Sports Analytics algorithms for performance prediction
Ieee account.
- Change Username/Password
- Update Address
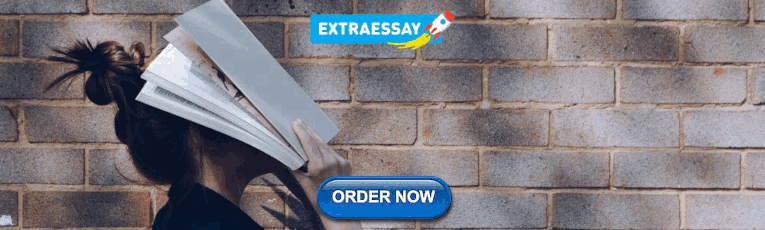
Purchase Details
- Payment Options
- Order History
- View Purchased Documents
Profile Information
- Communications Preferences
- Profession and Education
- Technical Interests
- US & Canada: +1 800 678 4333
- Worldwide: +1 732 981 0060
- Contact & Support
- About IEEE Xplore
- Accessibility
- Terms of Use
- Nondiscrimination Policy
- Privacy & Opting Out of Cookies
A not-for-profit organization, IEEE is the world's largest technical professional organization dedicated to advancing technology for the benefit of humanity. © Copyright 2024 IEEE - All rights reserved. Use of this web site signifies your agreement to the terms and conditions.

Aims and Scopes
The Journal of Sports Analytics (JSA) is a new high-quality research journal that aims to be the central forum for the discussion of practical applications of sports analytics research, serving team owners, general managers, coaches, fans, and academics.
We invite analytical research on any single sport or across sports or esports that seeks to improve our understanding of the game or strategies for improving a team or a league.
- player evaluation
- game strategies
- sports business
- roster construction
- contract negotiations
- data visualization
- physics in sports
- draft analysis
- sport psychology
- coaching evaluation
- technology in sports
- rule changes
- referee analytics
- health and injuries
- sports awards
- sports records
Impact Factor
Journal impact factor (JIF): 1.1
Immediacy index: 0.3
Journal citation indicator (JCI): 0.26
Submissions
We are currently soliciting general submissions for future issues.
There will be no author submission or publication fees for at least the first few volumes. All submissions will be double-blind peer reviewed. As experts in the field, submissions by members of our editorial board are also welcomed; such submissions will be carefully managed to ensure the integrity, privacy, and independence of the review. Initial submissions must be in PDF format and anonymized (names, affiliations, and acknowledgments removed), but otherwise may be in any convenient style. There are no page limits. If accepted for publication, your paper must be revised to meet our style guidelines.
Your submission may not be under review at any other journal while it is under review at the Journal of Sports Analytics and it may not have been previously published or accepted for publication in a journal. Presentations at conferences, appearances in conference proceedings, and working papers posted online are typically not considered as previous publication, and such submissions are welcomed. It is often useful to incorporate comments from seminars and conferences into a longer, more detailed version to submit to the journal for review.
Submit your paper to the Journal of Sports Analytics
Subscriptions and Access
The contents of at least the first few volumes will be freely available online. Hard copy subscriptions are also available.
View the online archive of the Journal of Sports Analytics
Co-Editors-in-Chief
Philip maymin, fairfield university, eugene shen, advisory board, andrew berry, cleveland browns, memphis grizzlies, james click, houston astros, tim connelly, denver nuggets, dallas mavericks, eric decosta, baltimore ravens, kevin demoff, los angeles rams, fmr. new orleans pelicans, jessica gelman, kraft analytics group, dan kantrovitz, oakland athletics, jacksonville jaguars, fulham football club, ted leonsis, washington capitals, washington wizards, baltimore orioles, daryl morey, philadelphia 76ers, associate editors, ambra macis, university of brescia, marica manisera, hockey and golf, simon fraser university, new york yankees.

Editorial Board
Daniel adler, minnesota twins, nyu and north yard analytics, benjamin alamar, steven angel, national basketball association, new york mets, zaheer benjamin, vision insights, matt bollero, milwaukee bucks, michael clutterbuck, harry crane, rutgers university, pittsburgh pirates, sarah gelles, sam grossman, cincinnati reds, karim kassam, zelus analytics, shane kupperman, new orleans pelicans, carolina panthers, dennis lock, buffalo bills, christopher long, chanteclair farm, annika foundation, dean oliver, trevor patch, colorado rockies, ryan rodenberg, florida state university, jason rosenfeld, joe sheehan, toronto blue jays, san diego padres, laurence stewart, manchester city fc, patrick sund, golden state warriors, mitch tanney, coterie insurance, john urschel, victor wang, cleveland indians, swish analytics, mike zarren, boston celtics, tucker zeleny.
- Reference Manager
- Simple TEXT file
People also looked at
Perspective article, artificial intelligence and machine learning in sport research: an introduction for non-data scientists.
- Institute for Health and Sport, Victoria University, Melbourne, VIC, Australia
In the last two decades, artificial intelligence (AI) has transformed the way in which we consume and analyse sports. The role of AI in improving decision-making and forecasting in sports, amongst many other advantages, is rapidly expanding and gaining more attention in both the academic sector and the industry. Nonetheless, for many sports audiences, professionals and policy makers, who are not particularly au courant or experts in AI, the connexion between artificial intelligence and sports remains fuzzy. Likewise, for many, the motivations for adopting a machine learning (ML) paradigm in sports analytics are still either faint or unclear. In this perspective paper, we present a high-level, non-technical, overview of the machine learning paradigm that motivates its potential for enhancing sports (performance and business) analytics. We provide a summary of some relevant research literature on the areas in which artificial intelligence and machine learning have been applied to the sports industry and in sport research. Finally, we present some hypothetical scenarios of how AI and ML could shape the future of sports.
Introduction
It was in Moneyball ( Lewis, 2004 ), the famous success storey of the Major League Baseball team “Oakland Athletics,” that using in-game play statistics came under focus as a means to assemble an exceptional team. Despite Oakland Athletics' relatively small budget, the adoption of a rigorous data-driven approach to assemble a new team led to the playoffs in the year 2002. An economic evaluation of the Moneyball hypothesis ( Hakes and Sauer, 2006 ) describes how, at the time, a baseball hitters' salary was not truly explained by the contribution of a player's batting skills to winning games. Oakland Athletics gained a big advantage over their competitors by identifying and exploiting this information gap. It's been almost two decades since Moneyball principles, or SABRmetrics ( Lewis, 2004 ) was introduced to baseball. SABR stands for Society for American Baseball Research and SABRmetricians are those scientists who gather the in-game data and analyse it to answer questions that will lead to improving team performance. Since the success of the Oakland Athletics, most MLB teams started employing SABRmetricians. The ongoing and exponential increase of computer processing power has further accelerated the ability to analyse “big data,” and indeed, computers increasingly are taking charge of the deeper analysis of data sets, through means of artificial intelligence (AI). Likewise, the surge in high-quality data collection and data aggregation (accomplished by organisations like Baseball Savant/StatCast, ESPN and others) are key ingredients to the spike in the accuracy and breadth of analytics that was observed in the MLB in recent years.
The adoption of AI and statistical modelling in sports has become therefore more prominent in recent years as new technologies and research applications are impacting professional sports at various levels of sophistication. The wide applicability of machine learning algorithms, combined with increasing computing processing power as well as access to more and new sources of data in recent years, has made sports organisations hungry for new applications and strategies. The overriding aim is still to make them more competitive on and off the field–in athletic and business performance. The benefits of leveraging the power of AI can, in that regard, take different forms from optimising business or technical decision making to enhancing athlete/team performance but also increasing demand for attendance at sporting events, as well as promoting alternative entertainment formats of the sport.
We next list some areas where AI and machine learning (ML) have left their footprints in the world of sports ( Beal et al., 2019 ) and provide some examples of applications in each (some of the listed applications could overlap with one or more of the areas).
• Game activity/analytics: match outcome modelling, player/ball Tracking, match event (e.g., shot) classification, umpire assistance, sports betting .
• Talent identification and acquisition: player recruitment, player performance measurement, biomechanics .
• Training and coaching: assessment of team formation efficacy, tactical planning, player injury modelling .
• Fan and business focused: measurement of a player's economic value, modelling demand for event attendance, ticket pricing optimisation (variable and dynamic), wearable and sensor design, highlight packaging, virtual and augmented reality sport applications, etc .
The field of AI (particularly ML) offers new methodologies that have proven to be beneficial for tackling the above challenges. In this perspective paper we aim to provide sports business professionals and non-technical sports audiences, coaches, business leaders, policy makers and stakeholders with an overview of the range of AI approaches used to analyse sport performance and business centric problems. We also discuss perspectives on how AI could shape the future of sports in the next few years.
Research on AI and ML in Sports
In this section, we will not be reviewing examples of how AI has been applied to sports for a specific application, but rather, we will look at the intersection of AI and sports at a more abstract level, discussing some research that either surveyed or summarised the application of AI and ML in sports.
One of the earliest works discussing the potential applications of artificial intelligence in sports performance, and its positive impact on improving decision-making is by Lapham and Bartlett (1995) . The paper discusses how expert systems (i.e., a knowledge-based database used for reasoning) can be used for sports biomechanics purposes. Bartlett (2006) reviewed developments in the use of AI in sports biomechanics (e.g., throwing, shot putting, football kicking, …) to show that, at the time of writing, expert systems were marginally used in sports biomechanics despite being popular for “gait analysis” whereas Artificial Neural Networks were used for applications such as performance patterns in training and movement patterns of sports performers. An Artificial Neural Network (ANN) is a system that mimics the functionality of a human brain. ANNs are used to solve computational problems or estimate functions from a given data input, by imitating the way neurons are fired or activated in the human brain. Several (layers of) artificial neurons, known as perceptrons, are connected to perform computations which return an output as a function of the provided input ( Anderson, 1995 ).
Bartlett (2006) predicted that multi-layer ANNs will play a big role in sports technique analysis in the future. Indeed, as we discuss later, multi-layer ANNs, now commonly referred to as Deep Learning, have become one of the most popular techniques in sports related analytics. Last but not least Bartlett (2006) described the applications of Evolutionary Computation and hybrid systems in the optimization of sports techniques and skill learning. Further discussion around the applications of AI in sports biomechanics can be found in Ratiu et al. (2010) . McCabe and Trevathan (2008) discussed the use of artificial intelligence for prediction of sporting outcomes, showing how the behaviour of teams can be modelled in different sporting contests using multi-layer ANNs.
Between 2006 and 2010, machine learning algorithms, particularly ANNs were becoming more popular amongst computer scientists. This was aided by the impressive improvements in computer hardware, but also due to a shift in mindset in the AI community. Large volumes of data were made public amongst researchers and scientists (e.g., ImageNet a visual database delivered by Stanford University), and new open-source machine learning competitions were organised (such as Netflix Prize and Kaggle). It is these types of events that have shaped the adoption of AI and machine learning in many different fields of study from medicine to econometrics and sports, by facilitating access to training data and offering free open-source tools and frameworks for leveraging the power of AI. Note that, in addition to ANN, other machine learning techniques are utilised in such competitions, and sometimes these can be used in combination with one another. For instance, some of the techniques that went into the winning of the Netflix prize include singular value decomposition combined with restricted Boltzmann machines and gradient boosted decision trees.
Other examples discussing ANNs in sports include Novatchkov and Baca (2013) who discuss how ANNs can be used for understanding the quality of execution, assisting athletes and coaches, and training optimisation. However, the applications of AI to sports analytics go beyond the use of ANNs. For example, Fister et al. (2015 ) discussed how nature-inspired AI algorithms can be used to investigate unsolved research problems regarding safe and effective training plans. Their approach ( Fister et al., 2015 ) relies on the notion of artificial collective intelligence ( Chmait et al., 2016 ; Chmait, 2017 ) and the adaptability of algorithms to adapt to a changing environment. The authors show how such algorithms can be used to develop an artificial trainer to recommend athletes with an informed training strategy after taking into consideration various factors related to the athlete's physique and readiness. Other types of scientific methods that include Bayesian approaches have been applied to determining player abilities ( Whitaker et al., 2021 ) but also predicting match outcomes ( Yang and Swartz, 2004 ). Bayesian analysis and learning is an approach for building (statistical and inference) models by updating the probability for a hypothesis as more evidence or information becomes available by using Bayes' theorem ( Ghosh et al., 2007 ).
There are numerous research papers in which AI and ML is applied to sport, and it is not our aim to comprehensively discuss these works here 1 . However, we refer to a recent survey that elaborates on this topic. Beal et al. (2019) surveyed the applications of AI in team sports. The authors summarised existing academic work, in a range of sports, tackling issues such as match outcome modelling, in-game tactical decision making, player performance in fantasy sport games, and managing professional players' sport injuries. Work by Nadikattu (2020) presents, at an abstract level, discussions on how AI can be implemented in (American) sports from enhancing player performance, to assisting coaches to come up with the right formations and tactics, to developing automated video highlights of sports matches and supporting referees using computer vision applications.
We emphasise that the application of AI in sports is not limited to topics of sports performance, athlete talent identification or the technical analysis of the game. The (off the field) business side of sports organisations is rapidly shifting towards a data driven culture led by developing profiles of their fans and their consumer preferences. As fans call for superior content and entertainment, sport organisations must react by delivering a customised experience to their patrons. This is often achieved by the use of statistical modelling as well as other machine learning solutions, for example, to understand the value of players from an economic perspective. As shown in Chmait et al. (2020a) , investigating the relationship between the talent and success of athletes (to determine the existence of what is referred to as superstardom phenomenon or star power) is becoming an important angle to explore value created in sport. To provide an idea of the extent of such work, we note some sports in which the relationship between famous players/teams and their effect on audience attendance or sport consumption has been studied:
• In soccer ( Brandes et al., 2008 ; Jewell, 2017 ),
• In Major League Baseball ( Ormiston, 2014 ; Lewis and Yoon, 2016 )
• In the National Basketball Association ( Berri et al., 2004 ; Jane, 2016 )
• In tennis: superstar player effect in demand for tennis tournament attendance ( Chmait et al., 2020a ), the presence of a stardom effect in social media ( Chmait et al., 2020b ), player effect on German television audience demand for live broadcast tennis matches ( Konjer et al., 2017 )
• And similarly, in Cricket ( Paton and Cooke, 2005 ), Hockey ( Coates and Humphreys, 2012 ), and in the Australian Football League ( Lenten, 2012 ).
AI algorithms are being used in Formula 1 (F1) to improve the racing tactics of competing teams by analysing data from hundreds of sensors in the F1 car. Recent work by Piccinotti (2021) shows how artificial intelligence can provide F1 with automated ways for identifying tyre replacement strategies by modelling pit-stop timing and frequency as sequential decision-making problems.
Researchers from Tennis Australia and Victoria University devised a racket recommendation technique based on real HawkEye (computer vision system) data. An algorithm was used to recommend a selection of rackets based on movement, hitting pattern and style of the player with the aim to improve the player's performance ( Krause, 2019 ).
Accurate and fair judging of sophisticated skills in sports like gymnastics is a difficult task. Recently, a judging system was developed by Fujitsu Ltd. The system scores a routine based on the angles of a gymnast's joints. It uses AI to analyse 3D laser sensors that capture the gymnasts' movements ( Atiković et al., 2020 ).
Finally, it is important to note the exceptionally successful adoption of AI in board games like Chess, Checkers, Shogi and the Chinese game of GO, as well as virtual games (like Dota2 and StarCraft). In the last couple of decades, AI has delivered a staggering rise in performance in such areas to the point that machines (almost) constantly defeat human world champions. We refer to some notable solutions like Schaeffer et al. (2007) Checkers artificial algorithm, DeepBlue defeating Kasparov in Chess ( Campbell et al., 2002 ), AlphaGo Zero defeating Lee Sedol in Go ( Silver et al., 2017 ) (noting that AlphaZero is also unbeatable in chess) and Vinyals et al. (2019) AlphaStar in StarcraftII as well as superhuman AI for multiplayer poker ( Brown and Sandholm, 2019 ). Commonly, in these types of games or sports, AI algorithms rely on a Reinforcement Learning approach (which we will describe later) as well as using techniques like the Monte-Carlo Search Trees to explore the game and devise robust strategies to solve and play these games. Some of the recent testbeds used to evaluate AI agents and algorithms are discussed in Hernández-Orallo et al. (2017 ). For a broader investigation of AI in board and virtual/computer games refer to Risi and Preuss (2020) .
The rise of applying AI and ML is unstoppable and to that end, one might be wondering how AI an ML tools work and why are they different from traditional summary analytics. We touch upon these considerations in the next section.
The Machine Learning Paradigm
To understand why ML is used in a wide range of applications, we need to take a look into the difference between recent AI approaches to learning and traditional analytics approaches. At a higher conceptual level, one can describe old or traditional approaches to sports analytics, as starting off with some set of rules that constitute the problem definition, some data that is to be processed using a program/application which will then deliver answers to the given problem. In contrast, in a machine learning/predictive analytics paradigm, the way this process works is fundamentally different. For instance, in some approaches of the ML paradigm, one typically starts by feeding the program with answers and corresponding data to a specific problem, with an algorithm narrowing down the rules of the problem. These rules are later used for making predictions and they are evaluated or validated by testing their accuracy over new (unseen) data.
To that end, machine learning is an area of AI that is concerned with algorithms that learn from data by performing some form of inductive learning. In simple terms, ML prediction could be described as a function 2 from a set of inputs i 1 , i 2 , …, i n , to forecast an unknown value y , as follows f ( w 1 * i 1 , w 2 * i 2 , …, w n * i n ) = y , where w t is the weight of input t .
Different types or approaches of ML are used for different types of problems. Some of the most popular are supervised learning, unsupervised learning , and reinforcement learning :
• In supervised learning, we begin by observing and recording both inputs (the i 's) and outputs (the y 's) of a system, for a given period of time. This data (collection of correct examples of inputs and their corresponding outputs) is then analysed to derive the rules that underly the dynamics of the observed system, i.e., the rules that map a given input to its correct output.
• Unlike the above, in unsupervised learning, the correct examples or outputs from a given system are not available. The task of the algorithm is to discover (previously unnoticed) patterns in the input data.
• In reinforcement learning, an algorithm (usually referred to as an agent) is designed to take a series of actions that maximise its cumulative payoff or rewards over time. The agent then builds a policy (a map of action selection rules) that return a probability of taking a given action under different conditions of the problem.
For a thorough introduction to the fundamentals of machine learning and the popular ML algorithms see Bonaccorso (2017) . The majority of AI applications in sports are based on one or more of the above approaches to ML. In fact, in most predictive modelling applications, the nature of the output y that needs to be predicted or analysed could influence the architecture of the learning algorithm.
Explaining the details of how different ML techniques work is outside the scope of this paper. However, to provide an insight into how such algorithms function in layman's terms and the differences between them, we briefly present (hypothetical) supervised, unsupervised and reinforcement learning problems in the context of sports. These examples will assist the professionals but also applied researchers who work in sport to better understand the way that data scientists think so to facilitate talking to them about their approach and methodology, without requiring to dive deep into the details of the underlying analytics.
Supervised Learning: Predicting Player Injury
Many sports injuries (e.g., muscle strain) can be effectively treated or prevented if one is able to detect them early or predict the likelihood of sustaining them. There could be many different (combinations of) reasons/actions leading to injuries like muscle strain. For example, in the Australian Football League, some of hypotheses put forward leading to muscle strain include: muscle weakness and lack of flexibility, fatigue, inadequate warm-up, and poor lumbar posture ( Brockett et al., 2004 ). Detecting the patterns that can lead to such injuries is extremely important both for the safety of the players, and for the success and competitiveness of the team.
In a supervised learning scenario, data about the players would be collected from previous seasons including details such as the number of overall matches and consecutive matches they played, total time played in each match, categorised by age, number of metres run, whether or not they warmed up before the match, how many times they were tackled by other players, and so on , but more importantly, whether or not the players ended up injured and missed their next match.
The last point is very important as it is the principal difference between supervised learning and other approaches: the outcome (whether or not the player was injured) is known in the historical data that was collected from previous seasons. This historical data is then fed (with the outcome) to a machine learning algorithm with the objective of learning the patterns (combination of factors) which led to an injury (and usually assigning a probability of the likelihood of an injury given these patterns). Once these patterns are learnt, the algorithm or model is then tested on new (unseen data) to see if it performs well and indeed predicts/explains injury at a high level of accuracy (e.g., 70% of the time). If the accuracy of the model is not as required, the model is tuned (or trained with slightly different parameters) until it reaches the desired or acceptable accuracy. Note here that we did not single out a specific algorithm or technique to achieve the above. Indeed, this approach can be applied using many different ML algorithms such as Neural Networks, Decision Trees and regression models.
Unsupervised Learning: Fan Segmentation
We will use a sport business example to introduce the unsupervised learning approach. Most sports organisations keep track of historical data about their patrons who attended their sporting events, recording characteristics such as their gender, postcode, age, nationality, education, income, marital status, etc. A natural question of interest here is to understand if the different segments of customers/patrons will purchase different categories (e.g., price, duration, class etc.) of tickets.
Some AI algorithms are designed to help split the available data, so that each data point (historical ticket sale) sits in a group/class that is similar to the other data points (other sales) in that same class given the recorded features. The algorithm will then use some sort of a similarity or distance metric to classify the patrons according to the category of tickets that they might purchase.
This is different from how supervised learning algorithms, like those discussed in the previous section, work. As we described before, in supervised learning we instruct the algorithm with the outcome in advance while training it (i.e., we classify/label each observation based on the outcome: injury or no injury, cheap or expensive seats, …). In the unsupervised learning approach, there is no such labelling or classification of existing historical data. It is the mission of the unsupervised learning algorithm to discover (previously unnoticed) patterns in the input data and group it into (two or more) classes.
Imagine the following use case where an Australian Football League club aims to identify a highly profitable customer segment within its entire set of stadium attendees, with the aim to enhance its marketing operations. Mathematical models can be used to discover (segments of) similar customers based on variations in some customer attributes within and across each segment. A popular unsupervised learning algorithm to achieve such goal is the K-means clustering algorithm which finds the class labels from the data. This is done by iteratively assigning the data points (e.g., customers) from the input into a group/class based on the characteristics of this input. The essence is that the groups or classes to which the data points are assigned to are not defined prior to exploring the input data (although the number of groups or segments can be pre-defined) but are rather dynamically formed as the K-means algorithm iterates over the data points. In the context of customer segmentation, when presenting the mathematical model (K-means algorithm) with customer data, there is no requirement to label a portion (or any of) of this data into groups in advance in order to train the model as usually done in supervised models.
Reinforcement Learning: Simulations and Fantasy Sports
As mentioned before, in reinforcement learning, an algorithm (such as Q-learning and SARSA algorithms) learns how to complete a series of tasks (i.e., solve a problem) by interacting with an (artificial) environment that was designed to simulate the real environment/problem at hand. Unlike the case with supervised learning, the algorithm is not explicitly instructed about the right/accurate action in different states/conditions of the environment (or steps of problem it is trying to solve). But rather it incrementally learns such a protocol through reward maximisation.
In simple terms, reinforcement learning approaches represent problems using what are referred to as: an agent (a software algorithm), and a table of states and actions . When the agent executes an action, it transitions from one state to another and it receives a reward or a penalty (a positive or negative numerical score respectively) as a result. The reward/penalty associated with the action-state combination is then stored in the agent's table for future reference and refinement. The agent's goal is to take the action that maximises its reward. When the agent is still unaware of the expected rewards from executing a given action when at a given state, it takes a random action and updates its table following that action. After many (thousands of) iterations over the problem space, the agent's table holds (a weighted sum of) the expected values of the rewards of all future actions starting from the initial state.
Reinforcement learning has been applied to improve the selection of team formations in fantasy sports ( Matthews et al., 2012 ). Likewise, the use of reinforcement learning is prominent in online AI bots and simulators like chess, checkers, Go, poker, StarCraft, etc.
Finally, it is important to also note the existence of genetic or evolutionary algorithms, sometimes referred to as nature/bio-inspired algorithms. While such algorithms are not typically considered to be ML algorithms (but rather search techniques and heuristics), they are very popular in solving similar types of problems tackled by ML algorithms. In short, the idea behind such algorithms is to run (parallel) search, selection and mutation techniques, by going over possible candidate solutions of a problem. The solutions are gradually optimised until reaching a local (sub-optimal) or global maximum (optimal solution). To provide a high-level understanding of evolutionary algorithms, consider the following sequence of steps:
• We start by creating (a population of) initial candidate or random strategies/solutions to the problem at hand.
• We assess these candidate solutions (using a fitness function) and assign scores to each according to how well they solve the problem at hand.
• We then pick a selection of these candidate solutions that performed best at stage two above. We then combine ( crossbreed ) these together to generate ( breed) new solutions (e.g., take some attributes from one candidate solution and others from another candidate solution in order to come up with a new solution).
• We then apply random changes ( mutations ) to the resulting solutions from the previous step.
• We repeat the solution combination/crossbreeding process until a satisfactory solution is reached.
Evolutionary algorithms can be used as alternative means for training machine learning algorithms such as reinforcement learning algorithms and deep neural networks.
The Future of AI in Sport
There is no doubt that AI will continue to transform sports, and the ways in which we play, watch and analyse sports will be innovative and unexpected. In fact, machine learning has drastically changed the way we think about match strategies, player performance analytics but also how we track, identify and learn about sport consumers. A Pandora's box of ethical issues is emerging and will increasingly need to be considered when machines invade the traditionally human centred and naturally talented athlete base of sport. It is unlikely that AI will completely replace coaches and human experts, but there is no doubt that leveraging the power of AI will provide coaches and players with a big advantage and lead over those who only rely on human expertise. It will also provide sport business managers with deeper, real time insights into the behaviours, needs and wants of sport consumers and in turn AI will become a main producer of sport content that is personalised and custom made for individual consumers. But human direction and intervention seems to be, at least in the near future, still essential working towards elite sport performance and strategic decision making in sport business. The sporting performance on the field is often produced as an entertainment spectacle, where the sporting context is the platform for generating the business of sport. Replacing referees with automated AI is clearly possible and increasingly adopted in various sports, because it is more accurate and efficient, but is it what the fans want?
What might the future of sport with increasingly integrated AI look like? Currently, most of the research in AI and sports is specialised. That is to provide performance or business solutions and solve specific on and off field problems. For instance, scientists have successfully devised solutions to tackle problems like player performance measurement, and quantifying the effect of a player/team on demand for gate attendance. Nevertheless, our research has not identified studies (yet) that provide a 360-degree analysis on, for example, the absolute value of an athlete by taking into account all the dimensions of his or her performance on how much business can be developed, for example in regard to ticket sales or endorsement deals.
One of the main challenges to achieve such a comprehensive analysis is mainly due to the fact that data about players and teams, and commercial data such as ticket sales and attendance numbers, are kept proprietary and are not made public to avoid providing other parties with competitive information. Moreover, privacy is an important consideration as well. Regulations about data privacy and leakage of personal identification details must be put in place to govern the use and sharing of sports (performance and consumption) data. Data ownership, protection, security, privacy and access will all drive the need for comprehensive and tight legislation and regulation that will strongly influence the speed and comprehensiveness of the adoption of AI in sport. To that end, it is worth considering privacy and confidentiality implications independently when studying the leagues' journey of AI adoption compared to that of individual teams and ultimately the individual players. Eventually, the successful adoption of AI in a sports league will likely depend on the teams in that league and their players to be willing to share proprietary data or insights with other teams in the league. Performance data of players in particular is becoming a hot topic of disputation. It may well be AI that will determine the bargaining power of players and their agents in regard to the value of their contracts. As an extension of this it will then also be AI providing the information that will determine if players are achieving the performance objectives set by coaches and as agreed to in contracts. In other words, confidentiality and ownership of league, team or player level data will become an increasing bone of legal contention and this will be reflected in the complexity of contractual agreements and possible disputes in the change rooms and on the field of play. Being in control of which data can or cannot, and will or will not, be used is at stake.
From an economic perspective, relying on artificial algorithms could increase the revenue of sports organisations and event organisers when enabled to apply efficient variable and dynamic pricing strategies and build comprehensive and deep knowledge consumer platforms. Different types of ML algorithms can be adopted to deliver more effective customer marketing via personalisation and to increase sales funnel conversion rates.
Finally, for a window on the future of data privacy, it might be useful to return to baseball where the addiction to big data started its spread across the high-performance sport industry. Hattery (2017 , p. 282) explains that in baseball “using advanced data collection systems … the MLB teams compete to create the most precise injury prediction models possible in order to protect and optimise the use of their player-assets. While this technology has the potential to offer tremendous value to both team and player, it comes with a potential conflict of interest. Players' goals are not always congruent with those of the organisation: the player strives to protect his own career while the team is attempting to capitalise on the value of an asset. For this reason, the player has an interest in accessing data that analyses his potential injury risk. This highlights a greater problem in big data: what rights will individuals possess regarding their own data points?”
This privacy issue can be further extended to the sport business space Dezfouli et al. (2020) have shown how AI can be designed to manipulate human behaviour. Algorithms learned from humans' responses who were participating in controlled experiments. The algorithms identified and targeted vulnerabilities in human decision-making. The AI succeeded in steering participants towards executing particular actions. So, will AI one day be shaping the spending behaviour of sports fans by exploiting their fan infused emotional vulnerabilities and monitoring their (for example) gambling inclinations? Will AI sacrifice the health of some athletes in favour of the bigger team winning the premiership? Or is this already happening? Time will tell.
Data Availability Statement
The original contributions presented in the study are included in the article, further inquiries can be directed to the corresponding author.
Author Contributions
NC and HW had major contribution to the writing of this manuscript. NC contributed to the writing of the parts around artificial intelligence and machine learning and provided examples of these. HW shaped the scope of the manuscript and wrote and edited many of its sections particularly the introduction and the discussion. Both authors contributed to the article and approved the submitted version.
Conflict of Interest
The authors declare that the research was conducted in the absence of any commercial or financial relationships that could be construed as a potential conflict of interest.
Publisher's Note
All claims expressed in this article are solely those of the authors and do not necessarily represent those of their affiliated organizations, or those of the publisher, the editors and the reviewers. Any product that may be evaluated in this article, or claim that may be made by its manufacturer, is not guaranteed or endorsed by the publisher.
1. ^ For conferences and published articles on AI and sports analytics see Swartz (2020) .
2. ^ Note that such function is also found in regression techniques where the weights/coefficients are unknown. In ML, it is usually the case where both the function and its weights are unknown and are determined using various search techniques and algorithms.
Anderson, J. A. (1995). An introduction to Neural Networks . Cambridge, MA: MIT Press.
Google Scholar
Atiković, A., Kamenjašević, E., Nožinović, M. A., Užičanin, E., Tabaković, M., and Curić, M. (2020). Differences between all-around results in women's artistic gymnastics and ways of minimizing them. Balt. J. Health Phys. Act. 12, 80–91. doi: 10.29359/BJHPA.12.3.08
CrossRef Full Text | Google Scholar
Bartlett, R. (2006). Artificial intelligence in sports biomechanics: new dawn or false hope? J. Sports Sci. Med. 5, 474–479.
PubMed Abstract | Google Scholar
Beal, R., Norman, T. J., and Ramchurn, S. D. (2019). Artificial intelligence for team sports: a survey. Knowl. Eng. Rev. 34. doi: 10.1017/S0269888919000225
PubMed Abstract | CrossRef Full Text | Google Scholar
Berri, D. J., Schmidt, M. B., and Brook, S. L. (2004). Stars at the gate: the impact of star power on NBA gate revenues. J. Sports Econom. 5, 33–50. doi: 10.1177/1527002503254051
Bonaccorso, G. (2017). Machine Learning Algorithms . Birmingham: Packt Publishing Ltd.
Brandes, L., Franck, E., and Nuesch, S. (2008). Local heroes and superstars: an empirical analysis of star attraction in German soccer. J. Sports Econom. 9, 266–286. doi: 10.1177/1527002507302026
Brockett, C. L., Morgan, D. L., and Proske, U. W. E. (2004). Predicting hamstring strain injury in elite athletes. Med. Sci. Sports Exerc. 36, 379–387. doi: 10.1249/01.MSS.0000117165.75832.05
Brown, N., and Sandholm, T. (2019). Superhuman AI for multiplayer poker. Science 365, 885–890. doi: 10.1126/science.aay2400
Campbell, M., Hoane Jr, A. J., and Hsu, F. H. (2002). Deep blue. Artif. Intell. 134, 57–83. doi: 10.1016/S0004-3702(01)00129-1
Chmait, N. (2017). Understanding and measuring collective intelligence across different cognitive systems: an information-theoretic approach. in IJCAI (Melbourne), 5171–5172.
Chmait, N., Dowe, D. L., Li, Y. F., Green, D. G., and Insa-Cabrera, J. (2016). Factors of collective intelligence: how smart are agent collectives? in Proceedings of the Twenty-second European Conference on Artificial Intelligence (Prague), 542–550.
Chmait, N., Robertson, S., Westerbeek, H., Eime, R., Sellitto, C., and Reid, M. (2020a). Tennis superstars: the relationship between star status and demand for tickets. Sport Manag. Rev . 23, 330–347. doi: 10.1016/j.smr.2019.03.006
Chmait, N., Westerbeek, H., Eime, R., Robertson, S., Sellitto, C., and Reid, M. (2020b). Tennis influencers: the player effect on social media engagement and demand for tournament attendance. Telemat Inform. 50:101381. doi: 10.1016/j.tele.2020.101381
Coates, D., and Humphreys, B. R. (2012). Game attendance and outcome uncertainty in the National Hockey League. J. Sports Econom. 13, 364–377. doi: 10.1177/1527002512450260
Dezfouli, A., Nock, R., and Dayan, P. (2020). Adversarial vulnerabilities of human decision-making. Proc. Nat. Acad. Sci. U.S.A. 117, 29221–29228. doi: 10.1073/pnas.2016921117
Fister Jr, I., Ljubič, K., Suganthan, P. N., Perc, M., and Fister, I. (2015). Computational intelligence in sports: challenges and opportunities within a new research domain. Appl. Math. Comput. 262, 178–186. doi: 10.1016/j.amc.2015.04.004
Ghosh, J. K., Delampady, M., and Samanta, T. (2007). An Introduction to Bayesian Analysis: Theory and Methods . Berlin: Springer Science and Business Media.
Hakes, J. K., and Sauer, R. D. (2006). An economic evaluation of the Moneyball hypothesis. J. Econ. Perspect. 20, 173–186. doi: 10.1257/jep.20.3.173
Hattery, M. (2017). Major League Baseball players, big data, and the right to know: the duty of Major League Baseball teams to disclose health modelling analysis to their players. Marquette Sports Law Rev. 28, 257–283. https://scholarship.law.marquette.edu/sportslaw/vol28/iss1/9/
Hernández-Orallo, J., Baroni, M., Bieger, J., Chmait, N., Dowe, D. L., Hofmann, K., et al. (2017). A new AI evaluation cosmos: ready to play the game? AI Mag. 38, 66–69. doi: 10.1609/aimag.v38i3.2748
Jane, W.-J. (2016). The effect of star quality on attendance demand: the case of the National Basketball Association. J. Sports Econom. 17, 396–417. doi: 10.1177/1527002514530405
Jewell, R. T. (2017). The effect of marquee players on sports demand: the case of US Major League Soccer. J. Sports Econom. 18, 239–252. doi: 10.1177/1527002514567922
Konjer, M., Meier, H. E., and Wedeking, K. (2017). Consumer demand for telecasts of tennis matches in Germany. J. Sports Econom. 18, 351–375. doi: 10.1177/1527002515577882
Krause, L. (2019). Exploring the influence of practice design on the development of tennis players (Doctoral dissertation). Victoria University, Footscray, VIC, Australia.
Lapham, A. C., and Bartlett, R. M. (1995). The use of artificial intelligence in the analysis of sports performance: a review of applications in human gait analysis and future directions for sports biomechanics. J. Sports Sci. 13, 229–237. doi: 10.1080/02640419508732232
Lenten, L. J. (2012). Comparing attendances and memberships in the Australian Football League: the case of hawthorn. Econ Labour Relat. Rev. 23, 23–38. doi: 10.1177/103530461202300203
Lewis, M. (2004). Moneyball: The Art of Winning an Unfair Game . New York, NY: WW Norton and Company.
Lewis, M., and Yoon, Y. (2016). An empirical examination of the development and impact of star power in Major League Baseball. J. Sports Econom. 19, 155–187. doi: 10.1177/1527002515626220
Matthews, T., Ramchurn, S., and Chalkiadakis, G. (2012). Competing with humans at fantasy football: Team formation in large partially-observable domains. in Proceedings of the AAAI Conference on Artificial Intelligence , Vol. 26 (Vancouver, BC), 1394–1400.
McCabe, A., and Trevathan, J. (2008). Artificial intelligence in sports prediction. in Fifth International Conference on Information Technology: New Generations (IEEE: Las Vegas, NV), 1194–1197. doi: 10.1109/ITNG.2008.203
Nadikattu, R. R. (2020). Implementation of new ways of artificial intelligence in sports. J. Xidian Univ. 14, 5983–5997. doi: 10.2139/ssrn.3620017
Novatchkov, H., and Baca, A. (2013). Artificial intelligence in sports on the example of weight training. J. Sports Sci. Med. 12, 27–37.
Ormiston, R. (2014). Attendance effects of star pitchers in major league baseball. J. Sports Econom. 15, 338–364. doi: 10.1177/1527002512461155
Paton, D., and Cooke, A. (2005). Attendance at county cricket: an economic analysis. J. Sports Econom. 6, 24–45. doi: 10.1177/1527002503261487
Piccinotti, D. (2021). Open Loop Planning for Formula 1 Race Strategy Identification. Menlo Park, CA: Association for the Advancement of Artificial Intelligence.
Ratiu, O. G., Badau, D., Carstea, C. G., Badau, A., and Paraschiv, F. (2010). Artificial intelligence (AI) in sports, in Proceedings of the 9th WSEAS International Conference on Artificial Intelligence, Knowledge Engineering, and Data Bases (Cambridge, UK), 93–97.
Risi, S., and Preuss, M. (2020). From chess and Atari to StarCraft and beyond: how game AI is driving the world of AI. KI-Künstliche Intell. 34, 7–17. doi: 10.1007/s13218-020-00647-w
Schaeffer, J., Burch, N., Björnsson, Y., Kishimoto, A., Müller, M., Lake, R., et al. (2007). Checkers is solved. science 317, 1518–1522. doi: 10.1126/science.1144079
Silver, D., Schrittwieser, J., Simonyan, K., Antonoglou, I., Huang, A., Guez, A., et al. (2017). Mastering the game of go without human knowledge. Nature 550, 354–359. doi: 10.1038/nature24270
Swartz, T. B. (2020). Where should I publish my sports paper? Am. Stat. 74, 103–108. doi: 10.1080/00031305.2018.1459842
Vinyals, O., Babuschkin, I., Czarnecki, W. M., Mathieu, M., Dudzik, A., Chung, J., et al. (2019). Grandmaster level in StarCraft II using multi-agent reinforcement learning. Nature 575, 350–354. doi: 10.1038/s41586-019-1724-z
Whitaker, G. A., Silva, R., Edwards, D., and Kosmidis, I. (2021). A Bayesian approach for determining player abilities in football. J. R. Stat. Soc. Series C 70, 174–201. doi: 10.1111/rssc.12454
Yang, T. Y., and Swartz, T. (2004). A two-stage Bayesian model for predicting winners in major league baseball. J. Data Sci. 2, 61–73. doi: 10.6339/JDS.2004.02(1).142
Keywords: artificial intelligence, machine learning, sports business, sports analytics, sport research, future of sports
Citation: Chmait N and Westerbeek H (2021) Artificial Intelligence and Machine Learning in Sport Research: An Introduction for Non-data Scientists. Front. Sports Act. Living 3:682287. doi: 10.3389/fspor.2021.682287
Received: 18 March 2021; Accepted: 15 November 2021; Published: 08 December 2021.
Reviewed by:
Copyright © 2021 Chmait and Westerbeek. This is an open-access article distributed under the terms of the Creative Commons Attribution License (CC BY) . The use, distribution or reproduction in other forums is permitted, provided the original author(s) and the copyright owner(s) are credited and that the original publication in this journal is cited, in accordance with accepted academic practice. No use, distribution or reproduction is permitted which does not comply with these terms.
*Correspondence: Nader Chmait, nader.chmait@vu.edu.au
This article is part of the Research Topic
The Future of Sport Business
- Architecture and Design
- Asian and Pacific Studies
- Business and Economics
- Classical and Ancient Near Eastern Studies
- Computer Sciences
- Cultural Studies
- Engineering
- General Interest
- Geosciences
- Industrial Chemistry
- Islamic and Middle Eastern Studies
- Jewish Studies
- Library and Information Science, Book Studies
- Life Sciences
- Linguistics and Semiotics
- Literary Studies
- Materials Sciences
- Mathematics
- Social Sciences
- Sports and Recreation
- Theology and Religion
- Publish your article
- The role of authors
- Promoting your article
- Abstracting & indexing
- Publishing Ethics
- Why publish with De Gruyter
- How to publish with De Gruyter
- Our book series
- Our subject areas
- Your digital product at De Gruyter
- Contribute to our reference works
- Product information
- Tools & resources
- Product Information
- Promotional Materials
- Orders and Inquiries
- FAQ for Library Suppliers and Book Sellers
- Repository Policy
- Free access policy
- Open Access agreements
- Database portals
- For Authors
- Customer service
- People + Culture
- Journal Management
- How to join us
- Working at De Gruyter
- Mission & Vision
- De Gruyter Foundation
- De Gruyter Ebound
- Our Responsibility
- Partner publishers

