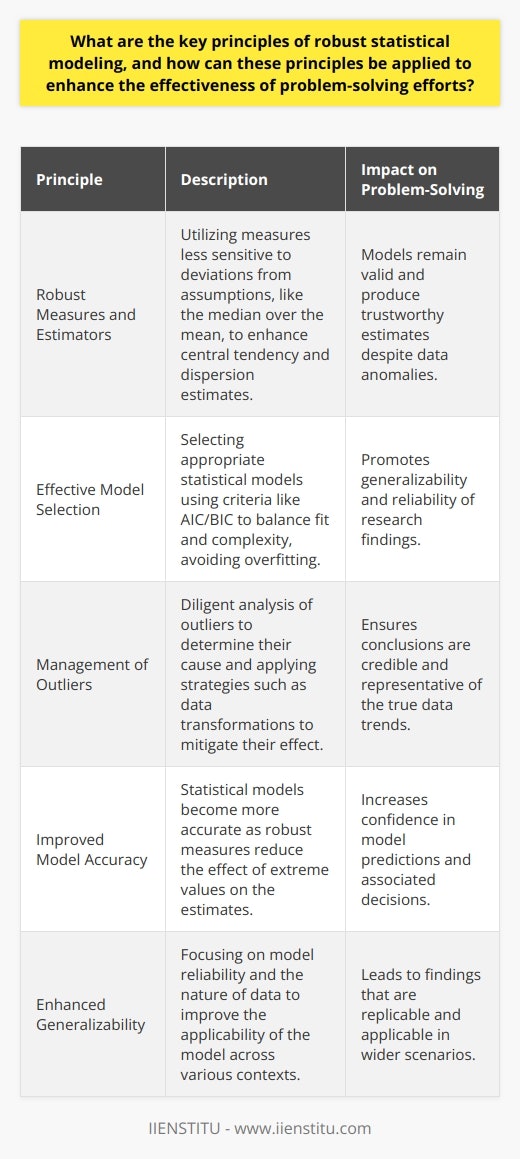
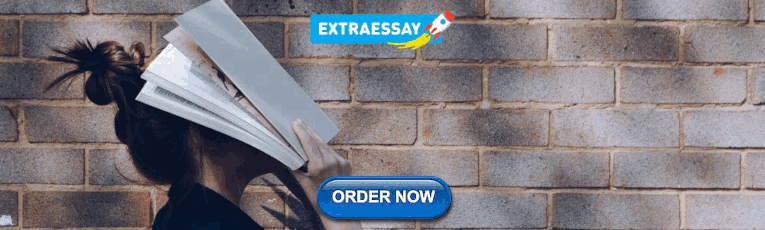
How can the utilizations of time series analysis in statistics support trend identification and forecasting in the context of complex/problem-solving situations?
Identifying Trends with Time Series Analysis: A crucial aspect of time series analysis in statistics is trend identification. Time series analysis allows statisticians to discern patterns in data collected over time. These trends indicate changes in variables, creating a historical line that tracks these alterations across a span of time. Support for Complex Problem Solving: In complex problem-solving situations, time series analysis can provide valuable support. Specifically, it can facilitate independent, variable-dependent trend analysis and insights into relationships within data sequences. This is vital for complex situations requiring deeper analysis. Time Series Analysis for Forecasting: Another primary use of time series analysis is for forecast predictions in future scenarios. By analyzing the trends identified, predictions can suggest plausible future scenarios. This forecasting capability can be critical in planning and preparation for potential future events based on the observed trends. Predictive Modeling: Predictive modeling can be improved with time series analysis. It helps understand population trends or related metrics. By revealing underlying patterns, time series analysis supports data-driven decision making in complex situations. In summary, time series analysis plays an instrumental role in statistics. Through trend identification and forecasting, it provides invaluable support for complex problem-solving situations. This statistical tool is essential for those working in an environment that requires a clear, predictive understanding of data over time.
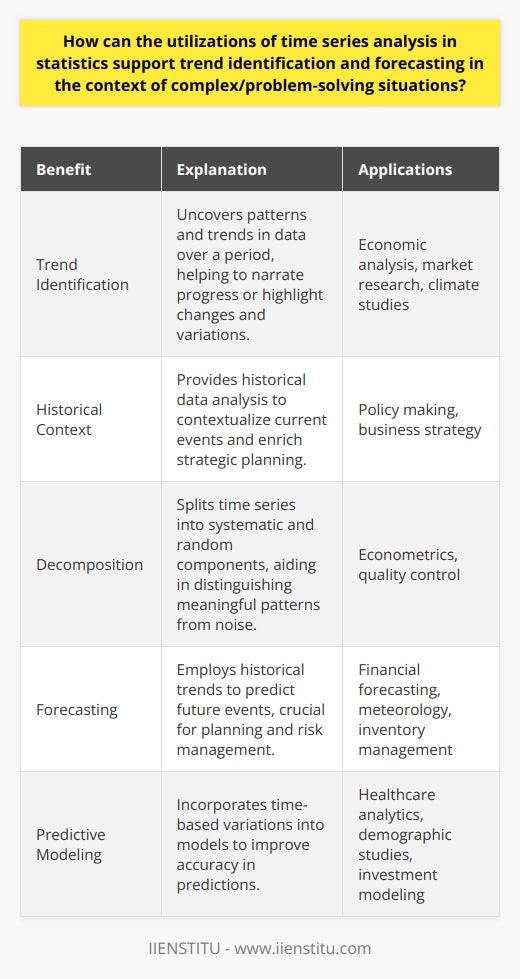
How can statistics help with problem solving?
Effective Use of Statistics Statistics offers efficient problem-solving tools. They provide the ability to measure, forecast, and make informed decisions. When faced with a problem, statistics help in gathering relevant data. Understanding the Problem Statistics helps to describe the problem objectively. Before proceeding with problem solving, a clear definition of the problem is necessary. Statistics describe problems quantitatively, bringing precision in problem definition. Identifying Solutions Statistics aids in identifying potential solutions. By using predictive analytics, statistics can forecast the outcomes of various solutions. Thus, it assists in the selection of most efficient solution based on the forecasted results. Evaluating Results Once a solution is implemented, statistics help in evaluation. They measure the effectiveness of the solution by comparing the outcomes with the predicted results. Promoting Continuous Improvement Statistics guide continuous improvement. They pinpoint deviations, enabling identification of areas of improvement. This leads to enhanced effectiveness in problem solving. Statistics has a pivotal role in problem solving. The data-driven approach enhances the credibility of the problem-solving process and the ultimate solutions. The various statistical tools improve both the efficiency and effectiveness, leading to better solutions.
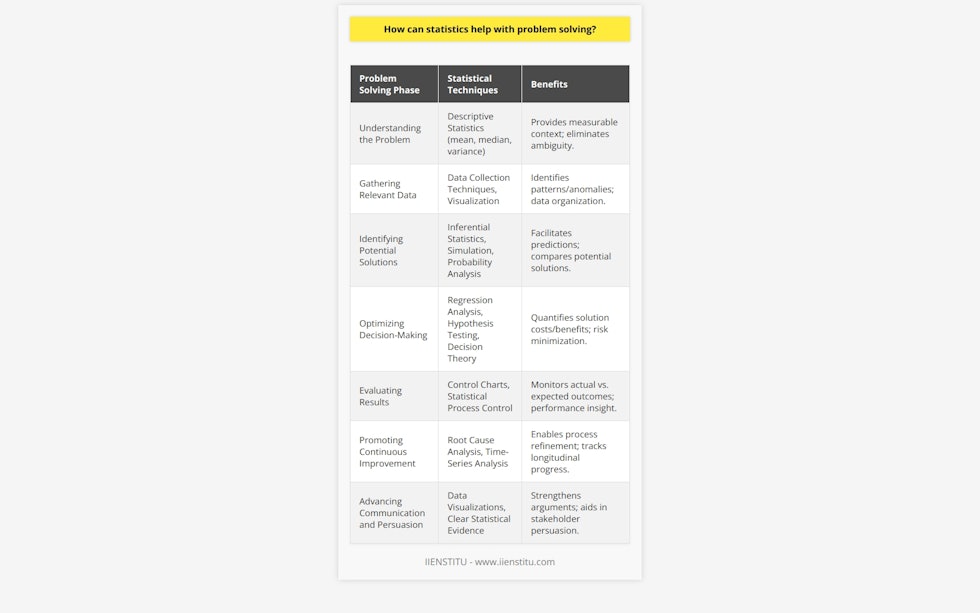
Why is data analysis important in problem solving?