Your purchase has been completed. Your documents are now available to view.
Journal of Quantitative Analysis in Sports
An official journal of the american statistical association.
- Online ISSN: 1559-0410
- Print ISSN: 2194-6388
- Type: Journal
- Language: English
- Publisher: De Gruyter
- First published: January 1, 2005
- Publication Frequency: 4 Issues per Year
Analyzing and Exploring the Impact of Big Data Analytics in Sports Sector
- Original Research
- Published: 31 March 2021
- Volume 2 , article number 184 , ( 2021 )
Cite this article
- Amandeep Kaur 1 ,
- Ramandeep Kaur 2 &
- Gagandeep Jagdev ORCID: orcid.org/0000-0001-9432-9648 3
1582 Accesses
9 Citations
Explore all metrics
Big data are the first-ever use of data and statistics enabling us to make effective decisions in professional sports. The primary focus of the research paper is to evaluate how the use of big data has benefited sports. The research work conducted in the paper focuses on extracting information from the constructed database as per user requirements. Two different databases have been constructed by gathering data from espncricinfo.com. The first database consists of the records of batsmen comprising 16 attributes relevant to the international career of the batsmen. The second database contains records of bowlers involving 18 attributes relevant to the international career of the bowlers. The research paper encompasses a detailed flowchart and an algorithm detailing the systematic approach followed to mine required data from the database. The research has been conducted focusing to come up with a user-friendly tool that would offer optimal results via simplified scripts and queries in tabular and visualization form. The research enables any cricket fan or a layman to mine the information in which he/she is interested. The research paper elaborates on the use of the Hortonworks Hue 2.2.0 framework in analyzing the gathered data and extracting information via appropriate tools of the framework as per need. The screenshots included in the research work enable the general masses to have insight into the workflow of the conducted research and to gain a better understanding of performing different functions on different tools. As compared to past research, the research work does not make use of any statistical tools which are difficult to understand and work upon. The constructed database is made available to the framework and the queries and scripts are formatted to get results in tabular and graphical form. The research work enables coaches and instructors to select the best batsmen and outstanding bowlers to construct a strong team via mining the relevant databases and come out with the best outcome. The research work even enables general masses to build their teams after analyzing the past performance of different batsmen and bowlers and construct their teams and participate in fantasy gaming platforms like Dream11, MyTeam11, Howzat, etc. The research paper illustrates the work conducted on sentiment analysis using Python and Java programming languages to predict the popularity of batsmen or bowlers via word cloud to get a deep insight into the public opinion regarding upcoming tournaments based on past performances of the players and the teams.
This is a preview of subscription content, log in via an institution to check access.
Access this article
Price includes VAT (Russian Federation)
Instant access to the full article PDF.
Rent this article via DeepDyve
Institutional subscriptions
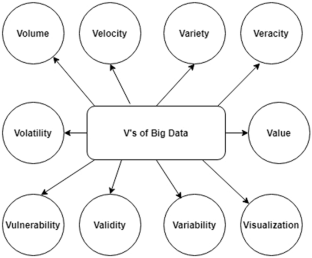
Similar content being viewed by others
Big Data from Collection to Use in Competitive Games—A Study Case on Badminton
The Application of Big Data Technology in Competitive Sports Research
Sports Data Analytics: A Case Study of off-Field Behavior of Players
Rein R, Memmert D. Big data and tactical analysis in elite soccer: future challenges and opportunities for sports science. Springer Plus. 2016;5(1410):1–13.
Google Scholar
Jhawar MG, Pudi V. Predicting the outcome of ODI cricket matches: a team composition based approach. In: European conference on machine learning and principles and practice of knowledge discovery in databases; 2016. pp. 1–11.
Kalgotra P, et al. Predictive modelling in sports leagues: an application in Indian Premier League. In: SAS Global Forum; 2013. pp. 1–11.
Podeszwa K. Top 20 big data cases in sport. Divante. 2016. http://divante.co/blog/top-20-big-data-cases-sport/ . Accessed 10 Jan 2021.
Hutchins B. Tales of the digital sublime: tacing the relationship between big data and professional sport. Convergence. 2016;22(5):494–509.
Article Google Scholar
Millington B, Millington R. The datafication of everything: toward a sociology of sport and big data. Sociol Sport J. 2015;32:140–60.
Liu Q, Xinsen H. Development strategy of competitive sports system in big data era. J Cap Inst Phys Educ. 2015;2:156–9.
Gandomi A, Haider M. Beyond the hype: big data concepts, methods and analytics. Int J Inf Manag. 2015;35(2):37–44.
Wu X, et al. Data mining with big data. IEEE Trans Knowl Data Eng. 2014;26(1):97–107.
Rajaraman UJD. Mining of massive datasets. Cambridge University Press; 2012.
Gupta M, George JF. Toward the development of a big data analytics capability. Inf Manag. 2016;53(8):1049–64.
Kent P, et al. Finding big value in big data: unlocking the power of high performance analytics. In: Liebowitz J, editor., et al., Big data and business analytics. CRC Press Taylor & Francis Group; 2013. p. 87–102.
Agarwal R, Dhar V. Big data, data science, and analytics: the opportunity and challenge for IS research. Inf Syst Res. 2014;25(3):443–8.
Chen HM, et al. Business intelligence and analytics: from big data to big impact. MIS Q. 2012;36(4):1165–88.
Constantiou ID, Kallinikos J. New games, new rules: big data and the changing context of strategy. J Inf Technol. 2015;30(1):44–57.
Singh S, Kaur P. IPL visualization and prediction using HBase. In: Information technology and quantitative management (ITQM2017), Procedia Computer Science; 2017. Vol. 122, pp. 910–915.
Agarwal S, et al. Cricket team prediction with hadoop: statistical modeling approach. In: Information technology and quantitative management (ITQM2017), Elsevier, Procedia Computer Science; 2017. Vol. 122, pp. 525–532.
Passi K, Pandey N. Increased prediction accuracy in the game of cricket using machine learning. Int J Data Min Knowl Manag Process. 2018;8(2):19–36.
Thenmozhi D, et al. Moneyball - data mining on cricket dataset. In: IEEE second international conference on computational intelligence in data science (ICCIDS-2019); 2019. pp. 1–6.
Hatharasinghe MM, Poravi G. Data mining and machine learning in cricket match outcome prediction: missing links. In: IEEE 5th international conference for convergence in technology (I2CT), Pune, India; 2019. pp. 1–4.
George G, et al. Big data and management. Acad Manag J. 2014;57(2):321–6.
Lytras MD, et al. Big-data and data analytics research: from metaphors to value space for collective wisdom in human decision making and smart machines. Int J Semant Web Inf Syst. 2017;13:1–10.
Gunther WA, et al. Debating big data: a literature review on realizing value from big data. J Strateg Inf Syst. 2017;26(3):191–209.
Bhimani A. Exploring big data’s strategic consequences. J Inf Technol. 2015;30(1):66–9.
Nandimath J, et al. Big data analysis using apache hadoop. In: IEEE IRI 2013, San Francisco, CA, USA; 2013. pp. 700–703.
https://thetechmusings.wordpress.com/2011/02/28/hadoop-an-inspirational-clone-of-gfs/hadoop_framework/ . Accessed 05 Jan 2021.
Wunderlich F, Memmert D. Innovative approaches in sports science—lexicon-based sentiment analysis as a tool to analyze sports-related twitter communication. Appl Sci. 2020;10(431):1–12.
Drus Z, Khalid H. Sentiment analysis in social media and its application: systematic literature review. In: The fifth information systems international conference 2019. Elsevier, Procedia Computer Science; 2019. Vol. 161, pp. 707–714.
Kumar A, Garg G. Systematic literature review on context-based sentiment analysis in social multimedia. Multimed Tools Appl. 2020;79:15349–80.
Agarwal A, Toshniwal D. Application of lexicon based approach in sentiment analysis for short tweets. In: IEEE international conference on advances in computing and communication engineering, Paris, France; 2018. pp. 189–193.
Rodrigues AP, Chiplunkar NN. A new big data approach for topic classification and sentiment analysis of Twitter data. In: Evolutionary Intelligence, Springer Verlag; 2019. pp. 1–11.
Download references
Author information
Authors and affiliations.
Guru Gobind Singh Khalsa College, Bhagta Bhai Ka, Bathinda, Punjab, India
Amandeep Kaur
Bhai Behlo Khalsa Girls College, Phephre Bhai Ke, Mansa, Punjab, India
Ramandeep Kaur
Punjabi University Guru Kashi College, Damdama Sahib, Bathinda, Punjab, India
Gagandeep Jagdev
You can also search for this author in PubMed Google Scholar
Corresponding author
Correspondence to Gagandeep Jagdev .
Ethics declarations
Conflict of interest.
On behalf of all authors, the corresponding author states that there is no conflict of interest.
Additional information
Publisher's note.
Springer Nature remains neutral with regard to jurisdictional claims in published maps and institutional affiliations.
This article is part of the topical collection “Applications of Cloud Computing, Data Analytics and Building Secure Networks” guest edited by Rajnish Sharma, Pao-Ann Hsiung and Sagar Juneja.
Rights and permissions
Reprints and permissions
About this article
Kaur, A., Kaur, R. & Jagdev, G. Analyzing and Exploring the Impact of Big Data Analytics in Sports Sector. SN COMPUT. SCI. 2 , 184 (2021). https://doi.org/10.1007/s42979-021-00575-y
Download citation
Received : 24 November 2020
Accepted : 09 March 2021
Published : 31 March 2021
DOI : https://doi.org/10.1007/s42979-021-00575-y
Share this article
Anyone you share the following link with will be able to read this content:
Sorry, a shareable link is not currently available for this article.
Provided by the Springer Nature SharedIt content-sharing initiative
- Data mining
- Sentiment analysis
Advertisement
- Find a journal
- Publish with us
- Track your research
sports analytics Recently Published Documents
Total documents.
- Latest Documents
- Most Cited Documents
- Contributed Authors
- Related Sources
- Related Keywords
Sports Analytics
Harnessing the predictive power of lower-division statistics of cricketers to predict their rates of success at the international level.
Abstract: Statistics has always been an integral part of the sporting world. Selectors pick players based on numerous factors such as averages, strike-rates, runs scored or goals scored. Teams have exclusive ‘talent hunters’, who spend weeks, if not months, trying to uncover talent from different parts of the world. With the rise of this new niche field called Sports Analytics, teams can now perform player evaluations on tons of data that is available. This paper aims to examine the factors that truly indicate the capacity of cricket players to perform at the top-most level – international cricket. Though this research has been carried out on cricket data, it is hoped that similar methods can be used to hunt for true talent in other sports! Keywords: Cricket Analytics, Random Forest, Principal Component Analysis, Dimensionality Reduction.
Evaluating Actions in Sports Analytics with Deep Learning
Storytelling with sports analytics.
Sports analytics in the wild (i.e., ubiquitously) is a thriving industry. Swing tracking is a key feature in sports analytics. Therefore, a centimeter-level tracking resolution solution is required. Recent research has explored deep neural networks for sensor fusion to produce consistent swing-tracking performance. This is achieved by combining the advantages of two sensor modalities (IMUs and depth sensors) for golf swing tracking. Here, the IMUs are not affected by occlusion and can support high sampling rates. Meanwhile, depth sensors produce significantly more accurate motion measurements than those produced by IMUs. Nevertheless, this method can be further improved in terms of accuracy and lacking information for different domains (e.g., subjects, sports, and devices). Unfortunately, designing a deep neural network with good performance is time consuming and labor intensive, which is challenging when a network model is deployed to be used in new settings. To this end, we propose a network based on Neural Architecture Search (NAS), called SwingNet, which is a regression-based automatic generated deep neural network via stochastic neural network search. The proposed network aims to learn the swing tracking feature for better prediction automatically. Furthermore, SwingNet features a domain discriminator by using unsupervised learning and adversarial learning to ensure that it can be adaptive to unobserved domains. We implemented SwingNet prototypes with a smart wristband (IMU) and smartphone (depth sensor), which are ubiquitously available. They enable accurate sports analytics (e.g., coaching, tracking, analysis and assessment) in the wild. Our comprehensive experiment shows that SwingNet achieves less than 10 cm errors of swing tracking with a subject-independent model covering multiple sports (e.g., golf and tennis) and depth sensor hardware, which outperforms state-of-the-art approaches.
Insights of the Olympics: Athens to Rio
Abstract: The Olympics is one of the eye-catching platforms for the sports community as well as sports’ lovers in the 21st Century. Sports also adopted the marvelous advancements that took place in vivid fields of technologies. In this modern era of analytics, every category demands prior research before indulging in the actual event. The sports analysis is the primary focus of this paper which mainly displays analysis of comprehensive data about different sport events involved in Olympics taking place from its beginning to the year 2016. The changing time brings new challenges and understanding the tactics along with the playing style of the opponent has become a necessity which basically constitutes to sports analytics. The analysis of the Olympics revealed certain riveting and fascinating facts referring to different aspects and areas. Numerous captivating results can be observed after carrying out research and analysis over the dataset of the Olympics. The analysis implies survey of age wise distribution of the competitors along with the winners' age classification. The medals tally list of countries with respective medals won till Rio Olympics is displayed and studied. In addition, the different venues which hosted the Olympics along with their respective year and number of participants is listed. The competitors with highest numbers of medals also contribute to our research's aim. The Indian Olympic medalists are also focused keeping their participation and winning ratio in consideration. With our research, we would like to light up unexposed and unexplored gospel. Keywords: Statistical Data, Sports Analytics, Data visualization, Factors, The Olympics
Estimating player value in American football using plus–minus models
Abstract Calculating the value of football player’s on-field performance has been limited to scouting methods while data-driven methods are mostly limited to quarterbacks. A popular method to calculate player value in other sports are Adjusted Plus–Minus (APM) and Regularized Adjusted Plus–Minus (RAPM) models. These models have been used in other sports, most notably basketball (Rosenbaum, D. T. 2004. Measuring How NBA Players Help Their Teams Win. http://www.82games.com/comm30.htm#_ftn1; Kubatko, J., D. Oliver, K. Pelton, and D. T. Rosenbaum. 2007. “A Starting Point for Analyzing Basketball Statistics.” Journal of Quantitative Analysis in Sports 3 (3); Winston, W. 2009. Player and Lineup Analysis in the NBA. Cambridge, Massachusetts; Sill, J. 2010. “Improved NBA Adjusted +/− Using Regularization and Out-Of-Sample Testing.” In Proceedings of the 2010 MIT Sloan Sports Analytics Conference) to estimate each player’s value by accounting for those in the game at the same time. Football is less amenable to APM models due to its few scoring events, few lineup changes, restrictive positioning, and small quantity of games relative to the number of teams. More recent methods have found ways to incorporate plus–minus models in other sports such as Hockey (Macdonald, B. 2011. “A Regression-Based Adjusted Plus-Minus Statistic for NHL players.” Journal of Quantitative Analysis in Sports 7 (3)) and Soccer (Schultze, S. R., and C.-M. Wellbrock. 2018. “A Weighted Plus/Minus Metric for Individual Soccer Player Performance.” Journal of Sports Analytics 4 (2): 121–31 and Matano, F., L. F. Richardson, T. Pospisil, C. Eubanks, and J. Qin (2018). Augmenting Adjusted Plus-Minus in Soccer with Fifa Ratings. arXiv preprint arXiv:1810.08032). These models are useful in coming up with results-oriented estimation of each player’s value. In American football, many positions such as offensive lineman have no recorded statistics which hinders the ability to estimate a player’s value. I provide a fully hierarchical Bayesian plus–minus (HBPM) model framework that extends RAPM to include position-specific penalization that solves many of the shortcomings of APM and RAPM models in American football. Cross-validated results show the HBPM to be more predictive out of sample than RAPM or APM models. Results for the HBPM models are provided for both Collegiate and NFL football players as well as deeper insights into positional value and position-specific age curves.
Random forest model identifies serve strength as a key predictor of tennis match outcome
Tennis is a popular sport worldwide, boasting millions of fans and numerous national and international tournaments. Like many sports, tennis has benefitted from the popularity of rigorous record-keeping of game and player information, as well as the growth of machine learning methods for use in sports analytics. Of particular interest to bettors and betting companies alike is potential use of sports records to predict tennis match outcomes prior to match start. We compiled, cleaned, and used the largest database of tennis match information to date to predict match outcome using fairly simple machine learning methods. Using such methods allows for rapid fit and prediction times to readily incorporate new data and make real-time predictions. We were able to predict match outcomes with upwards of 80%accuracy, much greater than predictions using betting odds alone, and identify serve strength as a key predictor of match outcome. By combining prediction accuracies from three models, we were able to nearly recreate a probability distribution based on average betting odds from betting companies, which indicates that betting companies are using similar information to assign odds to matches. These results demonstrate the capability of relatively simple machine learning models to quite accurately predict tennis match outcomes.
A Dockerized Big Data Architecture for Sports Analytics
Abstract The revolution of big data has also affected the area of sports analytics. Many big companies have started to see the benefits of combining sports analytics and big data to make a profit. Aggregating and processing big sport data from different sources becomes challenging if we rely on central processing techniques, which hurts the accuracy and the timeliness of the information. Distributed systems come to the rescue as a solution to these problems and the MapReduce paradigm is promising for large-scale data analytics. In this study, we present a big data architecture based on Docker containers in Apache Spark. We demonstrate the architecture on four data-intensive case studies including structured analysis, streaming, machine learning methods, and graph-based analysis in sport analytics, showing ease of use.
A Survey on Applications of Modern Deep Learning Techniques in Team Sports Analytics
Export citation format, share document.
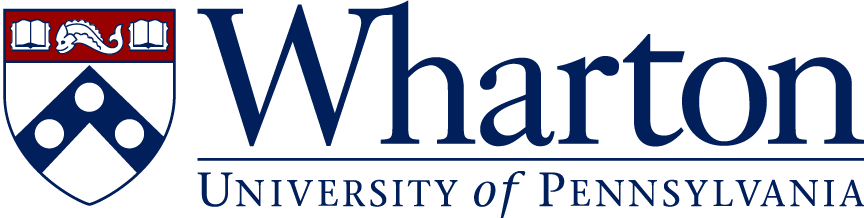
- Youth Program
- Wharton Online
Research projects in sports analytics allow our students to advance their understanding of statistics, dive into interesting datasets, and solve real business problems. WSABI aims to use insights from faculty and community to power the sports industry, both off and on the field of play.
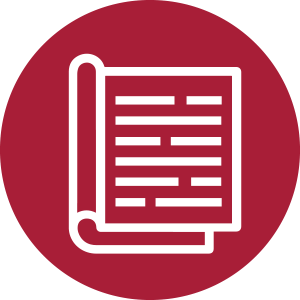
Published Research
By Ryan S. Brill (W’23), Sameer K. Deshpande and Abraham J. Wyner
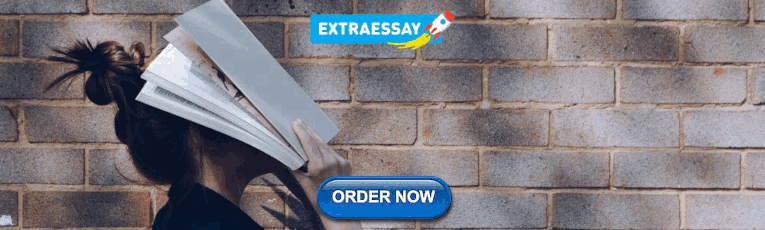
A Bayesian analysis of the time through the order penalty in baseball
Research focus: baseball, Bayesian statistics, mathematical modeling, pitching, time through order penalty
By Namita Nandakumar (W’18)
What Does It Mean To Draft Perfectly? An Evaluation Of Draft Strategy In The National Hockey League
Research focus: National Hockey League, amateur draft, game theory
By Sameer K. Deshpande* and Abraham Wyner
A hierarchical Bayesian model of pitch framing
Research focus: Baseball; Bayesian modeling; uncertainty quantification.
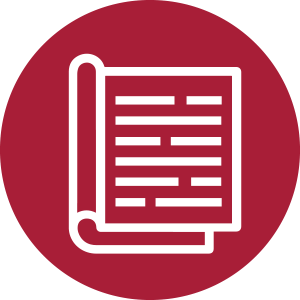
By Shane T. Jensen, Kenneth E. Shirley and Abraham J. Wyner
BAYESBALL: A BAYESIAN HIERARCHICAL MODEL FOR EVALUATING FIELDING IN MAJOR LEAGUE BASEBALL
Research focus: Spatial models, Bayesian shrinkage, baseball fielding
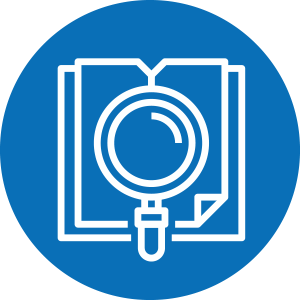
Wharton Moneyball Academy students Jake Federman, Eshan Mehere, Aidan Resnick, and Maxwell Resnick named 2021 Diamond Dollars Case Competition winners at the SABR Virtual Analytics Conference.
Research focus: Devise an improved metric for Game Score to evaluate the performance of starting pitchers.
Zach Bradlow (W22), Zach Drapkin (W22), Ryan Gross (PhD), Sarah Hu (W23) were finalists in the 2021 Big Data Bowl hosted by the National Football League
Research focus: Creating a relative skill rating system based upon success in coverage matchups to measure defender skill
Jake Flancer (W21) completed “NCAA Plus Minus”
January 2021
Using compiled data from college basketball R package bigballR, this project presents open source college basketball player +/- models, most notably the prior-informed sRAPM metric.
Matt Popowitz (C20) completed “RE+: Factoring Player and Team Hitting Ability into Run Expectancy and the True Value of a given Stolen Base”
September 2020
For years, run expectancy in a given inning was determined according to the base-out state. Now, we take into account both the current batter and the rest of the team’s offensive prowess. Using our findings, we are able to determine how valuable (or costly) a given stolen base attempt truly is!
Jake Flancer (W21) presented his work showcasing hockey analytics at the RIT Sports Analytics Conference
September 2019
A Bayesian Model for Estimating NHL Team Scoring
Jacob Richey (C21) presented his work at the Carnegie Mellon Sports Analytics Conference,
November 2019
Factoring Strength of Schedule into Player Analysis, Player elo, done in conjunction with Professor Adi Wyner
Jack Soslow (W19), Jake Flancer (W21), Eric Dong (ENG19), Andrew Castle (W21) were finalists in the inaugural Big Data Bowl hosted by the National Football League
Research focus: Using autoencoded receiver routes to optimize yardage
- Election 2024
- Entertainment
- Newsletters
- Photography
- Personal Finance
- AP Investigations
- AP Buyline Personal Finance
- AP Buyline Shopping
- Press Releases
- Israel-Hamas War
- Russia-Ukraine War
- Global elections
- Asia Pacific
- Latin America
- Middle East
- Election Results
- Delegate Tracker
- AP & Elections
- Auto Racing
- 2024 Paris Olympic Games
- Movie reviews
- Book reviews
- Personal finance
- Financial Markets
- Business Highlights
- Financial wellness
- Artificial Intelligence
- Social Media
Sports analytics may be outnumbered when it comes to artificial intelligence
Philadelphia 76ers’ Daryl Morey pauses while speaking at a news conference before an NBA basketball game against the Atlanta Hawks, Friday, Feb. 9, 2024, in Philadelphia. (AP Photo/Matt Slocum)
This March 2023 photo provided by the Sloan Sports Analytics Conference shows Sue Bird, center, posed with Jessica Gelman, left and Daryl Morley, right, after presenting Bird with the Lifetime Achievement Alpha Award at the 2023 Sloan Sports Analytics Conference in Boston. Jessica Gelman has become an influential leader and innovator in the sports industry. She co-founded the MIT Sloan Sports Analytics Conference. She teamed with Philadelphia 76ers President Daryl Morey to not only found Sloan, but guide it into the preeminent forum for number-crunchers. (Jeff Pinette/Sloan Sports Analytics Conference via AP)
Philadelphia 76ers’ Daryl Morey speaks during a news conference before an NBA basketball game against the Atlanta Hawks, Friday, Feb. 9, 2024, in Philadelphia. (AP Photo/Matt Slocum)
- Copy Link copied
BOSTON (AP) — When it comes to artificial intelligence, the sports analytics crowd may be outnumbered.
The people who killed the sacrifice bunt and turned NBA games into a 3-point shooting contest aren’t quite sure what will happen when AI fully invades sports — whether in the front office or on the field.
“I’ve been in computer science a long time. This is the first thing we don’t understand,” Philadelphia 76ers team president Daryl Morey said Friday at the MIT Sloan Sports Analytics Conference.
“That’s mind-boggling,” Morey said. “We’ve actually now created something, with 0’s and 1’s, where every step we’ve made the creation, but we don’t understand the results.”
The MIT conference annually brings together thousands of number-crunching sports nerds, who turn their data models loose on hot topics such as diversity , gambling or reversing the slowing pace of baseball games . But this year’s gathering had a decidedly AI focus, with panels and working papers on the potential for generative artificial intelligence to transform sports.
One talk looked at baseball strategy, another on how to provide Olympic content for the 200-plus countries competing in four dozen different sports, and a research paper used AI to provide player tracking data from a soccer broadcast.
Morey, one of the conference’s founders, was on a panel called “Winning with AI: The future of AI in sports.” The discussion touched on potential for improvements in scheduling, player safety, advertising, ticket sales and broadcasts that convert the on-field action into a Disney cartoon.
Kevin Lopes, an ESPN vice president for development and innovation, compared AI to the iPhone, which transformed everyday life by giving everyone with some coding skills the chance to come up with their own applications.
“I think about that when I think about generative AI,” Lopes said. “I don’t think anyone quite knows what that is yet. That’s fascinating to me, and what’s going to be the next thing.
“We exist in this moment in history, in my humble opinion, that every day we’re seeing new incremental innovations in AI,” he said. “What’s it going to be for the freshman at MIT in two years.”
Make no mistake, though: AI is already here.
Ballplayer-turned-broadcaster Carlos Peña said AI can be used to help a batter eliminate his blind spots. (But players will resist, he said, unless it’s stripped of its “mathiness” and translated into simple guidance such as, “look for the fastball up and in.”)
Anticipating complaints from scouts and others who say analytics can’t replace intuition, Pena said: “That’s not what we’re trying to do here. What we’re trying to do is enhance intuition.”
Christopher Jackson, the head of digital data and analytics for the Olympics, said AI can help create website content to satisfy fans of lower-profile sports from far-flung countries that usually don’t draw the attention of the mainstream media. One problem: Olympic planning is measured in decades, while major changes in AI come around every six months or so.
Amazon Web Services global head Julie Souza said the NFL is saving $2 million a year by running AI on its schedule, which has 1 quadrillion — that’s a one followed by 15 zeros — potential options that must account for holidays, shared stadiums and travel. AI is already dissecting which plays — and even which bodily poses — are most likely to cause injuries in a football game, she said.
“Rules are changing to make the game safer, to make the players more protected,” Souza said, adding that the information can trickle up from football to the military and others.
“It’s not just the NFL benefitting from this,” she said. “We’re not going back. There’s no way to go back from this. We’re just learning more and making the game safer. Which is great not just for the league, but for us on fans to have our players on the field more.”
Morey said the 76ers use AI for productivity — speeding up routine tasks — but it isn’t quite sharp enough yet to outdo the humans they have trying to improve their predictive modeling. “We haven’t found a ton there, but that will change,” he said.
And they will keep trying.
“There’s a lot of scary things with this, but it sort of is. This is happening,” Morey said. “There isn’t going to be a way to do all the safety stuff. … There isn’t actually going to be any way to control it. You really just lean into it, honestly, to help your business, to help what you’re doing.
“And there could be a very scary thing you hit. But what’s the alternative? Not embracing it?” he said. “That makes no sense.”
AP sports: https://apnews.com/sports

Get your ticket
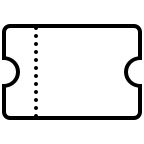
Research Papers Competition
The Sloan Sports Analytics Conference showcases cutting-edge research that is frequently featured in top media outlets throughout the world and has even changed the way sports are analyzed. The Research Papers Competition is an ideal way to build your reputation within the field of sports analytics.
This year’s competition will feature six sports tracks – Basketball, Baseball, Soccer, Football, Business of Sports, and Other Sports.
Abstract Submissions for SSAC24 are now closed
Abstracts are selected based on the novelty, academic rigor, and impact of the research.
All submissions are required to be open-source and a link to the author's GitHub repository or other repository supporting the research will be required.
Please refer to our Research Papers Rules page for full details on the submission and evaluation process. We look forward to reading your contribution!
Competition Format
The competition consists of the following phases:
- Abstract Phase
Authors submit abstracts. Based on the judged merits of their abstract submissions, a select group of authors will be invited to submit full manuscripts.
- Full Manuscript Phase
Invited authors submit full manuscripts. Referees will evaluate every manuscript, and authors of the best submissions will be invited to give a presentation on their findings at the conference. The referees will also select a separate set of authors who will be invited to present their work during a poster session, as well as a final set of three authors to give a deep-dive of their work in an open-source workshop.
- Conference Phase I
a. Presentations
Invited authors will present their findings during the first day of the conference. Based on the quality of the presentation and manuscript, one paper per sports track (see tracks below) and one wildcard will be selected to present at the conference in front of a panel of industry experts. The judge scores will be tabulated and the winners will be announced following presentations.
b. Poster Competition
All posters selected for the conference will be entered into a competition for Best Poster, determined by a combination of a fan and judges vote during the weekend of the conference.
Note: this competition is independent of the presentation finals, and none of the posters will advance to the presentation finals.
Timeline (all times Eastern Time)
Abstract submission due – Oct. 01, 2023, 11:59 p.m. EST
Full paper requests sent out – Mid-October 2023
Full paper submission due (if selected) – Dec. 01, 2023, 11:59 p.m. EST
Finalists and posters announced – Mid-January 2024
Submission of poster (if selected) – Early-February 2024
Submission of presentation (if selected) – Mid-February 2024
Conference presentations (if selected) – Conference Day
Open-Source Requirement
For the Sloan Sports Analytics Conference, the Research Papers competition has been a tremendous opportunity for researchers to both share their work with the community and improve the application of analytics across sports. We are excited to continue requiring all papers to be open-source for SSAC 2024 to further the impact of the great work of researchers in the industry.
Open-source research helps advance our mission to democratize analytics in sports by allowing researchers to build on top of the models and methods of their peers, both amplifying the effect of their research and better enabling widespread adoption of their work. We strongly believe that continued research into sports analytics is what makes our games more exciting and participants more effective.
All papers will be required to submit a link to the team's GitHub repository, or another open-source repository, with the data used to conduct the research. This should include any publicly available data or private data used in the research. For any private / proprietary data, please use your best judgement to anonymize any personal information before sharing publicly. The code running the models is not required to be submitted, but is encouraged, as it contributes to the communal spirit of open-source work by which researchers build off of each other's work to further the application of analytics across sports.
Sports Tracks
Based on abstract content, all submissions will be entered into one of the following Sports Tracks:
- Basketball – All submissions related to the sport of basketball.
- Baseball – All submissions related to the sport of baseball.
- Soccer – All submissions related to the sport of soccer.
- Football – All submissions related to the sport of American football.
- Business of Sports – All submissions related to the business of owning, managing, or marketing a sport, or to new technology or ideas which could change the face of the sport.
- Other Sports – All submissions related to the playing of a sport that is not included in the above Sports Tracks.
Abstract Guidelines
Abstract submissions should be submitted online, and must use the following guidelines:
- Abstracts must contain fewer than 500 words, including title and body.
- Abstracts may include up to two tables or figures combined (e.g. 1 figure and 1 table, or 2 tables).
- Each abstract should contain the following sections:
- Introduction – What question is this research trying to answer? Why is it an important question for the industry?
- Methods – Description of relevant statistical methods used, including data sources or data collection procedures
- Results – Description of actual (not promised) results along with relevant statistics
- Conclusion – The overall takeaway from the study, including how the results will impact the sports industry
Evaluation of Submissions
The conference seeks submissions that report research pertaining to the use of analytics in the sports industry. We are open to contributions ranging from evaluating players and game strategies, to examining the success factors for sports business. In the abstract and full paper submission process, research will be evaluated on, but not necessarily limited to, the following criteria:
- Novelty of research – Does the research provide interesting insight into new models or challenge existing beliefs?
- Academic rigor / validity of model – Are the methodologies of the model and results fundamentally sound and appropriate?
- Reproducibility – Can the model and results be replicated independently?
- Application – What are the applications or potential applications of the insights from the research?
In evaluating presentation finalists at the 2024 SSAC, the above factors will be supplemented by the following criteria, as judged by a panel of academics and industry executives from team management and sports business operations:
- Interest / impact – Is there significant interest in the proposed question in the field of study or the community at large? What are the benefits or impact of the model or application?
The Research Papers team will review all abstracts. The Review Committee will evaluate all manuscript submissions. The Review Committee consists of the Research Papers team, as well as academic professors and experts from top universities in fields including statistics, information sciences, and economics. The industry panel that makes the final winner selection will decide on the basis of the paper and the presentation at the 2024 Sloan Sports Analytics Conference. In these final evaluations, more weight will be given to the final presentation, specifically the highlighted application and impact of the research.
Conflict of Interest Policy
Our objective is to ensure an unbiased evaluation of submissions throughout the process. We are aware that members of the evaluation committee may have had relationships with authors who have submitted papers. When possible, potential conflicts of interest are avoided by minimizing the review of research by the following:
- Authors who have collaborated with the reviewer on previous submissions
- Current or former students who worked with the reviewer
- Colleagues from the same organization
- Any other previous relationships with the author that may prevent an unbiased evaluation of the paper
All potential conflicts of interest will be managed as best as possible while still maintaining the quality of the review process. Final reviews will occur without knowledge of the names of the authors.
Rights and Permissions
All authors retain ownership rights to the research and the right to publish the research after the conference. Upon submission, authors grant access to 42 Analytics to make their research available for public viewing online and in print, for conference use for the Sloan Sports Analytics Conference. Authors are responsible for obtaining permission from third parties to reprint copyrighted information such as data, tables, or figures that may be protected by copyright.
SSAC 2024 Research Papers & Authors Profiles
2024 research paper finalists, approaching in-venue quality tracking from broadcast video using generative ai.
Short Abstract: Over the last 25 years, soccer tracking data has provided a deeper understanding of the ways that players and teams play the game. Although traditional tracking systems require in-venue installation, there is a current push to track players remotely from broadcast footage. However, tracking data obtained from broadcast footage is inherently incomplete due to players being out of the broadcast camera’s field of vision. We address this issue in this paper, leveraging generative AI to predict highly accurate locations of the players for the large portions of games where they cannot be visually perceived.
Author(s): Harry Hughes
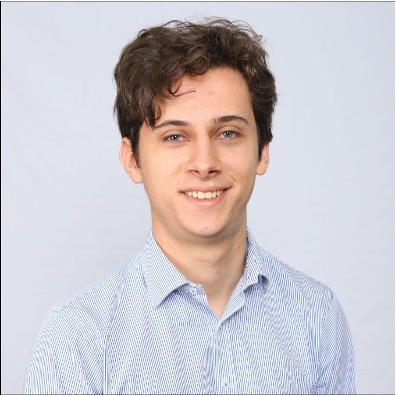
Harry Hughes is a doctoral student focusing on how modern artificial intelligence techniques can be applied to sports data. With an undergraduate degree in Software Engineering at the University of Queensland, he is currently working at Stats Perform developing the company's broadcast tracking system.
Michael Horton
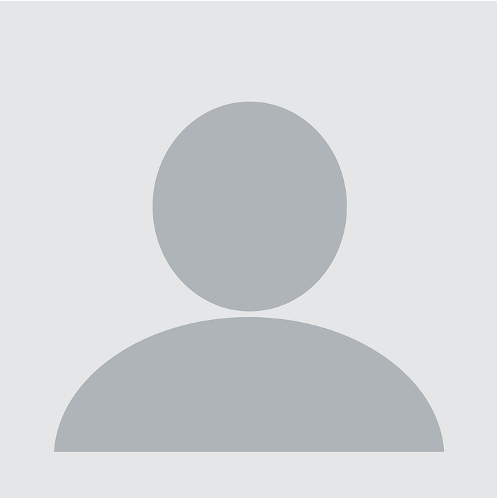
Michael Stokl
Harshala Gammulle

Clinton Fookes

Sridha Sridharan
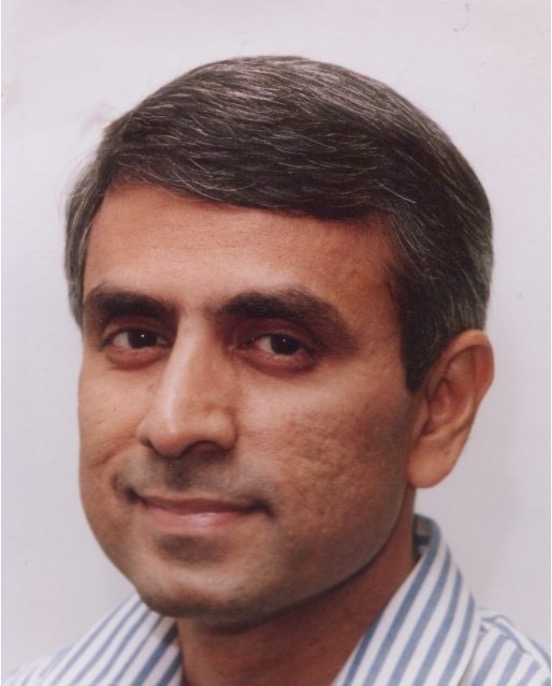
Sateesh Pedagadi

Patrick Lucey
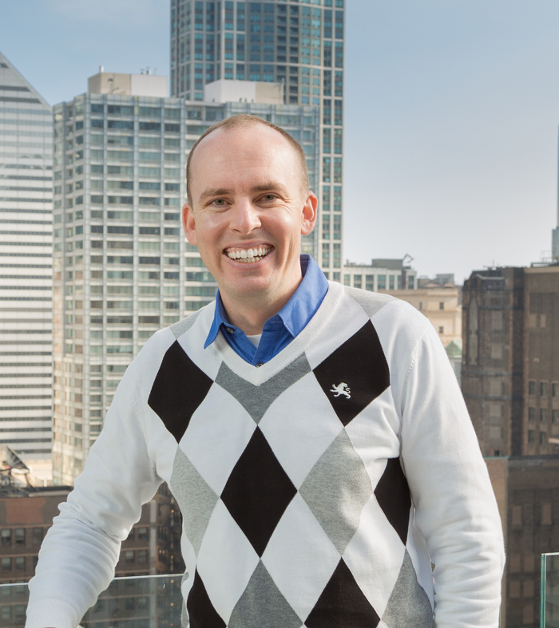
Estimating NBA Team Shot Selection Efficiency from Aggregations of True, Continuous Shot Charts: A Generalized Additive Model Approach
Short Abstract: We develop a novel type of basketball shot chart, a true shot chart, that uses a generalized additive model (GAM) to estimate total shot proficiency continuously in the half-court as a continuous, 3-D surface ( https://sportdataviz.syr.edu/TrueShotChart/ ). Unlike conventional shot charts, which do not consider free throw scoring pursuant to a shot from a given location, true shot charts incorporate total points, from the field and free throw line, pursuant to each shot in an NBA game (from 2016-2022 in the study) toward improved explanatory power of offensive efficiency variation across NBA team-seasons. Whereas conventional shot charts show a league-wide three-point premium over the period of the data, true shot charts show a deepening dispremium since 2018, as the free throw rate for three-point attempts is substantially less than that for two-point attempts. Lastly, we develop a novel shot chart summary measure, shot selection efficiency, as the Pearson correlation between expected proportional volume and expected true points, from the field and free throw line, across the half court space; polynomial regression and XGBoost modeling suggest shot selection efficiency is not only win productive, but a “Moneyball” or partly supra-payroll source of wins.
Author(s): Justin Ehrlich