Data Analysis and Problem-Solving: A Crucial Connection Data analysis stands as a critical tool in problem solving in the contemporary business environment. Essentially, it offers insightful measurements of challenges. By examining data, we uncover patterns and trends to identify problems. Identification of Issues The initial step in problem-solving involves the recognition of a problem. It is here that data analysis proves vital. It grants a robust basis for this recognition, presenting objective rather than subjective identifiers. Understanding the Nature of Problems Once we identify a problem, we must understand its nature. In-depth data analysis can provide a detailed insight into why problems arise. It examines multiple variable relationships, often revealing root causes. Generating Solutions Data analysis aids in creating suitable solutions. By understanding the problem from a data perspective, we can draw up potential fixes. These solutions are often grounded on empirical evidence, hence sound and reliable. Evaluating Outcomes After solution implementation, evaluation follows closely. Analyzing data post-implementation helps measure the effectiveness of the solution. It provides a measure on the success of the problem-solving process. In conclusion, data analysis is a strong ally in problem-solving. It facilitates issue identification, enhances understanding, helps to generate solutions, and evaluates outcomes. By utilizing this tool, we can significantly improve our problem-solving efforts, leading to more effective and measurable results.
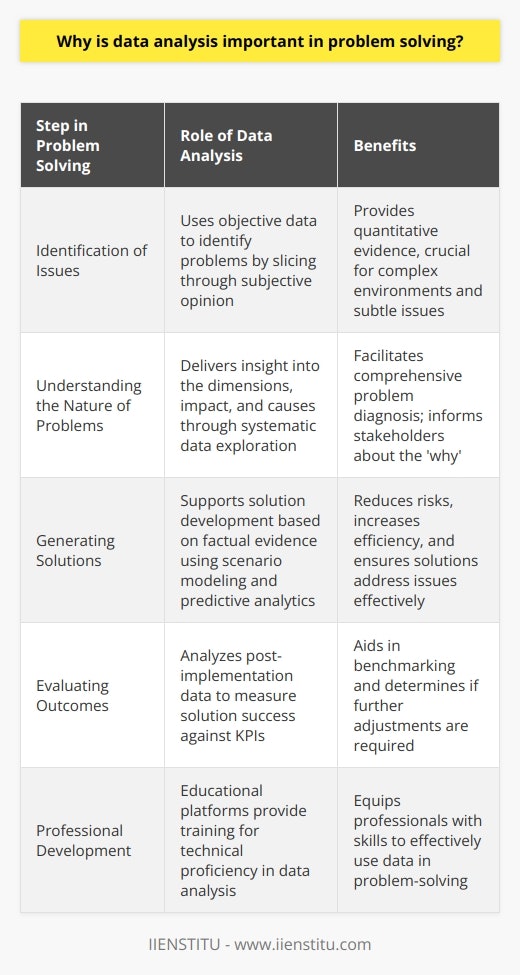
How does statistics make you a better thinker?
Enhancing Reasoning and Decision Making Skills Statistics equips one with necessary tools to question and interpret data intelligently. It sharpens critical reasoning abilities by offering ways to identify patterns or anomalies, thus improving decision-making efficiency. Understanding Probabilities and Predictions Statistics introduces individuals to the concept of probability, enabling them to weigh the likelihood of different scenarios accurately. Consequently, it allows them to make precise and informed predictions, honing their thinking and analytical skills. Building Quantitative Literacy Statistics promotes quantitative literacy, a vital skill in a data-driven world. Understanding numerical information helps individuals decipher complex data and convert it into actionable insights. This heightens critical thinking abilities and enables better understanding of the world. Critiquing Data Effectively Statistics improves a person's ability to critically analyze presented data. Using statistical tools, one can identify manipulation or misinterpretation in data, preventing them from taking misleading information at face value. Developing Logical Reasoning Statistics fosters effective problem-solving skills by inciting logical reasoning. It drives individuals to meticulously analyze data, look for patterns and draw logical conclusions, thus streamlining strategic decision-making processes. In conclusion, mastering the use of statistics can effectively enhance a person's thinking capacity. It works on multiple fronts ranging from decision-making to quantitative literacy to critiquing data, making one a more discerning and astute individual. Statistics, therefore, plays a pivotal role in developing vital cognitive abilities.
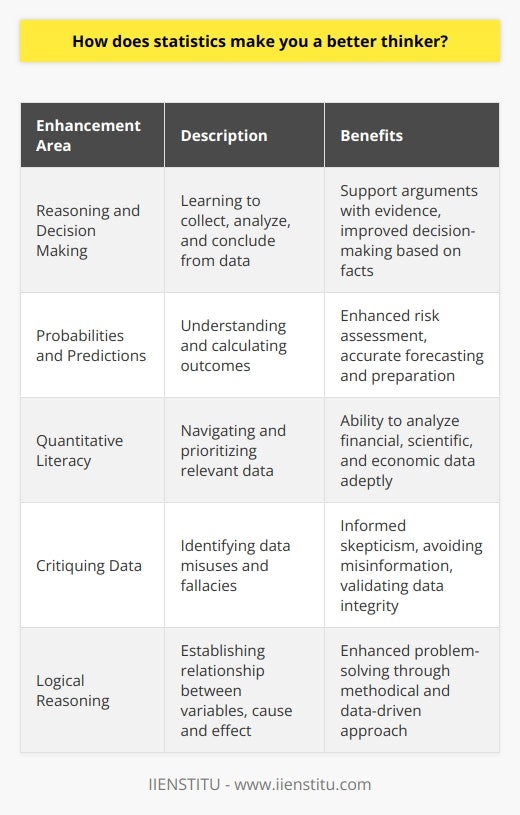
Yu Payne is an American professional who believes in personal growth. After studying The Art & Science of Transformational from Erickson College, she continuously seeks out new trainings to improve herself. She has been producing content for the IIENSTITU Blog since 2021. Her work has been featured on various platforms, including but not limited to: ThriveGlobal, TinyBuddha, and Addicted2Success. Yu aspires to help others reach their full potential and live their best lives.
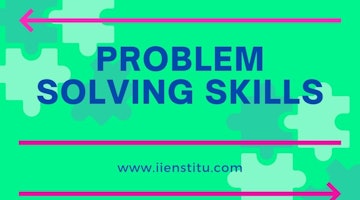
What are Problem Solving Skills?
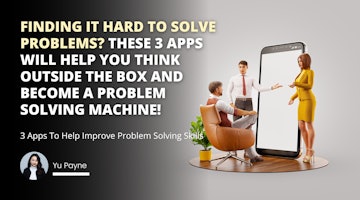
3 Apps To Help Improve Problem Solving Skills
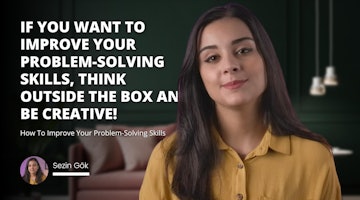
How To Improve Your Problem-Solving Skills
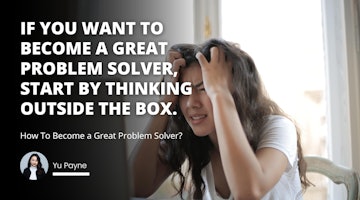
How To Become a Great Problem Solver?