Dr. Justin A. Ehrlich is an associate professor specializing in sport analytics, machine learning, and computer science. His diverse research spans virtual reality, 3D human pose estimation, advanced visualization, ranking and rating in sports, the business of sport, and the analysis of risks associated with developing Chronic Traumatic Encephalopathy (CTE) in football players. As a faculty member in Syracuse University's Big Data Cluster, Dr. Ehrlich continues to contribute significantly to the field, focusing on big data, rating and ranking methodologies, on-field performance analysis, and advanced shot charts and visualizations. His dedication to advancing sport analytics is evident in the breadth and impact of his research contributions, including innovative approaches to understanding and visualizing player performance on the field.
Shane Sanders
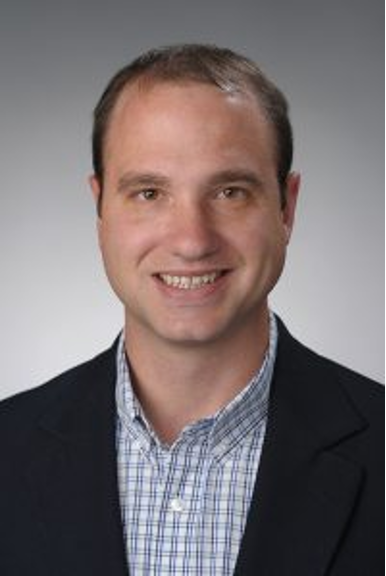
Dr. Shane Sanders is a Professor of Sport Analytics at Syracuse University and an author at sportquant.substack.com. During the summer months, Sanders has done extensive consulting on player acquisition in professional basketball and has published leading academic work in sports economics, statistics, and game theory. In total, he has authored or co-authored 80+ articles in leading journals of these fields, as well as a popular economics book, The Economic Reason. His work has been cited on NPR, in USA Today, in a U.S. Supreme Court sports antitrust case, and other prominent outlets. When not thinking about himself, Sanders–along with his wife, Bhavneet–helps coach his older daughter, Simran, for various middle school spelling, math, and science pursuits. Last year, Simran qualified for and placed well at the Scripps National Spelling Bee. Sanders also helps coach his younger daughter, Nanki, in soccer skills and in her budding academic interests. Hailing from Zionsville, Indiana–”land of Brad Stevens”– Sanders and his brethren have always been disproportionately crazy about basketball. Under the influence of this so-called “hysteria,” his parents, Dennis and Debby, actually bought and maintained an old high school gym for two decades strong.
Feeling the Pressure: A Unified Framework for Automating Pass Rushing Statistics in NFL Games
Short Abstract: In spite of the importance of the pass rush in professional football, pass rushing statistics only include the final outcomes of a play, e.g., sack and pass-made. They do not capture the dynamics of the pass rush or fine-grained insights throughout a play on how much pressure a rusher generates during the rush. In this paper, we propose a unified framework that enables the estimation of defensive pressure scores throughout a play with high accuracy and performance for rusher and blocker identification, rusher-blocker match-up and pressure score estimation and show the real-world applications of our framework including enriched analytics.
Author(s): Sungmin Hong
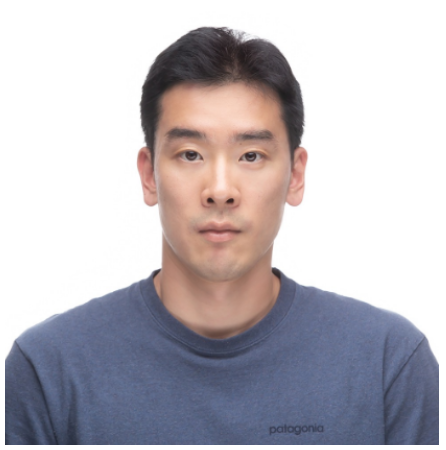
Sungmin Hong is an Applied Scientist at Amazon Generative AI Innovation Center where he helps expedite the variety of use cases of AWS customers. Before joining Amazon, Sungmin was a postdoctoral research fellow at Harvard Medical School. He holds a Ph.D. in Computer Science from New York University. Outside of work, Sungmin enjoys hiking, reading and cooking.
Laura Kulowski

Laura Kulowski is an Applied Scientist at Amazon’s Generative AI Innovation Center, where she works closely with customers to build generative AI solutions. She holds a PhD in Earth and Planetary Sciences from Harvard University. In her free time, Laura enjoys biking and skiing.
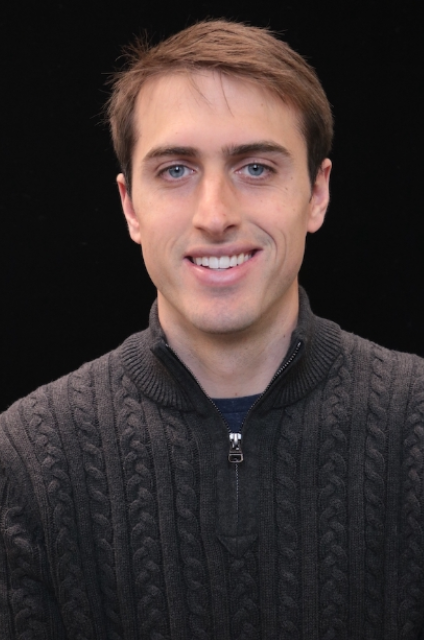
Dan Volk is a Data Scientist at the AWS Generative AI Innovation Center, where he leverages generative AI to create novel solutions to complex problems. He has ten years of experience in machine learning, deep learning and time-series analysis and holds a Master’s in Data Science from UC Berkeley. Outside of work, Dan is a backpacker, snowboarder, mountain biker, drummer, and lifelong fan of all Seattle sports. Bring back the Sonics!
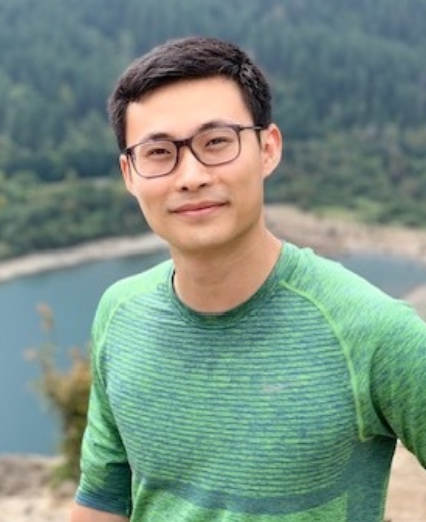
Henry Wang is an Applied Scientist at the AWS Generative AI Innovation Center, where he builds innovative GenAI solutions and co-leads Sports vertical with Dan. He holds a Master’s in Computational Science and Engineering from Harvard University. Outside of work, he loves to play golf casually and compete in tennis at amateur level.
Keegan Abdoo
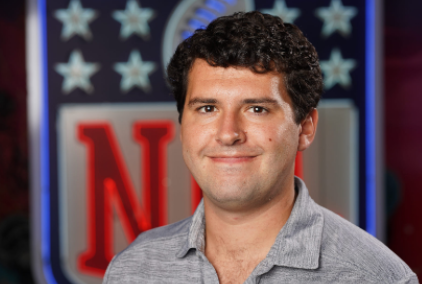
Keegan Abdoo is a Manager of Research and Analytics in the Next Gen Stats department at the National Football League. He has helped build out the Next Gen Stats platform over the last six seasons and was promoted to his current role in January 2023. Coming from a background of charting football, Keegan has strived to expand the NGS toolbox to classify more schematic data. Outside of work, he enjoys skiing, live music, and exploring all of the great restaurants Los Angeles has to offer.
Conor McQuiston
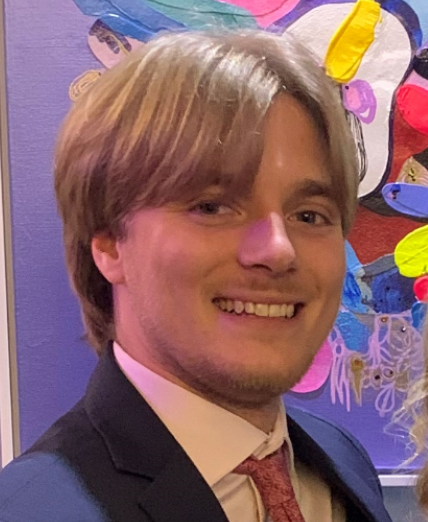
Conor McQuiston is a Research Analyst in the Next Gen Stats department at the National Football League. Since joining Next Gen Stats in October 2022, Conor has used his physics background to help the team to develop and communicate new tracking data metrics to NFL media and all 32 clubs. Prior to joining NGS, he interned as an analytics assistant with the Arizona Cardinals and as a football research intern with Pro Football Focus (PFF). Outside of work, he enjoys going to the beach, reading about history, and trying out new recipes.
Kyeong Hoon (Jonathan) Jung
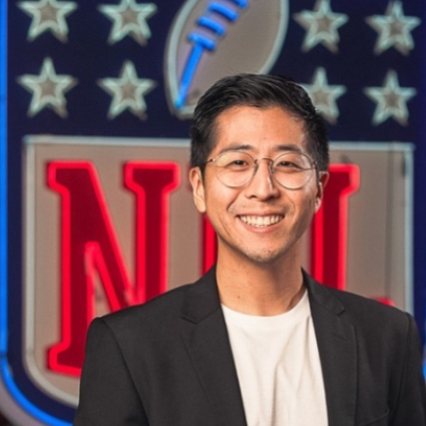
Kyeong Hoon (Jonathan) Jung is a Principal Software Engineer at the National Football League. He has been with the Next Gen Stats team for the last eight years helping to build out the platform from streaming the raw data, building out microservices to process the data, to building API's that exposes the processed data. He has collaborated with the Amazon Machine Learning Solutions Lab in providing clean data for them to work with as well as providing domain knowledge about the data itself. Outside of work, he enjoys cycling in Los Angeles and hiking in the Sierras.
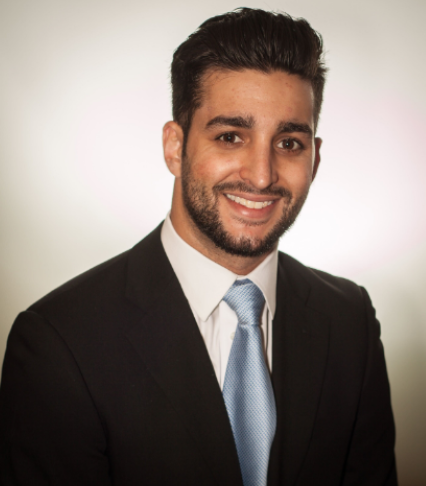
Mike Band is a Senior Manager of Research and Analytics for Next Gen Stats at the National Football League. Since joining the team in 2018, he has been responsible for ideation, development, and communication of key stats and insights derived from player-tracking data for fans, NFL broadcast partners, and the 32 clubs alike. Mike brings a wealth of knowledge and experience to the team with a master's degree in analytics from the University of Chicago, a bachelor's degree in sport management from the University of Florida, and experience in both the scouting department of the Minnesota Vikings and the recruiting department of Florida Gator Football.
Diego Socolinsky
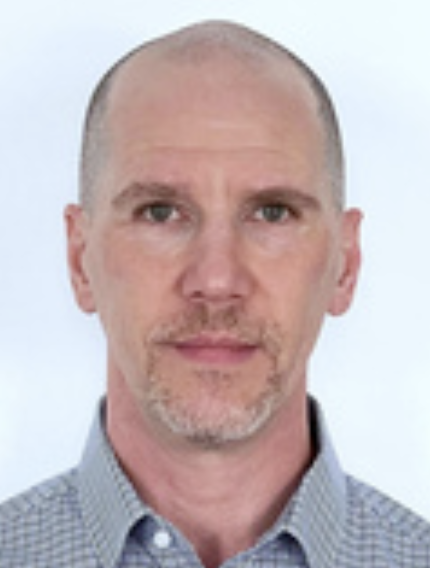
Diego Socolinsky is a Senior Applied Science Manager with the AWS Generative AI Innovation Center, where he leads the delivery team for the Eastern US and Latin America regions. He has over twenty years of experience in machine learning and computer vision, and holds a PhD degree in mathematics from The Johns Hopkins University.
Measuring Individual Competitiveness and its Impact on Sporting Success
Short Abstract: Although the sports industry pours millions of dollars into understanding talent, we do not know: how to measure individuals’ attitudes towards competition, when these attitudes are formed, how they vary both within individuals over time and across individuals, and, more fundamentally, how important competitiveness is for sporting success. We measure competitiveness and answer these questions by leveraging a rich, dynamic panel dataset on hundreds of top young prospects from a renowned professional soccer academy during the decade leading up to professionalism. The ideas and methods are applicable to all other sports.
Author(s): Julene Palacios-Saracho
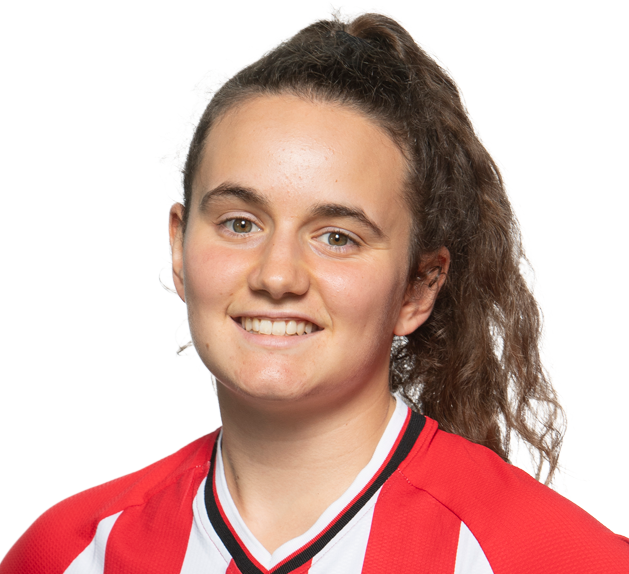
Born in Gorliz (Spain). Currently studying a joint degree BA in Business Economics and BSc in Industrial Engineering. I love two intersections: (1) between math, economics and engineering, and (2) between science and sports. Played my first game in Spain’s professional soccer leagues at age 15. My hobbies include reading science (particularly, physics) and music (soundtracks). I play soccer for Athletic Bilbao Women and I am a big fan of Athletic Bilbao.
Ander Palacios-Saracho

Born in Gorliz (Spain). Currently studying a BSc in Data Science and Artificial Intelligence. Fascinated by the power of data, analytics, and recent advances in technology to try to answer all types of scientific questions, in both the social and natural sciences. Hobbies include soccer, bodyboarding, and free-diving in underwater kelp forests to observe octopus. I am a big fan of Athletic Bilbao, Nottingham Forest, Liverpool FC, and Tadej Pogacar.
No More Throwing Darts at the Wall: Developing Fair Handicaps for Darts using a Markov Decision Process
Short Abstract: Darts is a popular sport that caters to players of all different abilities, and it is therefore common for opponents to have mismatched skill levels. Handicaps are useful interventions that address this mismatch, keeping the game competitive by increasing the weaker player’s chances of winning. However, the design of handicaps in darts has historically been a lot like “throwing darts at the wall” with no rigorous approach. To fill this gap, we develop a framework to model the game of darts with different handicaps, allowing us to evaluate current approaches and design a novel, fairer handicap system.
Author(s): Timothy C.Y. Chan
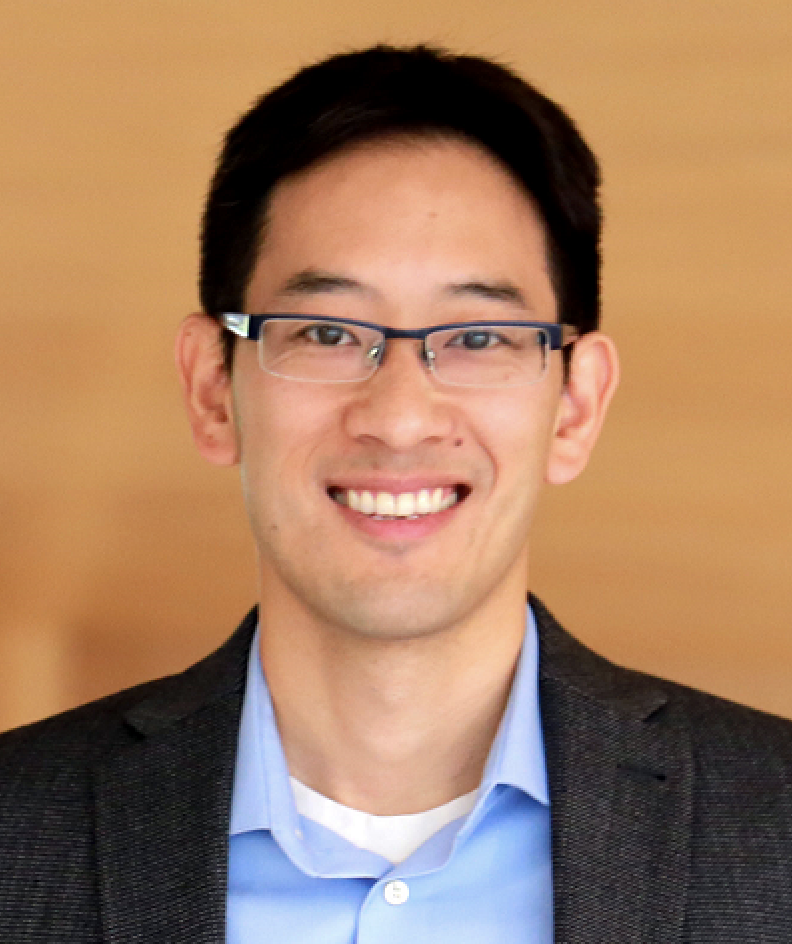
Timothy Chan is the Associate Vice-President and Vice-Provost, Strategic Initiatives at the University of Toronto, the Canada Research Chair in Novel Optimization and Analytics in Health, a Professor in the Department of Mechanical and Industrial Engineering, and a Senior Fellow of Massey College. His primary research interests are in operations research, optimization, and applied machine learning, with applications in healthcare, medicine, sustainability, and sports. Along with co-author Doug Fearing, he received the MIT Sloan Sports Analytics Conference research paper award in 2013. He recently got back into playing tennis and he is never going back.
Craig Fernandes
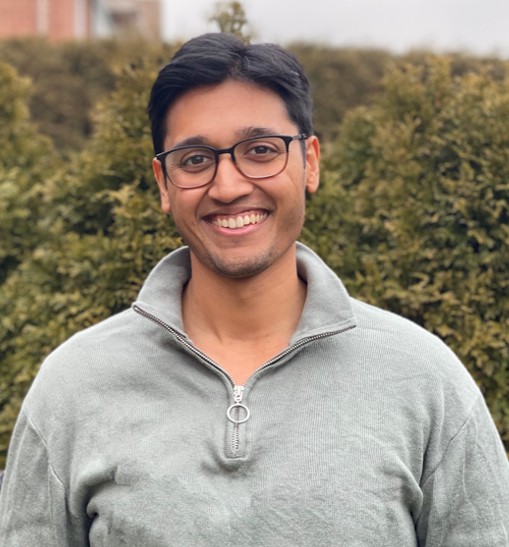
Craig Fernandes is a third-year Operations Research PhD Candidate and Vanier Scholar at the University of Toronto supervised by Profs. Timothy Chan and Ningyuan Chen. His research focuses on optimization, game theory & AI/ML techniques applied in economics, emerging markets, and sports. His research has been featured at MIT's Sloan Sports Analytics Conference (SSAC), the New England Symposium on Statistics in Sports (NESSIS), and the Sport Innovation (SPIN) Summit hosted by Own the Podium. He also previously worked as a research data scientist at Amazon and will be conducting a research visit this summer at Dartmouth College's Tuck School of Business. He picked up golf during the pandemic and is now an enthusiast.
Rachael Walker
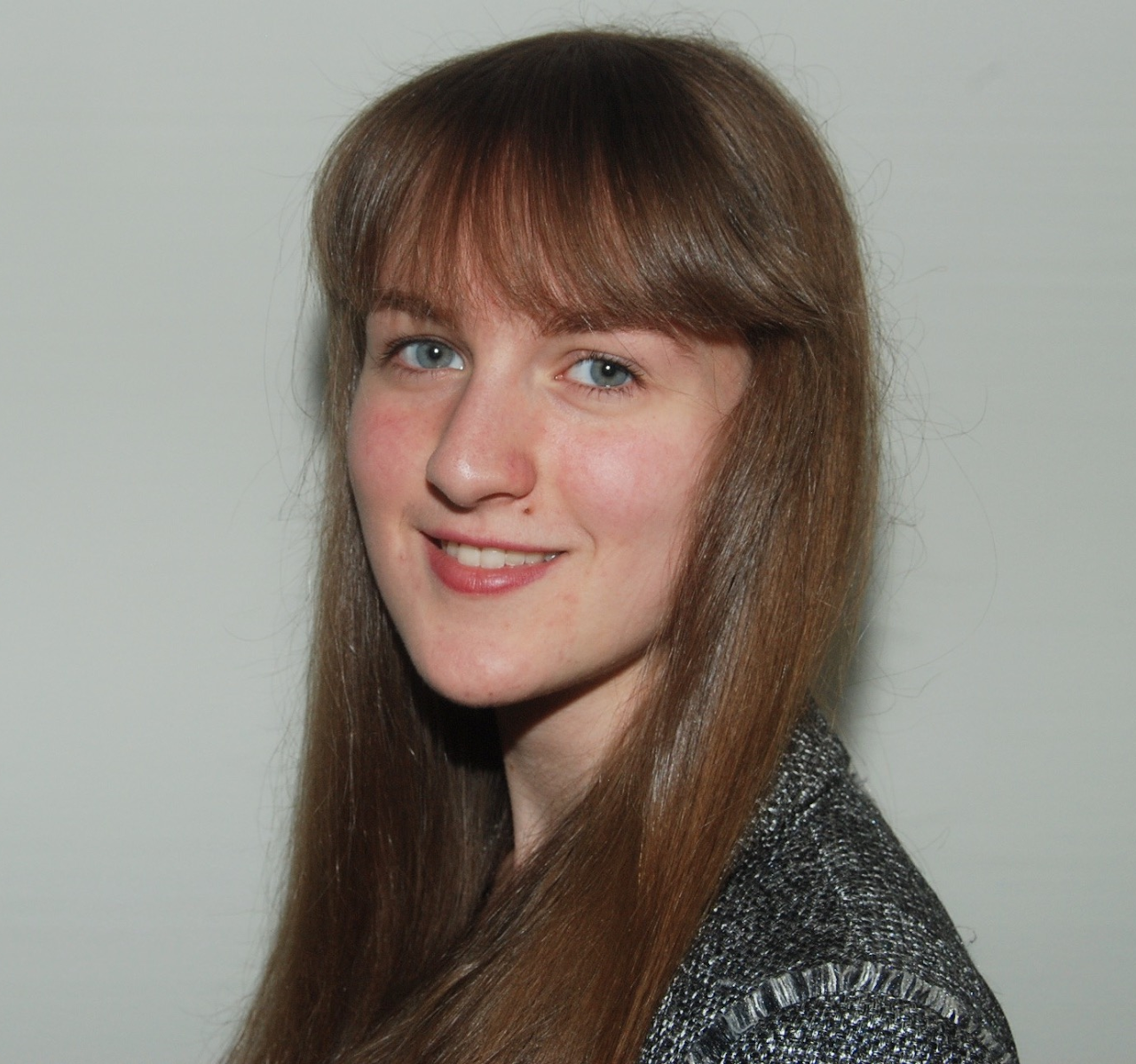
Rachael Walker graduated from the University of Toronto with a BASc in Industrial Engineering and a minor in Artificial Intelligence Engineering. For her undergraduate thesis, she researched the application of stochastic optimization models in sports. Specifically, she examined how Markov models can be used to design fair handicap systems in the game of darts. Rachael recently started a new role on the data science team at the private equity firm Birch Hill Equity Partners. She is also an undeterred fan of the Toronto Maple Leafs.
Optimizing Baseball Fielder Positioning with Consideration for Adaptable Hitters
Short Abstract: This paper presents a novel approach to positioning baseball fielders to maximize expected outs or minimize expected runs allowed against an opposing hitter. We find evidence that our positioning approach is an improvement over MLB average positioning in terms of both hits and runs allowed. We then extend our approach to adaptable hitters who adjust their batted ball strategy in response to the defense’s positioning strategy by modeling the interaction as a zero-sum game and solving for an equilibrium pair of strategies. We demonstrate two examples where the game theory model is appropriate: against shift-beating hitters who pull the ball less frequently when the defense shifts against them and against pull-heavy left-handed hitters who threaten to bunt against an extreme shift.
Author(s): William Melville
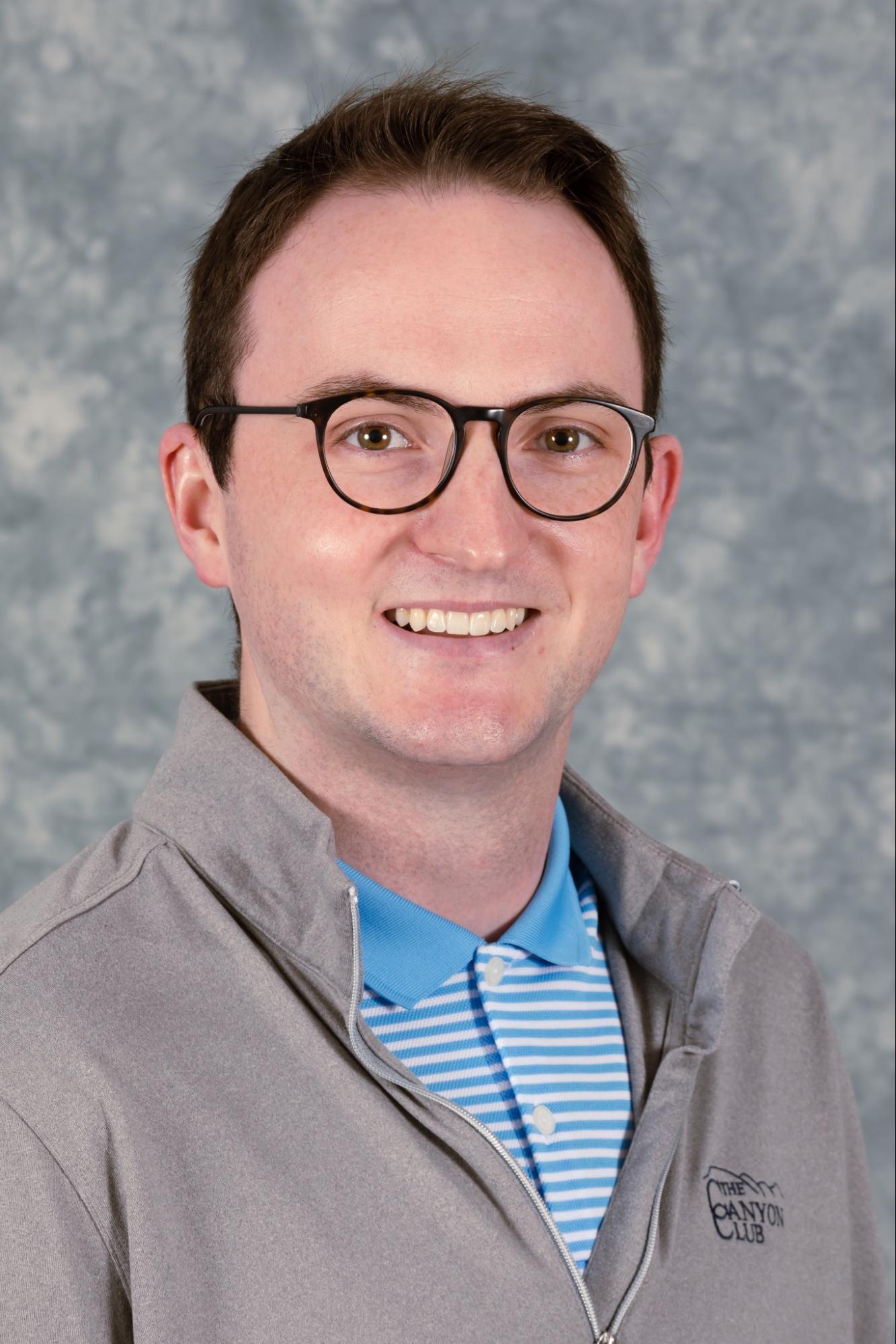
William Melville received his undergraduate degree in applied and computational mathematics at BYU in 2020 before starting a job as an analyst with the Texas Rangers. He returned to BYU in 2022 where he is currently pursuing a PhD in computer science. His research focuses on applications of game theory to baseball strategy. His passion for baseball runs much deeper than just the analytics; he also has a great appreciation for the equipment of the game and makes wooden bats in his home woodshop.
Samuel Wise