Have a language expert improve your writing
Run a free plagiarism check in 10 minutes, generate accurate citations for free.
- Knowledge Base
Hypothesis Testing | A Step-by-Step Guide with Easy Examples
Published on November 8, 2019 by Rebecca Bevans . Revised on June 22, 2023.
Hypothesis testing is a formal procedure for investigating our ideas about the world using statistics . It is most often used by scientists to test specific predictions, called hypotheses, that arise from theories.
There are 5 main steps in hypothesis testing:
- State your research hypothesis as a null hypothesis and alternate hypothesis (H o ) and (H a or H 1 ).
- Collect data in a way designed to test the hypothesis.
- Perform an appropriate statistical test .
- Decide whether to reject or fail to reject your null hypothesis.
- Present the findings in your results and discussion section.
Though the specific details might vary, the procedure you will use when testing a hypothesis will always follow some version of these steps.
Table of contents
Step 1: state your null and alternate hypothesis, step 2: collect data, step 3: perform a statistical test, step 4: decide whether to reject or fail to reject your null hypothesis, step 5: present your findings, other interesting articles, frequently asked questions about hypothesis testing.
After developing your initial research hypothesis (the prediction that you want to investigate), it is important to restate it as a null (H o ) and alternate (H a ) hypothesis so that you can test it mathematically.
The alternate hypothesis is usually your initial hypothesis that predicts a relationship between variables. The null hypothesis is a prediction of no relationship between the variables you are interested in.
- H 0 : Men are, on average, not taller than women. H a : Men are, on average, taller than women.
Here's why students love Scribbr's proofreading services
Discover proofreading & editing
For a statistical test to be valid , it is important to perform sampling and collect data in a way that is designed to test your hypothesis. If your data are not representative, then you cannot make statistical inferences about the population you are interested in.
There are a variety of statistical tests available, but they are all based on the comparison of within-group variance (how spread out the data is within a category) versus between-group variance (how different the categories are from one another).
If the between-group variance is large enough that there is little or no overlap between groups, then your statistical test will reflect that by showing a low p -value . This means it is unlikely that the differences between these groups came about by chance.
Alternatively, if there is high within-group variance and low between-group variance, then your statistical test will reflect that with a high p -value. This means it is likely that any difference you measure between groups is due to chance.
Your choice of statistical test will be based on the type of variables and the level of measurement of your collected data .
- an estimate of the difference in average height between the two groups.
- a p -value showing how likely you are to see this difference if the null hypothesis of no difference is true.
Based on the outcome of your statistical test, you will have to decide whether to reject or fail to reject your null hypothesis.
In most cases you will use the p -value generated by your statistical test to guide your decision. And in most cases, your predetermined level of significance for rejecting the null hypothesis will be 0.05 – that is, when there is a less than 5% chance that you would see these results if the null hypothesis were true.
In some cases, researchers choose a more conservative level of significance, such as 0.01 (1%). This minimizes the risk of incorrectly rejecting the null hypothesis ( Type I error ).
Receive feedback on language, structure, and formatting
Professional editors proofread and edit your paper by focusing on:
- Academic style
- Vague sentences
- Style consistency
See an example
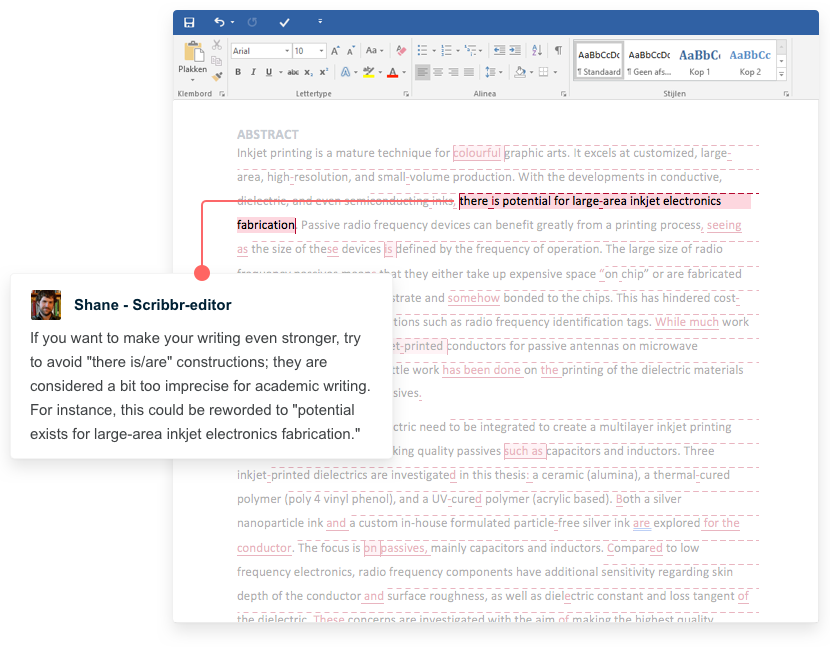
The results of hypothesis testing will be presented in the results and discussion sections of your research paper , dissertation or thesis .
In the results section you should give a brief summary of the data and a summary of the results of your statistical test (for example, the estimated difference between group means and associated p -value). In the discussion , you can discuss whether your initial hypothesis was supported by your results or not.
In the formal language of hypothesis testing, we talk about rejecting or failing to reject the null hypothesis. You will probably be asked to do this in your statistics assignments.
However, when presenting research results in academic papers we rarely talk this way. Instead, we go back to our alternate hypothesis (in this case, the hypothesis that men are on average taller than women) and state whether the result of our test did or did not support the alternate hypothesis.
If your null hypothesis was rejected, this result is interpreted as “supported the alternate hypothesis.”
These are superficial differences; you can see that they mean the same thing.
You might notice that we don’t say that we reject or fail to reject the alternate hypothesis . This is because hypothesis testing is not designed to prove or disprove anything. It is only designed to test whether a pattern we measure could have arisen spuriously, or by chance.
If we reject the null hypothesis based on our research (i.e., we find that it is unlikely that the pattern arose by chance), then we can say our test lends support to our hypothesis . But if the pattern does not pass our decision rule, meaning that it could have arisen by chance, then we say the test is inconsistent with our hypothesis .
If you want to know more about statistics , methodology , or research bias , make sure to check out some of our other articles with explanations and examples.
- Normal distribution
- Descriptive statistics
- Measures of central tendency
- Correlation coefficient
Methodology
- Cluster sampling
- Stratified sampling
- Types of interviews
- Cohort study
- Thematic analysis
Research bias
- Implicit bias
- Cognitive bias
- Survivorship bias
- Availability heuristic
- Nonresponse bias
- Regression to the mean
Hypothesis testing is a formal procedure for investigating our ideas about the world using statistics. It is used by scientists to test specific predictions, called hypotheses , by calculating how likely it is that a pattern or relationship between variables could have arisen by chance.
A hypothesis states your predictions about what your research will find. It is a tentative answer to your research question that has not yet been tested. For some research projects, you might have to write several hypotheses that address different aspects of your research question.
A hypothesis is not just a guess — it should be based on existing theories and knowledge. It also has to be testable, which means you can support or refute it through scientific research methods (such as experiments, observations and statistical analysis of data).
Null and alternative hypotheses are used in statistical hypothesis testing . The null hypothesis of a test always predicts no effect or no relationship between variables, while the alternative hypothesis states your research prediction of an effect or relationship.
Cite this Scribbr article
If you want to cite this source, you can copy and paste the citation or click the “Cite this Scribbr article” button to automatically add the citation to our free Citation Generator.
Bevans, R. (2023, June 22). Hypothesis Testing | A Step-by-Step Guide with Easy Examples. Scribbr. Retrieved August 10, 2024, from https://www.scribbr.com/statistics/hypothesis-testing/
Is this article helpful?
Rebecca Bevans
Other students also liked, choosing the right statistical test | types & examples, understanding p values | definition and examples, what is your plagiarism score.
- Skip to main content
- Skip to primary sidebar
- Skip to footer
Additional menu

The 5 steps of the solving problem process
August 17, 2023 by MindManager Blog
Whether you run a business, manage a team, or work in an industry where change is the norm, it may feel like something is always going wrong. Thankfully, becoming proficient in the problem solving process can alleviate a great deal of the stress that business issues can create.
Understanding the right way to solve problems not only takes the guesswork out of how to deal with difficult, unexpected, or complex situations, it can lead to more effective long-term solutions.
In this article, we’ll walk you through the 5 steps of problem solving, and help you explore a few examples of problem solving scenarios where you can see the problem solving process in action before putting it to work.
Understanding the problem solving process
When something isn’t working, it’s important to understand what’s at the root of the problem so you can fix it and prevent it from happening again. That’s why resolving difficult or complex issues works best when you apply proven business problem solving tools and techniques – from soft skills, to software.
The problem solving process typically includes:
- Pinpointing what’s broken by gathering data and consulting with team members.
- Figuring out why it’s not working by mapping out and troubleshooting the problem.
- Deciding on the most effective way to fix it by brainstorming and then implementing a solution.
While skills like active listening, collaboration, and leadership play an important role in problem solving, tools like visual mapping software make it easier to define and share problem solving objectives, play out various solutions, and even put the best fit to work.
Before you can take your first step toward solving a problem, you need to have a clear idea of what the issue is and the outcome you want to achieve by resolving it.
For example, if your company currently manufactures 50 widgets a day, but you’ve started processing orders for 75 widgets a day, you could simply say you have a production deficit.
However, the problem solving process will prove far more valuable if you define the start and end point by clarifying that production is running short by 25 widgets a day, and you need to increase daily production by 50%.
Once you know where you’re at and where you need to end up, these five steps will take you from Point A to Point B:
- Figure out what’s causing the problem . You may need to gather knowledge and evaluate input from different documents, departments, and personnel to isolate the factors that are contributing to your problem. Knowledge visualization software like MindManager can help.
- Come up with a few viable solutions . Since hitting on exactly the right solution – right away – can be tough, brainstorming with your team and mapping out various scenarios is the best way to move forward. If your first strategy doesn’t pan out, you’ll have others on tap you can turn to.
- Choose the best option . Decision-making skills, and software that lets you lay out process relationships, priorities, and criteria, are invaluable for selecting the most promising solution. Whether it’s you or someone higher up making that choice, it should include weighing costs, time commitments, and any implementation hurdles.
- Put your chosen solution to work . Before implementing your fix of choice, you should make key personnel aware of changes that might affect their daily workflow, and set up benchmarks that will make it easy to see if your solution is working.
- Evaluate your outcome . Now comes the moment of truth: did the solution you implemented solve your problem? Do your benchmarks show you achieved the outcome you wanted? If so, congratulations! If not, you’ll need to tweak your solution to meet your problem solving goal.
In practice, you might not hit a home-run with every solution you execute. But the beauty of a repeatable process like problem solving is that you can carry out steps 4 and 5 again by drawing from the brainstorm options you documented during step 2.
Examples of problem solving scenarios
The best way to get a sense of how the problem solving process works before you try it for yourself is to work through some simple scenarios.
Here are three examples of how you can apply business problem solving techniques to common workplace challenges.
Scenario #1: Manufacturing
Building on our original manufacturing example, you determine that your company is consistently short producing 25 widgets a day and needs to increase daily production by 50%.
Since you’d like to gather data and input from both your manufacturing and sales order departments, you schedule a brainstorming session to discover the root cause of the shortage.
After examining four key production areas – machines, materials, methods, and management – you determine the cause of the problem: the material used to manufacture your widgets can only be fed into your equipment once the machinery warms up to a specific temperature for the day.
Your team comes up with three possible solutions.
- Leave your machinery running 24 hours so it’s always at temperature.
- Invest in equipment that heats up faster.
- Find an alternate material for your widgets.
After weighing the expense of the first two solutions, and conducting some online research, you decide that switching to a comparable but less expensive material that can be worked at a lower temperature is your best option.
You implement your plan, monitor your widget quality and output over the following week, and declare your solution a success when daily production increases by 100%.
Scenario #2: Service Delivery
Business training is booming and you’ve had to onboard new staff over the past month. Now you learn that several clients have expressed concern about the quality of your recent training sessions.
After speaking with both clients and staff, you discover there are actually two distinct factors contributing to your quality problem:
- The additional conference room you’ve leased to accommodate your expanding training sessions has terrible acoustics
- The AV equipment you’ve purchased to accommodate your expanding workforce is on back-order – and your new hires have been making do without
You could look for a new conference room or re-schedule upcoming training sessions until after your new equipment arrives. But your team collaboratively determines that the best way to mitigate both issues at once is by temporarily renting the high-quality sound and visual system they need.
Using benchmarks that include several weeks of feedback from session attendees, and random session spot-checks you conduct personally, you conclude the solution has worked.
Scenario #3: Marketing
You’ve invested heavily in product marketing, but still can’t meet your sales goals. Specifically, you missed your revenue target by 30% last year and would like to meet that same target this year.
After collecting and examining reams of information from your sales and accounting departments, you sit down with your marketing team to figure out what’s hindering your success in the marketplace.
Determining that your product isn’t competitively priced, you map out two viable solutions.
- Hire a third-party specialist to conduct a detailed market analysis.
- Drop the price of your product to undercut competitors.
Since you’re in a hurry for results, you decide to immediately reduce the price of your product and market it accordingly.
When revenue figures for the following quarter show sales have declined even further – and marketing surveys show potential customers are doubting the quality of your product – you revert back to your original pricing, revisit your problem solving process, and implement the market analysis solution instead.
With the valuable information you gain, you finally arrive at just the right product price for your target market and sales begin to pick up. Although you miss your revenue target again this year, you meet it by the second quarter of the following year.
Kickstart your collaborative brainstorming sessions and try MindManager for free today !
Ready to take the next step?
MindManager helps boost collaboration and productivity among remote and hybrid teams to achieve better results, faster.

Why choose MindManager?
MindManager® helps individuals, teams, and enterprises bring greater clarity and structure to plans, projects, and processes. It provides visual productivity tools and mind mapping software to help take you and your organization to where you want to be.