Samuel Wise is an undergraduate at Brigham Young University in his final semester as a Statistics major with an emphasis in Data Science and a minor in Economics. He is from Walnut Creek, California and enjoys exploring his passion for sports through data analytics. He recently has been interested in ranking algorithms and their applications in sports. He is an avid fan of football and the New Orleans Saints, as well as basketball and the UFC. When he is not watching sports, he is watching movies and playing video games with his friends.
Grant Nielson
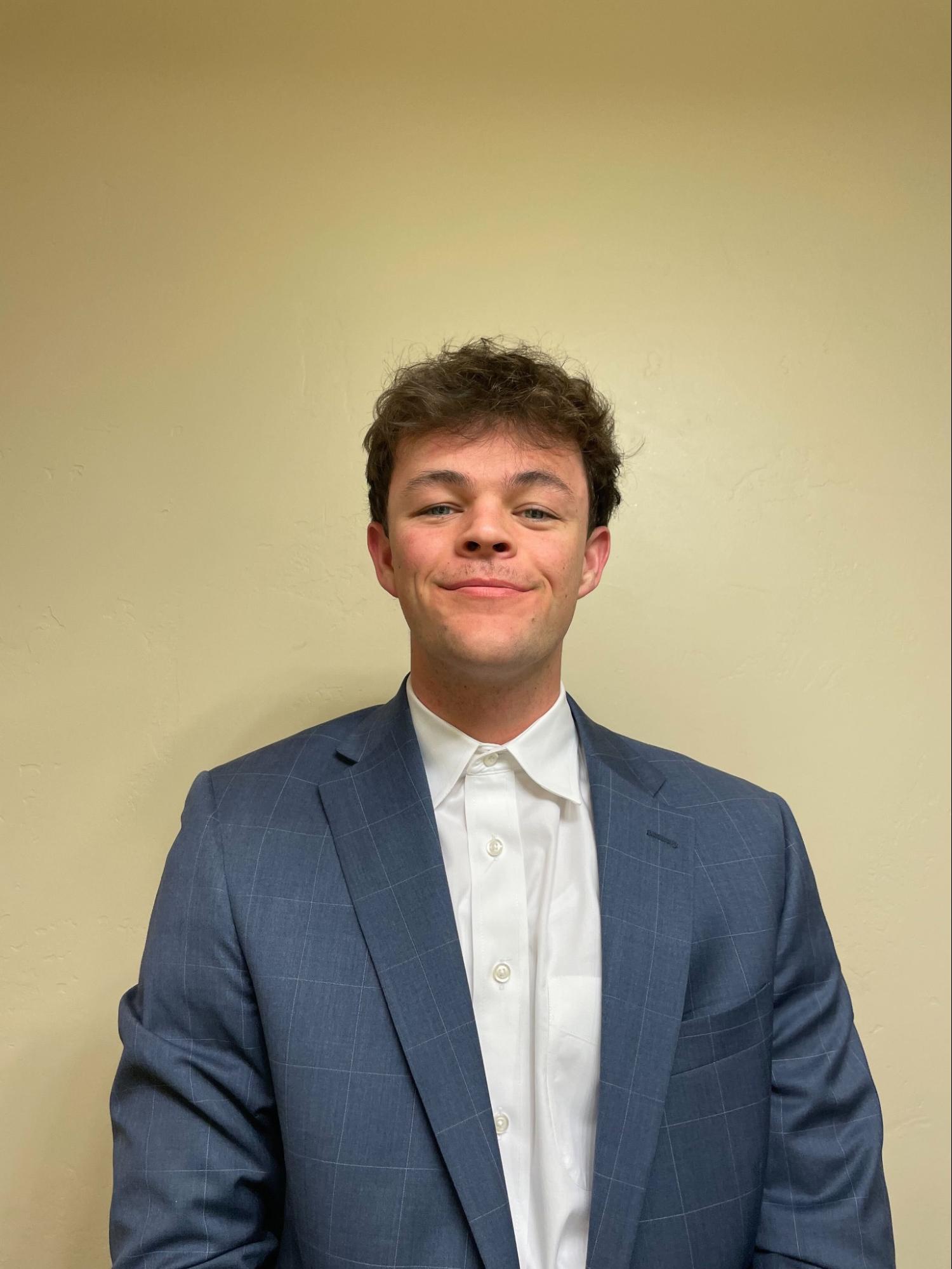
Grant Nielson is in his final undergraduate year studying Statistics at BYU. He has enjoyed this past year working on baseball projects with IDeA labs, helping him towards his goals of attending grad school and working with a major league team. Having Dallas roots, his favorite memory is watching his Texas Rangers win the World Series in person last year. He also enjoys biking along the Wasatch Front, watching Moneyball, and playing piano and basketball.
Tristan Mott

Tristan Mott grew up in Austin, TX and later moved to Alpine, UT. He enjoys spending his free time traveling, backpacking, and fly fishing. In his third year at BYU, he is working on undergraduate degrees in computer engineering (major) and computer science (minor). He is passionate about playing and watching sports and loves working on research projects for the Texas Rangers and BYU baseball teams. He aspires to someday receive a PhD in computer science so that he can make contributions to the fields of sports, machine learning, and game theory.
Christopher Archibald

Christopher Archibald is an Assistant Professor of Computer Science at BYU, where he has been since 2019. His research focuses on Artificial Intelligence and Strategic Reasoning, including Sports Analytics. He received his undergraduate degree in Computer Engineering from BYU in 2006 and a PhD in Computer Science from Stanford University in 2011 under the supervision of Yoav Shoham. From 2011 to 2013 he was a Postdoctoral Fellow at the University of Alberta under the supervision of Michael Bowling. From 2013 to 2019 he was an assistant professor at Mississippi State University. He enjoys teaching, playing games with his kids, and learning about obscure sports.
David Grimsman
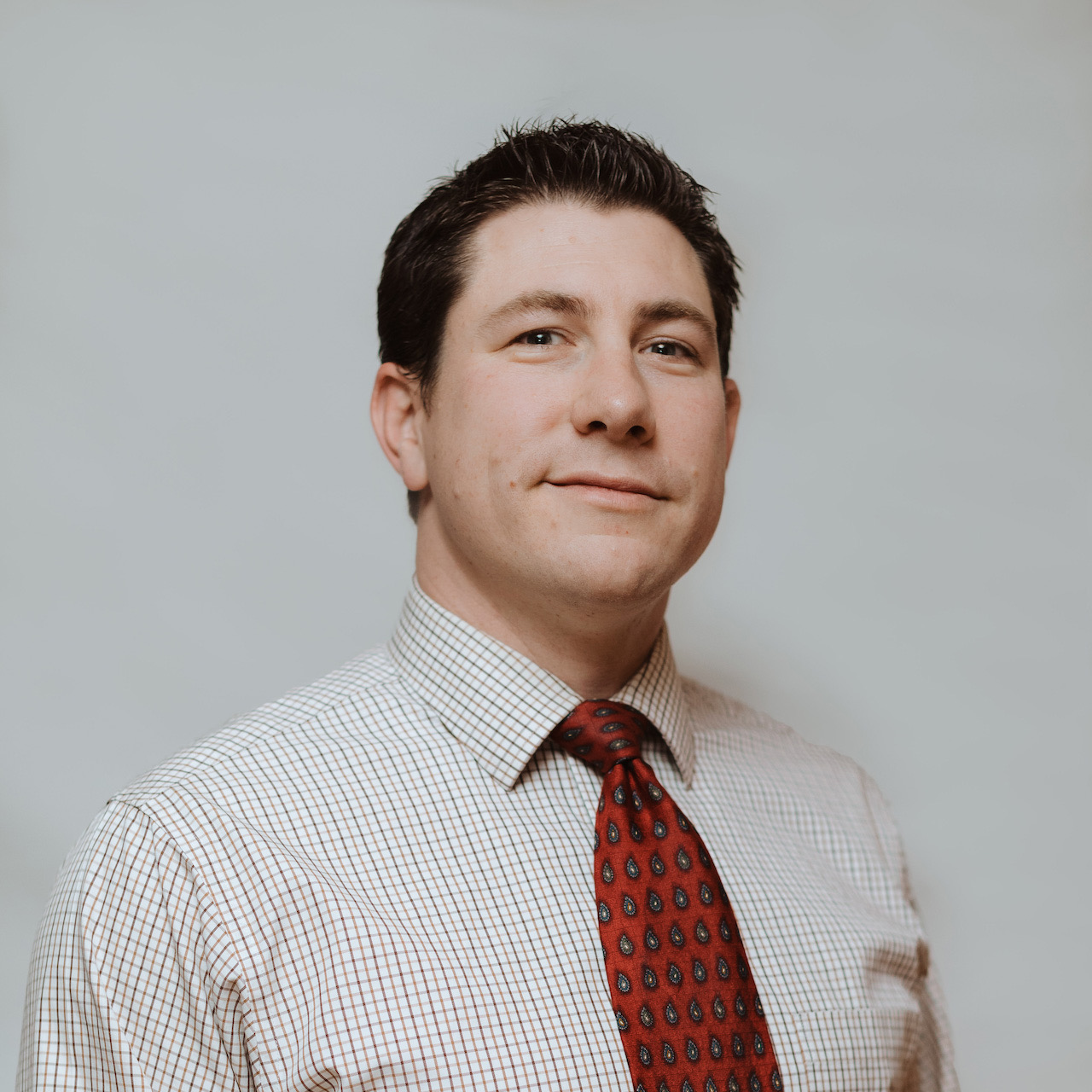
David Grimsman is an Assistant Professor in the Computer Science Department at Brigham Young University. He completed BS in Electrical and Computer Engineering at Brigham Young University in 2006 as a Heritage Scholar, and with a focus on signals and systems. After working for BrainStorm, Inc. for several years as a trainer and IT manager, he returned to Brigham Young University and earned an MS in Computer Science in 2016. He then received his PhD in Electrical and Computer Engineering from UC Santa Barbara in 2021. His research interests include multi-agent systems, game theory, distributed optimization, network science, linear systems theory, and security of cyberphysical systems.
The Strain of Success: A Predictive Model for Injury Risk Mitigation and Team Success in Soccer
Short Abstract: Player injuries in soccer significantly impact team performance, club financial stability and player welfare, with the ‘Big Five’ European soccer leagues experiencing a staggering £513 million in injury-related costs during the 2021/22 season. In this paper, we present a novel forward-looking team selection model, framed as a Markov decision process and optimised with Monte Carlo tree search, that balances team performance with the risk of long-term player unavailability due to injury. We demonstrate that real-world teams could reduce the incidence of player injury by ~13% and wages inefficiently spent on injured players by ~11% using our data-driven team selection model.
Author(s): Gregory Everett

Gregory is a PhD candidate at the University of Southampton, where his research is focused on the use of AI to optimize team performance in soccer. Gregory has published papers previously in this field, focusing on optimizing collective team positioning and predicting player behaviour. He has also gained practical experience through an internship at SentientSports, and has a passion for the development of AI models to enhance the effectiveness of operational processes within soccer clubs.
Dr. Ryan Beal
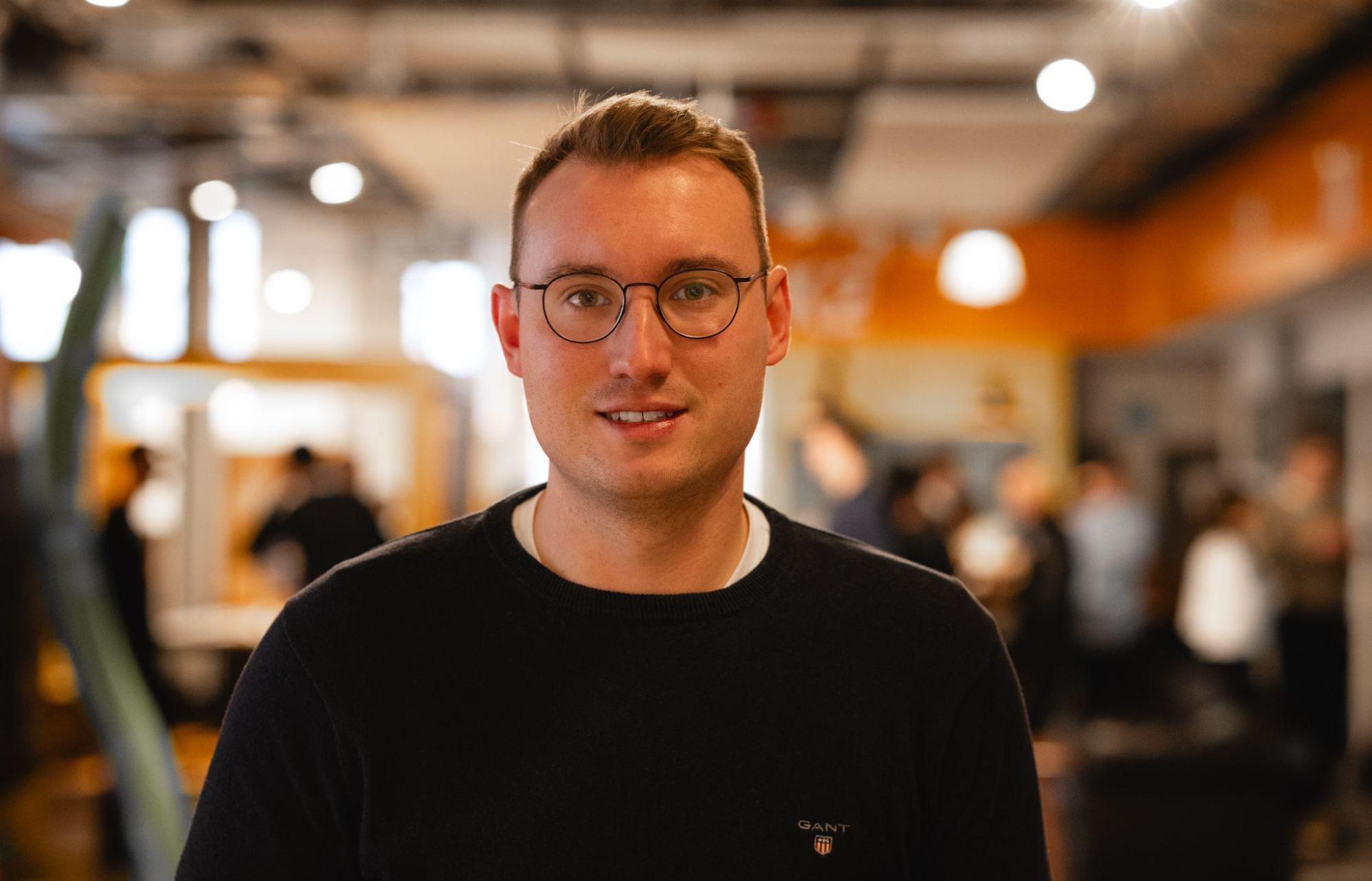
Ryan is the CEO and Co-Founder of SentientSports, an AI startup dedicated to helping sports brands unlock the full potential of AI. The company specializes in providing personalized content and real-time analytics for innovative fan experiences. Holding a PhD from the University of Southampton, his research focused on AI applications in team sports, a domain in which he has published numerous papers. At SentientSports, Ryan has collaborated with many leading sports brands, including leading clubs in the Premier League and NFL. He has been recognized with a Royal Academy of Engineering 1851 Enterprise Fellowship, and his work has been featured in media outlets such as The Athletic and The Times.
Dr. Tim Matthews
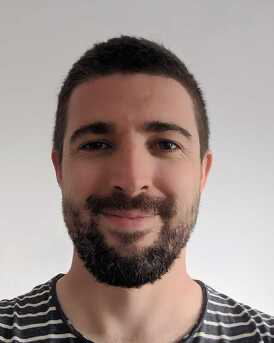
Tim is CTO and co-founder of SentientSports as well as holding a PhD in Computer Vision and Artificial Intelligence from the University of Southampton. His research includes creating SquadGuru, an AI system that surpasses 99% of human players in fantasy sports. At SentientSports, Dr. Matthews leads initiatives in developing AI tools for performance analysis and fan engagement, collaborating with various European football teams, including major English Premier League clubs. His work focuses on merging advanced AI techniques with sports analytics to enhance team performance and decision-making.
Prof. Sarvapali Ramchurn
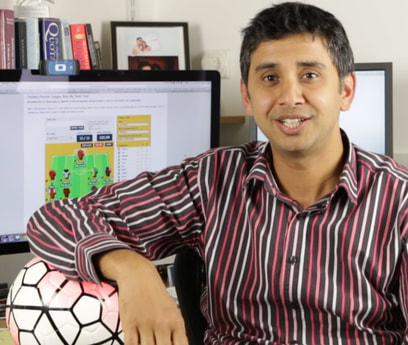
Sarvapali is a leading expert in Artificial Intelligence at the University of Southampton, with a specific focus on trustworthy and responsible AI. He is a co-founder and the Chairman of SentientSports, and also serves as the CEO of Responsible AI UK, the UK government's flagship AI program. Additionally, Professor Ramchurn directs the UKRI Trustworthy Autonomous Systems Hub and has been recognized as a Turing Fellow. His significant academic contributions are evident in his over 7000 citations, and his work has gained widespread recognition, featuring in prominent media outlets like BBC News, New Scientist, Sky News, BBC Click, and Wired.
Prof. Timothy Norman
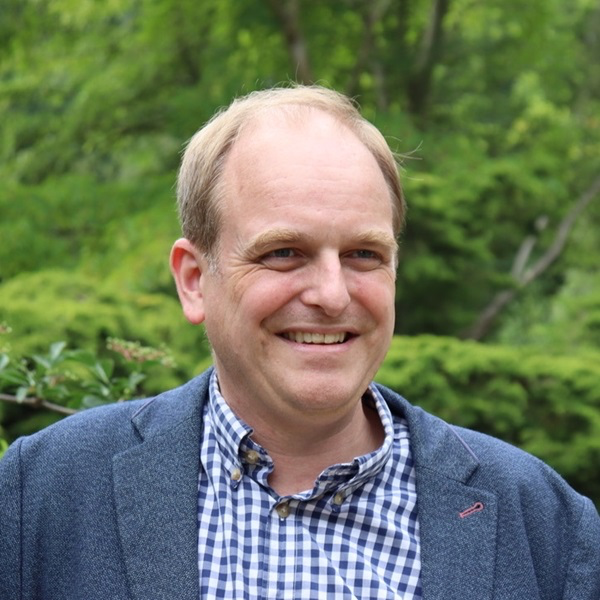
Professor Timothy Norman is the Head of Electronics and Computer Science at the University of Southampton and serves as the director of the UKRI Minds CDT. His extensive academic career is marked by a strong focus on broader AI topics, including multi-agent systems and AI planning and scheduling. He has been involved in sports research for the past 6 years with a number of his students.
2024 Poster Presenters
How to predict the performance of nba draft prospects.
Short Abstract: We introduce a new mathematical system for predicting outcomes of NBA draft prospects based on the outcomes of other previously drafted players. This approach, which is completely general and applicable to any sport, forms predictions as relevance-weighted averages of prior outcomes using a precise and theoretically justified assessment of relevance derived from principles of information theory. Crucially, a measure called “fit” indicates in advance the unique reliability of each individual prediction and dynamically focuses each prediction on the combinations of predictive variables and previous players that are most informative for the prediction task. Relevance-based prediction addresses complexities that are beyond the capacity of conventional prediction models, but in a way that is more transparent, more flexible, and more theoretically justified than widely used machine learning algorithms.
Author(s): David Turkington

David Turkington is a Founding Partner of Cambridge Sports Analytics. He is also Senior Managing Director and Head of State Street Associates, State Street Corporation’s Cambridge-based innovation hub. Dave is the author of more than 40 peer-reviewed scholarly articles and co-author of three books: Prediction Revisited, Asset Allocation: From Theory to Practice and Beyond, and A Practitioner’s Guide to Asset Allocation. His scholarly research has garnered numerous awards, including the prestigious Harry M. Markowitz Award for his research in relevance-based prediction. Dave graduated summa cum laude from Tufts University with a Bachelor of Arts degree in Mathematics and Quantitative Economics.
Megan Czasonis
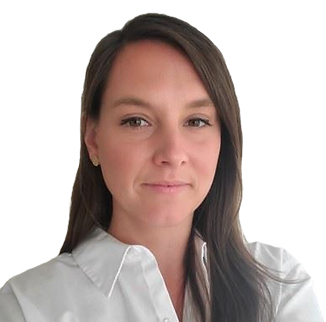
Megan Czasonis is a Founding Partner of Cambridge Sports Analytics. She is also Managing Director and Head of Portfolio Management Research at State Street Associates, State Street’s renowned innovation hub located at Harvard Square. Megan has published extensively in peer-reviewed journals and is a co-recipient of the prestigious Harry M. Markowitz Award for her research in relevance-based prediction. Megan is a coauthor of the acclaimed book, Prediction Revisited, which introduces an alternative mathematical system for forming predictions from data. She holds a Bachelor of Science degree in Economics and Finance from Bentley University.
Mark Kritzman
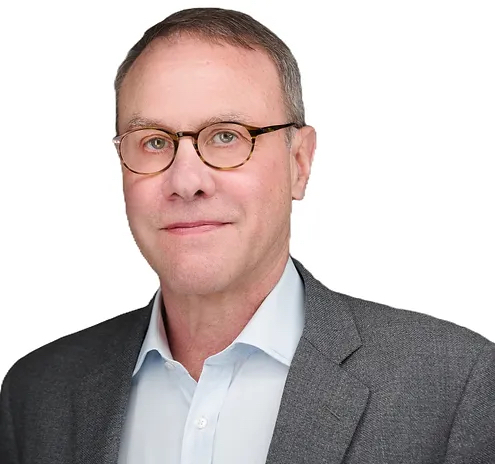
Mark Kritzman is a Founding Partner of Cambridge Sports Analytics. He is also a Founding Partner of Windham Capital Management and State Street Associates, and he teaches a graduate finance course at MIT Sloan. He has served on several boards including the Government Investment Corporation of Singapore, the Institute for Quantitative Research in Finance, the International Securities Exchange, The Investment Fund for Foundations, the MIT Sloan Finance Group, Protego Trust Corporation, and St. John’s University. He has published more than 100 articles in peer-reviewed journals and is the author or co-author of eight books including Prediction Revisited, Asset Allocation: From Theory to Practice and Beyond, The Portable Financial Analyst, and Puzzles of Finance. Mark has won numerous awards for his scholarly research including the Harry M. Markowitz Award for his research in relevance-based prediction. In 2004, Mark was elected a Batten Fellow at the Darden Graduate School of Business Administration, University of Virginia. Mark holds a Bachelor of Science degree in Economics from St. John’s University and a Master of Business Administration degree with distinction from New York University.
Cel Kulasekaran

Cel Kulasekaran is a Founding Partner of Cambridge Sports Analytics. He is also Managing Partner at Windham Capital Management, where he leads applied research for Windham’s asset management business and its strategic partnerships. Cel is the founding architect of Windham’s innovation arm, Windham Labs, which delivers portfolio optimization and risk management technology to investors worldwide. His research has appeared in peer-reviewed academic journals. Cel holds a Bachelor of Science degree in Mathematical Sciences with distinction from Worcester Polytechnic Institute and a Master of Arts degree in Mathematical Finance from Boston University.
Noisy Judgments: A probability surface-based analysis of umpiring variability
Author(s): Emily-Anne Patt