Explore MindManager
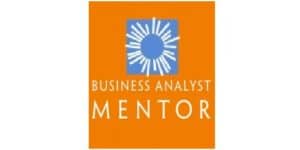
The 5 Steps in Problem Analysis
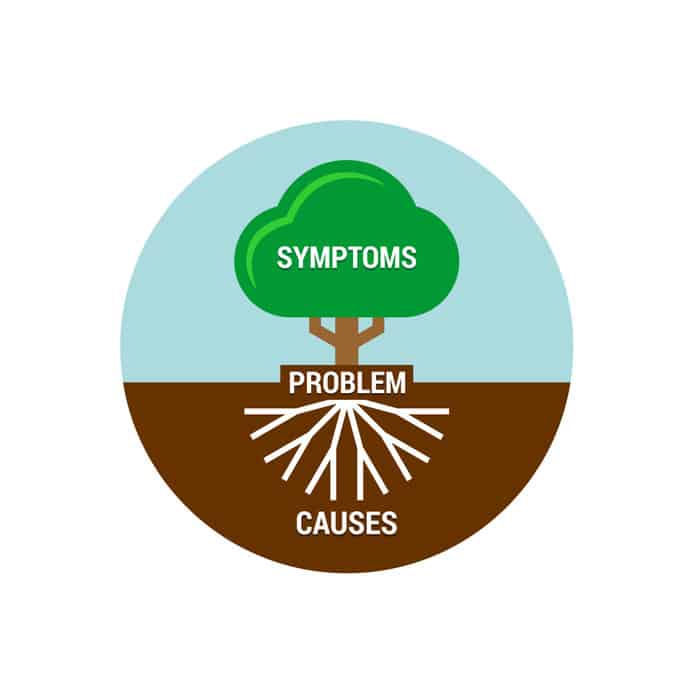
One technique that is extremely useful to gain a better understanding of the problems before determining a solution is problem analysis .
Problem analysis is the process of understanding real-world problems and user’s needs and proposing solutions to meet those needs. The goal of problem analysis is to gain a better understanding of the problem being solved before developing a solution.
There are five useful steps that can be taken to gain a better understanding of the problem before developing a solution.
- Gain agreement on the problem definition
- Understand the root-causes – the problem behind the problem
- Identify the stakeholders and the users
- Define the solution boundary
- Identify the constraints to be imposed on the solution
Table of Contents
Gain agreement on the problem definition.
The first step is to gain agreement on the definition of the problem to be solved. One of the simplest ways to gain agreement is to simply write the problem down and see whether everyone agrees.
Business Problem Statement Template

A helpful and standardised format to write the problem definition is as follows:
- The problem of – Describe the problem
- Affects – Identify stakeholders affected by the problem
- The results of which – Describe the impact of this problem on stakeholders and business activity
- Benefits of – Indicate the proposed solution and list a few key benefits
Example Business Problem Statement
There are many problems statement examples that can be found in different business domains and during the discovery when the business analyst is conducting analysis. An example business problem statement is as follows:
The problem of having to manually maintain an accurate single source of truth for finance product data across the business, affects the finance department. The results of which has the impact of not having to have duplicate data, having to do workarounds and difficulty of maintaining finance product data across the business and key channels. A successful solution would have the benefit of providing a single source of truth for finance product data that can be used across the business and channels and provide an audit trail of changes, stewardship and maintain data standards and best practices.
Understand the Root Causes Problem Behind the Problem
You can use a variety of techniques to gain an understanding of the real problem and its real causes. One such popular technique is root cause analysis, which is a systematic way of uncovering the root or underlying cause of an identified problem or a symptom of a problem.
Root cause analysis helps prevents the development of solutions that are focussed on symptoms alone .
To help identify the root cause, or the problem behind the problem, ask the people directly involved.
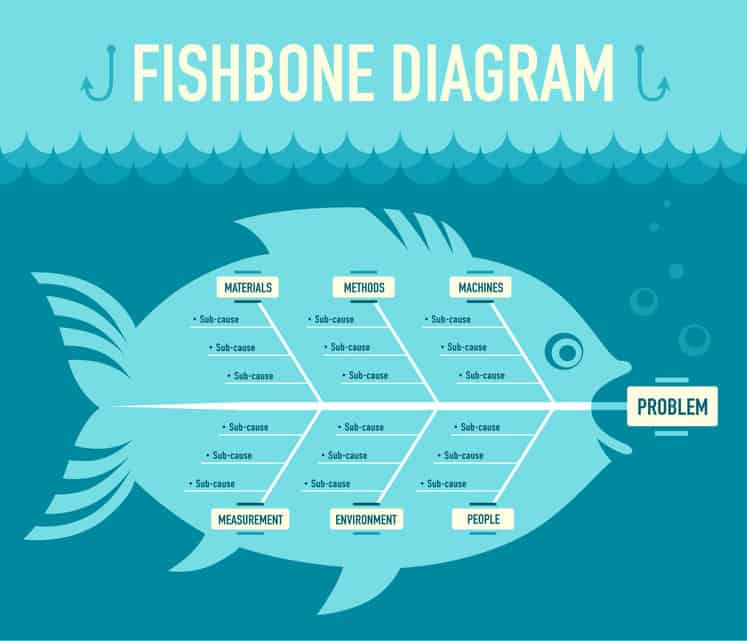
The primary goal of the technique is to determine the root cause of a defect or problem by repeating the question “Why?” . Each answer forms the basis of the next question. The “five” in the name derives from an anecdotal observation on the number of iterations needed to resolve the problem .
Identify the Stakeholders and the Users
Effectively solving any complex problem typically involves satisfying the needs of a diverse group of stakeholders. Stakeholders typically have varying perspectives on the problem and various needs that must be addressed by the solution. So, involving stakeholders will help you to determine the root causes to problems.
Define the Solution Boundary
Once the problem statement is agreed to and the users and stakeholders are identified, we can turn our attention of defining a solution that can be deployed to address the problem.
Identify the Constraints Imposed on Solution
We must consider the constraints that will be imposed on the solution. Each constraint has the potential to severely restrict our ability to deliver a solution as we envision it.
Some example solution constraints and considerations could be:-
- Economic – what financial or budgetary constraints are applicable?
- Environmental – are there environmental or regulatory constraints?
- Technical – are we restricted in our choice of technologies?
- Political – are there internal or external political issues that affect potential solutions?
Conclusion – Problem Analysis
Try the five useful steps for problem solving when your next trying to gain a better understanding of the problem domain on your business analysis project or need to do problem analysis in software engineering.
The problem statement format can be used in businesses and across industries.
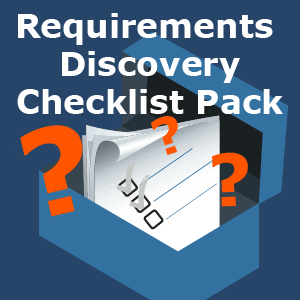
Jerry Nicholas
Jerry continues to maintain the site to help aspiring and junior business analysts and taps into the network of experienced professionals to accelerate the professional development of all business analysts. He is a Principal Business Analyst who has over twenty years experience gained in a range of client sizes and sectors including investment banking, retail banking, retail, telecoms and public sector. Jerry has mentored and coached business analyst throughout his career. He is a member of British Computer Society (MBCS), International Institute of Business Analysis (IIBA), Business Agility Institute, Project Management Institute (PMI), Disciplined Agile Consortium and Business Architecture Guild. He has contributed and is acknowledged in the book: Choose Your WoW - A Disciplined Agile Delivery Handbook for Optimising Your Way of Working (WoW).
Recent Posts
Introduction to Train the Trainer for a Business Analyst
No matter the industry, modern professionals need to continuously improve themselves and work on up skilling and re-skilling to maintain satisfactory success within their field. This is particularly...
CliftonStrengths for a Business Analyst | Be You
Today, the job of a business analyst is probably more challenging than ever. The already intricate landscape of modern business analysis has recently gone through various shifts, mainly due to the...

- Connect with us on LinkedIn
- Our Blog RSS Feed
Stay informed.
Keep up with the latest in careers, hiring and industry developments.

The Top 10 Characteristics of Problem Solvers
September 24th, 2017
Have you ever noticed that some people seem to be natural born problem solvers? Look closer, and you’ll discover that problem solving is more a skill than a gift. Effective problem solvers share ten common characteristics.