Emily-Anne Patt is the manager for quantitative intelligence and methodologies supporting security and resilience at Alphabet, Inc. based in Washington, D.C. Her background is in econometrics and financial economics, with prior experience in several US government agencies. Fenway Park will always be home, but these days you can find her at Nationals Park or coaching the scholar athletes at the Washington Nationals Youth Baseball Academy.
James Stockton
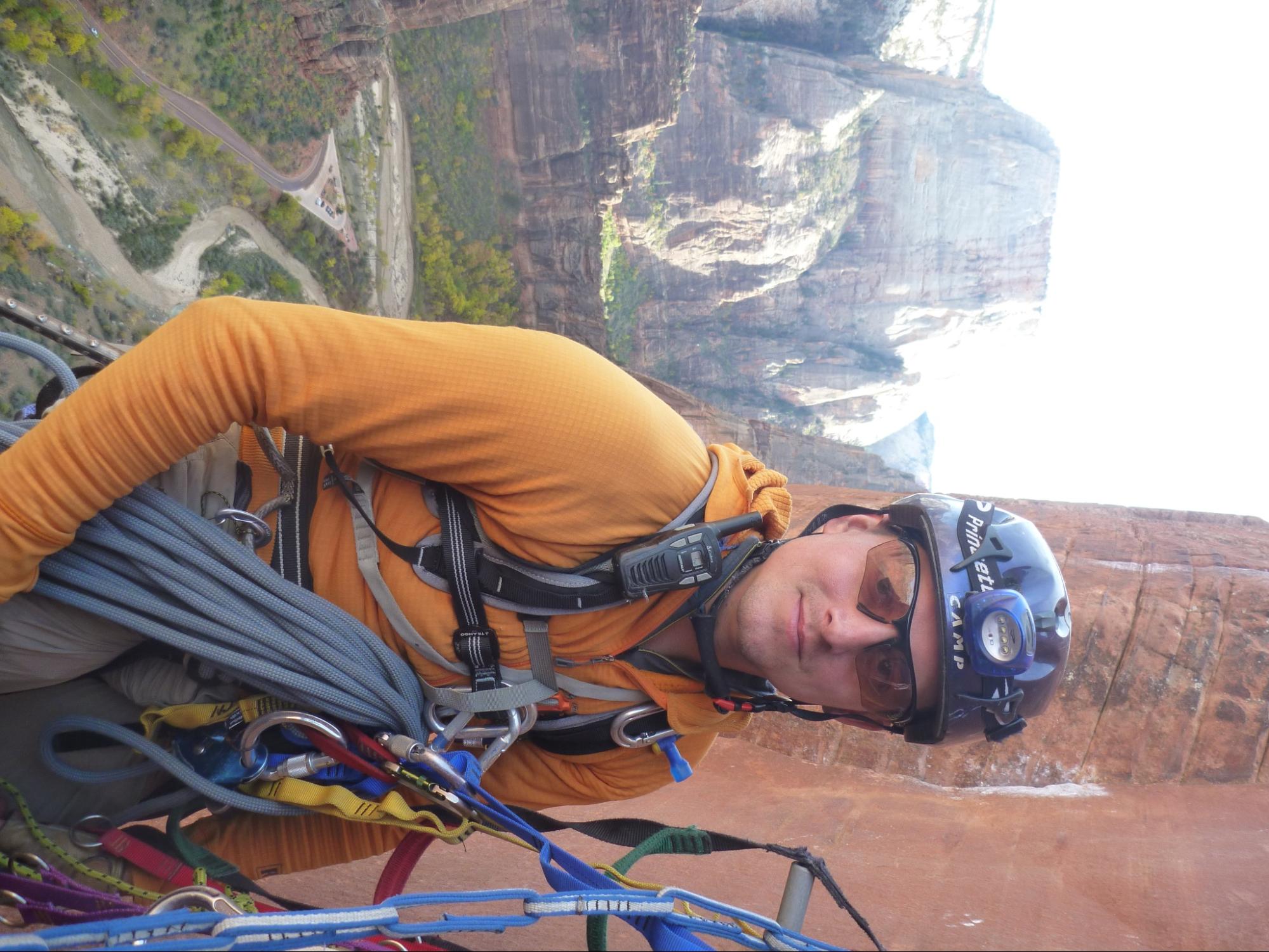
James Stockton, PhD. is the lead data scientist at Altamira Technologies supporting the United States Air Force Chief Data and AI Office based in northern Virginia. His background is in astronomy and astrophysics and he has worked as a data scientist in private industry, academia, and supported federal customers in the DoD/IC space for over a decade. He's an avid climber and mountaineer and will happily talk about mechanical advantage haul systems longer than any sane person should.
Player Pressure Map - A Novel Representation of Pressure in Soccer for Evaluating Player Performance in Different Game Contexts
Short Abstract: In Noisy Judgments: A probability surface-based analysis of umpiring variability, we establish the size, shape, and position of the strike zone as called during an MLB game by building a prior probability surface from 5.3 million called balls and strikes between the 2008 and 2022 seasons. We used this surface to evaluate changes in the actual strike zone over time, stress-testing the reliability of the model with established baseball facts related to batter and pitcher handedness and seasonal shifts. A sensitivity study shows the validity of the surface at lower pitch counts, allowing us to evaluate individual player and umpire performance across games and within a game. This analysis leads us to propose a novel methodology for evaluating a catcher's framing ability--the Framing Induced Strike Zone (FISZ).
Author(s): Chaoyi Gu
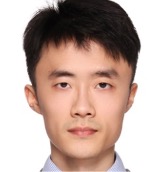
Aaron (Chaoyi Gu) received the MS degree (First class) in sports analytics and technologies from the Institute for Sport Business, Loughborough University, London, United Kingdom in 2020. Chaoyi is currently a Ph.D. from the Institute for Digital Technologies, Loughborough University, London. His research interests include machine learning, multi-agent systems, and sports analytics.
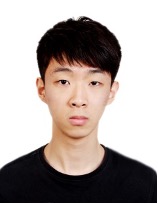
Jiaming (Jamie) Na is a PhD student at Loughborough University's Institute for Digital Technologies. With a background in statistics and a long-time passion for sports, his research centers on advancing sports analytics through computer vision AI models. His work aims to revolutionize performance analysis and strategic decision-making in athletics.
Yisheng Pei
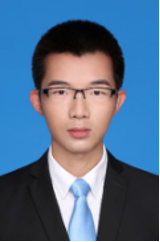
Yisheng Pei is a third-year PhD student at Loughborough University London and a loyal Arsenal fan. His research interest lies in machine learning and deep learning applications in sports analytics, particularly soccer. He is always looking forward to understanding and quantifying players’ efforts on the pitch.n should.
Varuna De Silva

Varuna De Silva is a Reader (Associate Professor) in Machine Intelligence at Loughborough University. He directs the machine intelligence lab at Loughborough University London, where his team works on multi agent reinforcement learning, multimodal machine learning and their applications in Sports Analytics and Autonomous Systems.
A model-based risk-impact analysis of dribble actions in women's soccer
Short Abstract: Our paper presents a model-based approach to quantify individual dribbles in women's soccer based on value and risk attributes. By analyzing over 48,000 dribbles in the 2023 Women's World Cup using machine learning techniques, we measure the expected probability of success and the expected threat of each dribble. The results highlight players who outperform expectations, but can also be used to analyze the playing philosophy of different teams. The findings have implications for player recruitment and development as well as team tactics in women's football. The source code can be found on https://github.com/stefanthiem/xT_Dribbles_Pressure .
Author(s): Tobias Beckman

After completing his master's degree in mathematics, Tobias joined the European consultancy d-fine in 2019. Having played soccer enthusiastically from an early age, he quickly joined the company's sports analytics team. There, he now uses his knowledge to quantify the youth development work of soccer clubs, develop methods for match analysis and develop customized scouting platforms using modern open-source tools.
Gerhard Waldhart
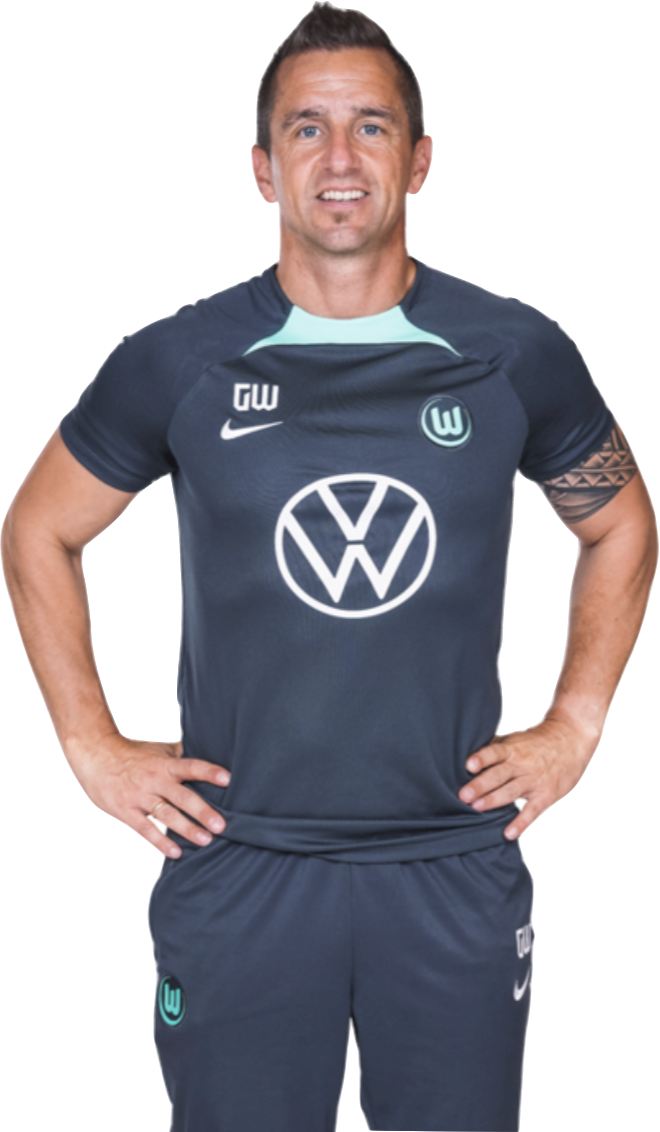
Gerhard is a sports scientist and soccer analyst, currently serving as the Head of Match Analysis for VfL Wolfsburg Women, champion of the German Bundesliga in 2022 and UWCL Women’s Champions League Finalist 2023. Gerhard has been a licensed coach for 17 years and is currently pursuing a master's degree in match analysis at the University of Cologne – always looking for an added edge through data and analytics.
Stefan Thiem
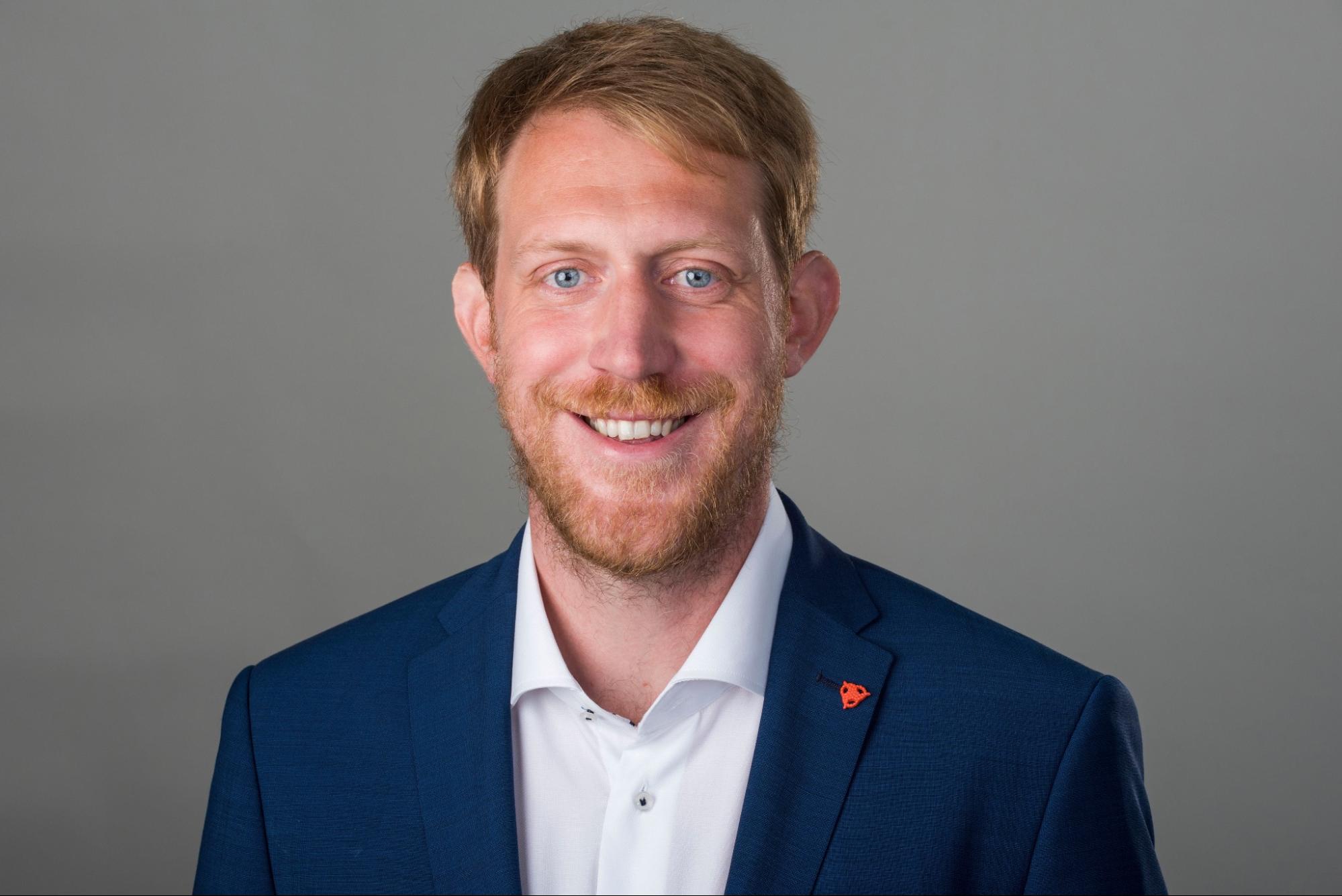
Stefan is a Senior Consultant at the European consultancy d-fine, where he works as a data scientist. In his projects, he supports sports clubs gaining insights from data for match analysis and talent evaluation. He graduated from the University of Münster with a PhD in sports economics and with a bachelor’s degree in mathematics. In addition, he has been holding a soccer coaching license from the UEFA for seven years. Stefan is very passionate about sports in general and he maintains his own sports podcast, where he covers and discusses interesting scientific questions related to sports.
Oliver Wohak
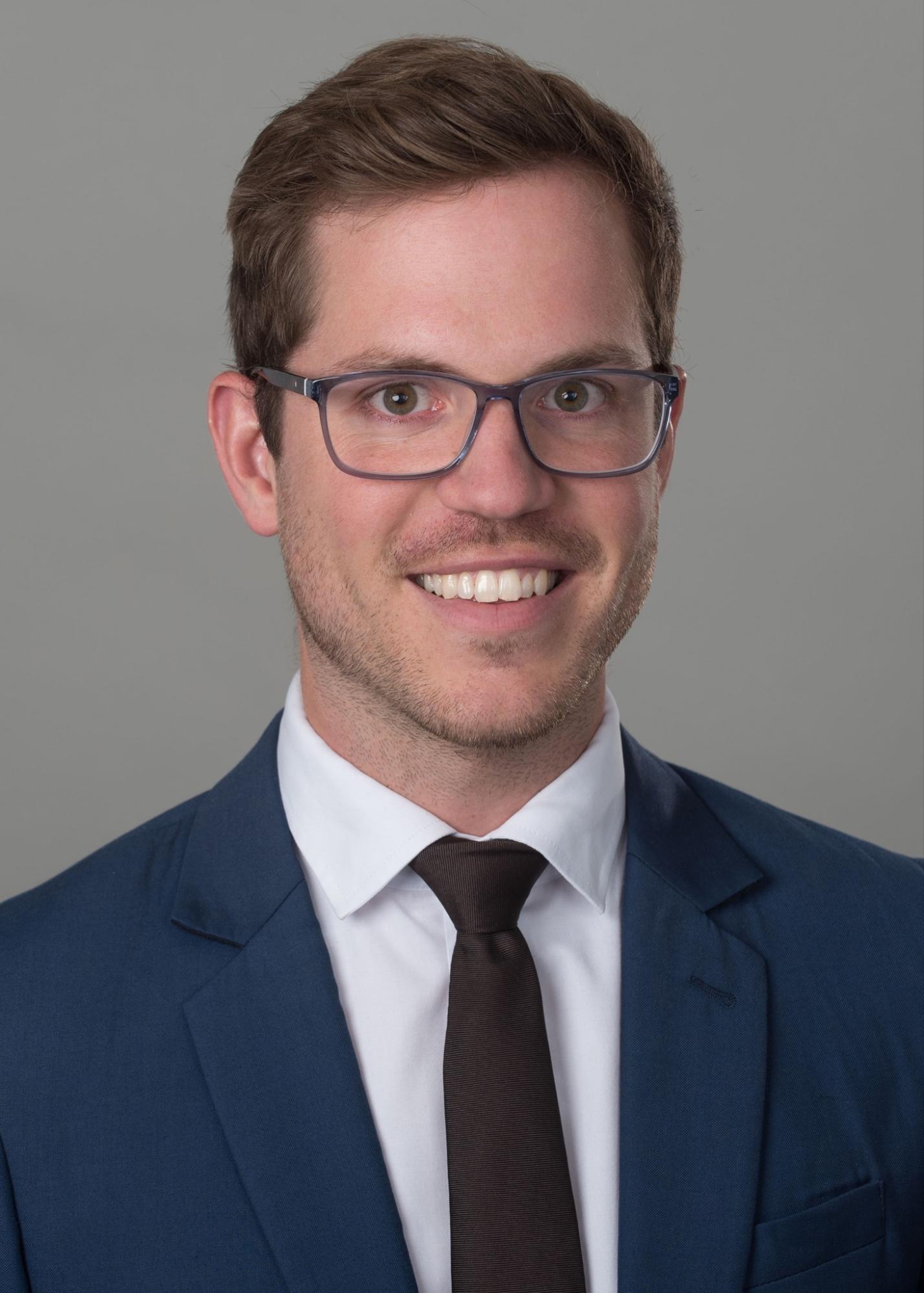
Oliver is a Senior Manager at the European consultancy d-fine, where he supports his clients in tackling the most complex challenges in digital transformation. Driven by his passion for sports and complemented by his master’s degrees in physics and Business Administration, Oliver built up the Sports Analytics team within d-fine and has been supporting clubs and associations in developing internal data analytics and IT solutions for over 5 years. While his main focus is on scouting, match analysis and talent development in soccer, his experience also includes ice hockey, basketball and handball. Stemming from his time in the US, Oliver is an avid American Football fan, roots for the Eagles and especially enjoys Fantasy Football season.
Using Tracking Data to Build Offensive Line Development Tools
Short Abstract: American football has in recent years made drastic shifts towards the quantitative. The proliferation of charting and tracking data has given us the ability to better evaluate and value players, but player development has been left wanting. In this paper we use NFL's Next Gen Stats data to build tools for offensive linemen in pass protection, which will help teams more efficiently watch film, monitor performance and fitness, build rosters, and game plan, with player development as the central focus.
Author(s): Eric Eager

Eric Eager is the VP of Research and Development at SumerSports. He holds a doctorate in mathematical biology from the University of Nebraska - Lincoln, and has published over 30 papers, including three at the MIT Sloan Sports Analytics Conference. He is the co-author of the book: Football Analytics with Python and R: Learning Data Science Through the Lens of Sports.
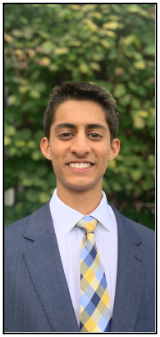
Tej Seth is a data scientist at SumerSports with a focus on football analytics. He graduated from the University of Michigan, where he worked with the football team. He is an avid Lions fan and outside of football loves listening to podcasts and going to the gym.
Ben Brown
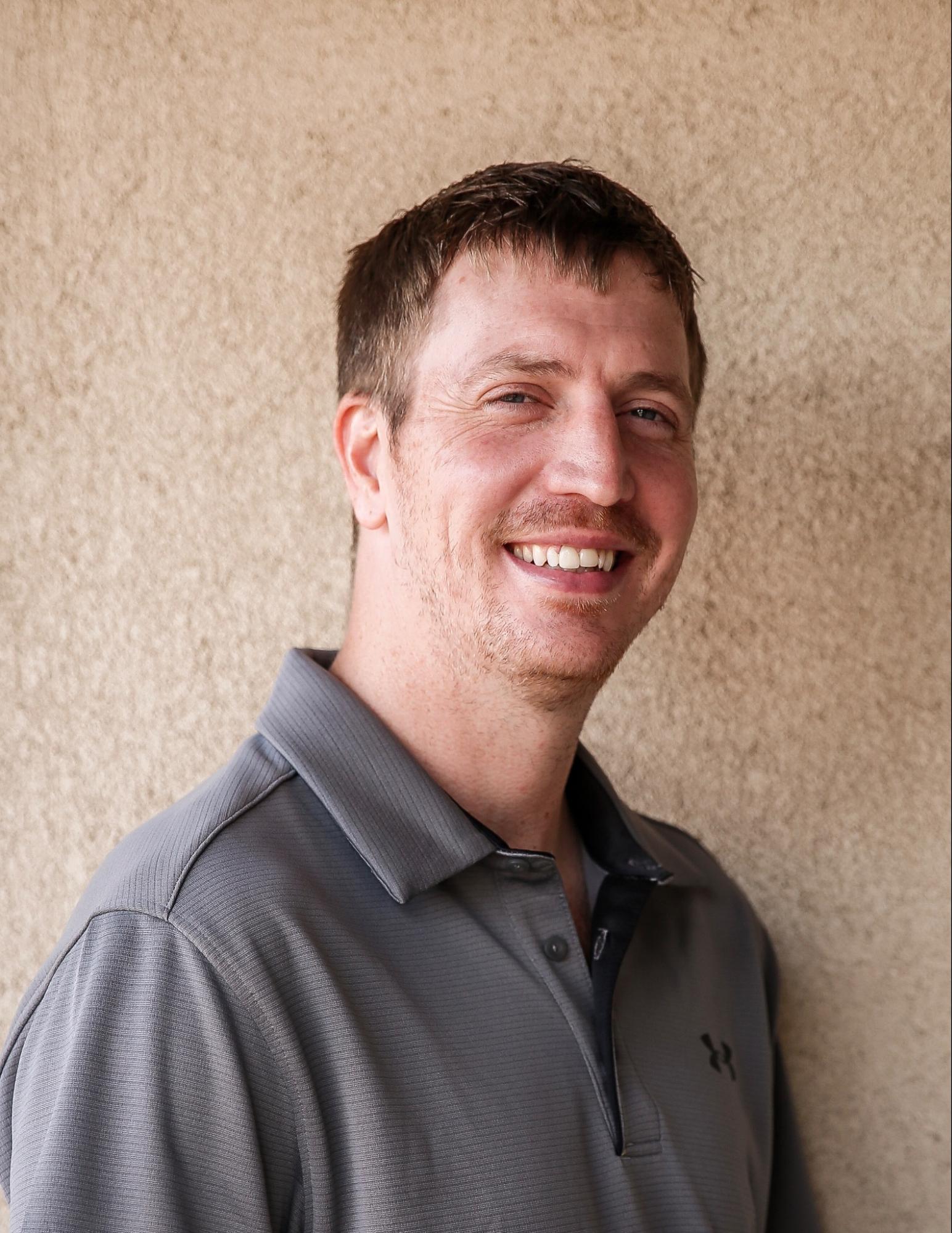
Ben Brown is a data scientist at SumerSports. Prior to joining Sumer, he was the Head of Betting Innovation at PFF, where he won numerous DFS tournaments at both the NFL and NCAA level and hosted the PFF Daily Betting Podcast and various live streams.
Haley English
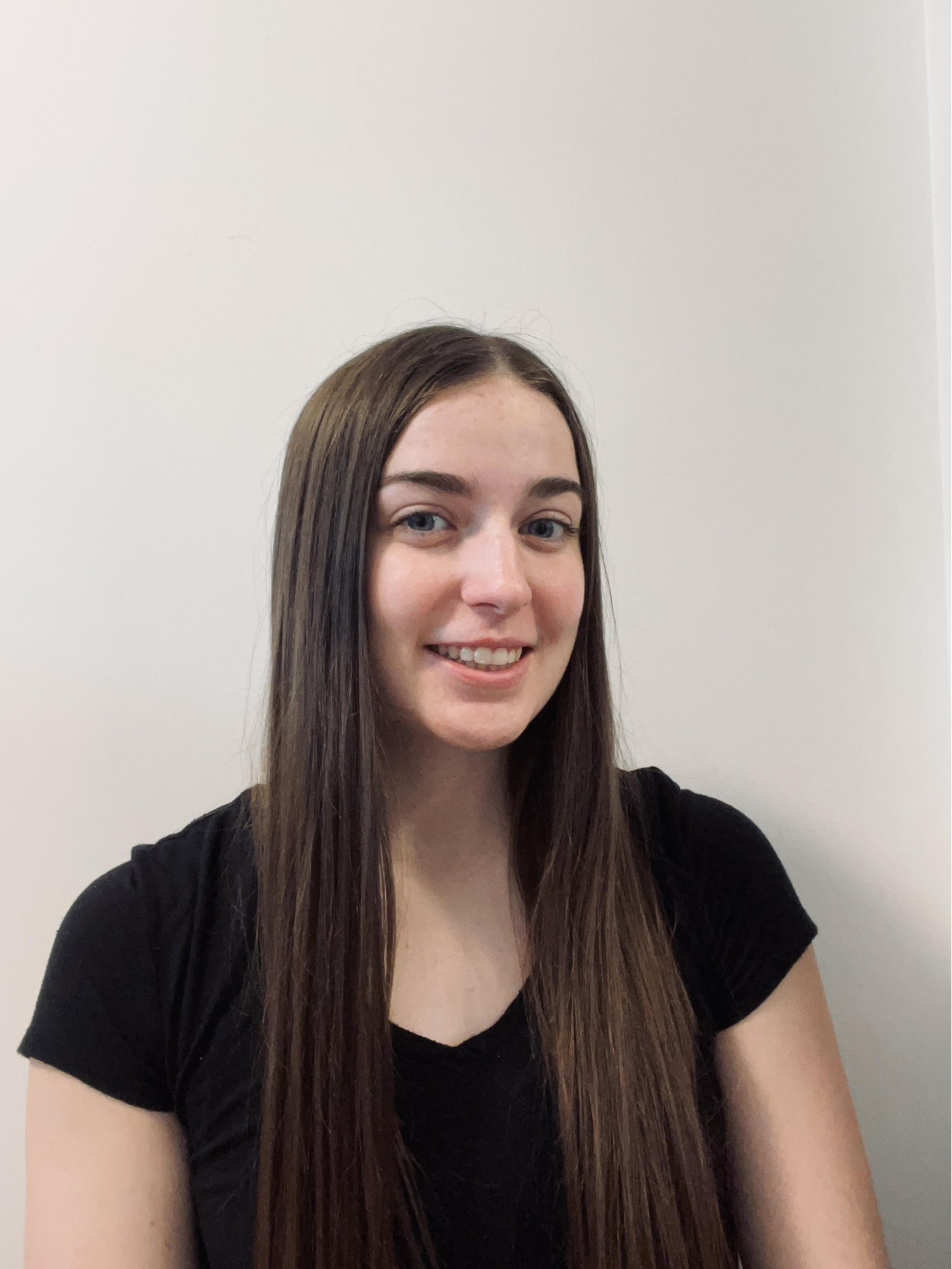
Haley English is currently a Football Information Intern with the Detroit Lions. She recently graduated from Villanova University and previously interned at Pro Football Focus. Outside of football analytics, she enjoys taking trips to the lake and watching her former sport, gymnastics.
Geoff Schwartz
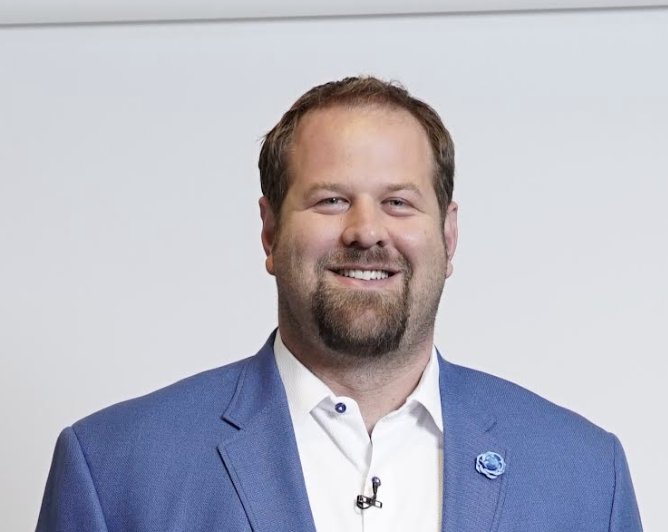
From his pre-Bar Mitzvah stuttering days to being drafted 241st (out of 252) in the 2008 NFL draft, Geoff Schwartz has overcome adversity to exceed all expectations and impressively succeed in life. His eight-year career included stints with the Carolina Panthers, Minnesota Vikings, Kansas City Chiefs and New York Giants, where he signed a 4-year deal to become a starting guard on the Eli Manning-led team. Unfortunately, injuries derailed Geoff’s career and caused his retirement from the NFL in February 2017. Before his retirement Geoff began a seamless transition to a career in media. He can be heard daily on Sirius XM Radio 373 hosting PAC-12 Today and on the weekend hosting Fox Sports Radio. Geoff is a Fox Sports NFL gambling analyst, providing digital and written content. He hosts his own podcast, Geoff Schwartz Is Smarter Than You, where Geoff makes the average football fan smarter. He appears routinely on sports radio talk shows and podcasts throughout the country offering his insights on football, other sports plus a wide variety of non-sports related topics.
How Much Do Faceoffs Matter? Translating Faceoffs to Goals, Wins, and Championships in Hockey
Short Abstract: In hockey, faceoffs have long been acknowledged as important drivers of puck possession, but their actual impact on scoring outcomes remains inadequately measured. It is acceptedly evident that a center winning 54% of their faceoffs outperforms one with a 51% success rate, but the tangible extent of this advantage in terms of goals, wins, and losses remains underexplored. This research fills the void by continuing the effort to translate faceoff results to scoring outcomes, measuring faceoff performance in goals, wins, and losses in a novel manner. We explore evidence that faceoffs are an undervalued championship-caliber market inefficiency and offer models enabling General Managers to see role-specific projections of how different personnel and usage could maximize offense, defense, and championship chances.
Author(s): Tad Berkery
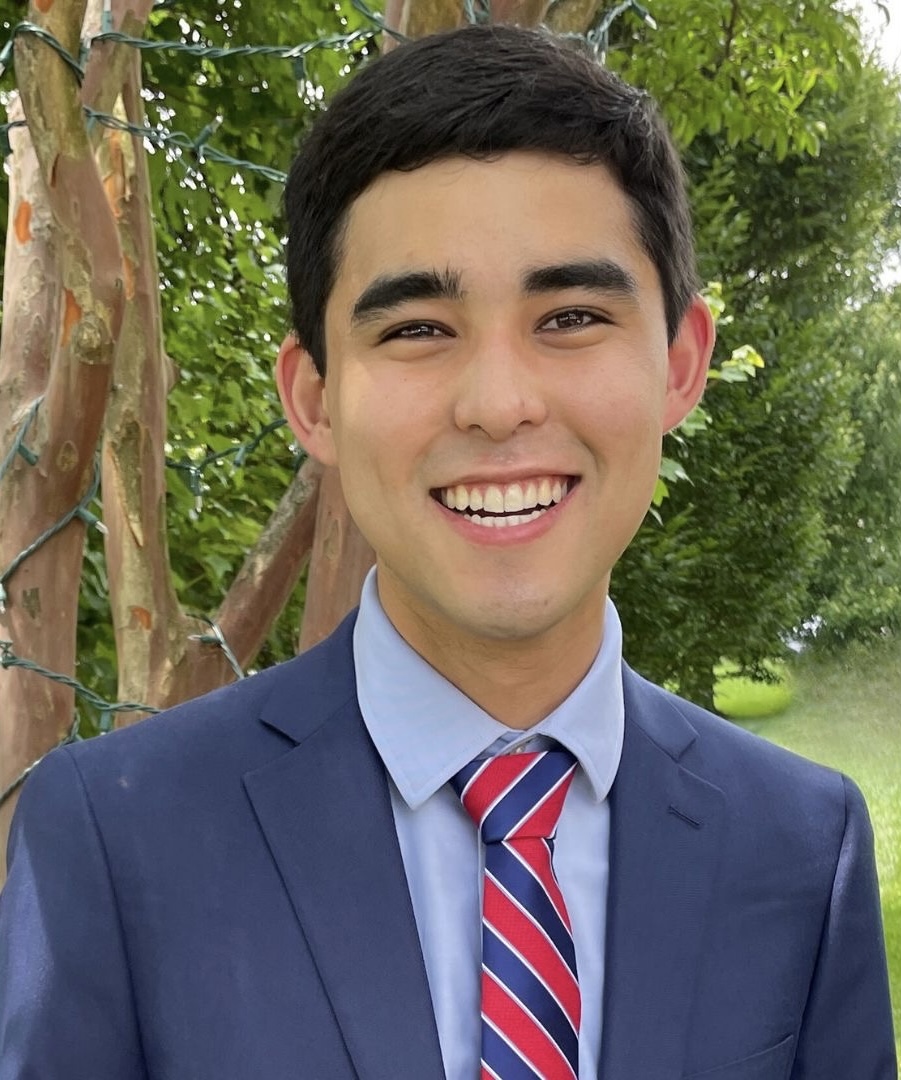
Tad is a senior at Johns Hopkins University majoring in Computer Science and Economics and minoring in Computational Medicine and Applied Mathematics & Statistics. He has completed research or worked directly for teams spanning the Big Ten, MLB, NHL, and NFL. Tad has also been featured in The Washington Post and is the author of What’s the “Right” Career? . In his free time, you can find him hanging out with family and friends, playing games, or trying his hand at becoming an at-home barista.
Chase Seibold

Chase is a recent graduate of Syracuse University, holding Bachelor's degrees in Economics and Sport Analytics, along with a Master's degree in Applied Data Science. During his time at Syracuse, he interned with Wasserman in the baseball department and contributed to the Washington Nationals R&D group as an intern. He is currently an R&D Analyst for the Nationals.
Max Stevens
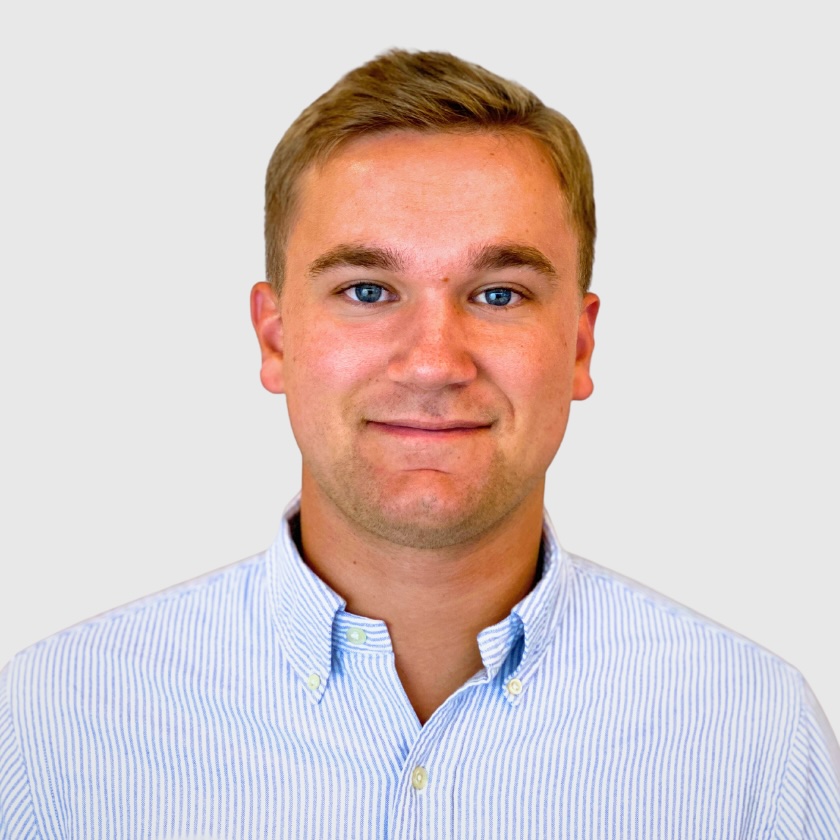
My name is Max Stevens, and I am currently a student at Johns Hopkins University, set to graduate in May 2024 with degrees in Applied Mathematics & Statistics and Economics. Professionally, I have gained valuable experience as a Data Analyst intern at Attain Sports, a sports holding company with interests in baseball and soccer. My role involved predictive modeling for game scenarios and applying my analytical skills to enhance the business aspects of their sports portfolio. Previously, I was a member of the Johns Hopkins football team. I am a dedicated fan of Boston sports having grown up in Lexington, Massachusetts. Most recently, I have discovered a passion for European football and have pledged myself to the Tottenham Hotspur football club, COYS.
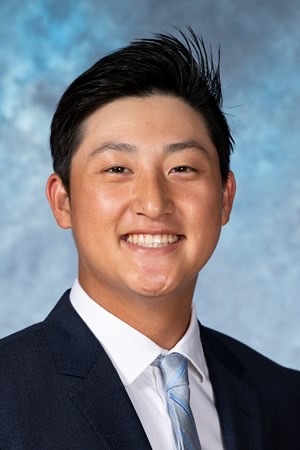
Justin is a recent graduate from Johns Hopkins who was a part of the Johns Hopkins University Sports Analytics Research Group for three years. During his time working under Dr. Anton Dahbura, he worked on projects in baseball, football, and hockey, including projects for the Baltimore Orioles and Ravens. Since graduating in May with degrees in Computer Science and Applied Mathematics & Statistics, he has been working on the AWS Cloudfront Team as a Software Developer Engineer.
Anton Dahbura
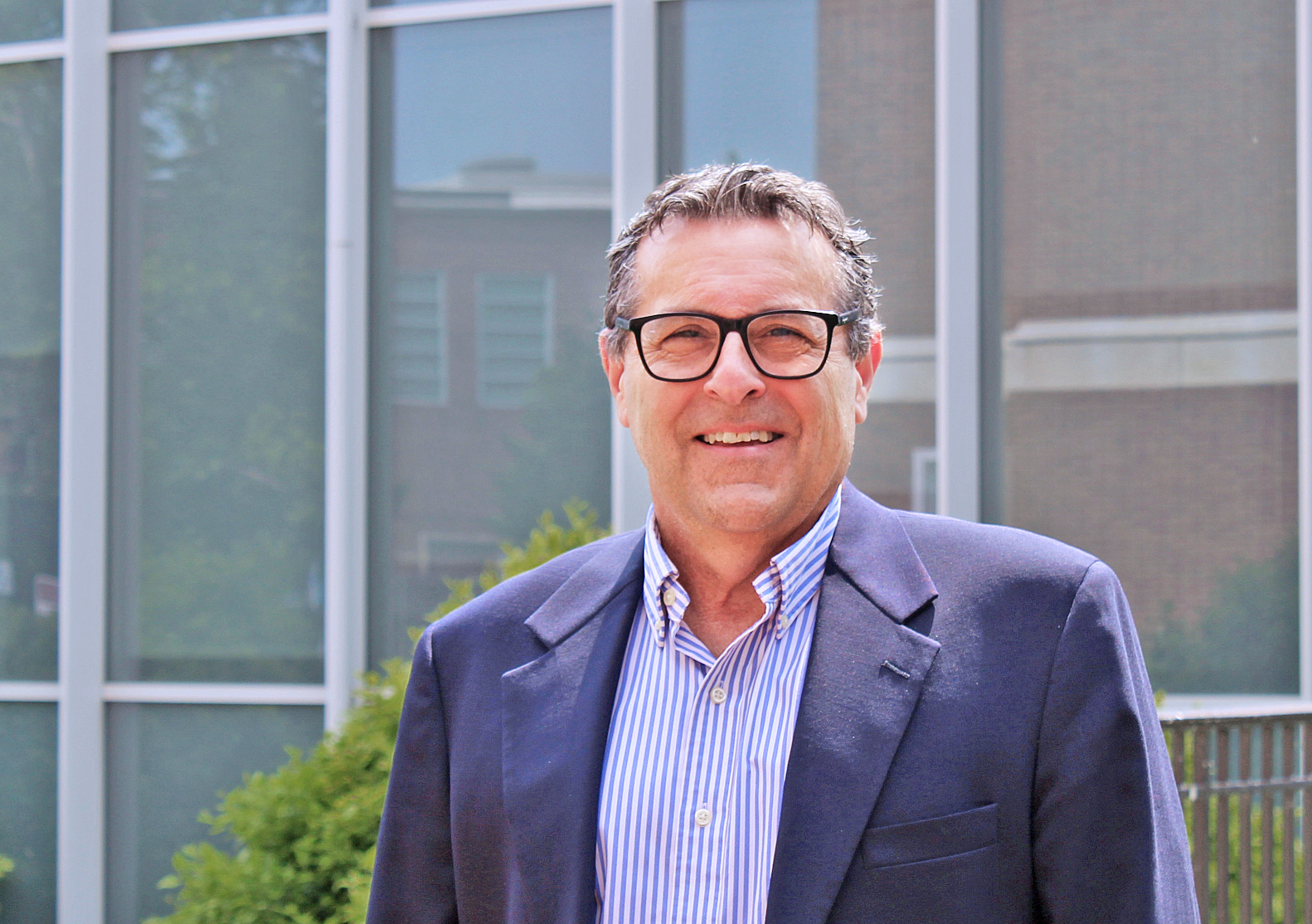
Anton (Tony) Dahbura received the BSEE, MSEE, and PhD in Electrical Engineering and Computer Science from the Johns Hopkins University in 1981, 1982, and 1984, respectively. He served as a researcher at AT&T Bell Laboratories, was an Invited Lecturer in the Department of Computer Science at Princeton University, and served as Research Director of the Motorola Cambridge Research Center in Cambridge, Massachusetts. In January, 2012 he was named Executive Director of the Johns Hopkins University Information Security Institute in Baltimore and joined the faculty of the Johns Hopkins University Department of Computer Science as an Associate Research Scientist. In September 2018 he was named Co-Director of the Johns Hopkins University Institute for Assured Autonomy. Tony has had a love for baseball since childhood. He was named to the National Under-18 Baseball Team of El Salvador, where he lived for most of his early years, and was an outfielder for Johns Hopkins as an undergraduate. In 2010 he became co-owner of the Hagerstown Suns, the low-A affiliate of the Washington Nationals.
Analyzing NBA Player Positions and Interactions with Density-Functional Fluctuation Theory
Short Abstract: Player tracking data can enhance the quantification of player abilities and our understanding of team composition and broader team strategies. In this work, we demonstrate how density-functional fluctuation theory (DFFT), an extension of a Nobel Prize-winning physics approach, can process basketball tracking data by treating players as interacting densities. By training the interactions on different play outcomes, we can evaluate play-outcome likelihoods based on player positions, determine which players are in strong or weak positions, and understand which players consistently instigate strong responses from the opposing team (i.e., ‘player gravity’). We find that our approach not only identifies the overall strengths of a player, but also identifies subtleties such as those who are left-handed (e.g., D. Russell) or who instigate changes non-locally through frequent passes (e.g., N. Jokic).
Author(s): Boris Barron
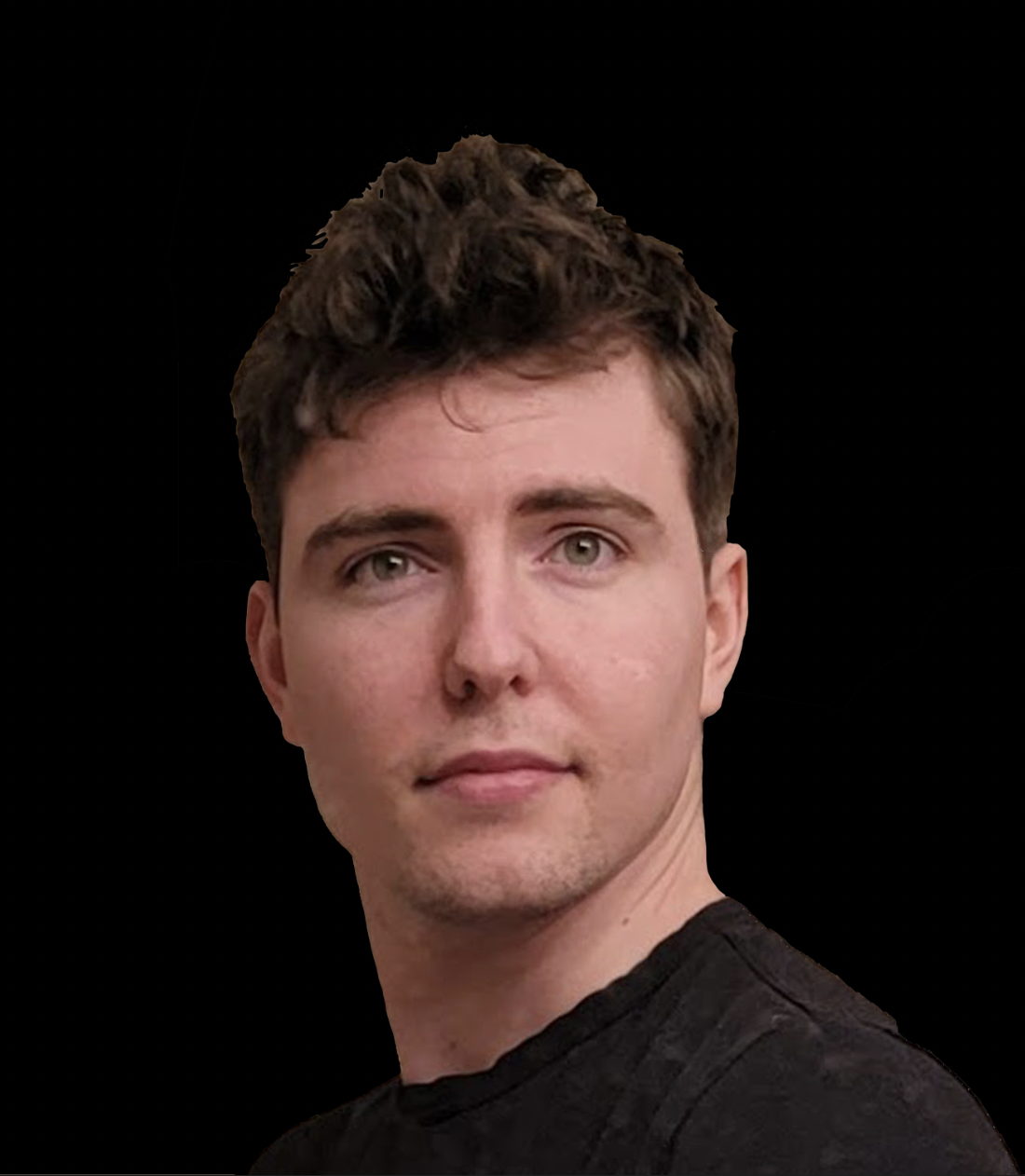
Boris Barron is a PhD candidate in Theoretical Physics at Cornell University, where he is affiliated with the Cornell Population Center. He holds a Master of Science in Mathematical and Theoretical Physics from the University of Oxford and a Bachelor of Science in Biophysics from York University. His research experience has spanned microfluidics, laser stability physics, machine learning, and increasingly complex systems in the broadest sense. Recently, he has been developing a novel framework for understanding residential segregation, with work that has included presentations at the U.S. Census Bureau, American Sociological Association (ASA), American Physical Society (APS), and the Population Association of America (PAA). His work has been funded in part by NSERC PGS-D. Beyond academia, Boris used to be a competitive ballroom dancer and is a speed typist, averaging over 120 words per minute – height limitations have prevented him from being a serious contender in basketball.
Nathan S. Sitaraman
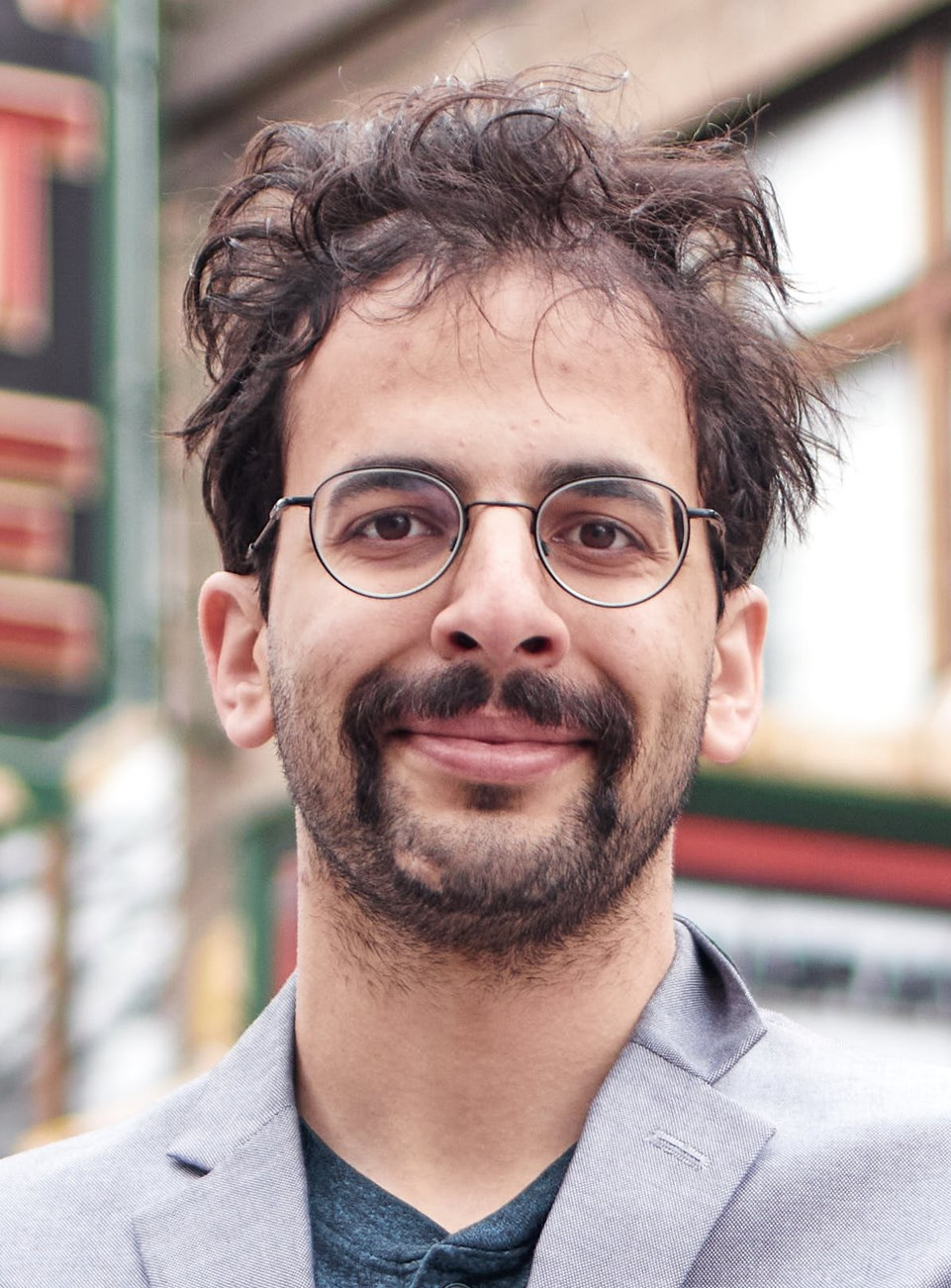
Nathan Sitaraman is a postdoctoral associate in the Cornell Laboratory for Accelerator-based ScienceS and Education (CLASSE) and a part-time consultant for the Dallas Mavericks. He graduated from Yale University cum laude with a bachelor's degree in physics, and completed his PhD in physics at Cornell. His research focuses on improving teamwork among superconducting electrons, as well as the much more complicated problem of improving teamwork among basketball players.
Tomas A. Arias
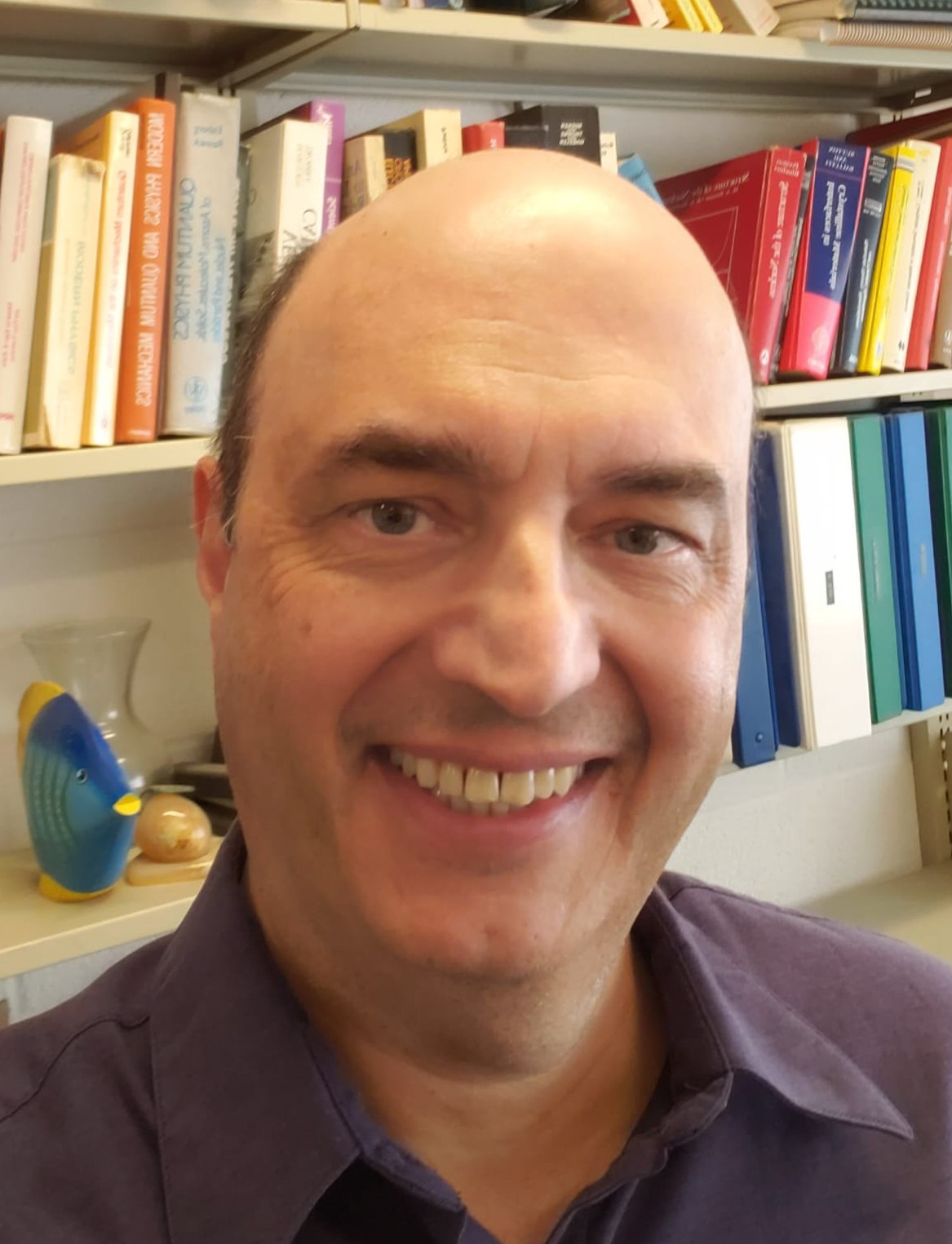
Tomás Arias, a Professor of Physics at Cornell University, holds SB and PhD degrees in Physics from the Massachusetts Institute of Technology, where he also briefly served on the faculty in the late 1990s before joining Cornell. He is an expert in developing novel density-functional theory (DFT) methods, with thirty years of experience and more than 60 publications on this topic alone, contributing to a total of 91 publications with over 25,000 citations. His recent work involves the development of joint density-functional theory (JDFT), a specialized method for analyzing the equilibrium of liquids and solids, and density functional fluctuation theory (DFFT), which extends the theory to understand the collective behaviors of human crowds and animal groups, as well as human residential segregation and group sports.
Previous Research Paper Finalists
Full paper submission form, full paper submissions for ssac24 are due friday, december 1, 2023 at 11:59pm et., open source competition - voting.
Review our SSAC 2022 open source finalists below and cast your vote for your favorite submission HERE !
2022 Open Source Finalists
Alejandro Rodriguez Pascal, Ishan Mehta, Muhammad Khan, Rose Yu, Frank Rodriz
Matthew Wear, Ryan Beal, Tim Matthews, Gopal Ramchurn, Tim Norman
DeMars DeRover
Connor Heaton, Prasenjit Mitra
Subscribe for the latest news and updates
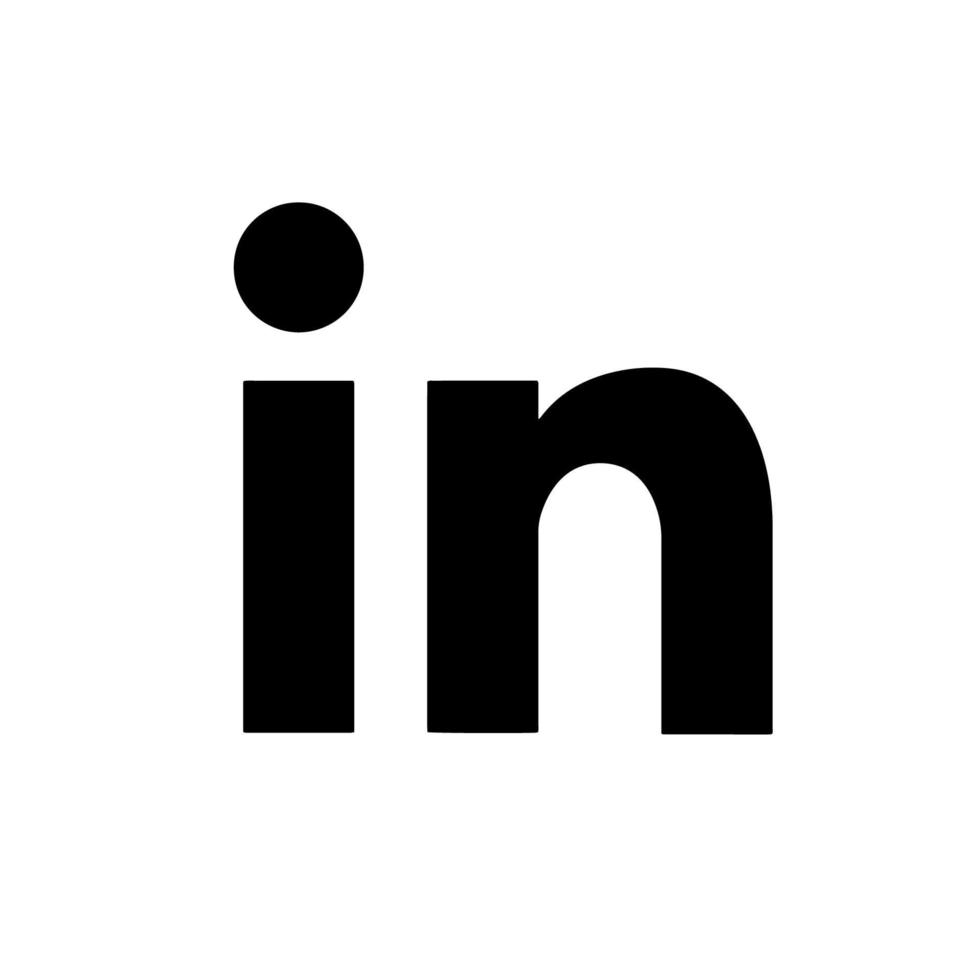
Bringing together the leading figures in sports analytics, business, and technology.
- Japanese (日本)
Our new research: Enhancing blockchain analytics through AI