1. They have an “attitude”!
Simply expressed, effective problem solvers invariably see problems as opportunities, a chance to learn something new, to grow, to succeed where others have failed, or to prove that “it can be done”. Underlying these attitudes is a deeply held conviction that, with adequate preparation, the right answer will come.
2. They re-define the problem.
Problem solving is a primary consulting skill. Seasoned consultants know that, very often, the initial definition of the problem (by the client) is incorrect or incomplete. They learn to discount statements such as, “Obviously, the problem is that …” and follow their own leadings, but…
3. They have a system.
Perhaps the most common model is the old consulting acronym: DACR/S in which the letters stand for Describe, Analyze, Conclude, and Recommend/Solve. As with many formulas, its usefulness stems from the step-by-step approach it represents. Effective problem solvers take the steps in order and apply them literally. For example, in describing the problem (the first step), they strenuously avoid making premature judgments or ruling out possibilities. In analyzing the information, they are careful that their own prejudices do not interfere. In developing conclusions, they are aware of the need to test them thoroughly. Finally, most astute problem solvers recognize that there is almost always more than one solution, so they develop several alternatives from which to choose.
4. They avoid the experience trap.
The world is becoming increasingly non-linear. Things happen in pairs, triads, and groups and often don’t follow traditional lines from past to present and cause to effect. In such an environment, where synchronicity and simultaneity rather than linearity prevails, past experience must be taken with a grain of salt. Seasoned problem solvers know the pitfalls of relying on what worked in the past as a guide to what will work in the future. They learn to expect the unexpected, illogical, and non-linear.
5. They consider every position as though it were their own.
For effective problem solvers, standing in the other person’s shoes is more than a cute saying. It’s a fundamental way of looking at the problem from every perspective. This ability to shift perspectives quickly and easily is a key characteristic of effective problem solvers. As one especially capable consultant put it, “I take the other fellow’s position, and then I expand upon it until I understand it better than he does”.
6. They recognize conflict as often a prerequisite to solution.
When the stakes are high in a problem situation, the parties are often reluctant to show their hands and cautious about giving away too much. In such instances, managed conflict can be an effective tool for flushing out the real facts of a situation.
7. They listen to their intuition.
Somewhere during the latter stages of the fact-finding (description) process, effective problem solvers experience what can best be called, “inklings”-gut-level feelings about the situation. When this happens, they listen, hypothesize, test and re-test. They realize that, while intuition may be partially innate, effective intuition is overwhelmingly a developed faculty-and they work to develop it!
8. They invariably go beyond “solving the problem”.
On a time scale, just solving the problem at hand brings you to the present, to a point you might call, ground-zero. Truly effective problem solvers push further. They go beyond simply solving the problem to discover the underlying opportunities that often lie concealed within the intricacies of the situation. Implicit in this approach is the premise that every problem is an opportunity in disguise.
9. They seek permanent solutions.
Permanent, as opposed to band-aid solutions, has two characteristics: (1) they address all aspects of the problem, and (2) they are win/win in that they offer acceptable benefits to all parties involved. Symptomatic problem solving, like bad surgery or dentistry, leaves part of the decay untouched, with the result that, over time, it festers and erupts. Just for the record, a permanent solution is one that STAYS solved and doesn’t come back to bite you.
10. They gain agreement and commitment from the parties involved.
It’s easy, in the heady rush of finding “the answer” to a problem, to fail to gain agreement and commitment on the part of everyone involved. For effective problem solvers, just “going along” via tacit agreement isn’t enough. There must be explicit statements from all parties that they concur and are willing to commit to the solution. Agreement and concurrence really constitute a third characteristic of the “permanent” solution discussed above, but they are so often ignored that it is important that they be viewed separately.
Written by Shale Paul, Copyright Coach University. All Rights Reserved.
Share this:
Comments are closed.
- Newsletter Content
- Software Sales Candidates
What is Problem-Solving in Nursing? (With Examples, Importance, & Tips to Improve)

Whether you have been a nurse for many years or you are just beginning your nursing career, chances are, you know that problem-solving skills are essential to your success. With all the skills you are expected to develop and hone as a nurse, you may wonder, “Exactly what is problem solving in nursing?” or “Why is it so important?” In this article, I will share some insight into problem-solving in nursing from my experience as a nurse. I will also tell you why I believe problem-solving skills are important and share some tips on how to improve your problem-solving skills.
What Exactly is Problem-Solving in Nursing?
5 reasons why problem-solving is important in nursing, reason #1: good problem-solving skills reflect effective clinical judgement and critical thinking skills, reason #2: improved patient outcomes, reason #3: problem-solving skills are essential for interdisciplinary collaboration, reason #4: problem-solving skills help promote preventative care measures, reason #5: fosters opportunities for improvement, 5 steps to effective problem-solving in nursing, step #1: gather information (assessment), step #2: identify the problem (diagnosis), step #3: collaborate with your team (planning), step #4: putting your plan into action (implementation), step #5: decide if your plan was effective (evaluation), what are the most common examples of problem-solving in nursing, example #1: what to do when a medication error occurs, how to solve:, example #2: delegating tasks when shifts are short-staffed, example #3: resolving conflicts between team members, example #4: dealing with communication barriers/lack of communication, example #5: lack of essential supplies, example #6: prioritizing care to facilitate time management, example #7: preventing ethical dilemmas from hindering patient care, example #8: finding ways to reduce risks to patient safety, bonus 7 tips to improve your problem-solving skills in nursing, tip #1: enhance your clinical knowledge by becoming a lifelong learner, tip #2: practice effective communication, tip #3: encourage creative thinking and team participation, tip #4: be open-minded, tip #5: utilize your critical thinking skills, tip #6: use evidence-based practices to guide decision-making, tip #7: set a good example for other nurses to follow, my final thoughts, list of sources used for this article.

BRAND NEW Future Problem Solving Resource Library. We’re just getting started and are still uploading content. Keep an eye out for additional articles and free tools in the coming months.

- Global Issues
- Community Projects
- Creative Writing
- Storytelling
- Problem-Solving Method
- Real World Issues
- Future Scenarios
- Authentic Assessments
- 5Cs of Learning
- Youth Protection
- DEIB Commitment
- International Conference
- Find FPS Near Me
- Partner With Us

Why is problem solving important?
Future Problem Solving teaches students HOW to think, not WHAT to think, using a problem solving process. Just why is problem solving important? We answer that question.
Retired affiliate directors Brenda Darnell (Kentucky) and Cyd Rogers (Texas) collaborated together to share some tips on the important role parents play in their Future Problem Solving students’ experience.
Students increase their understanding about a topic or situation
In Global Issues, students analyze and work through important futuristic topics. In Community Projects, students gain understanding about a community issue they identify, then implement real solutions as a result of that understanding. Although Creative Writing and Storytelling produce a creative and imaginative product, they begin by developing a basis of information about the topic their stories are based on.
Problem solving promotes critical and creative thinking
The process students use in Future Problem Solving alternates between creative/divergent thinking to generate ideas and critical/convergent thinking to focus and analyze those ideas and to make decisions about them. Creative thinking is important because it broadens our perspective by pushing us out of a linear way of thinking. It instills curiosity and encourages questions, opening us up to the concept of many new ideas. It allows us to think without judgment, showing us that there are many perspectives to any issue, and many innovative solutions to any problem.
Critical thinking helps us think clearly and systematically, teaching us how to break down information and improve our ability to comprehend. It helps identify bias and promote open-mindedness. Knowing how to sort through all the “extra noise” helps us reflect, develop, and justify our decisions. Critical thinking promotes the development of many crucial life and career skills, such as logical thinking, decision-making, and open-mindedness.
Problem solving is empowering
Continually learning and expanding one’s knowledge helps students tackle personal challenges in their lives. Futuristic topics allow students to address world problems that will exist in the future. Self-confidence is boosted as students learn that they can make a difference. Problem solving in our program helps students develop persistence, embrace change, and overcome obstacles – all of which are empowering life skills.
Problem solving is a collaborative approach
Working cooperatively with others helps students realize that a variety of knowledge, perspectives, and experiences can enhance the outcome. Empathy is developed, and relationships are strengthened as students learn to respect other perspectives, opinions and differences. Problem solving improves decision-making, teaches compromise, and reduces conflict as students work together rather than against each other. Students learn to share ideas appropriately and with respect. They become more invested in finding a solution, which can encourage them to take ownership of the problem and follow through on any actions needed to implement the solution.
Problem solving is an essential skill for the workplace
Future Problem Solving addresses the top skills desired in the workplace identified by the World Economic Forum. FPS alumni tell us time and time again that their participation has helped them in their careers.
- During my entire K-12 tenure, no other experience was more influential or beneficial to my ability to think broadly and creatively than FPS. – Evan, Restoration Biologist
- In FPS I learned to see undesirable and/or unforeseen circumstances as solvable challenges rather than insurmountable problems. – Danielle, Master Social Worker
- I learned about conflict and compromise and negotiating the dynamics of a group of very strong-willed, often stubborn teammates under intense pressure – skills I’ve drawn on in my life ever since. – Reuben, History/Social Science Content Support Lead, MA Dept. of Elementary & Secondary Education
- FPS taught me a problem solving process that I still use today. Whether the problem is change or distrust in an election, the steps to address it are largely the same. – David, Attorney, Wisconsin Ethics Commission
A specific model
A specific model provides a foundation for effective problem solving, as it helps one strategize, prioritize, and make decisions. Future Problem Solving uses the Creative Problem Process (CPS) created by Alex Osborne in the 1940s. He and his colleague, Sidney Parnes, worked to further develop the process in the 50s and 60s. CPS is the basis for many creative problem solving methods used in the business world today.
Terminology in the many problem solving models that exist may vary, but the process and thinking skills are the same. All models incorporate three basic elements: (1) understanding the challenge/ issue/ situation; (2) generating ideas; and (3) preparing for action.
Understanding the challenge/issue/situation | Step 1: Identify challenges Step 2: Select an underlying problem |
Generating ideas | Step 3: Produce solution ideas |
Preparing for action | Step 4: Generate and select criteria Step 5: Evaluate solutions Step 6: Develop an action plan |
In summary, perhaps Bill Gates in The Road Ahead, says it best: “More than ever, an education that emphasizes general problem-solving skills will be important. In a changing world, education is the best preparation for being able to adapt.”
Additional resources
- What Is Creative Thinking and Why Is It Important? (LifeHack)
- Why is Creativity Important and What Does it Contribute? (National Youth Council of Ireland)
- Why Is Critical Thinking Important? A Survival Guide (University of the People)
- What Are Critical Thinking Skills and Why Are They Important? (Coursera)
- 10 Ways Collaboration Can Benefit Problem Solving (Medium)
Related Articles
- Quick Start Guide: Learning the 6-step problem solving approach
- The Way We Go – Korean FPS
- 10 strategies for coping with perfectionism
- How do the UN SDGs for Quality Education and Future Problem Solving align?
- How do NYLC Service-Learning Standards and Future Problem Solving align?
- How do STEM and Future Problem Solving align?
April Michele

In the above program, the syntax error is in the first printf statement since the printf statement doesn’t end with a ‘;’. Now, until and unless this error is not rectified, the program will not get executed.
Once the error is rectified, one gets the desired output. Suppose the input is ‘good’ then the output is : Output:
(ii) Logical Error An error caused due to the implementation of a wrong logic in the program is called logical error. They are usually detected during the runtime. Take an example in C Language:
In the above code, the ‘for’ loop won’t get executed since n has been initialized with the value of 11 while ‘for’ loop can only print values smaller than or equal to 10. Such a code will result in incorrect output and thus errors like these are called logical errors. Once the error is rectified, one gets the desired output. Suppose n is initialised with the value ‘5’ then the output is : Output:
(iii) Runtime Error Any error which causes the unusual termination of the program is called runtime error. They are detected at the run time. Some common examples of runtime errors are : Example 1 :
If during the runtime, the user gives the input value for B as 0 then the program terminates abruptly resulting in a runtime error. The output thus appears is : Output:
Example 2 : If while executing a program, one attempts for opening an unexisting file, that is, a file which is not present in the hard disk, it also results in a runtime error.
6. Documentation : The program documentation involves :
- Problem Definition
- Problem Design
- Documentation of test perform
- History of program development
7. Program Maintenance: Once a program has been formed, to ensure its longevity, maintenance is a must. The maintenance of a program has its own costs associated with it, which may also exceed the development cost of the program in some cases. The maintenance of a program involves the following :
- Detection and Elimination of undetected errors in the existing program.
- Modification of current program to enhance its performance and adaptability.
- Enhancement of user interface
- Enriching the program with new capabilities.
- Updation of the documentation.
Control Structure- Conditional control and looping (finite and infinite)
There are codes which usually involve looping statements. Looping statements are statements in which instruction or a set of instructions is executed multiple times until a particular condition is satisfied. The while loop, for loop, do while loop, etc. form the basis of such looping structure. These statements are also called control structure because they determine or control the flow of instructions in a program. These looping structures are of two kinds :
In the above program, the ‘for’ loop gets executed only until the value of i is less than or equal to 10. As soon as the value of i becomes greater than 10, the while loop is terminated. Output:
In the above code, one can easily see that the value of n is not getting incremented. In such a case, the value of n will always remain 1 and hence the while loop will never get executed. Such loop is called an infinite loop. Output:
Please Login to comment...
Similar reads.
- School Programming
Improve your Coding Skills with Practice
What kind of Experience do you want to share?

MSU Extension
Problem-solving skills are an important factor in academic success.
Elizabeth Gutierrez, Michigan State University Extension - May 11, 2012
Updated from an original article written by [email protected]..
Parents and caregivers can ensure their children's success by teaching and modeling effective problem-solving at home.
Helping your child learn how to problem solve is a critical skill for school readiness. Parents and caregivers are a child’s first and most important teacher; therefore, modeling good problem solving skills is very important. Children learn by watching parents and caregivers handle different situations and solve problems. If a parent handles problems by yelling, throwing things, hitting, grabbing or using other unacceptable strategies, a child will learn to do the same thing.
Often, adults will prevent their children from seeing all conflicts or disagreements. Remember, it is important for children to see adults negotiate differences, compromise and resolve conflicts. Learning to negotiate differences in a constructive way and allowing children to see how this is done is very effective and important. If parent and caregivers handle these situations privately, there is no example for the child/children to learn from.
Children can learn how to be assertive verbally as a result of seeing and listening to how adults resolve conflict. Another simple way a child can learn how to be assertive verbally is by role-playing with puppets and through pretend play with an adult. When using these techniques, it is important to help your child think of constructive ways to respond to different situations. By using puppets and role-play, your child can also learn about how others may feel in specific situations. When using these techniques, it is important not to criticize or label the child for past misbehavior.
There are some basic steps to problem solving from Incredible Years :
- Identify the problem.
- List the possible solutions or courses of action.
- Weigh the possible solutions.
- Choose a solution to try.
- Put the solution into practice.
- Evaluate the solution.
Using effective problem solving techniques will help children avoid conflict with others in a school setting and in their everyday lives. It will also strengthen children’s beginning empathy skills and help them learn more positive attributions about another person’s intentions. Effective problem solving skills is essential for academic and social success.