Elliptic Research
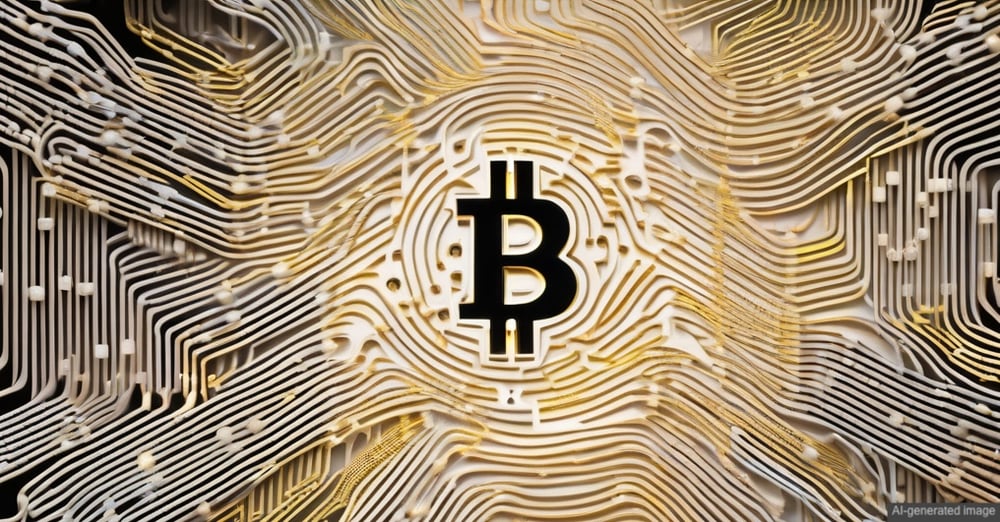
- Elliptic researchers have made advances in the use of AI to detect money laundering in Bitcoin. A new paper describing this work is co-authored with researchers from the MIT-IBM Watson AI Lab.
- A deep learning model is used to successfully identify proceeds of crime deposited at a crypto exchange, new money laundering transaction patterns and previously-unknown illicit wallets. These outputs are already being used to enhance Elliptic’s products.
- Elliptic has also made the underlying data publicly available . Containing over 200 million transactions, it will enable the wider community to develop new AI techniques for the detection of illicit cryptocurrency activity.
At Elliptic we have always pushed the boundaries of blockchain analytics, to enable our customers to more accurately and efficiently assess risk in cryptoassets. Part of this innovation has been exploring how artificial intelligence can be leveraged to improve the detection of money laundering and other financial crime on blockchains.
Blockchains provide fertile ground for machine learning techniques, thanks to the availability of both transaction data and information on the types of entities that are transacting, collected by us and others. This is in contrast to traditional finance where transaction data is typically siloed, making it challenging to apply these techniques.
Machine learning on the blockchain
We first published research on this topic in 2019, co-authored with researchers from the MIT-IBM Watson AI Lab. A machine learning model was trained to identify Bitcoin transactions made by illicit actors, such as ransomware groups or darknet marketplaces. The training data, compiled by Elliptic and containing over 200,000 bitcoin transactions, was made publicly available to encourage further experimentation and collaboration within this emerging field. That paper has now been cited nearly 400 times by researchers around the world, which demonstrates the impact it has had and continues to have in the fields of machine learning and anti-money laundering.
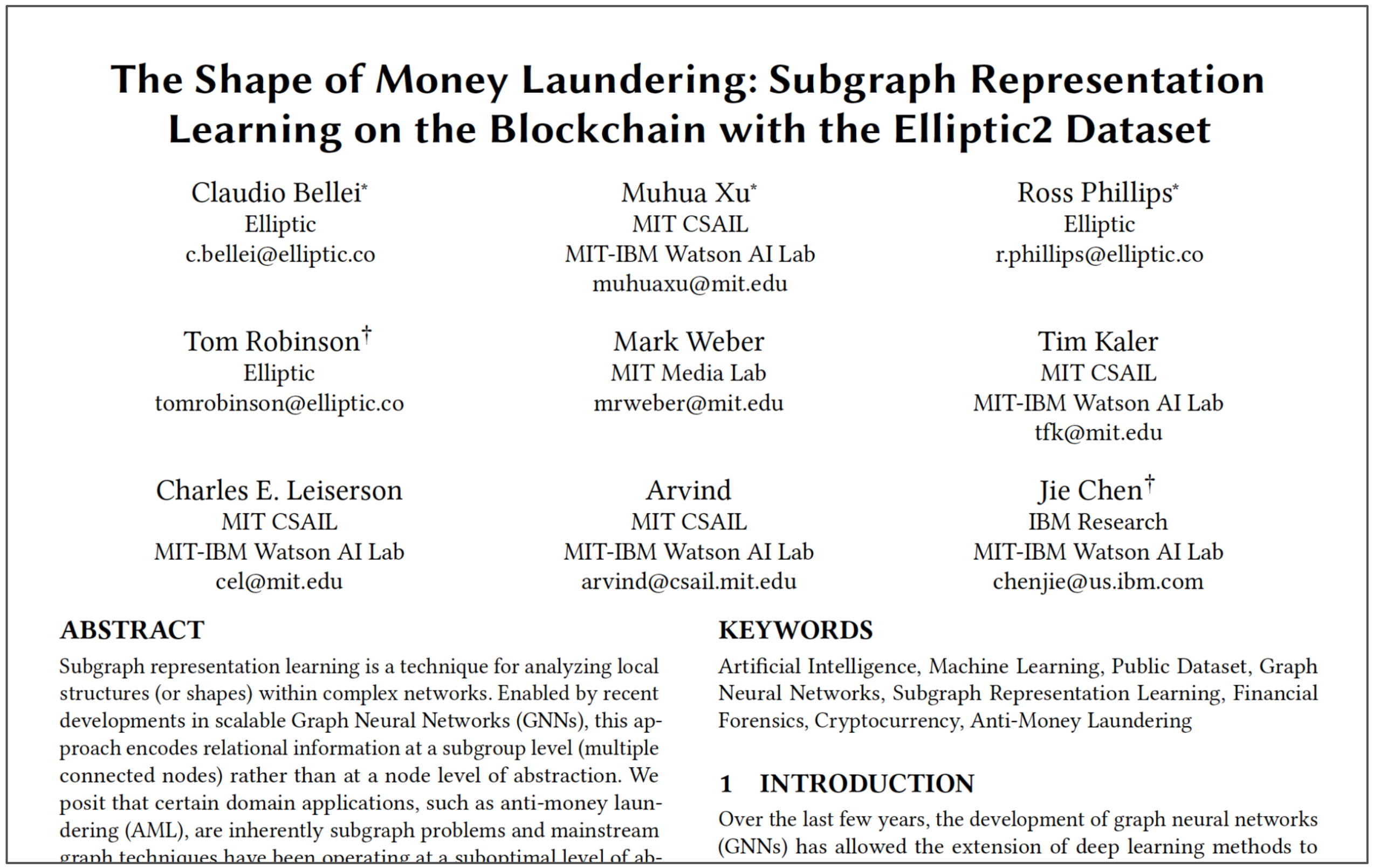
We have now released further research , applying new techniques to a much larger dataset, containing nearly 200 million transactions. This work is again co-authored by researchers from the MIT-IBM Watson AI Lab. Rather than identifying transactions made by illicit actors, a machine learning model is trained to identify “subgraphs”, chains of transactions that represent bitcoin being laundered. By identifying these subgraphs rather than illicit wallets, this approach allows us to focus on the “multi-hop” laundering process more generally rather than the on-chain behavior of specific illicit actors.
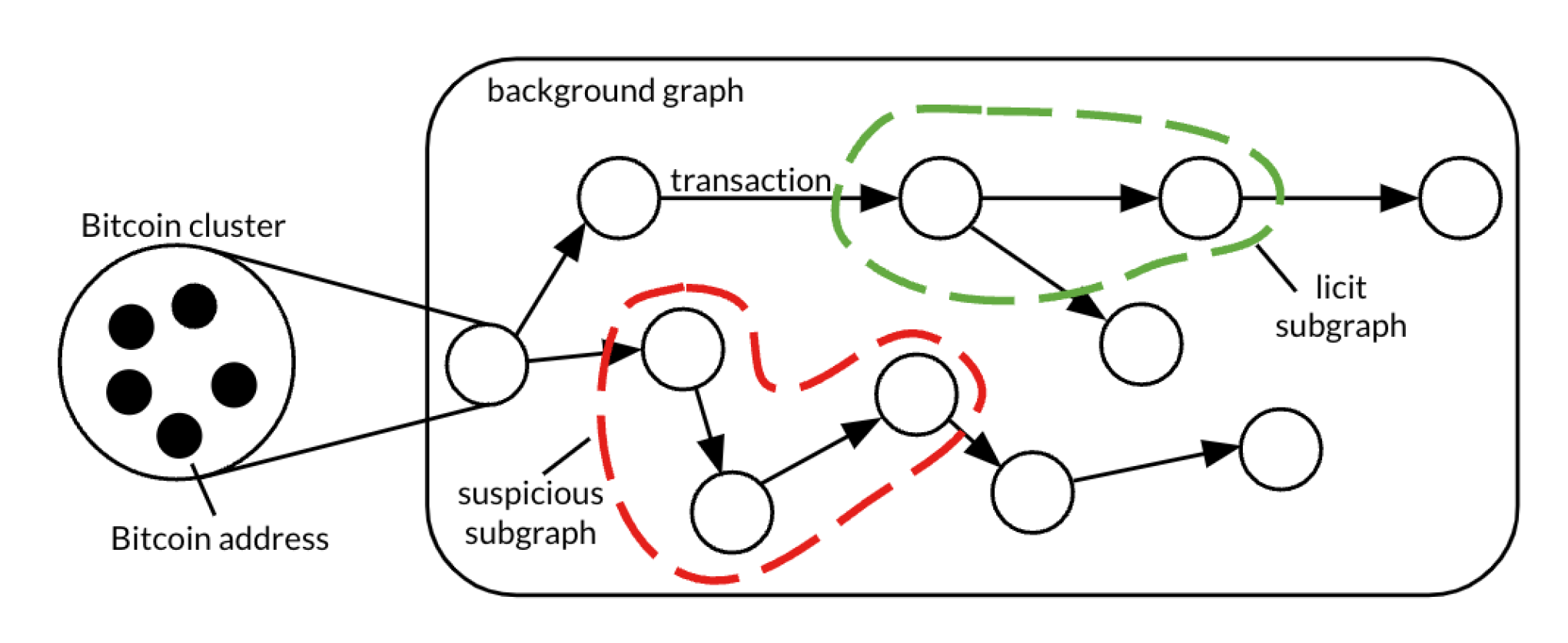
Testing our results
We worked with a cryptocurrency exchange to test whether this technique could be used to identify money laundering attempts through that business. Of 52 “money laundering” subgraphs predicted by the model and which ended with deposits to this exchange, the exchange confirmed that 14 had been received by users who had already been flagged as being linked to money laundering. On average less than one in 10,000 of these accounts are flagged as such, suggesting that the model performs very well * . Importantly, the exchange’s insights were based on off-chain information, suggesting that the model can identify money laundering that would not be identifiable using traditional blockchain analytical techniques alone.
We also investigated the types of money laundering patterns that the trained model was identifying. This revealed known money laundering patterns such as “peeling chains”, which can already be automatically detected in Elliptic’s transaction and wallet screening tools. However it also identified novel patterns such as the use of intermediary “nested services” in specific ways. Knowledge of these money laundering behaviors is of value to AML practitioners, and can be added to the suite of behaviours that can be detected with Elliptic’s tools.
.png?width=2500&height=1307&name=AI_Blog_image1_1200_627%20(1).png)
The machine learning model can also be used to help identify previously-unknown illicit wallets. When the model predicts that a given subgraph is an instance of money laundering, it implies that the funds have potentially originated from some type of illicit activity. Directed research can then be performed on these wallets to try to identify them. This approach has already enabled us to identify a number of previously unknown wallets used by illicit actors including ponzi schemes and darknet markets.
Sharing our data with the community
As well as releasing our research, we have also made the underlying data publicly available . The largest public dataset of its kind, “Elliptic2” will enable the development of new techniques for the detection of illicit cryptocurrency transactions by the wider community. It will also aid the development of the underlying graph neural network methods, used in applications including drug discovery, physics and computer vision.
This novel work demonstrates that AI methods can be applied to blockchain data to identify illicit wallets and money laundering patterns, which were previously hidden from view. This is made possible by the inherent transparency of blockchains and demonstrates that cryptoassets, far from being a haven for criminals, are far more amenable to AI-based financial crime detection than traditional financial assets. We have barely scratched the surface of what is possible in this domain, but this work has already led to benefits for Elliptic’s users. Further collaboration and data-sharing will be key to advancing these techniques further and combating financial crime in cryptoassets.
You can read the research paper in full here , and the Elliptic2 dataset is now available to access. To discuss the research, and find out more about how we are applying these new techniques to enhance our products, get in touch .
Featured Articles
Found this interesting? Share to your network.
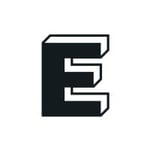
The latest deep-dives and data-driven analysis from our Research team covering their own investigations, as-its-happening monitoring of hacks and thefts, and more.
This blog is provided for general informational purposes only. By using the blog, you agree that the information on this blog does not constitute legal, financial or any other form of professional advice. No relationship is created with you, nor any duty of care assumed to you, when you use this blog. The blog is not a substitute for obtaining any legal, financial or any other form of professional advice from a suitably qualified and licensed advisor. The information on this blog may be changed without notice and is not guaranteed to be complete, accurate, correct or up-to-date.
Get the latest insights in your inbox
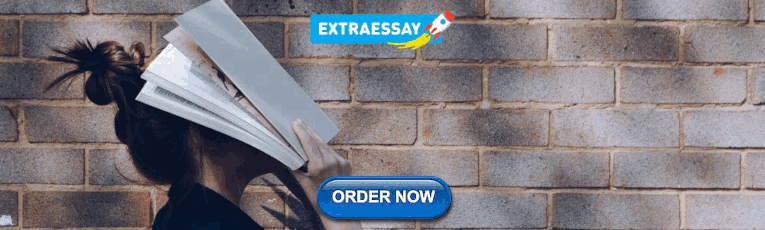
IMAGES
VIDEO
COMMENTS
Sports Analytics is an emerging research area with several applications in a variety of fields. These could be, for example, the prediction of an athlete's or a team's performance, the estimation of an athlete's talent and market value, as well as the prediction of a possible injury. Teams and coaches are increasingly willing to embed such "tools" in their training, in order to improve ...
1. Introduction. Players' performance prediction by using current and past data has gained attention, particularly in basketball [1], [2].Sports analytics and forecasting through these data is a rapid growing field with many methods that can be implemented from a different perspective for each situation [3].In a team, and specifically for the technical staff and coaches, the knowledge of ...
The Journal of Sports Analytics (JSA) is a new high-quality research journal that aims to be the central forum for the discussion of practical applications of sports analytics research, serving team owners, general managers, coaches, fans, and academics. We invite analytical research on any single sport or across sports or esports that seeks to ...
In this perspective paper, we present a high-level, non-technical, overview of the machine learning paradigm that motivates its potential for enhancing sports (performance and business) analytics. We provide a summary of some relevant research literature on the areas in which artificial intelligence and machine learning have been applied to the ...
The Sports Analytics Market was val ued at USD 0.38. billion in 2017 and is expected to reach USD 2.09 billion by. 2022, registering a CAGR of 30.13% over the forecast per iod. of 2018- 2023 ...
The Journal of Quantitative Analysis in Sports (JQAS), an official journal of the American Statistical Association, publishes timely, high-quality peer-reviewed research on the quantitative aspects of professional and amateur sports, including collegiate and Olympic competition. The scope of application reflects the increasing demand for novel methods to analyze and understand data in the ...
This research attempts to gather all the proper analytics used in sports as state-of-the-art performance indicators through sports data in decision making for basketball games, teams and players. Data mining is looking for unknown structure and data analytics [11], [12]. Hence, this could help decision-making and predict uncertain data [13].
Abstract Competitive sports data visualization is an increasingly important research direction in the field of information visualization. It is also an important basis for studying human behavioral pattern and activity habits. In this paper, we provide a taxonomy of sports data visualization and summarize the state-of-the-art research from four aspects of data types, main tasks and ...
The research paper elaborated on the role played by data analytics in the field of sports. Hadoop-based Hortonworks Sandbox 2.2.0 has been used to perform data mining on the collected database. The queries have been written on Query Editor as per the required information to extract information in tabular and graphical format.
Aims & Scope The Journal of Sports Analytics (JSA) aims to be the central forum for the discussion of practical applications of sports analytics research, serving team owners, general managers, coaches, fans, and academics. We invite analytical research on any single sport or across sports that seeks to improve our understanding of the game or strategies for improving a team or a league.
With the rise of this new niche field called Sports Analytics, teams can now perform player evaluations on tons of data that is available. This paper aims to examine the factors that truly indicate the capacity of cricket players to perform at the top-most level - international cricket. Though this research has been carried out on cricket ...
Club Information. The Wharton Sports Analytics & Research Journal (WSARJ) is a vibrant group dedicated to dissecting the sports world from various angles. Primarily, we manage the undergraduate Wharton Sports Journal, reviewing submissions and providing expert, analytical feedback. We also engage in research to deepen our sports knowledge.
This Undergraduate Research Paper is brought to you for free and open access by the Teaching and Learning Center at UW Tacoma Digital Commons. It has been accepted for inclusion in Access*: Interdisciplinary Journal of ... Computer simulations are becoming more popular in the field of sports analytics, especially football (SportsLine, 2019 ...
In this section, an intelligent architecture for sport results prediction is presented, proposing steps of a possible ML framework, and describing the characteristics of the data used for sport results prediction, and how this fits within the framework. Our framework ( Fig. 4) focuses on result prediction for team sports rather than individual ...
15032-3476d-1pass-r02.indd 160 3/25/2020 12:32:49 PM. The future of sport data analytics 161. amount of data collected will continue to grow in the future, and the ease of col-. lecting it will ...
C. Perin & R. V uillemot & C. D. Stolper & J. Stasko & J. Wood & S. Carpendale / Sports Data V isualization ST AR. Figure 1: The 98 sports data visualization articles from both. academics and ...
Literature review. Sports analytics has been applied in different sports including baseball, basketball, football and soccer. Cao compared logistic regression, ANNs, Support Vector Machine (SVM) and Naïve Bayes in predicting game outcomes in the National Basketball Association (NBA) using data from 2005 to 2009 seasons as a training set and the 2010 season as a test set.
RESEARCH. Research projects in sports analytics allow our students to advance their understanding of statistics, dive into interesting datasets, and solve real business problems. WSABI aims to use insights from faculty and community to power the sports industry, both off and on the field of play.
Discover how sports analytics works and how different sports use data to provide meaningful insights. Plus, discover what it takes to become a sports data analyst. ... The global sports market grew from $354.96 billion in 2021 to $496.52 billion in 2022, according to the business research company's research, ...
Updated 1:10 PM PDT, March 2, 2024. BOSTON (AP) — When it comes to artificial intelligence, the sports analytics crowd may be outnumbered. The people who killed the sacrifice bunt and turned NBA games into a 3-point shooting contest aren't quite sure what will happen when AI fully invades sports — whether in the front office or on the field.
The Research Papers Competition is an ideal way to build your reputation within the field of sports analytics. This year's competition will feature six sports tracks - Basketball, Baseball, Soccer, Football, Business of Sports, and Other Sports. Abstract Submissions for SSAC24 are now closed. Abstracts are selected based on the novelty ...
The 10 groundbreaking AI research papers for 2024 collectively symbolize the frontiers of innovation in artificial intelligence. These papers represent the culmination of diverse advancements, from ethical frameworks to quantum computing, and healthcare applications to climate modeling.
In this paper, we have endeavored to discover a novel paradigm of research in cricket analytics known as the timing index, based on a real life IoT-based implementation and case study. ... Journal of Sports Analytics, 1, 19-31. [13] McGinn, E. (2013). The effect of batting during the evening in cricket. Journal of Quantitative Analysis in ...
In this perspective paper, we present a high-level, non-technical, overview of the machine learning paradigm that motivates its potential for enhancing sports (performance and business) analytics. We provide a summary of some relevant research literature on the areas in which artificial intelligence and machine learning have been applied to the ...
This research paper is all about AMC transactions on Computer-Human Interaction. This is a combination of code and documentation. In this research paper, the researchers have Themisto an automated documentation generation system. This explores how human-centered AI systems can support data scientists in Machine Learning code documentation.
We have now released further research, applying new techniques to a much larger dataset, containing nearly 200 million transactions.This work is again co-authored by researchers from the MIT-IBM Watson AI Lab. Rather than identifying transactions made by illicit actors, a machine learning model is trained to identify "subgraphs", chains of transactions that represent bitcoin being laundered.
Chartis Research hosts STORM 2024 on June 12-13, featuring the latest in QuantTech, Insurance Analytics, and more. Chartis Research is pleased to announce the STORM 2024 event - WICZ News
This paper focuses on cyber analytics which revolves around increasing the safety and soundness of the digital infrastructure. A cyber analytics work environment should enable multiple, simultaneous investigations, information foraging, and such others. Through this paper, the researchers attempt to present a prototype workspace for analysts ...