For more articles on child development, academic success, parenting and life skill development, please visit the Michigan State University Extension website.
This article was published by Michigan State University Extension . For more information, visit https://extension.msu.edu . To have a digest of information delivered straight to your email inbox, visit https://extension.msu.edu/newsletters . To contact an expert in your area, visit https://extension.msu.edu/experts , or call 888-MSUE4MI (888-678-3464).
Did you find this article useful?
Ready to grow with 4-H? Sign up today!
new - method size: 3 - Random key: 2, method: personalized - key: 2
You Might Also Be Interested In
MSU researcher awarded five-year, $2.5 million grant to develop risk assessment training program
Published on October 13, 2020
MSU Product Center helps Michigan food entrepreneurs survive and thrive throughout pandemic
Published on August 31, 2021
Protecting Michigan’s environment and wildlife through the Conservation Reserve Enhancement Program
Published on September 1, 2021
MSU Extension to undertake three-year, $7 million vaccination education effort
Published on August 17, 2021
MSU to study precision livestock farming adoption trends in U.S. swine industry
Published on March 15, 2021
MSU research team receives USDA grant to evaluate effectiveness, cost of new blueberry pest management strategies
Published on February 19, 2021
- child & family development
- early childhood development
- msu extension
- social and emotional development
- child & family development,
- early childhood development,
- msu extension,
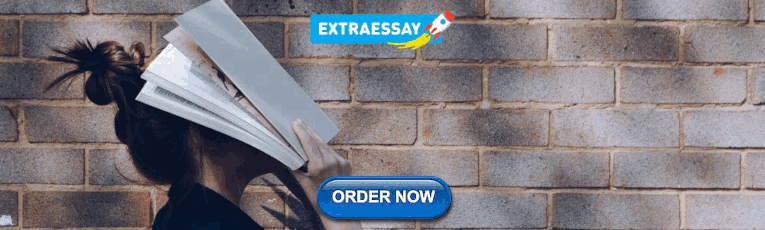
IMAGES
COMMENTS
A statistics problem typically contains four components: 1. Ask a Question. Asking a question gets the process started. It's important to ask a question carefully, with an understanding of the data you will use to find your answer. 2, Collect Data. Collecting data to help answer the question is an important step in the process.
The Problem-Solving Strategy consists of three steps: Step 1: Plan & Prepare, Step 2: Organize & Solve, and Step 3: Cross Reference. Step 1: Plan & Prepare. Questions for developing metacognition, defined as awareness or analysis of one's own learning or thinking processes (Merriam-Webster, 2023): Do I have the knowledge needed to complete ...
Consider statistics as a problem-solving process and examine its four components: asking questions, collecting appropriate data, analyzing the data, and interpreting the results. This session investigates the nature of data and its potential sources of variation. Variables, bias, and random sampling are introduced. View Transcript.
Four-Step Statistical Process: 1. Plan (Ask a question): formulate a statistical question that can be answered with data. A good deal of time should be given to this step as it is the most important step in the process. 2. Collect (Produce Data): design and implement a plan to collect appropriate data. Data can be collected through numerous ...
The first step to solve the statistics problem is to decide the problem that is the question or hypothetical test. Unless you know the question you can't process with other steps because this step will decide the parameter and population for your study. This is why this is the first and foremost step in How to solve statistics problems.
Learn how to conquer statistical problems by leveraging tools such as statistical software, graphing calculators, and online resources. Discover the key steps to effectively solve statistical challenges: define the problem, gather data, select the appropriate model, use tools like R or Python, and validate results. Dive into the world of DataCamp for interactive statistical learning experiences.
The descriptive statistical analysis allows organizing and summarizing the large data into graphs and tables. Descriptive analysis involves various processes such as tabulation, measure of central tendency, measure of dispersion or variance, skewness measurements etc. 2. Inferential Analysis.
The problem-solving process typically includes the following steps: Identify the issue: Recognize the problem that needs to be solved. Analyze the situation: Examine the issue in depth, gather all relevant information, and consider any limitations or constraints that may be present. Generate potential solutions: Brainstorm a list of possible ...
or scientific, method is the approach to formulating and solving these problems. The steps in the engineering method are as follows: 1. Develop a clear and concise description of the problem. 2. Identify, at least tentatively, the important factors that affect this problem or that may play a role in its solution. 1-1 THE ENGINEERING METHOD AND
This article is a practical introduction to statistical analysis for students and researchers. We'll walk you through the steps using two research examples. The first investigates a potential cause-and-effect relationship, while the second investigates a potential correlation between variables. Example: Causal research question.
Finding a suitable solution for issues can be accomplished by following the basic four-step problem-solving process and methodology outlined below. Step. Characteristics. 1. Define the problem. Differentiate fact from opinion. Specify underlying causes. Consult each faction involved for information. State the problem specifically.
Problem-solving is used in various fields, including business, engineering, science, and mathematics. Role of Statistics in Problem-Solving. Statistics is a powerful tool that can be used to help solve problems. Statistics uses probability theory as its base, so when your problem can be stated as a probability, you can reliably go to statistics ...
There are 5 main steps in hypothesis testing: State your research hypothesis as a null hypothesis and alternate hypothesis (H o) and (H a or H 1 ). Collect data in a way designed to test the hypothesis. Perform an appropriate statistical test. Decide whether to reject or fail to reject your null hypothesis. Present the findings in your results ...
The problem solving process typically includes: Pinpointing what's broken by gathering data and consulting with team members. Figuring out why it's not working by mapping out and troubleshooting the problem. Deciding on the most effective way to fix it by brainstorming and then implementing a solution. While skills like active listening ...
There are five useful steps that can be taken to gain a better understanding of the problem before developing a solution. Gain agreement on the problem definition. Understand the root-causes - the problem behind the problem. Identify the stakeholders and the users. Define the solution boundary.
These steps help ensure that the data science project delivers accurate, reliable, and meaningful insights that can help solve the problem statement. Data Science Problem Solving: A Deep Dive into ...
ples. The engineering or scientific methodis the approach to formulating and solving these problems. The steps in the engineering method are as follows: 1. Develop a clear and concise description of the problem. 2. Identify, at least tentatively, the important factors that affect this problem or that may play a role in its solution.
Step 1: Define the Problem. Differentiate fact from opinion. Specify underlying causes. Consult each faction involved for information. State the problem specifically. Identify what standard or expectation is violated. Determine in which process the problem lies. Avoid trying to solve the problem without data.
6. They recognize conflict as often a prerequisite to solution. When the stakes are high in a problem situation, the parties are often reluctant to show their hands and cautious about giving away too much. In such instances, managed conflict can be an effective tool for flushing out the real facts of a situation. 7.
Problem-solving in nursing is the vital foundation that makes up a nurse's clinical judgment and critical thinking skills. Having a strong problem-solving skillset is pertinent to possessing the ability and means to provide safe, quality care to a variety of patients. Nurses must rely on their clinical judgment and critical thinking skills to ...
Future Problem Solving uses the Creative Problem Process (CPS) created by Alex Osborne in the 1940s. He and his colleague, Sidney Parnes, worked to further develop the process in the 50s and 60s. CPS is the basis for many creative problem solving methods used in the business world today.
The several steps of this cycle are as follows : Step by step solution for a problem (Software Life Cycle) 1. Problem Definition/Specification: A computer program is basically a machine language solution to a real-life problem. Because programs are generally made to solve the pragmatic problems of the outside world.
By using puppets and role-play, your child can also learn about how others may feel in specific situations. When using these techniques, it is important not to criticize or label the child for past misbehavior. There are some basic steps to problem solving from Incredible Years: Identify the problem. List the possible solutions or courses of ...